Comprehensive analysis of cuproptosis-related genes involved in prognosis and tumor microenvironment infiltration of colorectal cancer
Highlight box
Key findings
• Two cuproptosis-related genes (CRGs), CDKN2A and DLAT, may play important roles in the development and prognosis of colorectal cancer (CRC).
What is known and what is new?
• CRGs play a crucial role in the occurrence and progression of multiple tumors.
• The prognostic risk model and nomogram based on CDKN2A and DLAT presented excellent performance in predicting overall survival (OS) in CRC.
• CDKN2A and DLAT expression was significantly associated with tumor development, chemotherapy sensitivity and immune cell infiltration in CRC.
What is the implication, and what should change now?
• This study highlights the important roles of CDKN2A and DLAT in the development of CRC.
• This study highlights the importance of CDKN2A and DLAT in predicting prognosis in CRC.
Introduction
Colorectal cancer (CRC) is a cancer of epithelial origin in the large intestine, including colon adenocarcinoma (COAD) and rectum adenocarcinoma (READ) (1). Based on official statistics, the incidence and mortality rates of CRC ranked third among all tumors in 2020 in China and will be constantly increasing in the future (2). Although various treatment options, including radical surgery, chemotherapy, and radiotherapy, have been used to treat patients with CRC, the 5-year survival rate remains very low (3). There is still no reliable biomarker for CRC diagnosis, which is a major cause of poor CRC prognosis (4). Therefore, new biomarkers for improving early diagnosis and guiding the individualized treatment of patients are urgently needed.
Copper is a redox-active transition element in the human body that is primarily absorbed by the stomach and small intestine and excreted into the bile (5). Moreover, as a cofactor for many enzymes, copper is involved in many physiological activities, such as iron collection, intracellular oxidative metabolism, and the regulation of energy conversion (6). The imbalance in copper homeostasis leads to oxidative stress and abnormal autophagy, which can cause various copper-related diseases, such as Menkes, cardiovascular, neurodegenerative, obesity, and Wilson’s disease (7).
Copper has been identified as the significant contributor to the processes of angiogenesis, cell proliferation, and invasion in tumors (8). Many studies have shown that cancer patients not only exhibit elevated copper levels in tumor tissues but generally also display higher copper serum levels (9,10). Studies also have found that elevated copper ion levels in cancer tissues can promote angiogenesis and immune escape, which, in turn, promotes tumor growth and metastasis (11,12). In contrast, using copper chelators can remove excess copper ions and inhibits the neovascularization and the development of tumors (13).
Recently, cuproptosis, a novel cell death pathway, has been identified. Mechanisms of cuproptosis: copper is directly bound to the lipoylated components of the tricarboxylic acid (TCA) cycle, leading to lipoylated protein aggregation and subsequent iron-sulfur cluster protein loss that could cause proteotoxic stress and ultimately cell death (14). Distinct from other forms of cell death, cuproptosis is characterized by copper-dependent, protein misfolding and proteasomal insufficiency. It has been reported that cuproptosis participates in the development and progression of multiple cancers (15). However, the role of cuproptosis in CRC is still unknown. As cuproptosis is a recently identified new type of programmed cell death, its potential as a novel target for CRC immunotherapy remains unclear.
Here, we analyzed the differential expression of cuproptosis-related genes (CRGs) between CRC and normal tissues through bioinformatics analysis and explored the relationship between CRGs, prognosis, and immune infiltration in CRC, aiming to offer novel ideas for the diagnosis and prognosis of CRC. We present this article in accordance with the TRIPOD reporting checklist (available at https://tcr.amegroups.com/article/view/10.21037/tcr-24-546/rc).
Methods
Data sources
RNA sequencing (RNA-seq) and clinical data of patients with CRC were downloaded from The Cancer Genome Atla (TCGA)-COAD/READ (https://portal.gdc.cancer.gov/, accessed on June 18, 2022). Overall, 698 samples were collected from patients with CRC, including 647 tumor and 51 normal samples. Patients without survival information were excluded. Transcripts per million (TPM) were used for normalizing RNA-seq data.
The GSE21510 and GSE41328 datasets were acquired from the Gene Expression Omnibus (GEO) database (https://www.ncbi.nlm.nih.gov/geo/) and were used to validate CDKN2A and DLAT expression. The GSE21510 dataset contained 19 and 25 homogenized cancer and homogenized normal tissues, respectively, and the GSE41328 dataset contained 10 cancer tissues paired with 10 normal tissues.
CRGs (LIPT1, CDKN2A, GLS, FDX1, MTF1, PDHA1, DLAT, LIAS, PDHB, and DLD) were identified in a previous study (14).
The protein expression levels of CDKN2A and DLAT in cancerous and paracancerous tissues were obtained from the Human Protein Atlas database (https://www.proteinatlas.org/).
Differential expression analysis, validation, and genetic alterations analysis
Using the package “limma” in R, differential expressions of CRGs between tumor and normal samples were identified according to thresholds of P<0.05 and |log2FC (fold change)|≥1.
Two datasets, GSE21510 and GSE41328, were obtained from GEO for validation. Box plots were generated to compare CDKN2A and DLAT expression in the two datasets using the R package “ggplot2”. Using the Human Protein Atlas database, CDKN2A and DLAT protein expression levels were compared between cancerous and para-cancerous tissues.
Somatic mutation data of patients with CRC were downloaded from the TCGA database, and changes in the genetic variations of CRGs were assessed using the R package “mafools”.
Functional analysis and protein-protein interaction (PPI) network based on CRGs
Gene Ontology (GO) and Kyoto Encyclopedia of Genes and Genomes (KEGG) enrichment analyses of CRGs were performed using the R package “clusterProfiler”. The PPI network analysis of the CRGs was performed using the GENEMANIA website (16).
Constructing the prognostic signature of CRGs
First, LASSO regression analysis was performed to narrow the gene range among the 10 CRGs. Then, univariate and multivariate Cox regression analyses were performed to identify CRGs related to prognosis and construct a prognostic signature. According to the prognostic signature, the risk score values of patients with CRC were calculated as follows: risk score = ∑(Expi ∗ Coefi), where Expi and Coefi represent the expression levels of identified CRGs and their regression coefficients derived from the multivariate Cox regression model, respectively. Patients with CRC were categorized into low- and high-risk groups based on the median risk score. The “survival” R package was used to compare overall survival (OS) between low- and high-risk groups. Time-dependent receiver operating characteristic (ROC) curves were plotted to evaluate the accuracy of the risk model in OS prediction. Additionally, the “survival” R package was used to compare OS between patients with low and high expressions of CDKN2A or DLAT.
Associations between the expression of CDKN2A and DLAT and different clinical characteristics or prognosis (OS) in different clinical subgroups
The “survival” R package was performed to evaluate the correlation between CDKN2A and DLAT expression and prognosis (OS) in different clinical subgroups of CRC. The box plots were adopted to compare the CDKN2A or DLAT expressions in patients with CRC with different clinical characteristics using the “ggplot2” package in R.
Drug sensitivity analysis
The “pRRophetic” package was used to analyze the drug sensitive prediction based on the Genomics of Drug Sensitivity in Cancer (GDSC) database. The samples’ half-maximal inhibitory concentration (IC50) was estimated by ridge regression, and the difference drug sensitivity between patients with high and low CDKN2A/DLAT expression were compared using the Wilcox test.
Nomogram construction and assessment
Using the “rms” package in R, a nomogram for patients with CRC was constructed by combining the CDKN2A and DLAT data with some clinical characteristics. Calibration cures were used to assess the predictive ability of the nomograms.
Analysis of the correlation with immune infiltration
Infiltration levels of 24 types of immune cells were estimated using single sample gene set enrichment analysis (ssGSEA) in the R package “GSVA.” Spearman’s analysis was performed to explore the correlation between CDKN2A or DLAT and the 24 immune infiltrating cells, and the differences in the level of immune infiltration between the high and low CDKN2A or DLAT expression groups were evaluated using the Wilcoxon rank sum test (17).
The TISIDB database (http://cis.hku.hk/TISIDB) was used to investigate the association between CDKN2A or DLAT expression and molecular or immune subtypes of CRC (18).
The Kaplan–Meier plotter (http://kmplot.com) was used to draw survival curves for patients with CRC with different expressions of CDKNA2 or DLAT based on different immune cell subgroups (19).
Potential immune checkpoint blockade (ICB) response was predicted with tumour immune dysfunction and exclusion (TIDE) algorithm (20).
Ethical statement
The study was conducted in accordance with the Declaration of Helsinki (as revised in 2013).
Statistical analysis
R software (version 4.2.1) was used for all statistical analyses. Comparative analysis between two groups was performed using the independent-sample t-test, Wilcoxon rank-sum test or Welch t-test. Statistical significance was set at P<0.05, and the significance levels were set as *, P<0.05, **, P<0.01, ***, P<0.001, and ns: not significant.
Results
Differential expression and genetic alterations of CRGs in CRC
We cataloged the ten genes that were closely associated with cuproptosis, including PDHA1, GLS, FDX1, LIAS, LIPT1, DLAT, DLD, MTF1, CDKN2A, and PDHB (14). As illustrated in Figure 1A, eight of the 10 CRGs were differentially expressed between CRC and normal tissues. Among these differentially expressed genes, four (CDKN2A, GLS, LIPT1, and PDHA1) were upregulated, whereas four (FDX1, DLD, DLAT and MTF1) were downregulated in CRC. We investigated the correlation between the expression of 10 CRGs, which showed a high correlation (Figure 1B). For example, DLAT was strongly and positively associated with DLD (Figure 1C).
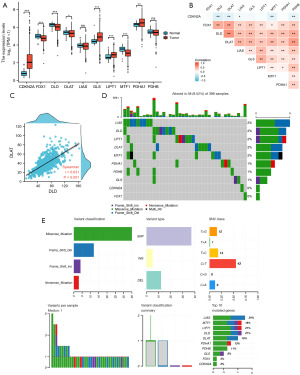
Using the TCGA database, we assessed the somatic mutations of 10 CRGs in CRC. Only 38 of the 399 samples (9.52%) demonstrated genetic variations, and in the primarily mutated genes, LIAS, DLD, LIPT1, DLAT, and MTF1, the frequency was only 2% (Figure 1D). Regarding the mutation classification, missense mutations were the most frequent. Single nucleotide polymorphisms were the most prevalent variant type, and C>T [43] was ranked at the top of the single nucleotide variant (SNV) classes (Figure 1E).
Functional enrichment and PPI network analysis of CRGs in CRC
GO enrichment analysis showed that the ten CRGs were primarily involved in acetyl-CoA biosynthesis from pyruvate, protein lipoylation, and TCA cycle (Figure 2A).
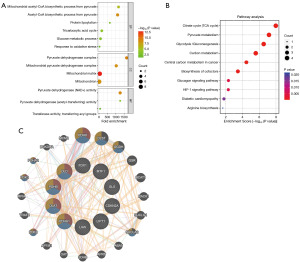
KEGG pathway enrichment analysis revealed that the ten CRGs were mainly implicated in the citrate cycle (TCA cycle), carbon metabolism, pyruvate metabolism, biosynthesis of cofactors, and HIF-1 signaling pathway (Figure 2B).
PPI network analysis was performed to investigate the functional interactions of CRGs, indicating that PDHA1, PDHB, DLAT, and DLD were hub genes (Figure 2C).
Construction of the prognostic risk model of CRGs in CRC
To further investigate the association between CRGs and CRC prognosis, we performed least absolute shrinkage and selection operator (LASSO), univariate, and multivariate Cox regression analyses of CRGs for OS. CDKN2A and DLAT were identified as the prognostic CRGs related to OS (Figure 3A,3B; Table 1). According to the results of OS analysis, CDKN2A and DLAT were selected for the follow-up analysis. Subsequently, based on CDKN2A and DLAT, a prognostic risk score model was established, and the risk score was calculated as follows: risk score = 0.159×CDKN2A+(−0.374)×DLAT. Risk score distributions, survival status, survival time, and CDKN2A and DLAT expression patterns are shown in Figure 3C. After calculating the risk score for each patient with CRC using the above formula, we found that patients with low risk scores had better OS (Figure 3D). Time-dependent ROC curve analysis showed that this risk score model had good prediction accuracy, with area under the curve values of 0.637, 0.652, and 0.687 at 1, 3, and 5 years, respectively (Figure 3E). We also observed that patients with low CDKN2A or high DLAT expression exhibited better OS (Figure 3F,3G).
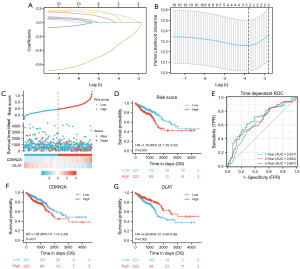
Table 1
Genes | Univariate analysis | Multivariate analysis | |||||
---|---|---|---|---|---|---|---|
Hazard ratio | 95% CI | P value | Hazard ratio | 95% CI | P value | ||
CDKN2A | 1.58 | 1.12–2.25 | 0.01 | 1.53 | 1.08–2.17 | 0.02 | |
DLAT | 0.58 | 0.40–0.82 | 0.002 | 0.6 | 0.42–0.85 | 0.004 |
CRGs, cuproptosis-related genes; CI, confidence interval.
CDKN2A and DLAT are associated with the progression of CRC
To clarify whether CDKN2A and DLAT would influence CRC progression, we analyzed the expressions of CDKN2A and DLAT in CRC with different clinical characteristics. As shown in Figure 4A-4C, Table S1, CDKN2A expression was significantly higher in the N1/N2 stage, pathologic stage III/IV, and lymphatic invasion than in the N0 stage, pathologic stage I/II, and no lymphatic invasion. However, DLAT expression was significantly lower in the N1/N2 stage, pathologic stage III/IV, and lymphatic invasion (Figure 4D-4F, Table S2).
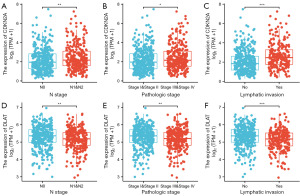
Prognostic value of CDKN2A and DLAT in different clinical subgroups of CRC
We examined the association between CDKN2A or DLAT expression and the prognosis (OS) of patients with CRC in different clinical subgroups. As shown in Figure 5A-5D, a low expression of CDKN2A was correlated with better OS in some clinical subgroups, including the T4 stage, M1 stage, pathologic stage IV, and age >65 years subgroups. However, a high expression of DLAT was associated with better OS in the T3, M0, N2, pathologic stage II, and age >65 years subgroups (Figure 5E-5I).
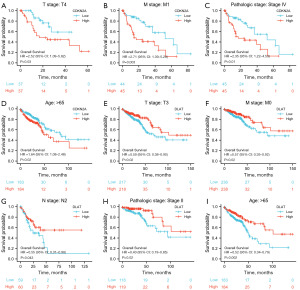
Drug sensitivity analysis between patients with high and low CDKN2A/DLAT expression in CRC
Due to that chemotherapy is a common treatment for CRC, we analyzed the sensitivity for commonly used chemotherapeutic agent between patients with high and low CDKN2A/DLAT expression in CRC. The results showed that the IC50 of 5-fluorouracil and gemcitabine was lower in patients with low CDKN2A expression than high CDKN2A expression (Figures 6A,6B). However, the IC50 of mitomycin C, doxorubicin, and paclitaxel showed no significant difference between patients with low CDKN2A expression and high CDKN2A expression (Figure 6C-6E). In addition, the IC50 of camptothecin, paclitaxel, gemcitabine, cisplatin, mitomycin c, 5-fluorouracil, and doxorubicin was lower in patients with high DLAT expression (Figure 6F-6L).
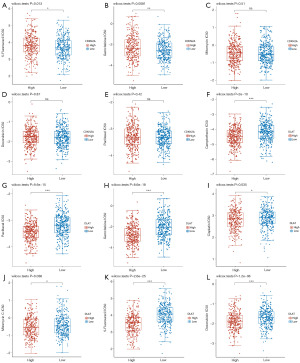
Nomogram development for CRC
We developed a nomogram to predict the individual survival rates of patients with CRC. The univariate and multivariate Cox regression analyses showed that CDKN2A, DLAT, and several clinical characteristics (age, pathological stage, and N, M stage) were independent prognostic predictors of OS (P<0.05) (Figure 7A,7B). Based on the result of multivariate Cox regression, a prognostic nomogram was constructed for predicting the 1-, 3-, and 5-year OS for patients with CRC (Figure 7C). The calibration curves revealed an optimal agreement between the nomogram’s prediction and actual observations (Figure 7D-7F).
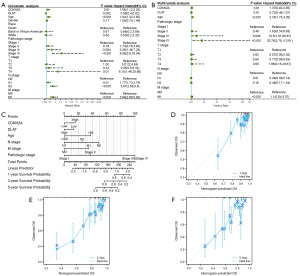
Validation of differential expression of CDKN2A and DLAT in CRC
To confirm the differential expression of CDKN2A and DLAT between CRC and normal tissues, two validation-independent datasets (GSE21510 and GSE41328) were collected. GSE21510 included 19 and 25 homogenized CRC and homogenized normal tissues, respectively, whereas GSE41328 included 10 CRC and paired normal tissues. In both GEO datasets, CDKN2A mRNA levels were significantly higher in CRC tissues than in normal tissues (Figure 8A,8B, Table S3), whereas DLAT mRNA levels were markedly lower (Figure 8C,8D, Table S3).
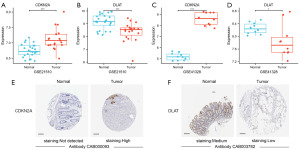
Next, we compared CDKN2A and DLAT protein levels in CRC and normal tissues using the Human Protein Atlas database. CDKN2A protein levels were significantly increased in CRC tissues compared with normal tissues, whereas DLAT protein levels were dramatically reduced (Figure 8E,8F).
Relationship between CDKN2A or DLAT and immune cell infiltration in CRC
First, the infiltration of 24 immune cells in CRC was estimated by the ssGSEA method. Subsequently, we investigated the associations between CDKN2A or DLAT expression and the infiltration of 24 immune cells using Spearman’s analysis. As shown in Figure 9A, the expression of CDKN2A was positively correlated with the infiltration of NK cells, iDCs, NK CD56bright cells, regulatory T cells (Treg), and dendritic cells (DCs), but negatively associated with the infiltration of T helper cells, Tcm cells, and Th2 cells. Meanwhile, the expression of DLAT was positively correlated with T helper cells, Th2 cells, Tcm cells, macrophages, and aDCs and negatively associated with NK CD56bright cells, NK cells, and pDC cells (Figure 9B).
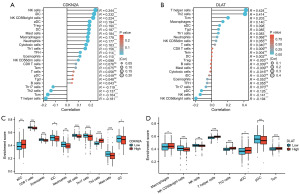
In addition, the enrichment scores of aDCs and Th2 cells was significantly higher in the CDKN2A high expression group than in the CDKN2A low expression group. But the enrichment scores of CD8 T cells, eosinophils, iDC, neutrophils, NK cells, Th17 cells, mast cells, and DCs was significantly higher in the CDKN2A low expression group than in the CDKN2A high expression group (Figure 9C). At the same time, the enrichment scores of Macrophages, T helper cells, Th2 cells, aDCs, and Tcm were markedly higher in the DLAT high expression group than those in the low expression group, while the enrichment scores of NK CD56bright cells, NK cells, and pDC cells were markedly lower (Figure 9D).
Association between CDKN2A or DLAT and immune or molecular subtypes in CRC
We examined the association of molecular and immune subtypes with CDKN2A or DLAT using the TISIDB database to understand the correlation between CDKN2A or DLAT and the immune components of CRC. CDKN2A and DLAT expressions were dramatically correlated with different immune subtypes (C1: wound healing, C2: IFN-gamma-dominant, C3: inflammatory, C4: lymphocyte-depleted, and C6: TGF-b-dominant) (Figure 10A-10D). Regarding COAD, CDKN2A was most expressed in the immune subtype of C6 among the five different immune subtypes, whereas the expression of DLAT was highest in the immune subtype of C2. For READ, CDKN2A and DLAT showed the highest expression in the immune subtypes C3 and C2, respectively.
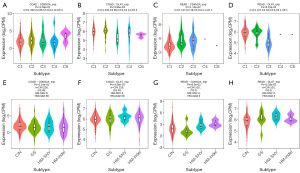
The expression of CDKN2A and DLAT differed in the various molecular subtypes (Figure 10E-10H). For COAD, CDKN2A and DLAT were both highest expressed in the HM-SNV molecular subtype among four different molecular subtypes. For READ, CDKN2A was highest expressed in the HM-indel molecular subtype, whereas DLAT expression was highest in the HM-SNV molecular subtype among four different molecular subtypes.
Prognostic values of CDKN2A and DLAT in CRC based on immune cells
We demonstrated that CDKN2A and DLAT expression levels were related to immune infiltration in CRC, and these two genes were also associated with CRC prognosis. Thus, we hypothesized that CDKN2A and DLAT affect CRC prognosis partly due to immune infiltration.
Therefore, based on CDKN2A and DLAT expression levels in READ, we performed a prognosis (OS) analysis in the related immune cell subgroups using the Kaplan-Meier plotter. We found that low expression of CDKN2A in decreased B cells, enriched eosinophils, and decreased mesenchymal stem cells was correlated with a better prognosis (Figure 11A-11C). Meanwhile, a high expression of DLAT was associated with a better prognosis in enriched B, enriched CD8+ T, decreased mesenchymal stem, enriched natural killer T, and decreased regulatory T cells, respectively (Figure 11D-11H).
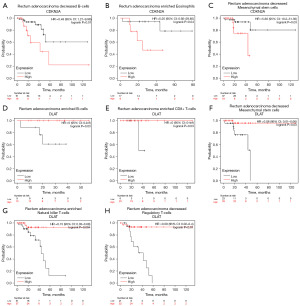
Relationship between CDKN2A/DLAT expression and immunotherapy
The TIDE algorithm was used to predict the effects of immunotherapy. The higher the TIDE score, the greater the likelihood of immune escape and the less effective the Immune checkpoint inhibitors treatment. Our results demonstrated that TIDE score was higher in CDKN2A high expression group than those in the low expression group (Figure 12A). But TIDE score between DLAT high and low expression group had no significance (Figure 12B).
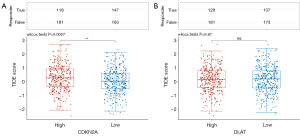
Discussion
CRC is one of the most common malignancies, with poor efficacy and poor prognosis (21). However, clinically useful biomarkers for CRC prognosis that are essential for CRC treatment are currently lacking (22). Cuproptosis is a new form of copper-dependent, oxidative stress-independent, and mito-chondrial induced cell death, and has been revealed to be involved in the development of malignant cancers (23). However, the specific role of cuproptosis in CRC is still unknown. Here, we comprehensively analyzed CRGs’ role in the development and prognosis of CRC.
We investigated the expression signatures and genetic alterations of the 10 CRGs in CRC tissues. Subsequently, CDKN2A and DLAT were identified as independent risk factors for OS and selected for further analysis. Based on CDKN2A and DLAT, a new risk score model and prognostic nomogram were constructed to predict the prognosis of patients with CRC. Further analysis of TME-related cells, and immune cell infiltration revealed that CDKN2A and DLAT affected CRC prognosis via tumor immunity.
Emerging research has revealed that cuproptosis participates in the development and treatment of cancers and that an imbalance of copper homeostasis leads to cancer cell growth and migration (24). In our research, we observed that most CRGs were differentially expressed between CRC and normal tissues. CRG mutations have been identified in up to 9.52% of CRC samples. These results indicated that cuproptosis may serve a major role in CRC and that targeting the cuproptosis-activating pathway is a potential specific target for CRC.
In further analysis, DLAT and CDKN2A were identified as independent risk factors for OS in patients with CRC, and a risk score model comprising these two genes was constructed. We confirmed that the risk score model has excellent predictive performance. CDKN2A is a well-known cell cycle inhibitor involved in regulating cell cycle-formed clusters (25). Increased CDKN2A expression has been strongly associated with the development of multiple tumors (26). CDKN2A promotes CRC metastasis by inducing epithelial-mesenchymal transition (27). A previous study demonstrated that high expression of CDKN2A was associated with poorer outcomes in patients with CRC (28). Consequently, CDKN2A expression can be used as a prognostic marker for CRC (29). In this study, CDKN2A was highly expressed in tumor tissues in CRC, and CRC patients with higher CDKN2A expression had worse prognosis, higher tumor stages, and poorer drug sensitivity. These findings suggest that CDKN2A may promoted tumor malignancy, development, and drug resistance in CRC. As CDKN2A is a well-known cell cycle inhibitor, it is possible that CDKN2A dysregulation may disrupt the normal cell cycle control and oncogenic pathways, leading to uncontrolled growth and aggressiveness of CRC. Further studies are needed to unravel the underlying mechanisms by which CDKN2A promotes CRC progression. The DLAT protein is the E2 subunit of the pyruvate dehydrogenase complex involved in the catabolic glucose pathway and functions as a protein acetyltransferase (30). DLAT is closely related to gastric cancer progression and may be a viable drug target (31). Previous studies have reported that DLAT correlates with COAD prognosis (32,33). The DLAT-associated pathway may be a therapeutic target for non-small cell lung cancer (34). Moreover, the DLAT can be utilized to predict the outcomes of patients with clear-cell renal cell carcinoma (35). Our study indicated that DLAT was lowly expressed in CRC, and lower expression of DLAT predicted a worse prognosis, higher tumor stages, and worse drug sensitivity for patients with CRC. These results suggested that DLAT exerted an inhibitory effect on CRC progression. Since DLAT is a key component of the pyruvate dehydrogenase complex (PDC), which plays a crucial role in glucose metabolism and energy production (30), so the low expression of DLAT in CRC may influence the normal metabolic processes, leading to tumor development. Restoration of DLAT expression or modulation of DLAT-related metabolic pathways could potentially inhibit tumor development and enhance treatment response.
A nomogram is a map of prognostic prediction based on various independent risk factors and is widely used to predict malignant tumor prognosis (36). The nomogram contains more detailed clinical information than the standard tumor-node-metastasis staging system and can provide more accurate estimates of patient survival probability (37). To the best of our knowledge, no previous study has reported the use of CDKN2A and DLAT in predicting CRC prognosis. Here, we first identified CDKN2A, DLAT, and some clinical characteristics as independent prognostic factors for OS. Then, based on these independent prognostic factors, a prognostic nomogram was established for predicting OS. The calibration curve demonstrated the good prediction ability of the prognostic nomogram. Overall, the developed nomogram provides a new and more effective approach to help clinicians better evaluate the outcomes of patients with CRC and select individualizing therapy, which may improve clinical outcomes.
Numerous studies have revealed that infiltrating immune cells in the TME are closely linked to malignant tumor prognosis (38-40). Several studies have demonstrated loss or inactivation of CDKN2A has been associated with reduced infiltration of immune cells into the tumor microenvironment (41,42). At the same time, DLAT expression has been observed to positively correlate with increased infiltration of immune cells, and can potentially promote immune cells recruitment through the modulation of chemokines like CXCL9 and CXCL10, which are critical for immune cell trafficking (43). In the present study, CDKN2A upregulation was associated with decreased infiltration of many immune cells, such as CD8 T cells, NK cells, DCs, and so on, which played important roles in tumor immunity. The reduction infiltration of these immune cells suggested a compromised antitumor immune response. So increased CDKN2A expression may result in reduced immune cell infiltration and compromised tumor immunity. In contrast, DLAT upregulation was associated with enhanced infiltration of Macrophages, T helper cells, Th2 cells, aDCs, and Tcm cells, which indicated a more active immune response against the tumor. Thus, the upregulation of DLAT expression may promote the recruitment and activation of immune cells, leading to a more favorable immune microenvironment for tumor control.
However, our study also has some limitations. First, our data were derived from public databases and lacked verification using large-scale, real-world data. Second, we only used the TCGA dataset to construct and validate the CRC prognostic model and lacked external cohorts from other public databases to validate the model. Finally, the correlation between CRGs and the TME was verified using bioinformatic analysis, which requires further experimental validation.
Overall, we comprehensively investigated the expression features, relationships, and mutational landscapes of the CRGs in CRC. CDKN2A and DLAT may play important roles in the development and prognosis of CRC. The prognostic risk model based on CDKN2A and DLAT presented excellent performance in predicting OS. CDKN2A and DLAT expressions were significantly associated with immune cell infiltration. Thus, our study provides novel insights into personalized prognostication and therapy for patients with CRC.
Conclusions
In conclusion, we comprehensively analyzed CRGs’ roles in CRC. CDKN2A and DLAT play important roles in CRC progression and outcomes and affect its prognosis through tumor immunity. Therefore, CDKN2A and DLAT may serve as novel biomarkers for predicting prognosis and new targets for immunotherapy in CRC.
Acknowledgments
We would like to acknowledge the TCGA database and GEO database for free data use. We would like to thank Editage (www.editage.cn) for English language editing.
Funding: This work was supported by
Footnote
Reporting Checklist: The authors have completed the TRIPOD reporting checklist. Available at https://tcr.amegroups.com/article/view/10.21037/tcr-24-546/rc
Peer Review File: Available at https://tcr.amegroups.com/article/view/10.21037/tcr-24-546/prf
Conflicts of Interest: All authors have completed the ICMJE uniform disclosure form (available at https://tcr.amegroups.com/article/view/10.21037/tcr-24-546/coif). The authors have no conflicts of interest to declare.
Ethical Statement: The authors are accountable for all aspects of the work in ensuring that questions related to the accuracy or integrity of any part of the work are appropriately investigated and resolved. The study was conducted in accordance with the Declaration of Helsinki (as revised in 2013).
Open Access Statement: This is an Open Access article distributed in accordance with the Creative Commons Attribution-NonCommercial-NoDerivs 4.0 International License (CC BY-NC-ND 4.0), which permits the non-commercial replication and distribution of the article with the strict proviso that no changes or edits are made and the original work is properly cited (including links to both the formal publication through the relevant DOI and the license). See: https://creativecommons.org/licenses/by-nc-nd/4.0/.
References
- Lv JL, Tan YJ, Ren YS, et al. Procyanidin C1 inhibits tumor growth and metastasis in colon cancer via modulating miR-501-3p/HIGD1A axis. J Adv Res 2024;60:215-31. [Crossref] [PubMed]
- Morgan E, Arnold M, Gini A, et al. Global burden of colorectal cancer in 2020 and 2040: incidence and mortality estimates from GLOBOCAN. Gut 2023;72:338-44. [Crossref] [PubMed]
- Almuhanna R, Al-Thoubaity F, Almalki K, et al. Clinicopathological Characteristics and Overall 5-Year Survival of Colorectal Cancer: A Retrospective Study. Med Sci (Basel) 2022;10:42. [Crossref] [PubMed]
- Zhang Y, Wang Y, Zhang B, et al. Methods and biomarkers for early detection, prediction, and diagnosis of colorectal cancer. Biomed Pharmacother 2023;163:114786. [Crossref] [PubMed]
- Lu J, Liu X, Li X, et al. Copper regulates the host innate immune response against bacterial infection via activation of ALPK1 kinase. Proc Natl Acad Sci U S A 2024;121:e2311630121. [Crossref] [PubMed]
- Więcek S, Paprocka J. Disorders of Copper Metabolism in Children-A Problem too Rarely Recognized. Metabolites 2024;14:38. [Crossref] [PubMed]
- Fan H, Wang K, Zhao X, et al. Emerging insights into cuproptosis and copper metabolism: implications for age-related diseases and potential therapeutic strategies. Front Aging Neurosci 2024;16:1335122. [Crossref] [PubMed]
- Wang X, Zhu M, Li S, et al. Novel mono-, bi-, tri- and tetra-nuclear copper complexes that inhibit tumor growth through apoptosis and anti-angiogenesis. J Inorg Biochem 2024;250:112403. [Crossref] [PubMed]
- Zhao Q, Yu M, Du X, et al. The Role of Cuproptosis Key Factor FDX1 in Gastric Cancer. Curr Pharm Biotechnol 2024; Epub ahead of print. [Crossref] [PubMed]
- Guan D, Zhao L, Shi X, et al. Copper in cancer: From pathogenesis to therapy. Biomed Pharmacother 2023;163:114791. [Crossref] [PubMed]
- Wang Z, Jin D, Zhou S, et al. Regulatory roles of copper metabolism and cuproptosis in human cancers. Front Oncol 2023;13:1123420. [Crossref] [PubMed]
- Abdolmaleki S, Aliabadi A, Khaksar S. Unveiling the promising anticancer effect of copper-based compounds: a comprehensive review. J Cancer Res Clin Oncol 2024;150:213. [Crossref] [PubMed]
- Wang Y, Tang T, Yuan Y, et al. Copper and Copper Complexes in Tumor Therapy. ChemMedChem 2024;19:e202400060. [Crossref] [PubMed]
- Tsvetkov P, Coy S, Petrova B, et al. Copper induces cell death by targeting lipoylated TCA cycle proteins. Science 2022;375:1254-61. [Crossref] [PubMed]
- Li L, Zhou H, Zhang C. Cuproptosis in cancer: biological implications and therapeutic opportunities. Cell Mol Biol Lett 2024;29:91. [Crossref] [PubMed]
- Franz M, Rodriguez H, Lopes C, et al. GeneMANIA update 2018. Nucleic Acids Res 2018;46:W60-4. [Crossref] [PubMed]
- Wei X, Tang Y, Zheng M, et al. KEAP1 overexpression is correlated with poor prognosis and immune infiltration in liver hepatocellular carcinoma. Front Med (Lausanne) 2024;11:1391843. [Crossref] [PubMed]
- Li C, Zhu X. DEP domain containing 1 as a biomarker for poor prognosis in lung adenocarcinoma. Heliyon 2024;10:e30642. [Crossref] [PubMed]
- Fei Q, Zhang X, Wang S, et al. A pan-cancer characterization of immune-related NFIL3 identifies potential predictive biomarker. J Cancer 2024;15:1271-86. [Crossref] [PubMed]
- Zhang L, Zhang X, Liu H, et al. MTFR2-dependent mitochondrial fission promotes HCC progression. J Transl Med 2024;22:73. [Crossref] [PubMed]
- Meier C, La Rocca G, Nawrot V, et al. Erk Inhibition as a Promising Therapeutic Strategy for High IL-8-Secreting and Low SPTAN1-Expressing Colorectal Cancer. Int J Mol Sci 2024;25:5658. [Crossref] [PubMed]
- Jirapongwattana N, Thongchot S, Pongpaibul A, et al. The combined tumour-based Fascin/Snail and stromal periostin reveals the effective prognosis prediction in colorectal cancer patients. PLoS One 2024;19:e0304666. [Crossref] [PubMed]
- Zheng Z, Fang J, Shen Y, et al. Copper exposure induces trophoblast cell cuproptosis by up-regulating lnc-HZ11. Ecotoxicol Environ Saf 2024;281:116641. [Crossref] [PubMed]
- Zhang MJ, Shi M, Yu Y, et al. Curcuminoid PBPD induces cuproptosis and endoplasmic reticulum stress in cervical cancer via the Notch1/RBP-J/NRF2/FDX1 pathway. Mol Carcinog 2024;63:1449-66. [Crossref] [PubMed]
- Li GG, Chu XF, Xing YM, et al. Baicalin Prevents Colon Cancer by Suppressing CDKN2A Protein Expression. Chin J Integr Med 2024; Epub ahead of print. [Crossref] [PubMed]
- Kwon S, Kim JW, Kim ES, et al. Assessment of TP53 and CDKN2A status as predictive markers of malignant transformation of sinonasal inverted papilloma. Sci Rep 2024;14:14286. [Crossref] [PubMed]
- Shi WK, Li YH, Bai XS, et al. The Cell Cycle-Associated Protein CDKN2A May Promotes Colorectal Cancer Cell Metastasis by Inducing Epithelial-Mesenchymal Transition. Front Oncol 2022;12:834235. [Crossref] [PubMed]
- Dong Y, Zheng M, Wang X, et al. High expression of CDKN2A is associated with poor prognosis in colorectal cancer and may guide PD-1-mediated immunotherapy. BMC Cancer 2023;23:1097. [Crossref] [PubMed]
- Kang N, Xie X, Zhou X, et al. Identification and validation of EMT-immune-related prognostic biomarkers CDKN2A, CMTM8 and ILK in colon cancer. BMC Gastroenterol 2022;22:190. [Crossref] [PubMed]
- Wen H, Qu C, Wang Z, et al. Cuproptosis enhances docetaxel chemosensitivity by inhibiting autophagy via the DLAT/mTOR pathway in prostate cancer. FASEB J 2023;37:e23145. [Crossref] [PubMed]
- Goh WQ, Ow GS, Kuznetsov VA, et al. DLAT subunit of the pyruvate dehydrogenase complex is upregulated in gastric cancer-implications in cancer therapy. Am J Transl Res 2015;7:1140-51. [PubMed]
- Chen S, Cao G, Wu W, et al. Mining novel cell glycolysis related gene markers that can predict the survival of colon adenocarcinoma patients. Biosci Rep 2020;40:BSR20201427. [Crossref] [PubMed]
- Yang W, Wang Y, Huang Y, et al. 4-Octyl itaconate inhibits aerobic glycolysis by targeting GAPDH to promote cuproptosis in colorectal cancer. Biomed Pharmacother 2023;159:114301. [Crossref] [PubMed]
- Chen Q, Wang Y, Yang L, et al. PM2.5 promotes NSCLC carcinogenesis through translationally and transcriptionally activating DLAT-mediated glycolysis reprograming. J Exp Clin Cancer Res 2022;41:229. [Crossref] [PubMed]
- Bian Z, Fan R, Xie L. A Novel Cuproptosis-Related Prognostic Gene Signature and Validation of Differential Expression in Clear Cell Renal Cell Carcinoma. Genes (Basel) 2022;13:851. [Crossref] [PubMed]
- Yang J, Zhou H, Li H, et al. Nomogram incorporating prognostic immune-inflammatory-nutritional score for survival prediction in pancreatic cancer: a retrospective study. BMC Cancer 2024;24:193. [Crossref] [PubMed]
- He Q, Luo Z, Zou H, et al. A prognostic nomogram that includes MPV in esophageal squamous cell carcinoma. Cancer Med 2023;12:20266-76. [Crossref] [PubMed]
- Peng Z, Ren Z, Tong Z, et al. Interactions between MFAP5 + fibroblasts and tumor-infiltrating myeloid cells shape the malignant microenvironment of colorectal cancer. J Transl Med 2023;21:405. [Crossref] [PubMed]
- Yin X, Wu Y, Song J. Characteristics of the immune environment in prostate cancer as an adjunct to immunotherapy. Health Sci Rep 2024;7:e2148. [Crossref] [PubMed]
- Xu Z, Zhao Y, Zhang Y, et al. Prediction of immunotherapy response of bladder cancer with a pyroptosis-related signature indicating tumor immune microenvironment. Front Pharmacol 2024;15:1387647. [Crossref] [PubMed]
- Ko KP, Huang Y, Zhang S, et al. Key Genetic Determinants Driving Esophageal Squamous Cell Carcinoma Initiation and Immune Evasion. Gastroenterology 2023;165:613-628.e20. [Crossref] [PubMed]
- Deng C, Li ZX, Xie CJ, et al. Pan-cancer analysis of CDKN2A alterations identifies a subset of gastric cancer with a cold tumor immune microenvironment. Hum Genomics 2024;18:55. [Crossref] [PubMed]
- Xu L, Wu P, Rong A, et al. Systematic pan-cancer analysis identifies cuproptosis-related gene DLAT as an immunological and prognostic biomarker. Aging (Albany NY) 2023;15:4269-87. [Crossref] [PubMed]