A novel prognostic model based on telomere-related lncRNAs in gastric cancer
Highlight box
Key findings
• The constructed prognostic model based on six long non-coding RNAs (lncRNAs) could predict the prognosis of gastric cancer patients accurately.
What is known and what is new?
• Telomeres are crucial for the growth and spread of cancer cells.
• In this study, we constructed a prognostic model of gastric cancer by telomere-related lncRNA and performed immune correlation analysis.
What is the implication, and what should change now?
• This prognostic model reveals the role of telomeric lncRNA in gastric cancer and suggests new possibilities for the prognosis and treatment of gastric cancer patients, and further mechanistic studies are needed in the future.
Introduction
Gastric cancer is a global health event, especially in East Asia, where it has the fifth highest incidence and third highest mortality rate (1,2). Globally, there were over one million instances of gastric cancer in 2020, which resulted in over 768,000 fatalities. One of the main causes of cancer-associated mortality in China is gastric cancer (3). The incidence and death rate of gastric cancer now exhibit a consistent declining trend. However, as the population ages, there will probably be an increase in the number of gastric cancer cases that come into the clinic. Additionally, some research indicates that the incidence of gastric cancer is rising among young adults, primarily those under 50, which could alter the disease’s epidemiological features and risk factors (2,4). Globally, there is a growing trend in the number of stomach cancer cases and fatalities. East Asia, especially China, has the highest rate of gastric cancer occurrence, death, and disability-adjusted life years (DALYs) of any region (4). By the time gastric cancer manifests symptoms, it is frequently advanced and uncurable (2,3). Recurrence is still rather prevalent at the moment, despite considerable advancements in complete therapy (5). Therefore, we should focus more on gastric cancer and perform a more thorough study of disease biomarkers.
Human telomeres are nucleoprotein complexes that consist of hexameric TTAGGGn DNA sequences and an associated protein complex called shelterin. It is found at the end of a linear chromosome, and to preserve stability and integrity, its nucleoprotein structure functions as a protective cap at the end of the chromosome (6-8). Telomeres become shorter with each division, and when they get shorter than a certain length, the DNA damage response and cellular senescence are triggered, which prevents normal cells from undergoing mitosis (6,8,9). By activating the telomere maintenance mechanism (TMM), cancer cells are able to proliferate indefinitely. However, recent studies indicate that there are two primary mechanisms at work: telomerase activation accounts for 90% of cancer cases, with the alternative lengthening of the telomeres (ALT) pathway accounting for the remaining 10% (6,7). We can also formulate anti-tumor strategies from this. A prospective study by Shi et al. discovered a strong correlation between a shortened cell-free DNA (cfDNA) telomere length and a higher risk of stomach cancer progression (10). According to a study by Wu et al., human telomerase reverse transcriptase (hTERT) in gastric cancer tissues binds to the Sp1 and Gli1 promoters to upregulate Gli1 expression and promote gastric cancer cell invasion and metastasis, and this suggests that the invasion and progression of gastric cancer are influenced by the hTERT/Sp1/Gli1 axis (11). Long non-coding RNA (lncRNA) may directly sponge miR-423-5p and inhibit the expression of SOX12 to regulate the miR-423-5p/SOX12 signaling axis, leading to gastric cancer progression (12).
LncRNAs are a sort of linear non-coding RNAs that are over 200 nucleotides in length, and in most cases, they are not significantly different from messenger RNAs (mRNAs) except that they do not have open reading frames (ORFs) (13). Protein-coding areas are typically thought to be linked to the development of cancer; however, lncRNAs can contribute to cancer by modulating target gene expression and function at the transcriptional, translational, and posttranslational stages to control cancer (13-16). Moreover, lncRNAs have the ability to function as oncogenes or suppressors to control the development and progression of cancer as well as to be markers or prognostic indicators for tumor diagnosis. These findings imply that lncRNAs are important in the development of human cancers, although the precise mechanism underlying their function is still unknown (14,17). Furthermore, earlier research has demonstrated that lncRNAs can be viable targets for therapeutic intervention (18). Jia et al. discovered that via the c-Jun/c-Fos/SREBP1 axis, lncRNA NEAT1 mediates RPRD1B, which in turn stimulates fatty acid metabolism and gastric cancer cell implantation in lymph nodes (19). According to research by Luo et al., drug-resistant gastric cancer cells exhibited high expression of the lncRNA EIF3J-DT, which might trigger autophagy and cause drug resistance in these cells by targeting ATG14 (20). In addition, an abundance of evidence suggests that lncRNAs are involved in other malignant tumors, including tumors of the breast, liver, lung, and colon (15,21). In summary, it is believed that lncRNAs have a significant role in both the pathophysiology of human malignant tumors and medication resistance.
As telomeres also play a pivotal role in the growth, invasion, and even metastasis of gastric cancer, there are currently many studies showing their roles in various cancer categories. However, the number of relevant studies in this area is still comparatively sparse. Consequently, using telomere-related differential genes, our work built a new bioinformatics-based prognostic model that may offer innovative therapeutic targets and biomarkers for the diagnosis, treatment, and prognosis of gastric cancer. We present this article in accordance with the TRIPOD reporting checklist (available at https://tcr.amegroups.com/article/view/10.21037/tcr-24-295/rc).
Methods
Data acquisition
The Cancer Genome Atlas (TCGA; https://www.cancer.gov) provided the transcriptome and clinical statistics of stomach adenocarcinoma (STAD) patients, comprising 375 tumor samples and 32 normal samples. Samples with incomplete data were eliminated. Then we converted the Ensembl ID to gene symbols, such as lncRNAs, protein-coding genes, microRNAs (miRNAs), and the fraction of lncRNAs was filtered from it. The study was conducted in accordance with the Declaration of Helsinki (as revised in 2013).
Identification of telomere-related lncRNAs (TRLs)
We extracted the lncRNAs (TRLs) from 2,093 telomere-related genes (TRGs) that we acquired from the website (http://www.cancertelsys.org/telnet/). Then, using the “limma” R package and setting the R-value >0.6 and P<0.001, Pearson correlation analysis was carried out. Stem from the co-expression analysis of the telomere-associated genes that were downloaded and the lncRNA expression data of the TCGA STAD samples, TRLs associated with gastric cancer were identified.
Differential expression analysis of TRLs
Using the Wilcoxon test, the expression levels of TRLs between STAD and normal stomach tissues were found. Obtaining differentially expressed TRLs needed setting screening criteria such as |log2fold change (FC)| >2 and false discovery rate (FDR) <0.01. The “heatmap” R program was then used to plot the telomere-related differentially expressed lncRNAs (TRDELs) as volcanoes and heatmaps.
Construction and validation of the prognosis model
We randomized STAD patients into two groups, the training group and the test group, to verify the association between TRDELs and overall survival (OS) of gastric cancer patients. In the training group, we took the intersection of TRLs and differentially expressed lncRNAs (DELs) to obtain several lncRNAs. Then we used the “survival” R package to attain fourteen prognostic TRLs of STAD patients by performing univariate Cox regression analysis and defining a P value <0.05 as the screening condition. We subsequently screened thirteen prognostic TRLs using least absolute shrinkage and selection operator (LASSO) Cox regression analysis. Finally, a predictive model composed of six lncRNAs was constructed via multivariate Cox regression analysis. The telomere-related prognostic risk scores were as follows: risk score = expression (lncRNA1k) × coefficient (lncRNA1k) + expression (lncRNA2k) × coefficient (lncRNA2k) + … + expression (lncRNAnk) × coefficient (lncRNAnk). Using the median risk score as the cut-off value, we respectively divide the samples in the training group and testing groups into two groups: high- and low-risk. Additionally, the “survival” and “survmine” R packages were used to draw survival and risk curves so as to explore the predictive value of the six TRL risk models. To assess the predictive accuracy of various clinicopathologic factors and risk scores on survival time, the R package “timeROC” was used to examine the receiver operating characteristic (ROC) of subjects with 1-, 3-, and 5-year survival.
Independent prognostic analysis
We conducted univariate and multivariate independent prognostic analyses to confirm whether the risk score is an independent prognostic element for STAD patients. The variables included risk score, staging, grading, age, and gender.
Establishment of nomogram and calibration curve
Using the “rms” R package, we created a nomogram with different clinicopathologic characteristics and risk scores based on the clinical data of patients with gastric cancer, such as age, gender, tumor, nodes, metastasis (TNM) stage, and grading. This allowed us to assess the patients’ OS at 1-, 3-, and 5-year intervals. Finally, we plotted a calibration curve to show the nomogram model’s predictive ability.
Gene set enrichment analysis
To further explore the potential functions of these genes in different risk subgroups for gastric cancer, we performed Kyoto Encyclopedia of Genes and Genomes (KEGG) enrichment analysis and functional annotation for the high- and low-risk groups by using the “cluster Profiler”, “org.Hs.eg.db”, and “ggplot2” R packages. P<0.05 and FDR <0.05 were considered statistically significant.
Tumor microenvironment (TME) and immune correlation analysis
First of all, we applied the ESTIMATE algorithm in R software to calculate the ESTIMATE score, stromal score, and immune score to estimate the score for each sample and plotted box plots for the scores of the TME in the high- and low-risk groups. Next, we downloaded the file of immune cell infiltration from TIMER 2.0 (http://timer.cistrome.org/) and performed correlation analysis of risk score and immune cells in R software, including CIBERSORT, TIMER, XCELL, QUANTISEQ, MCPcounter, EPIC and CIBERSORT, and the results were visualized to form bubble plots. Based on the immune cell composition, the samples were initially split into two groups: high and low expression. Then, survival analysis was used to display the survival curves, with a P value of less than 0.05 serving as the screening criterion. The gene set files relevant to immune checkpoints were acquired from earlier research, and we utilized R to identify the genes that showed significant variations between the high- and low-risk groups, with a P value of less than 0.05 serving as the screening criterion. The outcome was displayed in the form of another box plot.
Drug sensitivity
Using the “oncoPredict” R package, we calculated the sensitivity scores of each small molecule for patients in the high- and low-risk groups and visualized the results by “ggplot2” and “ggpubr” R packages. We were able to visualize two-dimensional (2D) images of the four most sensitive medications by applying the PubChem (https://pubchem.ncbi.nlm.nih.gov) website.
Principal component analysis (PCA)
Based on the expression of six TRLs, the samples were divided into three subtypes using the “ConsensusClusterPlus” R program. The survival curves were then plotted to determine whether patient survival varied across the three subtypes. The correlation between the three subtypes and the high- and low-risk groups was determined and shown using the “galluvial” R tool. We then performed PCA to show their distributions in two dimensions and explored whether these lncRNAs differed between the high- and low-risk groups and between samples of different subtypes.
Statistical analysis
All statistical analyses were carried out using R version 4.2.1 (Institute for Statistics and Mathematics, Vienna, Austria; https://www.r-project.org), survival, survmine, timeROC, rms, cluster Profiler, org.Hs.rg.db, ggplot2, oncoPredict, ggpubr, ConsensusClusterPlus, ggalluvial). A P value <0.05 was regarded as statistically significant.
Results
Identification of telomere-associated differential genes
From the TCGA database, we were able to acquire data concerning 407 patients, comprising 375 samples of gastric cancer and 32 samples of normal tissue. Next, 4,611 lncRNAs and 19,211 mRNAs were isolated. From the website, we were able to retrieve 2,093 TRGs. The correlation between the two sets of quantitative data can be examined using Pearson analysis. After using Pearson analysis to examine the relationship between TRLs and lncRNAs at an R>0.6 and P value of <0.001, we finally obtained 2,277 TRLs. In addition, considering (|log2FC| >2, FDR <0.01) as a criterion, we were able to identify 426 lncRNAs that showed differential expression in STAD samples compared to normal tissue samples. The heatmap showed where these 426 DELs were distributed (Figure 1A). A volcano plot was utilized to display the 165 TRDELs, which were obtained by integrating TELs and DELs. Of these, 127 were up-regulated lncRNAs, and 38 were down-regulated lncRNAs (Figure 1B).
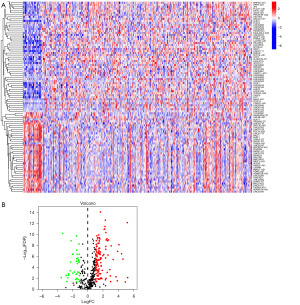
Construction and validation of prognostic risk assessment model
We examined the predictive ability of TRDELs by univariate Cox regression analysis using OS data of STAD patients from the TCGA database. Fourteen TRDELs were screened for prognostic relevance at the P<0.05 criterion, comprising ten high-risk lncRNAs (MIR100HG, GAS1RR, LINC01537, CFAP61-AS1, LINC01579, DIRRC1, LINC01094, LINC02185, LINC02716, ADAMTS9-AS1) and four low-risk lncRNAs (RABGAP1L-IT, POLH-AS1, DBH-AS1, REPIN-AS1) (Figure 2A). And we showed the distribution of these fourteen genes in tumor samples and normal samples with heatmap (Figure 2B). Then, to prevent overfitting prognostic features and exclude prognosis-related false-positive TRDELs, thirteen TRDELs were produced using LASSO Cox regression analysis. The results are shown using cvfit and lambda curves (Figure 2C,2D). After that, we performed multivariate Cox regression analysis to separate the six lncRNAs from the thirteen TRDELs listed above. Consequently, we utilized these six lncRNAs to construct the prognostic model, which included LINC01537, CFAP61-AS1, DIRC1, RABGAP1L-IT1, DBH-AS1, and REPIN1-AS1 (Table 1).
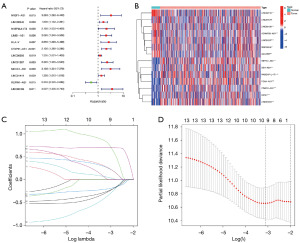
Table 1
ID | Coef |
---|---|
LINC01537 | 1.84132432609438 |
‘CFAP61-AS1’ | 0.478893727155692 |
DIRC1 | 0.971832877233469 |
‘RABGAP1L-IT1’ | −0.636261238970348 |
‘DBH-AS1’ | −0.983852856245592 |
‘REPIN1-AS1’ | −0.487934342697213 |
LncRNA, long non-coding RNA; coef, coefficient.
We applied the following formula to calculate the risk score for all STAD patients: Risk score = 1.84 × LINC01537 + 0.48 × CFAP61-AS1 + 0.97 × DIRC1 − 0.64 × RABGAP1L-IT1 − 0.98 × DBH-AS1 − 0.49 × REPIN1-AS1. We classified the training group and testing group, as well as the entire sample, into two groups, high-risk and low-risk, respectively, using the median value of the risk scores as the cut-off value. We discovered that there were differences between the high- and low-risk groups, confirming the accuracy of the constructed prognostic model. Additionally, the number of fatalities from gastric cancer increased along with the risk score (Figure 3A-3C). The Kaplan-Meier (K-M) curve revealed that the low-risk group had a better prognosis than the high-risk group, indicating that a higher risk score was associated with a lower prognosis in patients with STAD (Figure 3D). In addition, it is shown by the time-dependent ROC curve that the area under the curve (AUC) of OS was 0.689 at 1 year, 0.667 at 3 years, and 0.746 at 5 years (Figure 4A). In addition, we produced an ROC curve that demonstrated that the risk score’s predictive accuracy was higher than that of other clinicopathologic indicators (Figure 4B). The aforementioned findings show that the prognosis for patients with gastric cancer is well predicted by our prognostic model, which was developed by utilizing these six TRDELs.
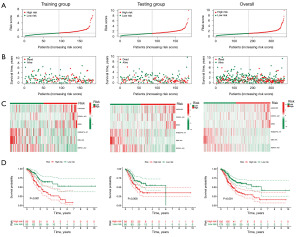
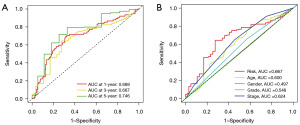
Independent prognostic analysis of risk score
We carried out univariate and multivariate independent prognostic analyses to further confirm whether risk score and other clinical features were independent prognostic factors. According to the univariate regression analysis, the patient’s prognosis was highly correlated with age (P=0.004), stage (P<0.001), and risk score (P=0.02) (Figure 5A). The results of multivariate regression analysis suggested that risk score (P=0.001), stage (P<0.001), and age (P<0.001) could all be independent predictors of prognosis (Figure 5B). Independent prognostic factors, including age and risk score (Figure 5C), were plotted as calibration curves, suggesting that the nomogram was able to predict the 1-, 3-, and 5-year survival rates of STAD patients with relative accuracy (Figure 5D). These results suggest that our nomogram with risk score can reliably predict OS in patients with STAD.
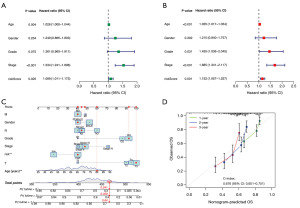
Gene set enrichment analysis
The high- and low-risk groups in the test group were subjected to KEGG functional enrichment analysis, with differentially expressed genes |log2FC| >1 and P<0.05 chosen as screening criteria. Using KEGG analysis, we were able to generate bubbles for the top eighteen pathways along with the genes that were associated with them. The results indicated that the focal adhesion and calcium signaling pathways were the pathways to which these genes were most closely linked (Figure 6A,6B). We next conducted gene set enrichment analysis (GSEA) enrichment analysis on the high- and low-risk groups to investigate the biological effects of these genes on gastric cancer in greater depth. This technique can be utilized in addition to KEGG pathway enrichment research. The findings showed that dilated cardiomyopathy, extracellular matrix (ECM) receptor interaction, focal adhesion, hypertrophic cardiomyopathy (hcm), and vascular smooth muscle contraction were the primary enriched pathways in the high-risk group (Figure 6C). The major pathways enriched in the low-risk group were allograft rejection, autoimmune thyroid disease, DNA replication, primary immunodeficiency, and the T-cell receptor signaling pathway (Figure 6D).
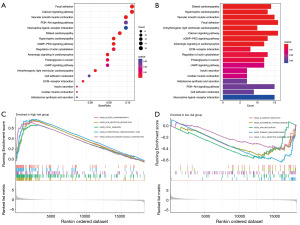
TME and immune correlation analysis
The interaction of surrounding mesenchymal stromal cells and immune cells with tumor cells forms the complex integrated system known as the TME and the TME is considered to be important for drug sensitivity, prognosis, and regression of tumors. Using the ESTIMATE technique, we computed the immune, stromal, and ESTIMATE scores. The TME score revealed that the high-risk group was superior to the low-risk group in terms of the ESTIMATE and stromal scores, but there was no discernible benefit in terms of the immune score (Figure 7A). Various softwares, including CIBERSORT, TIMER, XCELL, QUANTISEQ, MCPcounter, EPIC, CIBERSORT-ABS, and CIBERSORT, were applied to figure the bubble plot. The results showed a correlation between the risk score and tumor-infiltrating immune cells, indicating that the risk score was positively correlated with monocytes, M2 macrophages, eosinophils, and neutrophils and were negatively correlated with CD8+ T cells, CD4+ memory T cells activated, follicular helper T cells, regulatory T cells (Tregs), and M1 macrophages (Figure 7B, Table S1). Additionally, we continued with immune cell survival analysis based on the composition of various immune cells, such as macrophages, follicular helper T cells, cancer-associated fibroblasts, endothelial cells, and uncharacterized cells. The samples were divided into two groups, one for the high-risk and the other for low-risk. The results showed that there was a significant difference in the patient’s survival times between the two groups, with the low-risk group’s prognosis being better than the prognosis of the high-risk group in the immune cells of macrophages, endothelial cells, and cancer-associated fibroblasts, and the high-risk group’s prognosis being better in the immune cells of follicular helper T cells and uncharacterized cells (Figure 7C). At the same time, in both the high- and low-risk groups, the large majority of immunological checkpoints demonstrated noticeably distinct degrees of activation, with the low-risk group often exhibiting a higher degree of activation (Figure 7D).
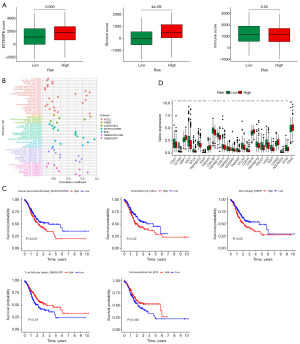
Drug sensitivity
Drug sensitivity analysis was undertaken on STAD patients in the TCGA database. We identified 73 small molecule complexes that were significantly different in the high- and low-risk groups in this study. The top four small molecule complexes with the smallest P values included MK-1775 (P=8e−09), ML323 (P=3.1e−09), oxaliplatin (P=8.7e−09), and PF-4708671 (P=2.9e−10) (Figure 8A-8D). We then applied the PubChem website to visualize the 2D images of these four drugs (Figure 8E-8H). These analyses led us to the conclusion that these small molecule complexes could have therapeutic value for individuals with STAD and could offer treatment options for patients with gastric cancer.
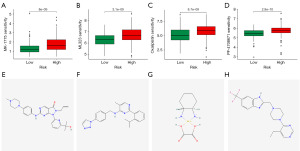
PCA
In accordance with the expression of the lncRNAs for which the models were built, we conducted a consensus clustering analysis to classify gastric cancer patients in the TCGA dataset. The optimal clustering variable was 3 (Figure 9A-9D), while the total cohort of gastric cancer patients was evenly split among clusters 1, 2, and 3. After performing survival analysis on samples from each cluster, we found that there were notable variations in patient survival among the three clusters, with cluster 2 offering the best prognosis (Figure 9E). The Sankey plot (Figure 9F) shows that cluster 1 is mostly distributed in the high-risk group, while clusters 2 and 3 are mostly distributed in the low-risk group. The t-distributed stochastic neighbor embedding (t-SNE) results showed three clusters. In addition, we used PCA to verify that the risk group and clusters had different PCAs (Figure 9G-9H). These results show that, according to the expression of modelled genes, it is possible to distinguish different subtypes. In most of the immune checkpoints, cluster 2 expressed more activity, such as CD160, TNFSF14, and TNFRSF25 (Figure 9I). Applying drug susceptibility analysis, we found 15 drugs that showed significantly different half-maximal inhibitory concentration (IC50) values between the different clusters (Figure 9J).
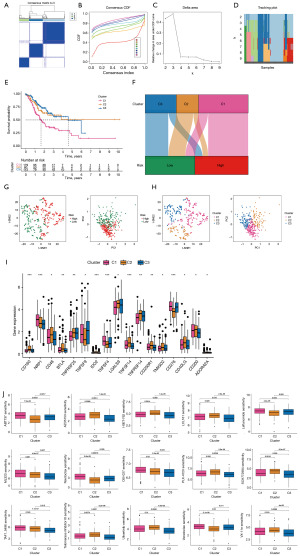
Discussion
Gastric cancer is a common type of malignant tumor, and it remains a main cause of death in China, despite recent declines in both incidence and death rates. Due to its subtle symptoms and discomfort in the stomach, patients frequently ignore gastric cancer in its early stages, and when it is discovered, it is usually discovered in a late stage. This is because the clinical manifestation of the disease is frequently not evident. The development of gastric cancer is significantly influenced by telomeres, and while TRLs and cancer have received less attention, telomere length has been the subject of numerous studies. Due to its subtle symptoms and discomfort in the stomach, patients frequently ignore gastric cancer in its early stages, and when it is discovered, it is usually discovered in a late stage. Telomeres play an essential role in the development of gastric cancer, and many studies have focused on the effect of telomere length on cancer, while the correlation between TRLs and cancer has been less studied.
The TCGA database was utilized in this study to filter 2,277 TRDELs for gastric cancer. Next, we conducted Cox and LASSO regression analyses, which allowed us to filter out six TRLs. We then used these six lncRNAs (LINC01537, CFAP61-AS1, DIRC1, RABGAP1L-IT1, DBH-AS1, and REPIN1-AS1) to establish a prognostic model, and we validated the model’s efficacy by separating the high- and low-risk groups using the median value of the risk score as the cut-off value. By directly binding to RIPK4, reducing RIPK4 binding to TRIM25, and lowering its ubiquitination level, LINC01537 promotes gastric metastasis and carcinogenesis. This, in turn, stimulates the NF-κB pathway in gastric cancer cells (22). Initially, thought to be the gene spanning the 2q33 breakpoint in chromosomal translocations, the DIRC1 gene has been shown through experiments to inhibit AKT/mTOR signaling. It is now thought that abnormalities in the PI3K/AKT/mTOR pathway are linked to the development and prognosis of gastric cancer, indicating that the DIRC1 gene may function as a marker for the disease (23-25). Regrettably, no conclusive research has been done to establish a connection between gastric cancer and RABGAP1L-IT1. It has been demonstrated that the lncRNA DBH-AS1, which is transcribed from chromosome 9q34 and possesses a polyadenylated tail, contributes to the growth of cancerous tumors such as hepatocellular carcinomas, esophageal carcinomas, and pancreatic carcinomas (26-28). It has been observed that CFAP61-AS1 and REPIN1-AS1 are helpful in determining the prognosis of STAD patients (29-31).
TME is a general term for all non-tumor components and their metabolites in tumor tissues, which mainly include extracellular mesenchyme, fibroblasts, immune cells and endothelial cells. Tumor cells can interact with surrounding cells through the cardiovascular system and lymphatic systems. Via the circulatory and lymphatic systems, tumor cells can communicate with surrounding cells. The TME can also participate in immunosuppression and angiogenesis processes, both of which are extensively involved in the development, invasion, metastasis, drug resistance, and other stages of tumor growth (32-34). Targeting TME in targeted therapy offers more benefits than directly targeting cancer cells since the genomes of cancer cells are unstable and prone to drug resistance (35). Furthermore, some studies have shown that the TME may have an impact on the prognosis of disease (34,36). For this reason, understanding the TME is crucial for the prognosis of STAD. In the TME, one of the very important types of cells is immune cells, of which the prominent ones are macrophages (33). Surprisingly, the TME can take advantage of the alteration of macrophages to play opposite roles at different stages, which can promote tumor progression and mediate anti-tumor effects (37-39). Tumor antigens produced by genetic mutations have the potential to trigger an immune response during tumor development. In addition, immune cells have the ability to penetrate the TME and engage in the regulation of tumor progression (40). Nonetheless, the depletion and functional inhibition of immune cells allow cancer cells to elude immune monitoring, and certain research has demonstrated a strong correlation between the TME and immunological escape in gastric cancer. On the other hand, cancer cells can spread quickly by eluding immune surveillance (32). Over the past few decades, immunotherapy has ignited new hope for the treatment of gastric cancer (41). Cancer immunotherapy is primarily classified into two categories: active and passive immunity. Active immunity is the use of vaccines, chimeric antigen receptors, and other methods to strengthen the immune response against tumor cells, whereas passive immunity is the use of in vitro synthesized immune components such as monoclonal antibodies. Today, the majority of treatment for gastric cancer is surgery-based comprehensive care because radiation has a low sensitivity to the disease. Currently, immunotherapy for gastric cancer is mainly built on cytotoxic immune cells, monoclonal antibodies, and gene transfer vaccines, and immunotherapy clinical trials have now yielded some promising results (42,43). With the rapid development in the field of immune checkpoint inhibition, the therapeutic options for gastric cancer are becoming increasingly abundant. Immune checkpoint inhibition can be used as a new targeted therapeutic option for advanced gastric cancer, and in advanced gastric adenocarcinoma, the combination of first-line treatment with nivolumab and chemotherapy improves the OS of patients with programmed cell death protein 1 (PD-L1)-positive disease (44). Immune checkpoint inhibitors (ICIs) have gradually become a breakthrough in advanced gastric cancer and have shown anti-tumor effects (41). According to our research, these six TRDELs are linked to cells that partially infiltrate the immune system, which could lead to new developments in immunotherapy. Furthermore, four small molecules with high differentials were filtered out: PF-4708671, ML323, oxaliplatin, and MK-1775. The results indicated that these small molecules may be important for treating patients with gastric cancer, which may be a novel approach to the disease treatment. Based on the expression of six lncRNAs, we conducted a cluster analysis of TRLs in gastric cancer and identified three clusters. According to K-M analysis, cluster 2 had the best chance of success. In addition, among the majority of immunological checkpoints, cluster 2 exhibited the highest activation. We also screened fifteen drugs based on these three clusters, which may be helpful in the treatment of gastric cancer.
The study’s strength is in the way it built a prognostic model of gastric cancer using telomere-related differential genes to predict the disease’s prognosis, look into the lncRNAs’ possible biological functions, examine the relationship between these lncRNAs and immune infiltration, and screen for four potential therapeutics. Our study, however, has a few limitations and restrictions. First, more validation of the study’s findings through trials is needed because the lack of clinical samples in this research leads to the unknown role of these TRLs in the treatment of gastric cancer. Second, it is still unknown how these six TRDELs in gastric cancer operate biologically and how they work.
Conclusions
In this study, we constructed a prognostic model of gastric cancer using six TRDELs, LINC01537, CFAP61-AS1, DIRC1, RABGAP1L-IT1, DBH-AS1, and REPIN1-AS1, which were capable of predicting the prognosis of gastric cancer patients, and screened four small molecule compounds. The results showed that they had the potential to predict the prognosis of gastric cancer patients, and it was found that the effects of these genes on gastric cancer progression might be related to immune infiltration, and the four small molecule compounds screened might play an impact on the treatment of gastric cancer. However, the results need to be further verified by applying clinical trials in the future.
Acknowledgments
Funding: This work was supported by
Footnote
Reporting Checklist: The authors have completed the TRIPOD reporting checklist. Available at https://tcr.amegroups.com/article/view/10.21037/tcr-24-295/rc
Peer Review File: Available at https://tcr.amegroups.com/article/view/10.21037/tcr-24-295/prf
Conflicts of Interest: All authors have completed the ICMJE uniform disclosure form (available at https://tcr.amegroups.com/article/view/10.21037/tcr-24-295/coif). The authors have no conflicts of interest to declare.
Ethical Statement: The authors are accountable for all aspects of the work in ensuring that questions related to the accuracy or integrity of any part of the work are appropriately investigated and resolved. The study was conducted in accordance with the Declaration of Helsinki (as revised in 2013).
Open Access Statement: This is an Open Access article distributed in accordance with the Creative Commons Attribution-NonCommercial-NoDerivs 4.0 International License (CC BY-NC-ND 4.0), which permits the non-commercial replication and distribution of the article with the strict proviso that no changes or edits are made and the original work is properly cited (including links to both the formal publication through the relevant DOI and the license). See: https://creativecommons.org/licenses/by-nc-nd/4.0/.
References
- Sung H, Ferlay J, Siegel RL, et al. Global Cancer Statistics 2020: GLOBOCAN Estimates of Incidence and Mortality Worldwide for 36 Cancers in 185 Countries. CA Cancer J Clin 2021;71:209-49. [Crossref] [PubMed]
- Smyth EC, Nilsson M, Grabsch HI, et al. Gastric cancer. Lancet 2020;396:635-48. [Crossref] [PubMed]
- Ajani JA, D'Amico TA, Bentrem DJ, et al. Gastric Cancer, Version 2.2022, NCCN Clinical Practice Guidelines in Oncology. J Natl Compr Canc Netw 2022;20:167-92. [Crossref] [PubMed]
- The global, regional, and national burden of stomach cancer in 195 countries, 1990-2017: a systematic analysis for the Global Burden of Disease study 2017. Lancet Gastroenterol Hepatol 2020;5:42-54. [Crossref] [PubMed]
- Joshi SS, Badgwell BD. Current treatment and recent progress in gastric cancer. CA Cancer J Clin 2021;71:264-79. [Crossref] [PubMed]
- Yuan X, Dai M, Xu D. Telomere-related Markers for Cancer. Curr Top Med Chem 2020;20:410-32. [Crossref] [PubMed]
- Shay JW, Wright WE. Telomeres and telomerase: three decades of progress. Nat Rev Genet 2019;20:299-309. [Crossref] [PubMed]
- Shay JW. Role of Telomeres and Telomerase in Aging and Cancer. Cancer Discov 2016;6:584-93. [Crossref] [PubMed]
- Gao J, Pickett HA. Targeting telomeres: advances in telomere maintenance mechanism-specific cancer therapies. Nat Rev Cancer 2022;22:515-32. [Crossref] [PubMed]
- Shi Y, Zhang Y, Zhang L, et al. Telomere Length of Circulating Cell-Free DNA and Gastric Cancer in a Chinese Population at High-Risk. Front Oncol 2019;9:1434. [Crossref] [PubMed]
- Wu L, Wang S, Tang B, et al. Human telomerase reverse transcriptase (hTERT) synergistic with Sp1 upregulate Gli1 expression and increase gastric cancer invasion and metastasis. J Mol Histol 2021;52:1165-75. [Crossref] [PubMed]
- Wang J, Wu M, Chang L, et al. The lncRNA TERC promotes gastric cancer cell proliferation, migration, and invasion by sponging miR-423-5p to regulate SOX12 expression. Ann Transl Med 2022;10:963. [Crossref] [PubMed]
- Quinn JJ, Chang HY. Unique features of long non-coding RNA biogenesis and function. Nat Rev Genet 2016;17:47-62. [Crossref] [PubMed]
- Yan H, Bu P. Non-coding RNA in cancer. Essays Biochem 2021;65:625-39. [Crossref] [PubMed]
- Chi Y, Wang D, Wang J, et al. Long Non-Coding RNA in the Pathogenesis of Cancers. Cells 2019;8:1015. [Crossref] [PubMed]
- Sanchez Calle A, Kawamura Y, Yamamoto Y, et al. Emerging roles of long non-coding RNA in cancer. Cancer Sci 2018;109:2093-100. [Crossref] [PubMed]
- Matsui M, Corey DR. Non-coding RNAs as drug targets. Nat Rev Drug Discov 2017;16:167-79. [Crossref] [PubMed]
- Ling H, Fabbri M, Calin GA. MicroRNAs and other non-coding RNAs as targets for anticancer drug development. Nat Rev Drug Discov 2013;12:847-65. [Crossref] [PubMed]
- Jia Y, Yan Q, Zheng Y, et al. Long non-coding RNA NEAT1 mediated RPRD1B stability facilitates fatty acid metabolism and lymph node metastasis via c-Jun/c-Fos/SREBP1 axis in gastric cancer. J Exp Clin Cancer Res 2022;41:287. [Crossref] [PubMed]
- Luo Y, Zheng S, Wu Q, et al. Long noncoding RNA (lncRNA) EIF3J-DT induces chemoresistance of gastric cancer via autophagy activation. Autophagy 2021;17:4083-101. [Crossref] [PubMed]
- Singh D, Assaraf YG, Gacche RN. Long non-coding RNA mediated drug resistance in breast cancer. Drug Resist Updat 2022;63:100851. [Crossref] [PubMed]
- Zhong GY, Tan JN, Huang J, et al. LncRNA LINC01537 Promotes Gastric Cancer Metastasis and Tumorigenesis by Stabilizing RIPK4 to Activate NF-κB Signaling. Cancers (Basel) 2022;14:5237. [Crossref] [PubMed]
- Li Z, Yang AJ, Wei FM, et al. Significant association of DIRC1 overexpression with tumor progression and poor prognosis in gastric cancer. Eur Rev Med Pharmacol Sci 2018;22:8682-9. [PubMed]
- Ying J, Xu Q, Liu B, et al. The expression of the PI3K/AKT/mTOR pathway in gastric cancer and its role in gastric cancer prognosis. Onco Targets Ther 2015;8:2427-33. [Crossref] [PubMed]
- Fattahi S, Amjadi-Moheb F, Tabaripour R, et al. PI3K/AKT/mTOR signaling in gastric cancer: Epigenetics and beyond. Life Sci 2020;262:118513. [Crossref] [PubMed]
- Bao J, Chen X, Hou Y, et al. LncRNA DBH-AS1 facilitates the tumorigenesis of hepatocellular carcinoma by targeting miR-138 via FAK/Src/ERK pathway. Biomed Pharmacother 2018;107:824-33. [Crossref] [PubMed]
- Lin ZX, Zhu LH, Huang JY, et al. Paclitaxel-resistant related lncRNA DBH-AS1 promotes the proliferation and invasion of esophageal cancer. Eur Rev Med Pharmacol Sci 2022;26:8903-13. [PubMed]
- Ye X, Wang LP, Han C, et al. Increased m(6)A modification of lncRNA DBH-AS1 suppresses pancreatic cancer growth and gemcitabine resistance via the miR-3163/USP44 axis. Ann Transl Med 2022;10:304. [Crossref] [PubMed]
- Zeng J, Li M, Dai K, et al. A Novel Glycolysis-Related Long Noncoding RNA Signature for Predicting Overall Survival in Gastric Cancer. Pathol Oncol Res 2022;28:1610643. [Crossref] [PubMed]
- Luo L, Li L, Liu L, et al. A Necroptosis-Related lncRNA-Based Signature to Predict Prognosis and Probe Molecular Characteristics of Stomach Adenocarcinoma. Front Genet 2022;13:833928. [Crossref] [PubMed]
- Zeng C, Liu Y, He R, et al. Identification and validation of a novel cellular senescence-related lncRNA prognostic signature for predicting immunotherapy response in stomach adenocarcinoma. Front Genet 2022;13:935056. [Crossref] [PubMed]
- Liu Y, Li C, Lu Y, et al. Tumor microenvironment-mediated immune tolerance in development and treatment of gastric cancer. Front Immunol 2022;13:1016817. [Crossref] [PubMed]
- Arneth B. Tumor Microenvironment. Medicina (Kaunas) 2019;56:15. [Crossref] [PubMed]
- Pottier C, Wheatherspoon A, Roncarati P, et al. The importance of the tumor microenvironment in the therapeutic management of cancer. Expert Rev Anticancer Ther 2015;15:943-54. [Crossref] [PubMed]
- Xiao Y, Yu D. Tumor microenvironment as a therapeutic target in cancer. Pharmacol Ther 2021;221:107753. [Crossref] [PubMed]
- Jiang Y, Wang C, Zhou S. Targeting tumor microenvironment in ovarian cancer: Premise and promise. Biochim Biophys Acta Rev Cancer 2020;1873:188361. [Crossref] [PubMed]
- Vitale I, Manic G, Coussens LM, et al. Macrophages and Metabolism in the Tumor Microenvironment. Cell Metab 2019;30:36-50. [Crossref] [PubMed]
- Jin MZ, Jin WL. The updated landscape of tumor microenvironment and drug repurposing. Signal Transduct Target Ther 2020;5:166. [Crossref] [PubMed]
- Pitt JM, Marabelle A, Eggermont A, et al. Targeting the tumor microenvironment: removing obstruction to anticancer immune responses and immunotherapy. Ann Oncol 2016;27:1482-92. [Crossref] [PubMed]
- Zhang Y, Zhang Z. The history and advances in cancer immunotherapy: understanding the characteristics of tumor-infiltrating immune cells and their therapeutic implications. Cell Mol Immunol 2020;17:807-21. [Crossref] [PubMed]
- Jin X, Liu Z, Yang D, et al. Recent Progress and Future Perspectives of Immunotherapy in Advanced Gastric Cancer. Front Immunol 2022;13:948647. [Crossref] [PubMed]
- Xie J, Fu L, Jin L. Immunotherapy of gastric cancer: Past, future perspective and challenges. Pathol Res Pract 2021;218:153322. [Crossref] [PubMed]
- Zhao Q, Cao L, Guan L, et al. Immunotherapy for gastric cancer: dilemmas and prospect. Brief Funct Genomics 2019;18:107-12. [Crossref] [PubMed]
- Högner A, Moehler M. Immunotherapy in Gastric Cancer. Curr Oncol 2022;29:1559-74. [Crossref] [PubMed]