Pathomics signatures and cuproptosis-related genes signatures for prediction of prognosis in patients with hepatocellular carcinoma
Highlight box
Key findings
• A nomogram model based on pathomics signatures and the genomics signatures could effectively predict the survival probability of hepatocellular carcinoma patients. The calibration curves further demonstrated the good predictive capability of the nomogram model.
What is known and what is new?
• Cuproptosis is a newly discovered type of programmed cell death that has been shown to be closely related to the occurrence and progression of malignant tumors.
• The new of this study is to integrate pathomic features and genomic features to analyze the prognosis of patients, providing a basis for clinicians to formulate patient diagnosis and treatment plans and whole-process management.
What is the implication, and what should change now?
• With the advent of whole slide images scanning technology, pathological images are gradually being digitized, and on this basis, computational pathology and the accumulation of a large number of tumor samples with molecular and histopathological data are generated, enabling researchers to study the relationship between tumor morphological characteristics and various omics data. Thus, it can make up for the deficiency of genomic information assessment of local tumor biopsy samples caused by tumor heterogeneity, and lay the foundation for the realization of tumor precision medicine.
Introduction
Primary liver cancer is among the most prevalent malignant tumors and ranks as the third leading cause of cancer-related deaths according to Global Cancer Statistics 2020 (1). Hepatocellular carcinoma (HCC) is the most common type of liver cancer, accounting 70–85% of all cases. Treatment decisions for HCC hinge upon tumor characteristics, disease stage, liver function, and patient age. Usual treatment options include local ablation, surgical resection or liver transplantation, catheter-based locoregional treatment, kinase and immune checkpoint inhibitors (2,3). Due to the heterogeneity of HCC, it leads to drug resistance, metastasis, and disease progression in patients (4-8). Consequently, current treatment outcomes are suboptimal, impacting overall survival (OS). Therefore, there is an urgent need for a new prognostic model to improve the accuracy for predicting the prognosis of HCC patients.
HCC is a highly heterogeneous tumor with a complex tumor microenvironment (TME) (7,8). In addition to confirmed clinical factors, there are many factors that can predict HCC, including cuproptosis-related genes (9,10), which have good predictive effects, indicating the clinical predictive potential of genetic signature. Haematoxylin and eosin (H&E)-stained slides provide intuitive images of the TME and tumor heterogeneity. Evaluation of H&E-stained slides by experienced pathologists is crucial for determining tumor-node-metastasis (TNM) staging and histological classification of HCC in clinical practice. However, the emergence of pathomics has revolutionized the application of traditional pathology in medical research and clinical practice. Pathomics is the fusion of digital pathology and artificial intelligence (11,12), which evaluates the diagnosis, treatment, and prognosis of tumors by extracting, screening, and analyzing the data features from pathological images. Pathomics features provide microstructural information of TME, which can complement tumor heterogeneity and enhance the predictive capability of existing models. Therefore, we assume that the pathgenomic model can effectively predict the prognosis of HCC.
By using the least absolute shrinkage and selection operator (LASSO) regression (13), multiple features can be integrated into a single feature, potentially significantly improving prediction performance. In the study, we constructed a competing-risk nomogram by integrating pathomics signatures (PS) and genomics signatures (GS) to predict the OS of HCC patients. We present this article in accordance with the TRIPOD reporting checklist (available at https://tcr.amegroups.com/article/view/10.21037/tcr-24-350/rc).
Methods
Patients and database
Comprehensive data of HCC patients were downloaded from The Cancer Genome Atlas (TCGA) database (https://portal.gdc.cancer.gov/), including publicly available gene expression profiles data, clinical parameters (follow-up data, age, T, N and M status) and whole slide images (WSIs). The inclusion criteria were as follows: (I) histologically diagnosed HCC patients; (II) complete clinical and follow-up information were available; (III) available digital pathology sections. Exclusion criteria were as follows: (I) WSIs were blurred or heavily discolored; (II) the patient’s survival time was less than 30 days. A total of 315 qualified samples were obtained from the TCGA database and randomly assigned to the training set (n=200) and the validation set (n=115). The study was conducted in accordance with the Declaration of Helsinki (as revised in 2013).
Framework of study
The framework of this study is shown in Figure 1, including (I) the construction of PS composed of multiple pathomics features extracted from WSIs, (II) the construction of GS composed of multiple genomics features, (III) the construction and validation of nomogram.
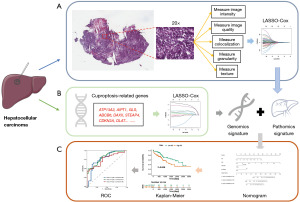
Pathomics feature extraction
We utilized AperioImageScope software (version 12.4.6) to magnify WSIs by 20×. The location of tumor tissue in HCC images was diagnosed and mapped independently by two experienced and renowned pathologists. In case of disagreement, it was reviewed and determined by one or more additional pathologists. This resulted to a typical 900×900 pixel screenshot.
Quantitative pathomics features were extracted from selected screenshots using CellProfiler software (version 4.0.7). The “UnmixColors” module separated H&E-stained images into haematoxylin and eosin-stained grayscale images, and the “ColorToGray” module converted them into grayscale images. Then the “MeasureColocalization” module was used to calculate the correlation between the colocalization and intensity of each hematoxylin and eosin stained image. Each module measures three grayscale images, and 294 pathological features were obtained. The detailed measurement process is depicted in Figure 1.
Screening of prognostic genes and signature construction
Twenty-five cuproptosis-related genes were collected from the published literatures (9,14) to construct prognostic models, including ATP13A2, MTF1, DBT, F5, NLRP3, LIPT1, NFE2L2, GLS, ABCB6, PDHB, COX17, CP, TFRC, LIAS, ALB, SNCA, DAXX, STEAP4, DLD, CDKN2A, SLC31A1, DBH, LIPT2, FDX1, DLAT (Table S1).
We included 25 genes and 294 pathomics features in LASSO-Cox regression to narrow down the range of candidate genes and pathomics features to establish a prognostic model. Finally, 11 pathomics features and 8 genes and their coefficients were retained, and the penalty parameter (λ) was determined by the minimum criterion. In this study, the optimal value of λ was determined by measuring partial likelihood deviation through a 10-fold cross-validation of the minimum criterion. PS and GS were constructed through linear combinations of selected features, and the PS and GS of the validation set were computed directly from the formulas obtained in the training set.
Nomogram development and validation
First, the clinical parameters (age, gender, T stage, N stage and M stage), PS and GS were analyzed by univariate Cox regression, and variables with a P value <0.05 were subsequently included in multivariate Cox regression analysis. Finally, a nomogram for prognosis was generated and applied to the validation set to assess discrimination and calibration. OS was assessed by generating time-related receiver operating characteristic (ROC) curves and calibration curves to compare the consistency between predicted and actual survival.
Statistical analysis
All statistical analyses were conducted using R studio (version 4.2.3, http://www.R-project.org). The Kaplan-Meier method was used to construct survival curves and the log-rank test was used to compare. Univariate and multivariate analyses were conducted using Cox regression analysis to calculate the hazard ratio (HR) with 95% confidence interval (CI). The LASSO-Cox regression method was applied using the “glmnet” package. The development, validation, and performance evaluation of the prognostic nomogram were carried out using the “rms” package. The time-dependent ROC curves were plotted using the “timeROC” package. Survival analyses were computed using the “survminer” package. All tests were two-tailed and P<0.05 was considered statistically significant.
Results
Signature construction
LASSO-Cox was used to deeply select pathomics features and genomics features, and the subset of the optimal features depended on the choice of lambda value, and we used 10-fold cross validation to find the optimal lambda value, resulting in 11 pathomics features and 8 genomics features (Figure 2). In the training set, the values of the obtained features and corresponding regression coefficients generated in LASSO-Cox were used to generate the formulas for PS and GS, respectively. The formulas are shown in the Tables 1,2.
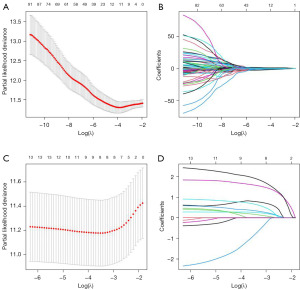
Table 1
GS | Coef |
---|---|
ATP13A2 | 0.6898 |
LIPT1 | 0.2437 |
GLS | 0.2022 |
ABCB6 | 1.4530 |
DAXX | 0.0785 |
STEAP4 | −0.1882 |
CDKN2A | 0.4530 |
DLAT | 1.6553 |
GS, genomics signatures.
Table 2
PS | Coef |
---|---|
Granularity_11_OrigGray | −0.2866 |
Granularity_13_Eosin | −0.7408 |
Granularity_4_Hematoxylin | 0.9904 |
Granularity_5_OrigGray | −1.3719 |
ImageQuality_MaxIntensity_Hematoxylin | −0.5796 |
ImageQuality_PercentMaximal_Hematoxylin | −0.3831 |
ImageQuality_ThresholdOtsu_OrigGray_2W | 1.1958 |
Intensity_PercentMaximal_Hematoxylin | −0.0306 |
Texture_Contrast_Hematoxylin_3_00_256 | −0.0037 |
Texture_Contrast_Hematoxylin_3_02_256 | −0.1456 |
Texture_Contrast_OrigGray_3_02_256 | −0.7125 |
PS, pathomics signatures.
Association of signatures with prognosis
The cut-off values of PS and GS were determined based on the median for each set, and patients were divided into high- or low-risk groups. The Kaplan-Meier survival curves of the training and validation sets divided into high or low PS are presented in Figure 3A,3B. The Kaplan-Meier survival curves for the training and validation sets stratified by high or low GS are shown in Figure 3C,3D. The time-dependent ROC curve showed good survival prediction value in both training set and validation set. Kaplan-Meier survival analysis showed statistically significant differences in survival time between high- and low-risk subgroups in the training set and validation datasets (PS: P=0.003 and <0.001, respectively; GS: P=0.008 and 0.004, respectively).
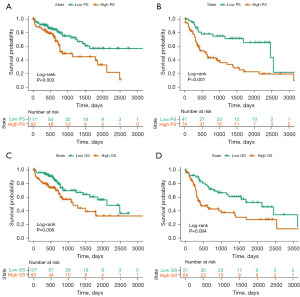
Development and validation of nomogram
In univariate Cox regression analysis, age, gender, T stage, N stage and M stage, PS, GS were significantly associated with OS (Figure 4A). After adjusting confounding factors, it was found that PS, GS, M stage and gender were independent predictors of OS in the multivariate Cox regression analysis (Figure 4B).
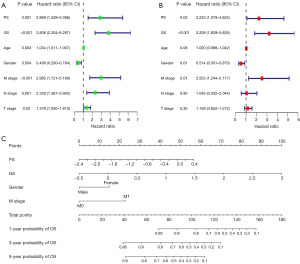
We used 4 independent predictors to create a prognostic nomogram to predict the OS in HCC patients (Figure 4C). Furthermore, the time-dependent ROC curve showed good survival prediction value in both training set and validation set (Figure 5A,5B). The area under the ROC curves at 1, 3 and 5 years was 0.750 (95% CI: 0.645–0.856), 0.830 (95% CI: 0.741–0.911) and 0.870 (95% CI: 0.774–0.958) in the training set and the validation set was 0.780 (95% CI: 0.689–0.873), 0.810 (95% CI: 0.719–0.899), and 0.760 (95% CI: 0.637–0.875), showing the wonderful performance of the nomogram. Furthermore, the calibration curves also showed that the nomogram model had good prediction ability (Figure 5C,5D).
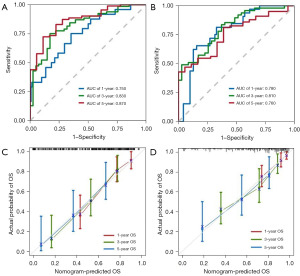
Discussion
In clinical practice, accurate prognostic factors are essential for risk stratification and overall management of HCC patients. Pathological diagnosis serves as the primary method for diagnosing HCC, while the prognosis depends on TNM staging and the Barcelona Clinic Liver Cancer (BCLC) staging (15). The TNM staging system utilizes tumor size, metastasis status, and pathological findings as prognostic indicators, which are vital for early prognosis and assessing surgical outcomes, albeit with limitations for advanced prognostication. The BCLC staging system provides a comprehensive assessment of HCC prognosis, considering factors such as nutritional status, tumor size, metastasis status, liver function parameters, and Okuda staging. However, research indicates that its predictive capability lags behind other models (16,17). A new prognostic model is urgently needed to improve the accuracy of prognostic assessment in HCC patients. In this study, the data of HCC patients in the TCGA database were used to construct PS and GS to predict the prognosis of HCC patients. We found that after risk stratification based on PS and GS, patients with higher risk scores had poorer outcomes. Additionally, a nomogram model based on PS and GS was developed and validated, demonstrating favorable predictive performance. This model could assist clinicians in proactive OS prediction and is pivotal for devising treatment strategies and optimizing overall patient care.
Pathomics, with the assistance of artificial intelligence, converts tissue sections into digital, high-fidelity data, for conducting quantitative and high-throughput studies from digital pathological sections. It subsequently establishes predictive models for pathological diagnosis and patient prognosis. This new technology with broad prospects has attracted more and more attention and has been applied to various types of tumor diagnosis, including bladder cancer (18), breast cancer (19), non-small cell lung cancer (20), and melanoma (21). The microscopic information provided by traditional pathologic methods is incomplete for this change. Pathomics can help overcome the limitations of subjective visual assessment and integrate multiple biomarkers (22). And it can also capture the microscopic structure of the tumor, providing characteristics of the cells and microenvironment within the tumor lesion. Previous research has demonstrated that pathomics can not only predict the prognosis of tumors but also guide their treatment (23). The key of pathomics is the extraction of pathological features, so far there is no expert consensus on the extraction method of pathological features (11,12). Two main research methods exist in pathomics: deep neural network-based methods and hand-crafted features. In this study, CellProfiler software was used to extract quantitative pathological features and deep machine learning was integrated to predict the prognosis of HCC patients. CellProfiler is a free, open-source software developed by computer biologist Anne Carpenter that enables biologists in all fields to create quantitative, repeatable image analysis workflows that measure images in batches (24). The software has been used for digital pathology analysis (25,26). Compared to complex deep learning segmentation and model building methods, its processing is more generic. Features extracted by hand are more advantageous in terms of interpretability and clinical practicality. In this study, PS generated based on digital pathological features also had a good prognostic prediction effect [univariate Cox regression analysis: HR =2.869 (95% CI: 1.529–5.386), P=0.001; multivariate Cox regression analysis: HR =2.233 (95% CI: 1.078–4.625)].
With the development of microarray technology and high-throughput sequencing technology, we can now identify key genes related to tumor prognosis and progression through bioinformatics analysis. This has led to the discovery of many prognostic markers and the establishment of various prognostic models (27,28). In this study, we selected prognostic genes with predictive performance based on bioinformatics and previous studies (9,10,14). First, 25 cuproptosis-related genes were selected from the TCGA database, and a prognostic model of HCC associated with 8 cuproptosis-related genes was constructed by LASSO regression analysis. Cuproptosis is a newly discovered type of programmed cell death that has been shown to be closely related to the occurrence and progression of malignant tumors (29). Different from the types of cell death such as necrotic apoptosis, pyrodeath, autophagy and ferroptosis, cuproptosis is a newly discovered regulatory mode of cell death. It mainly occurs through the accumulation of copper ions directly binding to the lipidized components of the tricarboxylic acid (TCA) cycle, leading to cell death (29). Although current treatment strategies can significantly improve the prognosis of HCC patients, the application of these therapies is still limited by tumor heterogeneity and tumor drug resistance. Therefore, it is of great significance to study the role of cuproptosis-related genes in HCC to improve the prognosis of HCC patients. GS was found to be an independent predictor of HCC prognosis in this study [univariate Cox regression analysis: HR =3.806 (95% CI: 2.304–6.287), P<0.001; multivariate Cox regression analysis: HR =3.208 (95% CI: 1.829–5.626), P<0.001].
Cancer morphology is influenced by genetic drivers, and computational pathology methods typically use tissue images such as entire slide images as input to predict clinical or genetic features. Therefore, the comprehensive analysis of pathological features and genomic data provides a feasible way to explore the potential mechanism of the tumor (30,31). Therefore, in this study, we constructed a nomogram model to predict prognostic risk of HCC patients based on PS and GS and internally verified it. The combined prediction effect was good and like other studies on prediction models, ROC curves were used to demonstrate the prediction ability of the nomogram model in this study. The AUC values for 1-, 3-, and 5-year OS prediction were 0.750, 0.830 and 0.870. In the validation set, the AUC scores of 1-, 3- and 5-year OS were 0.780, 0.810 and 0.760, respectively. The nomogram showed its great potential for guiding decision-making in HCC patients at different stages.
There are some limitations in this study. Considering that HCC is a typical polygenic disease, we applied only 8 cuproptosis-related genes in this study to establish the prognostic model may result in the omission of other genes that could accurately predict the prognosis of HCC. Future prospective studies can include additional clinical prognostic factors and other genes. In addition, WSIs may show some color heterogeneity, which may impact feature extraction and data analysis.
Conclusions
In conclusion, we established a nomogram combining genomics and pathomics to predict prognostic in HCC patients, thereby providing guidance for the exploration of more personalized treatment and prognosis assessment.
Acknowledgments
We sincerely thank the TCGA database for providing data and SMART (https://smart.servier.com/) for providing free vector images.
Funding: None.
Footnote
Reporting Checklist: The authors have completer the TRIPOD reporting checklist. Available at https://tcr.amegroups.com/article/view/10.21037/tcr-24-350/rc
Peer Review File: Available at https://tcr.amegroups.com/article/view/10.21037/tcr-24-350/prf
Conflicts of Interest: All authors have completed the ICMJE uniform disclosure form (available at https://tcr.amegroups.com/article/view/10.21037/tcr-24-350/coif). The authors have no conflicts of interest to declare.
Ethical Statement: The authors are accountable for all aspects of the work in ensuring that questions related to the accuracy or integrity of any part of the work are appropriately investigated and resolved. The study was conducted in accordance with the Declaration of Helsinki (as revised in 2013).
Open Access Statement: This is an Open Access article distributed in accordance with the Creative Commons Attribution-NonCommercial-NoDerivs 4.0 International License (CC BY-NC-ND 4.0), which permits the non-commercial replication and distribution of the article with the strict proviso that no changes or edits are made and the original work is properly cited (including links to both the formal publication through the relevant DOI and the license). See: https://creativecommons.org/licenses/by-nc-nd/4.0/.
References
- Sung H, Ferlay J, Siegel RL, et al. Global Cancer Statistics 2020: GLOBOCAN Estimates of Incidence and Mortality Worldwide for 36 Cancers in 185 Countries. CA Cancer J Clin 2021;71:209-49. [Crossref] [PubMed]
- Yang JD, Hainaut P, Gores GJ, et al. A global view of hepatocellular carcinoma: trends, risk, prevention and management. Nat Rev Gastroenterol Hepatol 2019;16:589-604. [Crossref] [PubMed]
- Rebouissou S, Nault JC. Advances in molecular classification and precision oncology in hepatocellular carcinoma. J Hepatol 2020;72:215-29. [Crossref] [PubMed]
- Torbenson MS. Hepatocellular carcinoma: making sense of morphological heterogeneity, growth patterns, and subtypes. Hum Pathol 2021;112:86-101. [Crossref] [PubMed]
- Hytiroglou P, Bioulac-Sage P, Theise ND, et al. Etiology, Pathogenesis, Diagnosis, and Practical Implications of Hepatocellular Neoplasms. Cancers (Basel) 2022;14:3670. [Crossref] [PubMed]
- El Jabbour T, Lagana SM, Lee H. Update on hepatocellular carcinoma: Pathologists' review. World J Gastroenterol 2019;25:1653-65. [Crossref] [PubMed]
- Quail DF, Joyce JA. Microenvironmental regulation of tumor progression and metastasis. Nat Med 2013;19:1423-37. [Crossref] [PubMed]
- Li L, Wang H. Heterogeneity of liver cancer and personalized therapy. Cancer Lett 2016;379:191-7. [Crossref] [PubMed]
- Qi X, Guo J, Chen G, et al. Cuproptosis-Related Signature Predicts the Prognosis, Tumor Microenvironment, and Drug Sensitivity of Hepatocellular Carcinoma. J Immunol Res 2022;2022:3393027. [Crossref] [PubMed]
- Chen Y, Tang L, Huang W, et al. Identification of a prognostic cuproptosis-related signature in hepatocellular carcinoma. Biol Direct 2023;18:4. [Crossref] [PubMed]
- Bera K, Schalper KA, Rimm DL, et al. Artificial intelligence in digital pathology - new tools for diagnosis and precision oncology. Nat Rev Clin Oncol 2019;16:703-15. [Crossref] [PubMed]
- Niazi MKK, Parwani AV, Gurcan MN. Digital pathology and artificial intelligence. Lancet Oncol 2019;20:e253-61. [Crossref] [PubMed]
- Tibshirani R. The lasso method for variable selection in the Cox model. Stat Med 1997;16:385-95. [Crossref] [PubMed]
- Li Y, Zeng X. A novel cuproptosis-related prognostic gene signature and validation of differential expression in hepatocellular carcinoma. Front Pharmacol 2023;13:1081952. [Crossref] [PubMed]
- Bruix J, Reig M, Sherman M. Evidence-Based Diagnosis, Staging, and Treatment of Patients With Hepatocellular Carcinoma. Gastroenterology 2016;150:835-53. [Crossref] [PubMed]
- Tannus RK, Almeida-Carvalho SR, Loureiro-Matos CA, et al. Evaluation of survival of patients with hepatocellular carcinoma: A comparative analysis of prognostic systems. PLoS One 2018;13:e0194922. [Crossref] [PubMed]
- Chen ZH, Hong YF, Lin J, et al. Validation and ranking of seven staging systems of hepatocellular carcinoma. Oncol Lett 2017;14:705-14. [Crossref] [PubMed]
- Chen S, Jiang L, Zheng X, et al. Clinical use of machine learning-based pathomics signature for diagnosis and survival prediction of bladder cancer. Cancer Sci 2021;112:2905-14. [Crossref] [PubMed]
- Ehteshami Bejnordi B, Veta M, Johannes van Diest P, et al. Diagnostic Assessment of Deep Learning Algorithms for Detection of Lymph Node Metastases in Women With Breast Cancer. JAMA 2017;318:2199-210. [Crossref] [PubMed]
- Yu KH, Zhang C, Berry GJ, et al. Predicting non-small cell lung cancer prognosis by fully automated microscopic pathology image features. Nat Commun 2016;7:12474. [Crossref] [PubMed]
- Brinker TJ, Hekler A, Enk AH, et al. Deep neural networks are superior to dermatologists in melanoma image classification. Eur J Cancer 2019;119:11-7. [Crossref] [PubMed]
- Cyll K, Ersvær E, Vlatkovic L, et al. Tumour heterogeneity poses a significant challenge to cancer biomarker research. Br J Cancer 2017;117:367-75. [Crossref] [PubMed]
- Feng L, Liu Z, Li C, et al. Development and validation of a radiopathomics model to predict pathological complete response to neoadjuvant chemoradiotherapy in locally advanced rectal cancer: a multicentre observational study. Lancet Digit Health 2022;4:e8-e17. [Crossref] [PubMed]
- McQuin C, Goodman A, Chernyshev V, et al. CellProfiler 3.0: Next-generation image processing for biology. PLoS Biol 2018;16:e2005970. [Crossref] [PubMed]
- Stirling DR, Swain-Bowden MJ, Lucas AM, et al. CellProfiler 4: improvements in speed, utility and usability. BMC Bioinformatics 2021;22:433. [Crossref] [PubMed]
- Carpenter AE, Jones TR, Lamprecht MR, et al. CellProfiler: image analysis software for identifying and quantifying cell phenotypes. Genome Biol 2006;7:R100. [Crossref] [PubMed]
- Chen X, Wang L, Hong L, et al. Identification of Aging-Related Genes Associated With Clinical and Prognostic Features of Hepatocellular Carcinoma. Front Genet 2021;12:661988. [Crossref] [PubMed]
- Chen S, Zhang E, Guo T, et al. A novel ferroptosis-related gene signature associated with cell cycle for prognosis prediction in patients with clear cell renal cell carcinoma. BMC Cancer 2022;22:1. [Crossref] [PubMed]
- Kahlson MA, Dixon SJ. Copper-induced cell death. Science 2022;375:1231-2. [Crossref] [PubMed]
- Ma L, Peterson EA, Shin IJ, et al. An advanced molecular medicine case report of a rare human tumor using genomics, pathomics, and radiomics. Front Genet 2023;13:987175. [Crossref] [PubMed]
- Li X, Yu X, Tian D, et al. Exploring and validating the prognostic value of pathomics signatures and genomics in patients with cutaneous melanoma based on bioinformatics and deep learning. Med Phys 2023;50:7049-59. [Crossref] [PubMed]