Establishment and validation of a prediction model for gastric cancer with perineural invasion based on preoperative inflammatory markers
Highlight box
Key findings
• We successfully established and validated a prediction model for gastric cancer (GC) patients with perineural invasion (PNI) based on hematological indicators.
What is known and what is new?
• Previous prediction models have focused on the predictive value of common tumor marker in the occurrence of PNI in GC, and have been tested on limited sample sizes.
• In this study, we established a prediction model for PNI in GC based on peripheral blood inflammatory markers by multivariate logistic regression analysis.
What is the implication, and what should change now?
• When constructing a prognostic model, it is crucial to consider the patients’ treatment mode, as this will enhance the accuracy, reliability, specificity, and sensitivity of the model. Our model can provide valuable insights for evaluating the risk of PNI in GC patients. This approach may serve as an adjunctive tool in clinical decision-making processes regarding PNI.
Introduction
Gastric cancer (GC) is the fifth most common cancer and the third leading cause of cancer-related death globally (1). In China, it is also a prevalent digestive tract tumor and a major cause of cancer mortality (2). Due to the lack of early symptoms in GC patients, 80–90% of patients are diagnosed with advanced-stage disease, with a 5-year overall survival of no more than 30% (3). Previous studies have confirmed that tumor size, histologic type, age, lymphovascular invasion, and perineural invasion (PNI), distant metastasis are important prognostic factors for GC (4-7).
PNI, first described in the 1800s by Russian and French pathologists, is characterized by cancer cell infiltration into the perineurium or neural fascicles, indicating an early step in local tumor spread (8,9). More and more studies have affirmed that PNI indicates a high invasive ability of cancer cells, and its prognostic value has been shown in various tumors, including colon cancer, squamous cell carcinoma of the tongue, pancreatic cancer, and prostate cancer (10-13). In GC, PNI is a common and significant independent prognostic factor affecting tumor recurrence and patient survival post-resection (14,15). The presence of PNI correlates significantly with tumor size, tumor, node, metastasis (TNM) stage, histological type, blood vessel invasion, and lymph node metastasis (14,16).
At present, paraffin-embedded tissue sections and histopathology of surgical specimens are used to evaluate PNI. The invasiveness of biopsy and the efficiency and timeliness PNI detection status may limit clinical decision-making (17). Preoperative identification of PNI may facilitate patient stratification and formulation of optimal treatment options. Several studies have documented the predictive value of medical images as an adjunctive diagnostic instrument in identifying PNI in GC (17-19). Consequently, considering that laboratory indicators also have the advantages of high cost-effectiveness and easy operation as ancillary assessments, their potential application in the predicting PNI in GC should not be disregarded. In recent years, some laboratory indicators have been confirmed to be significantly related to GC patients with PNI. For instance, carcinoembryonic antigen (CEA) ≥5 µg/L [odds ratio (OR) =5.870, 95% confidence interval (CI): 3.281–10.502] was shown to be a significant independent risk factor of PNI in advanced GC, with an area under the curve (AUC) value of 0.731 in a nomogram model (20). Immunoglobulin A (IgA) level (OR=1.61, 95% CI: 1.03–2.61) was found in the multivariable regression model to be one of the independent predictors of PNI in 261 GC patients (21). In addition, carbohydrate antigen 125 (CA125) was also shown to effectively indicate PNI positivity in gastric adenocarcinoma samples of 135 GC patients, with an AUC value of 0.714 (95% CI: 0.625–0.804) (22). Nevertheless, the above studies almost all reported the predictive value of common tumor markers in the occurrence of PNI in GC, and the limited sample size. Nowadays, accumulating studies have confirmed that systemic inflammatory response is associated with the tumor microenvironment (TME), serving a pivotal role in tumor progression (23). In the TME, various inflammatory cells such as lymphocytes, macrophages, monocytes, and neutrophils are present (24). During the onset of inflammation, conventional blood indicators such as neutrophils, lymphocytes, and platelets count often become abnormal (25,26). Studies have demonstrated that cellular inflammatory markers, including the neutrophil-to-lymphocyte ratio (NLR), platelet-to-lymphocyte ratio (PLR), and lymphocyte-to-monocyte ratio (LMR) are dysregulated in a variety of tumors and are often considered predictors of poor prognosis or malignant progression (27-29). Besides, fibrinogen may be involved in tumor-specific inflammatory response and is significantly elevated during an infection or inflammatory disease. Interestingly, malignant tumor cells can partially express fibrinogen (30,31). A recent study revealed that the systemic inflammation response index (SIRI = neutrophil count × monocyte count/lymphocyte count) is an independent risk factors for PNI in advanced GC, with an AUC value of 0.707 (95% CI: 0.653–0.761), sensitivity of 53.60%, and specificity of 87.9% (32). Despite this, the correlation between inflammatory markers in peripheral blood and PNI in GC is still unclear.
Therefore, this study was undertaken to establish a prediction model for PNI in GC based on peripheral blood inflammatory markers by multivariate logistic regression analysis. In addition, we assessed the prediction model using receiver operating characteristic-AUC (ROC-AUC), calibration curve analysis, and decision curve analysis (DCA). We present this article in accordance with the TRIPOD reporting checklist (available at https://tcr.amegroups.com/article/view/10.21037/tcr-24-481/rc).
Methods
Study patients and processing
The study was conducted in accordance with the Declaration of Helsinki (as revised in 2013) and approved by the Clinical Research Ethics Committee of Jiangsu Cancer Hospital (approval number: #KY-2024-012). The requirement for informed consent was waived in this retrospective study. A total of 1,195 patients with GC from the hospital were enrolled between October 2020 and December 2023. The diagnosis was based on the tissue sample obtained during gastroscopy and confirmed by postoperative pathology. Patients were staged according to the American Joint Committee on Cancer (AJCC)/Union for International Cancer Control (UICC) 8th edition staging system. The exclusion criteria for patients were as follows: (I) receipt of prior treatment before surgery; (II) history of other malignancies or hematological disorders; (III) distant metastasis; (IV) recurrent GC; (V) history of blood transfusion or related conditions; (VI) disorders or dysfunction of the heart, liver, or kidneys; (VII) incomplete collection of laboratory data. After applying these criteria, 1,004 GC patients were included in the study. All cases were randomly divided into a training set (modeling group) and a testing set (validation group) at a ratio of 7:3. Among them, 703 patients were assigned to the modeling group for building predictive models, and 301 patients were assigned to the validation group for internal validation. There was no statistically significant difference in clinical data between the two groups mentioned above (Table S1).
The research procedures of this study were as follows: firstly, we obtained laboratory data and pathological characteristics in GC patients treated with curative gastrectomy. Secondly, we analyzed the differences in laboratory indicators between PNI and PNI-negative groups to identify significant risk factors for PNI. Then, the optimal cut-off value for PNI was determined using ROC-AUC analysis. Finally, we formulated the prediction equation model and nomogram based on multivariate logistic regression results and assessed the model using ROC-AUC, calibration curve analysis, and DCA. Additionally, we verified the prediction model using the testing set. A flow chart of the study is provided in Figure 1.
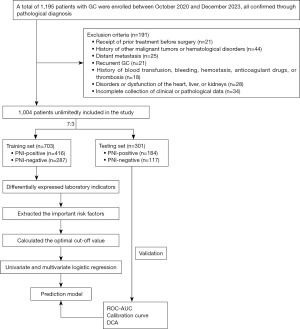
Laboratory methods
The fasting venous blood sample was collected from all patients in the morning. All laboratory indicators were checked before the cases received surgery treatment. Sysmex XE-2100 hematology analyzer and CS-5100 Hemagglutination analyzer (Sysmex, Kobe, Hyogo, Japan) were used to detect blood cell count and hemagglutination index. An Erytra automatic blood analyzer (Grifols, Barcelona, Spain) was used to detect patient blood type. The cobas® 8000 modular analyzer (Roche Diagnostics, Indianapolis, IN, USA) was used to detect the concentration of albumin and lactate dehydrogenase (LDH). The calculation formula was as follows: NLR = neutrophil-to-lymphocyte ratio, PLR = platelet-to-lymphocyte ratio, FLR = fibrinogen-to-lymphocyte ratio, LMR = lymphocyte-to-monocyte ratio, ALR = albumin-to-lymphocyte ratio. Then, the concentration of serum tumor markers [CEA, CA125, carbohydrate antigen 72-4 (CA72-4), carbohydrate antigen 19-9 (CA19-9), alpha-fetoprotein (AFP)] was measured with electrochemiluminescence assays in a Roche E601 Immunoassay Analyzer according to the manufacturer’s protocols.
PNI detection
The criteria of PNI were cancer cells inside the perineurium involving at least 33% of the circumference (33). Nerve structure was confirmed by hematoxylin and eosin staining. For each specimen, three independent representative tissue sections were prepared, and when PNI was observed in any of the three sections, the specimens were classified as PNI.
Statistical analysis
The software SPSS 20.0 (IBM Corp., Armonk, NY, USA) and GraphPad Prism v9.4.1 (GraphPad Software, San Diego, CA, USA) were employed for statistical analysis. The continuous variables in this study were judged by Kolmogorov-Smirnov test to be non-normally distributed. They were expressed as medians with 25th and 75th percentiles, and results were compared using Mann-Whitney U test. Chi-square test was used to compare categorical variables. The important risk factors of PNI were evaluated by random forest package in RStudio (RStudio, Boston, MA, USA). The ROC-AUCs were used to evaluate the discriminatory ability of the factors for PNI. The sensitivity-specificity relationship was determined using Youden’s index. Univariate and multivariate logistic regression analysis identified independent risk factors for PNI, with OR and 95% CI calculated. The prediction model was constructed from the multivariate logistic regression results, and its discriminatory ability was determined by ROC-AUC. The consistency between predicted and actual results was assessed through calibration curve analysis by the rms [6.4.0] software package (https://cran.r-project.org/web/packages/rms/). DCA was performed to assess the clinical utility of the model by the rmda [1.6] software package of R (4.2.1; https://cran.r-project.org/web/packages/rmda/). Subsequently, we verified the prediction model using the testing set. A two-sided P value of less than 0.05 was considered statistically significant.
Results
Patient characteristics in modeling group
In the modeling group, 416 GC patients (59.14%) were pathologically diagnosed with PNI; a typical micrograph of PNI is displayed in Figure 2. As shown in the Table 1, the medians of age and preoperative D-dimer, NLR, PLR, FLR, LMR, ALR, CEA, CA72-4, CA19-9, and CA125, and the difference between the two groups were statistically significant (all P<0.01). In contrast, gender (P=0.49), blood type (P=0.66), LDH (P=0.70), and AFP (P=0.67) showed no statistically significant differences between the two groups.
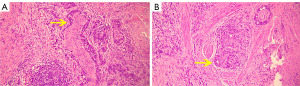
Table 1
Characteristics | PNI-negative (n=287) | PNI-positive (n=416) | χ2/Z | P value |
---|---|---|---|---|
Age (years) | 63 [55–69] | 66 [58–71] | −3.003 | 0.003 |
Gender | 0.569 | 0.49 | ||
Women | 74 (25.78) | 118 (28.37) | ||
Men | 213 (74.22) | 298 (71.63) | ||
Blood type | 0.195 | 0.66 | ||
A | 92 (32.06) | 143 (34.38) | ||
AB | 36 (12.54) | 33 (7.93) | ||
B | 78 (27.18) | 105 (25.24) | ||
O | 81 (28.22) | 135 (32.45) | ||
D-dimer (mg/L) | 0.31 [0.19–0.53] | 0.40 [0.26–0.77] | −5.182 | <0.001 |
NLR | 1.85 [1.47–2.50] | 2.14 [1.62–2.92] | −4.032 | <0.001 |
PLR | 117.33 [89.94–160.57] | 141.32 [110.19–190.77] | −5.779 | <0.001 |
FLR | 1.68 [1.28–2.19] | 2.12 [1.59–2.85] | −6.349 | <0.001 |
LMR | 3.96 [3.01–5.16] | 3.60 [2.74–4.60] | −3.376 | <0.001 |
ALR | 25.14 [20.98–31.93] | 27.91 [22.07–34.37] | −2.825 | 0.005 |
LDH (mmol/L) | 176.00 [156.00–199.00] | 175.00 [154.00–199.00] | −0.393 | 0.70 |
CEA (ng/mL) | 1.97 [1.34–3.16] | 2.46 [1.41–4.53] | −3.654 | <0.001 |
CA72-4 (U/mL) | 1.76 [1.50–3.43] | 2.34 [1.50–5.79] | −4.177 | <0.001 |
CA19-9 (U/mL) | 8.08 [5.42–14.20] | 10.55 [6.03–20.53] | −3.690 | <0.001 |
AFP (ng/mL) | 2.45 [1.77–3.40] | 2.39 [1.76–3.33] | −0.421 | 0.67 |
CA125 (U/mL) | 8.79 [6.26–12.70] | 9.97 [7.23–15.18] | −3.471 | <0.001 |
The measurement data were expressed as the median and quartile [25–75%], and the enumeration data were expressed as frequency and rate (%). PNI, perineural invasion; NLR, neutrophil-to-lymphocyte ratio; PLR, platelet-to-lymphocyte ratio; FLR, fibrinogen-to-lymphocyte ratio; LMR, lymphocyte-to-monocyte ratio; ALR, albumin-to-lymphocyte ratio; LDH, lactic dehydrogenase; CEA, carcinoembryonic antigen; CA72-4, carbohydrate antigen 72-4; CA19-9, carbohydrate antigen 19-9; AFP, alpha-fetoprotein; CA125, carbohydrate antigen 125.
Random forest algorithm to extract important ranking for PNI in GC patients
Random forest analysis showed that variables were the most important ranking influencing the occurrence of PNI. As depicted in the Figure 3, variables with statistically significant differences between PNI and PNI-negative were analyzed using a random forest package in RStudio. The variable with the highest relative importance was CEA, followed by FLR, D-dimer, PLR, CA19-9, CA72-4, CA125, LMR, ALR, NLR, and age.
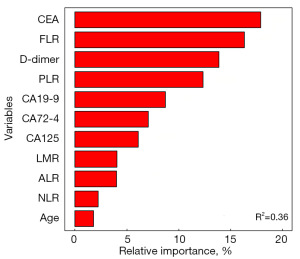
ROC curve of important risk factors for PNI in GC patients
In order to evaluate the discriminative power of CEA, FLR, D-dimer, PLR, CA19-9, CA72-4, CA125, LMR, ALR, NLR, and age between PNI and PNI-negative patients, the ROC-AUC was performed. As shown in Table 2, the best cut-off values of CEA, FLR, D-dimer, PLR, CA19-9, and CA72-4 for PNI prediction using the Youden’s index were 3.89 ng/mL, 2.08, 0.24 mg/L, 122.37, 14.85 U/mL, and 2.31 U/mL, respectively. The ROC-AUC of CEA diagnosis for PNI was 0.581 (95% CI: 0.539–0.623), corresponding to a sensitivity of 30.84% and a specificity of 86.07%. The ROC-AUC of FLR was 0.640 (95% CI: 0.599–0.681), with a sensitivity of 52.16% and a specificity of 71.43%. The ROC-AUC of D-dimer was 0.614 (95% CI: 0.572–0.656) with a sensitivity of 80.24% and specificity of 39.03%. The ROC-AUC of PLR was 0.627 (95% CI: 0.586–0.669, sensitivity: 66.11%, specificity: 55.41%). The ROC-AUC of CA19-9 was 0.581 (95% CI: 0.539–0.624, sensitivity: 37.11%, specificity: 77.36%).
Table 2
Factors | AUC (95% CI) | SE | P value | Sensitivity (%) | Specificity (%) | Cut-off value | Youden’s index |
---|---|---|---|---|---|---|---|
CEA (ng/mL) | 0.581 (0.539–0.623) | 0.021 | <0.001 | 30.84 | 86.07 | 3.89 | 0.169 |
FLR | 0.640 (0.599–0.681) | 0.021 | <0.001 | 52.16 | 71.43 | 2.08 | 0.236 |
D-dimer (mg/L) | 0.614 (0.572–0.656) | 0.022 | <0.001 | 80.24 | 39.03 | 0.24 | 0.193 |
PLR | 0.627 (0.586–0.669) | 0.021 | <0.001 | 66.11 | 55.41 | 122.37 | 0.215 |
CA19-9 (U/mL) | 0.581 (0.539–0.624) | 0.021 | <0.001 | 37.11 | 77.36 | 14.85 | 0.145 |
CA72-4 (U/mL) | 0.590 (0.548–0.633) | 0.022 | <0.001 | 50.84 | 64.46 | 2.31 | 0.153 |
CA125 (U/mL) | 0.577 (0.535–0.620) | 0.022 | <0.001 | 28.92 | 82.93 | 14.45 | 0.118 |
LMR | 0.575 (0.532–0.618) | 0.022 | 0.001 | 60.63 | 51.45 | 3.65 | 0.121 |
ALR | 0.562 (0.519–0.605) | 0.022 | 0.005 | 59.62 | 56.45 | 25.72 | 0.161 |
NLR | 0.590 (0.547–0.632) | 0.022 | <0.001 | 56.01 | 58.89 | 2.05 | 0.149 |
Age (years) | 0.566 (0.523–0.609) | 0.022 | 0.003 | 61.93 | 49.48 | 63.00 | 0.114 |
PNI, perineural invasion; GC, gastric cancer; CEA, carcinoembryonic antigen; FLR, fibrinogen-to-lymphocyte ratio; PLR, platelet-to-lymphocyte ratio; CA19-9, carbohydrate antigen 19-9; CA72-4, carbohydrate antigen 72-4; CA125, carbohydrate antigen 125; LMR, lymphocyte-to-monocyte ratio; ALR, albumin-to-lymphocyte ratio; NLR, neutrophil-to-lymphocyte ratio; AUC, area under the curve; SE, standard error; CI, confidence interval.
Univariate logistic regression analysis for PNI in GC patients
Using the occurrence of PNI as the dependent variable (negative =0, positive =1), CEA (≤3.89 ng/mL =0, >3.89 ng/mL =1), FLR (≤2.08 =0, >2.08 =1), D-dimer (≤0.24 mg/L =0, >0.24 mg/L =1), PLR (≤122.37 =0, >122.37=1), CA19-9 (≤14.85 U/mL =0, >14.85 U/mL =1), CA72-4 (≤ 2.31 U/mL =0, >2.31 U/mL =1), CA125 (≤14.45 ng/mL =0, >14.45 ng/mL =1), LMR (≤3.65 =0, >3.65 =1), ALR (≤25.72 =0, >25.72 =1), and NLR (≤2.05 =0, >2.05 =1), age (≤63 years =0, >63 years =1), as independent variables. The results showed that the above indicators were related to occurrence of PNI (Table 3).
Table 3
Factors | B | SE | Wald | P value | OR | 95% CI |
---|---|---|---|---|---|---|
CEA >3.89 ng/mL | 1.010 | 0.201 | 25.271 | <0.001 | 2.744 | 1.851–4.068 |
FLR >2.08 | 0.992 | 0.163 | 36.946 | <0.001 | 2.700 | 1.960–3.719 |
D-dimer >0.24 mg/L | 0.899 | 0.170 | 28.044 | <0.001 | 2.457 | 1.761–3.426 |
PLR >122.37 | 0.885 | 0.158 | 31.535 | <0.001 | 2.423 | 1.779–3.299 |
CA19-9 >14.85 U/mL | 0.697 | 0.174 | 16.082 | <0.001 | 2.008 | 1.428–2.822 |
CA72-4 >2.31 U/mL | 0.624 | 0.158 | 15.695 | <0.001 | 1.867 | 1.371–2.542 |
CA125 >14.45 ng/mL | 0.655 | 0.192 | 11.607 | 0.001 | 1.925 | 1.321–2.806 |
LMR >3.65 | −0.480 | 0.156 | 9.504 | 0.002 | 0.619 | 0.456–0.840 |
ALR >25.72 | 0.649 | 0.155 | 17.422 | <0.001 | 1.913 | 1.411–2.594 |
NLR >2.05 | 0.567 | 0.155 | 13.299 | <0.001 | 1.762 | 1.300–2.389 |
Age >63 years | 0.458 | 0.155 | 8.761 | 0.003 | 1.581 | 1.167–2.142 |
PNI, perineural invasion; GC, gastric cancer; CEA, carcinoembryonic antigen; FLR, fibrinogen-to-lymphocyte ratio; PLR, platelet-to-lymphocyte ratio; CA19-9, carbohydrate antigen 19-9; CA72-4, carbohydrate antigen 72-4; CA125, carbohydrate antigen 125; LMR, lymphocyte-to-monocyte ratio; ALR, albumin-to-lymphocyte ratio; NLR, neutrophil-to-lymphocyte ratio; B, Beta coefficient; SE, standard error; OR, odds ratio; CI, confidence interval.
Establishment a predictive risk prediction model for PNI in GC
To establish an accurate method for evaluating the possibility of PNI by using the above hematological indicators, we obtained a classification discriminant equation using the multivariate logistic regression analysis to ascertain whether GC patients have PNI as follows: Logit(P)= −1.211 + 0.695 × X1 + 0.546 × X2 + 0.686 × X3 + 0.653 × X4 + 0.515 × X5 + 0.518 × X6 (χ2=105.675, P<0.001; where X1 = CEA, X2 = FLR, X3 = D-dimer, X4 = PLR, X5 = CA19-9, X6 = CA72-4, Table 4), for which the critical value is 0.50, thus, if the Logit (P) of a case is larger than 0.50, it belongs to the PNI group. On the contrary, if the Logit (P) ≤0.50, it belongs to the PNI-negative groups. Based on the logistic regression model, we established a nomogram prediction model for PNI in GC patients (Figure 4A).
Table 4
Factors | B | SE | Wald | P value | OR | 95% CI |
---|---|---|---|---|---|---|
CEA (X1) | 0.695 | 0.216 | 10.405 | 0.001 | 2.004 | 1.314–3.507 |
FLR (X2) | 0.546 | 0.186 | 8.620 | 0.003 | 1.726 | 1.199–2.484 |
D-dimer (X3) | 0.686 | 0.182 | 14.259 | <0.001 | 1.986 | 1.391–2.835 |
PLR (X4) | 0.653 | 0.179 | 13.337 | <0.001 | 1.922 | 1.353–2.729 |
CA19-9 (X5) | 0.515 | 0.189 | 7.452 | 0.006 | 1.674 | 1.156–2.424 |
CA72-4 (X6) | 0.518 | 0.168 | 9.462 | 0.002 | 1.678 | 1.207–2.333 |
Constant | −1.211 | 0.197 | 37.895 | <0.001 | 0.298 | – |
Method: forward LR. PNI, perineural invasion; GC, gastric cancer; CEA, carcinoembryonic antigen; FLR, fibrinogen-to-lymphocyte ratio; PLR, platelet-to-lymphocyte ratio; CA19-9, carbohydrate antigen 19-9; CA72-4, carbohydrate antigen 72-4; B, Beta coefficient; SE, standard error; OR, odds ratio; CI, confidence interval; LR, likelihood ratio.
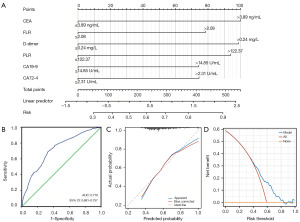
Immediately after, based on the predicted probability of PNI in GC patients using this model, the ROC-AUC was 0.719 (95% CI: 0.681–0.757, P<0.01), with a sensitivity of 68.51% and a specificity of 67.60% (Figure 4B). The calibration ability of the prediction model was evaluated through the Hosmer-Lemeshow goodness of fit test. The results indicated that there were no statistically significant differences between the predicted values of the model and the actual observed values (χ2=13.632, P=0.09) (Figure 4C). Besides, the DCA suggested that the clinical net benefit of intervention based on the predicted probability of the model is highest when the threshold probability is between 0.36 and 0.83 (Figure 4D).
Validation of the prediction model in testing set
Subsequently, we proceeded to validate the discriminant equation model using the testing set data, comprising 184 patients with PNI and 117 PNI-negative patients. There were 51 patients with GC mistaken for PNI and 56 PNI patients whose diagnoses were missed by the equation results (Table 5). The diagnostic efficiency for PNI in testing set was calculated by Clinical Calculator 1 (http://vassarstats.net/clin1.html). The sensitivity, specificity, positive predictive value (PPV), and negative predictive value (NPV) were 69.57%, 56.41%, 71.51%, and 54.10%, respectively (Table 6). As shown in Figure 5A, the ROC-AUC of the model was 0.791 (95% CI: 0.750–0.831, P<0.01). Further, the calibration curve showed that the model had good discrimination and accuracy (Figure 5B). The DCA indicated that when the threshold probability is between 0.27 and 0.87, the clinical net benefit is highest (Figure 5C).
Table 5
Results of criterion equation | Pathologic diagnosis | Total | |
---|---|---|---|
PNI-positive | PNI-negative | ||
PNI-positive | 128 | 51 | 179 |
PNI-negative | 56 | 66 | 122 |
Total | 184 | 117 | 301 |
PNI, perineural invasion.
Table 6
Diagnostic efficiency | Estimated value | 95% CI | |
---|---|---|---|
Lower limit | Upper limit | ||
Sensitivity (%) | 69.57 | 62.29 | 76.01 |
Specificity (%) | 56.41 | 46.94 | 65.45 |
Positive predictive value (%) | 71.51 | 64.21 | 77.87 |
Negative predictive value (%) | 54.10 | 44.86 | 63.07 |
Positive likelihood ratio | 1.59 | 1.27 | 2.00 |
Negative likelihood ratio | 0.54 | 0.43 | 0.68 |
PNI, perineural invasion; CI, confidence interval.
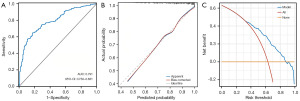
Discussion
PNI is characterized by an encounter between the cancer cells and neuronal fibers and holds an extremely poor prognosis in malignant tumors (34). A meta-analysis reported that the median of PNI in GC is 40.9% (range, 6.8–75.6%) (35). In our modeling group, PNI was pathologically diagnosed in 416 patients, representing 59.14% of the group. Paraffin-embedded tissue section of specimens and histopathology were used to evaluate PNI. Nevertheless, the positivity of PNI might be affected by the number of tissues obtained, biopsy techniques, tissue sections, and inter-observer differences. Additionally, some patients with PNI may not be surgical candidates. Therefore, identifying non-invasive, highly sensitive, and easily accessible indicators is crucial. Hematological indicators, known for their simplicity and clinical utility in tumor screening, have become a common method for cancer evaluation. This raises the question of whether blood-based biomarkers offer clinical value for PNI assessment in GC. To our knowledge, there are few studies on PNI and hematological indicators in GC.
In our study, the cases were randomly divided into a training set (modeling group) and a testing set (validation group) at a ratio of 7:3. In the modeling group, the medians of age, D-dimer, NLR, PLR, FLR, LMR, ALR, CEA, CA72-4, CA19-9, and CA125 were remarkably higher in GC patients with PNI than in those who were PNI-negative. Moreover, through the random forest algorithm, the top 5 important risk factors for PNI patients were CEA, FLR, D-dimer, PLR, and CA19-9. These results suggest that the occurrence of PNI in GC patients has a certain relationship with hematological indicators. Multivariate logistic regression analysis indicated that CEA >3.89 ng/mL, FLR >2.08, D-dimer >0.24 mg/L, PLR >122.37, CA19-9 >14.85 U/mL, and CA72-4 >2.31 U/mL are independently associated factors with PNI in GC patients. Meanwhile, we developed the equation: Logit(P)= −1.211 + 0.695 × CEA + 0.546 × FLR + 0.686 × D-dimer + 0.653 × PLR + 0.515 × CA19-9 + 0.518 × CA72-4 (χ2=105.675, P<0.001) to predict the presence of PNI. The model demonstrated that an ROC-AUC value of 0.719 (95% CI: 0.681–0.757) in the training set, with a sensitivity of 68.51% and a specificity of 67.60%. In addition, the calibration curve and DCA showed that the model has good discrimination and accuracy.
Growing evidence has indicated that there is an inflammatory link between tumor, microenvironment, and the systemic response. Different inflammatory markers have been analyzed in many cancers, including FLR and C-reactive protein in head and neck (36,37), PLR in thyroid carcinoma (28), and NLR in GC (38). Lymphocytes play a role in antitumor immune activity and tumor-related immune response (39). Lymphopenia has been observed in advanced cancer patients and its association with poor outcomes in patients with various types of cancer has been demonstrated (40). Fibrinogen plays a key role in coagulation, cell adhesion, systemic inflammation, and cancer progression (41,42). High levels of fibrin are indicators of coagulation and fibrinolysis activation, and almost all types of tumors exhibit abnormalities in coagulation and fibrinolysis, thereby promoting the body to be in a hypercoagulable state (43). D-dimer-containing species are soluble fibrin degradation products derived from plasmin-mediated degradation of cross-linked fibrin, which could be considered a biomarker of in vivo activation of both coagulation and fibrinolysis (44). Beyond that, platelets can secrete various growth factors to stimulate tumor cell differentiation, especially in advanced stage tumor patients who often have elevated platelets (45). Despite these insights, the relationship between blood-based biomarkers and PNI in GC remains inconclusive. PNI is posited as a novel pathway for tumor metastasis, with patients exhibiting increased levels of FLR, PLR, and D-dimer, suggesting heightened fibrinogen and platelet counts and reduced lymphocyte levels. These changes are associated with an elevated risk of PNI, indicating a poor prognosis (36,46,47). Common tumor markers such as CEA, CA19-9, and CA72-4 were identified as independent risk factors of GC with PNI in the present study, consistent with the findings of Liu et al. This study suggested that CEA ≥5 µg/L is a significant independent risk factor of PNI in advanced GC (20). Meanwhile, Huang et al. evaluated an optimal cut-off value of FLR of 2.555 (OR: 1.266, 95% CI: 1.031–1.555, P=0.02), where an FLR above 2.555 is a risk factor for GC with peritoneal dissemination (48).
Immediately after, we verified the discriminant equation model using the data of the testing set. The AUC-ROC was 0.791 (95% CI: 0.750–0.831, P<0.01) in the validation group. The sensitivity, specificity, PPV, and NPV were 69.57%, 56.41%, 71.51%, and 54.10%, respectively. These results suggested that the model has good predictive value for the risk of PNI in GC patients. The calibration curve also indicated that the predictive results were good in accordance with the actual results. PNI can serve as an important reference indicator for the biological behavior of GC, which is of great significance for improving tumor staging, selecting treatment plans rationally, and evaluating prognosis. A previous study suggested that PNI may be related to positive surgical margins (49). Therefore, preoperative prediction of PNI and appropriate expansion of tumor resection range during surgery maybe reduce tumor recurrence. In addition, PNI prediction is invaluable for patients ineligible for surgery, guiding the development of alternative treatment strategies.
The present study, a single-center retrospective analysis, has several inherent limitations. Firstly, potential selection bias and limitations in data extraction may have influenced the study’s conclusions. Secondly, the cut-off value of CEA, FLR, D-dimer, PLR, CA19-9, and CA72-4 was calculated only by mathematical methods, and the sensitivity and specificity of these laboratory data in predicting PNI require further verification in multi-center and large cohorts. Thirdly, some other inflammatory indicators associated with cancer progression, such as procalcitonin, interleukin-6, and C-reactive protein were not included in our study. Nonetheless, this study represents a novel approach, being the first to suggest the potential of preoperative CEA, FLR, D-dimer, PLR, CA19-9, and CA72-4 as predictors of PNI in GC patients.
Conclusions
We have successfully established and validated a predictive model for GC patients with PNI based on hematological indicators. The preoperative assessment of hematological inflammatory markers offers valuable insights for evaluating the risk of PNI in GC patients. This approach may serve as an adjunctive tool in clinical decision-making processes regarding PNI.
Acknowledgments
Funding: This work was supported by
Footnote
Reporting Checklist: The authors have completed the TRIPOD reporting checklist. Available at https://tcr.amegroups.com/article/view/10.21037/tcr-24-481/rc
Data Sharing Statement: Available at https://tcr.amegroups.com/article/view/10.21037/tcr-24-481/dss
Peer Review File: Available at https://tcr.amegroups.com/article/view/10.21037/tcr-24-481/prf
Conflicts of Interest: All authors have completed the ICMJE uniform disclosure form (available at https://tcr.amegroups.com/article/view/10.21037/tcr-24-481/coif). P.J. received funding form the Research Fund of Jiangsu Cancer Hospital (No. ZJ202106). D.M. received funding form the National Natural Science Foundation of China (No. 82002225). The other authors have no conflicts of interest to declare.
Ethical Statement: The authors are accountable for all aspects of the work in ensuring that questions related to the accuracy or integrity of any part of the work are appropriately investigated and resolved. The study was conducted in accordance with the Declaration of Helsinki (as revised in 2013) and approved by the Clinical Research Ethics Committee of Jiangsu Cancer hospital (approval number: #KY-2024-012). The requirement for informed consent was waived in this retrospective study.
Open Access Statement: This is an Open Access article distributed in accordance with the Creative Commons Attribution-NonCommercial-NoDerivs 4.0 International License (CC BY-NC-ND 4.0), which permits the non-commercial replication and distribution of the article with the strict proviso that no changes or edits are made and the original work is properly cited (including links to both the formal publication through the relevant DOI and the license). See: https://creativecommons.org/licenses/by-nc-nd/4.0/.
References
- Smyth EC, Nilsson M, Grabsch HI, et al. Gastric cancer. Lancet 2020;396:635-48. [Crossref] [PubMed]
- Sung H, Ferlay J, Siegel RL, et al. Global Cancer Statistics 2020: GLOBOCAN Estimates of Incidence and Mortality Worldwide for 36 Cancers in 185 Countries. CA Cancer J Clin 2021;71:209-49. [Crossref] [PubMed]
- Liu X, Shao L, Liu X, et al. Alterations of gastric mucosal microbiota across different stomach microhabitats in a cohort of 276 patients with gastric cancer. EBioMedicine 2019;40:336-48. [Crossref] [PubMed]
- Chang K, Song B. Venous Invasion and Perineural Invasion as Upstaging and Poor Prognostic Factors in N0 Gastric Cancers. Anticancer Res 2021;41:5803-10. [Crossref] [PubMed]
- Sakamoto S, Wada I, Omichi K, et al. Risk factors for remnant gastric cancer after distal gastrectomy for gastric cancer: a retrospective database review. J Gastrointest Oncol 2023;14:2334-45. [Crossref] [PubMed]
- Hu Z, Zuo Z, Miao H, et al. Incidence, Risk Factors and Prognosis of T4a Gastric Cancer: A Population-Based Study. Front Med (Lausanne) 2022;8:767904. [Crossref] [PubMed]
- Wang Z, Dong Z, Zhao G, et al. Prognostic role of myeloid-derived tumor-associated macrophages at the tumor invasive margin in gastric cancer with liver metastasis (GCLM): a single-center retrospective study. J Gastrointest Oncol 2022;13:1340-50. [Crossref] [PubMed]
- Chen Z, Fang Y, Jiang W. Important Cells and Factors from Tumor Microenvironment Participated in Perineural Invasion. Cancers (Basel) 2023;15:1360. [Crossref] [PubMed]
- Liu Q, Ma Z, Cao Q, et al. Perineural invasion-associated biomarkers for tumor development. Biomed Pharmacother 2022;155:113691. [Crossref] [PubMed]
- Nozawa H, Morikawa T, Kawai K, et al. Obstruction is associated with perineural invasion in T3/T4 colon cancer. Colorectal Dis 2019;21:917-24. [Crossref] [PubMed]
- Colonia-García A, Salazar-Peláez LM, Serna-Ortiz CA, et al. Prognostic value of lymphovascular and perineural invasion in squamous cell carcinoma of the tongue. Oral Surg Oral Med Oral Pathol Oral Radiol 2022;133:207-15. [Crossref] [PubMed]
- Wang J, Chen Y, Li X, et al. Perineural Invasion and Associated Pain Transmission in Pancreatic Cancer. Cancers (Basel) 2021;13:4594. [Crossref] [PubMed]
- Niu Y, Förster S, Muders M. The Role of Perineural Invasion in Prostate Cancer and Its Prognostic Significance. Cancers (Basel) 2022;14:4065. [Crossref] [PubMed]
- Wang K, Zhao XH, Liu J, et al. Nervous system and gastric cancer. Biochim Biophys Acta Rev Cancer 2020;1873:188313. [Crossref] [PubMed]
- Tao Q, Zhu W, Zhao X, et al. Perineural Invasion and Postoperative Adjuvant Chemotherapy Efficacy in Patients With Gastric Cancer. Front Oncol 2020;10:530. [Crossref] [PubMed]
- Jia H, Li R, Liu Y, et al. Preoperative Prediction of Perineural Invasion and Prognosis in Gastric Cancer Based on Machine Learning through a Radiomics-Clinicopathological Nomogram. Cancers (Basel) 2024;16:614. [Crossref] [PubMed]
- He Y, Yang M, Hou R, et al. Preoperative prediction of perineural invasion and lymphovascular invasion with CT radiomics in gastric cancer. Eur J Radiol Open 2024;12:100550. [Crossref] [PubMed]
- Zheng H, Zheng Q, Jiang M, et al. Contrast-enhanced CT based radiomics in the preoperative prediction of perineural invasion for patients with gastric cancer. Eur J Radiol 2022;154:110393. [Crossref] [PubMed]
- Li J, Xu S, Wang Y, et al. Spectral CT-based nomogram for preoperative prediction of perineural invasion in locally advanced gastric cancer: a prospective study. Eur Radiol 2023;33:5172-83. [Crossref] [PubMed]
- Liu SH, Hou XY, Zhang XX, et al. Establishment and validation of a predictive nomogram model for advanced gastric cancer with perineural invasion. Zhonghua Wei Chang Wai Ke Za Zhi 2020;23:1059-66. [PubMed]
- Liu J, Huang X, Chen S, et al. Nomogram based on clinical characteristics for preoperative prediction of perineural invasion in gastric cancer. J Int Med Res 2020;48:300060519895131. [Crossref] [PubMed]
- Ren T, Zhang W, Li S, et al. Combination of clinical and spectral-CT parameters for predicting lymphovascular and perineural invasion in gastric cancer. Diagn Interv Imaging 2022;103:584-93. [Crossref] [PubMed]
- Denk D, Greten FR. Inflammation: the incubator of the tumor microenvironment. Trends Cancer 2022;8:901-14. [Crossref] [PubMed]
- Khandia R, Munjal A. Interplay between inflammation and cancer. Adv Protein Chem Struct Biol 2020;119:199-245. [Crossref] [PubMed]
- Mantovani A, Allavena P, Sica A, et al. Cancer-related inflammation. Nature 2008;454:436-44. [Crossref] [PubMed]
- Balkwill F, Mantovani A. Inflammation and cancer: back to Virchow? Lancet 2001;357:539-45. [Crossref] [PubMed]
- Mosca M, Nigro MC, Pagani R, et al. Neutrophil-to-Lymphocyte Ratio (NLR) in NSCLC, Gastrointestinal, and Other Solid Tumors: Immunotherapy and Beyond. Biomolecules 2023;13:1803. [Crossref] [PubMed]
- Offi C, Romano RM, Cangiano A, et al. Clinical significance of neutrophil-to-lymphocyte ratio, lymphocyte-to-monocyte ratio, platelet-to-lymphocyte ratio and prognostic nutritional index in low-risk differentiated thyroid carcinoma. Acta Otorhinolaryngol Ital 2021;41:31-8. [Crossref] [PubMed]
- Karan C, Yaren A, Demirel BC, et al. Pretreatment PLR Is Preferable to NLR and LMR as a Predictor in Locally Advanced and Metastatic Bladder Cancer. Cancer Diagn Progn 2023;3:706-15. [Crossref] [PubMed]
- Li B, Deng H, Zhou Z, et al. The Prognostic value of the Fibrinogen to pre-albumin ratio in malignant tumors of the digestive system: a systematic review and meta-analysis. Cancer Cell Int 2022;22:22. [Crossref] [PubMed]
- Zhang Y, Cao J, Deng Y, et al. Pretreatment plasma fibrinogen level as a prognostic biomarker for patients with lung cancer. Clinics (Sao Paulo) 2020;75:e993. [Crossref] [PubMed]
- Cong R, Xu R, Ming J, et al. Construction of a preoperative nomogram model for predicting perineural invasion in advanced gastric cancer. Front Med (Lausanne) 2024;11:1344982. [Crossref] [PubMed]
- Liebig C, Ayala G, Wilks JA, et al. Perineural invasion in cancer: a review of the literature. Cancer 2009;115:3379-91. [Crossref] [PubMed]
- Melgarejo da Rosa M, Clara Sampaio M, Virgínia Cavalcanti Santos R, et al. Unveiling the pathogenesis of perineural invasion from the perspective of neuroactive molecules. Biochem Pharmacol 2021;188:114547. [Crossref] [PubMed]
- Deng J, You Q, Gao Y, et al. Prognostic value of perineural invasion in gastric cancer: a systematic review and meta-analysis. PLoS One 2014;9:e88907. [Crossref] [PubMed]
- Brkic FF, Stoiber S, Friedl M, et al. The Potential Prognostic Value of a Novel Hematologic Marker Fibrinogen-to-Lymphocyte Ratio in Head and Neck Adenoid-Cystic Carcinoma. J Pers Med 2021;11:1228. [Crossref] [PubMed]
- de Oliveira KG, Thebit MM, Andrade TU, et al. Prognostic value of pretreatment cardiovascular biomarkers in head and neck squamous cell carcinoma. Oral Dis 2021;27:1435-42. [Crossref] [PubMed]
- Kosuga T, Konishi T, Kubota T, et al. Clinical significance of neutrophil-to-lymphocyte ratio as a predictor of lymph node metastasis in gastric cancer. BMC Cancer 2019;19:1187. [Crossref] [PubMed]
- Feng F, Zheng G, Wang Q, et al. Low lymphocyte count and high monocyte count predicts poor prognosis of gastric cancer. BMC Gastroenterol 2018;18:148. [Crossref] [PubMed]
- Lu C, Chen Q, Li J, et al. The prognostic role of lymphocyte to monocyte ratio (LMR) in patients with Myelodysplastic Neoplasms. Hematology 2023;28:2210929. [Crossref] [PubMed]
- Perisanidis C, Psyrri A, Cohen EE, et al. Prognostic role of pretreatment plasma fibrinogen in patients with solid tumors: A systematic review and meta-analysis. Cancer Treat Rev 2015;41:960-70. [Crossref] [PubMed]
- Zhang Y, Liu N, Liu C, et al. High Fibrinogen and Platelets Correlate with Poor Survival in Gastric Cancer Patients. Ann Clin Lab Sci 2020;50:457-62. [PubMed]
- Wu X, Yu X, Chen C, et al. Fibrinogen and tumors. Front Oncol 2024;14:1393599. [Crossref] [PubMed]
- Wauthier L, Favresse J, Hardy M, et al. D-dimer testing: A narrative review. Adv Clin Chem 2023;114:151-223. [Crossref] [PubMed]
- Li Z, Qu Y, Yang Y, et al. Prognostic value of the neutrophil-to-lymphocyte ratio, platelet-to-lymphocyte ratio and systemic immune-inflammation index in patients with laryngeal squamous cell carcinoma. Clin Otolaryngol 2021;46:395-405. [Crossref] [PubMed]
- Zhang CL, Jiang XC, Li Y, et al. Independent predictive value of blood inflammatory composite markers in ovarian cancer: recent clinical evidence and perspective focusing on NLR and PLR. J Ovarian Res 2023;16:36. [Crossref] [PubMed]
- Yamagata K, Fukuzawa S, Ishibashi-Kanno N, et al. The Association between D-Dimer and Prognosis in the Patients with Oral Cancer. Dent J (Basel) 2020;8:84. [Crossref] [PubMed]
- Huang C, Liu Z, Xiao L, et al. Clinical Significance of Serum CA125, CA19-9, CA72-4, and Fibrinogen-to-Lymphocyte Ratio in Gastric Cancer With Peritoneal Dissemination. Front Oncol 2019;9:1159. [Crossref] [PubMed]
- De Franco L, Marrelli D, Voglino C, et al. Prognostic Value of Perineural Invasion in Resected Gastric Cancer Patients According to Lauren Histotype. Pathol Oncol Res 2018;24:393-400. [Crossref] [PubMed]