Identification of anoikis-related long non-coding RNA signature as a novel prognostic model in lung adenocarcinoma
Highlight box
Key findings
• This study comprehensively analyzed anoikis-related long non-coding RNA (lncRNA) in The Cancer Genome Atlas database and established a prognostic risk model to predict the outcomes of lung adenocarcinoma (LUAD) patients.
• Prognostic superiority: this prognostic risk model demonstrated good performance than tradition clinicopathologic parameters, and with good sensitivity and specificity for LUAD patients.
• Immunological associations: the signature showed a significant association with infiltration of immune cells, expression of immune checkpoints, as well as immune functions, suggesting links between anoikis-related lncRNA and the tumor immune microenvironment.
• Therapeutic relevance: the signature demonstrated potential sensitivity to chemo-/target-therapy, highlighting its clinical relevance for personalized treatment in LUAD.
What is known and what is new?
• Dysregulated expression of lncRNAs have been used to diagnose and predict the outcomes of LUAD patients. Combination of multiple lncRNAs could enhance the specificity and accuracy of the lncRNA-based prediction model.
• We identified seven prognostic anoikis-related lncRNAs to establish a prognostic model that showed good sensitivity and specificity, as well as its significance related to tumor immune microenvironment.
What is the implication, and what should change now?
• This study indicates that integrated analysis of anoikis-related lncRNAs will provide novel insight to diagnose and predict the outcomes of LUAD patients. Clinicians are urged to incorporate this signature into their repertoire of risk assessment protocols, while researchers are encouraged to embark upon a deeper exploration of the identified genes to uncover their potential as targets for therapeutic interventions.
Introduction
Lung cancer ranks the leading cause of cancer-related death worldwide (1,2). Lung adenocarcinoma (LUAD), as the major histopathological type of lung cancer, its prognosis remains unsatisfactory (3). The treatment strategies rely on tumor stages. But most of the patients are staged at advanced stages. Despite the advanced development in targeted therapy and immune therapy, the 5-year overall survival (OS) rate remains low (4-7). Therefore, identification of novel diagnostic and prognostic biomarkers is of great importance.
Anoikis, as a novel form of programmed cell death, demonstrates important roles in maintaining tissue homeostasis and preventing abnormal cell adhesion to abnormal extracellular matrix (ECM) (8-10). However, tumor cells could gain the capability to resist anoikis thereby enabling tumor cells to survive and metastasis to develop (11,12). In recent years, an increasing number of anoikis‐related genes (ARGs) have been identified in LUAD. Jin et al. confirmed that GDH1-mediated metabolic reprogramming of glutaminolysis promotes anoikis resistance and tumor metastasis in LKB1-deficient lung cancer (13). A prior study demonstrated that four ARGs (PLK1, SLC2A1, ANGPTL4, and CDKN3) were highly expressed in the tumor samples from clinical LUAD patients, and knockdown of these genes in LUAD cells by transfection with small interfering RNAs significantly inhibited LUAD cell proliferation and migration, and promoted anoikis (14). Diao et al. developed a risk model based on 16 ARGs, demonstrating that LUAD patients in the high-risk group exhibited shorter survival times. Additionally, the infiltration of tumor-infiltrating immune cells (M0 macrophages, neutrophils, resting mast cells, and activated memory CD4+ T cells), and the expression of immunosuppressive receptors (CTLA4, PD-1, LAG3, BTLA, and TIGIT) and immunosuppressive ligands (PD-L1, PD-L2, and TNFSF14) was higher in the high‐risk group. These factors may contribute to tumor immune escape in LUAD, further worsening patient prognosis (15). Wang et al. constructed a risk model based on 21 ARGs that effectively divided patients into low- and high-risk subgroups with distinct prognoses and patterns of immune cell infiltration, indicating that anoikis plays an important role in tumor microenvironment (TME) regulation and immune pathways in LUAD (16). Although the prognostic value and roles of ARGs have been studied in multiple solid cancers, including LUAD (15,17), the prognostic value of an anoikis-related long non-coding RNA (lncRNA) signature remains to be defined in LUAD.
LncRNA demonstrates important roles in cancer development by regulating gene expression through a variety of mechanisms, including transcriptional, post-transcriptional, and translational levels (18-21). A bunch of differentially expressed lncRNAs with specific biological functions have also been detected in LUAD (22,23). Ferroptosis-, cuproptosis-, or pyroptosis-related lncRNA signatures with prognostic values have been identified in LUAD (24-26). However, there is still lack of study concerning anoikis-related lncRNA in LUAD.
In this study, we constructed a prognostic risk model by comprehensively analyzing anoikis-related lncRNAs in LUAD. The prognostic predictive capability, clinicopathological significance, and immune landscape of the risk model were further explored. Finally, a nomogram was established to validate the potential clinical significance of the risk signature in LUAD. Our study would provide an overview of a novel prognostic model which could effectively diagnose and predict the outcomes of LUAD patients. We present this article in accordance with the TRIPOD reporting checklist (available at https://tcr.amegroups.com/article/view/10.21037/tcr-24-264/rc).
Methods
Data retrieval and identification of differentially expressed ARGs and lncRNAs
The RNA sequence data and corresponding clinical data of LUAD patients were downloaded from The Cancer Genome Atlas (TCGA) database. The study met the publication guidelines of TCGA, thus additional approval by the ethics committee was not required. The study was conducted in accordance with the Declaration of Helsinki (as revised in 2013).
A list of ARGs was downloaded from literature screening (27,28). The differentially expressed ARGs and lncRNAs were extracted using “edgeR” package in R software or Peal software. |Log(fold change)| >1 and adjusted P value <0.05 were defined as the cut-off criteria. Heatmap clustering was generated using “pheatmap” package in R software.
Acquisition of anoikis-related lncRNAs in LUAD
We identified anoikis-related lncRNAs using Spearman correlation coefficient analysis of gene expression data. With a threshold of |correlation coefficient| >0.4 and P<0.001, we selected the significant anoikis-related lncRNAs for further analysis. A correlation coefficient of 0.4 was chosen to ensure that only moderate to strong correlations were included. The P value of <0.001 indicated a high degree of statistical significance.
Expression of the anoikis-related lncRNAs
Differential expression of independent anoikis-related lncRNAs between LUAD tumor tissues and normal tissues was compared using “limma”, “plyr”, “reshape2”, and “ggpubr” packages.
Kaplan-Meier survival analysis
The correlations between the anoikis-related lncRNAs and OS of LUAD patients were analyzed using Kaplan-Meier survival analysis and log-rank test via “survival” package in R software. A P value <0.05 was considered statistically significant.
Construction of a prognostic risk model
The univariate Cox analysis was further performed to identify candidate prognostic lncRNA biomarkers. The candidate anoikis-related lncRNAs with a P value <0.01 were subjected to the multivariate Cox analysis to select independent prognostic biomarkers and construct a prognostic risk model in R software. Ultimately, seven prognostic lncRNAs were identified. The coefficients for constructing risk score model were derived from multivariate Cox regression analysis for significant lnRNAs. The risk score for all patients was determined by summing the regression coefficients of the selected lnRNAs multiplied by the corresponding expression values. In light of the coefficient and expression value of each lncRNA in our study, risk score = −0.2584 × AC026355.2 + 0.2478 × AL606489.1 + 0.1513 × AL031667.3 + 0.1511 × LINC02802 + 0.0652 × LINC01116 + (−1.7244) × AC018529.1 + 0.1004 × AP000844.2. Based on the calculated risk scores, the LUAD patients were categorized into low- and high-risk groups using the median value as the threshold.
Assessment of the performance of the prognostic risk model
A “survival” package was performed to investigate the prognostic value of the risk model in R software. The risk score distribution, survival status distribution and differential expression of the anoikis-related lncRNAs between the high- and low-risk groups were analyzed using “pheatmap” package. The receiver operating characteristic (ROC) was assessed by “survival ROC” package in R software.
Clinicopathological significance of the risk signature
The correlations between the risk score and various clinicopathological parameters, such as age, gender, T stage, N stage, M stage, and tumor-node-metastasis (TNM) stage, were analyzed using “limma” and “ggpubr” packages in R software.
Immune landscape analysis
The immune cell infiltrations were calculated using the CIBERSORT algorithm. The differences in the immune functions were analyzed using “GSVA”, “GSEABase”, “ggpubr” and “reshape2” packages based on “immune.gmt” file. The differences in the expression of immune checkpoints were analyzed using “limma”, “ggplot2”, “ggpubr” and “reshape2” packages. The correlations between the risk score and immune subtype were assessed using “limma” and “ggpubr” packages.
Construction of nomogram
A nomogram was established by combining the risk score and clinicopathological factors (age, gender, T stage, N stage, M stage, and TNM stage) using “survival”, “timeROC”, and “regplot” packages. Then, the discrimination and calibration of the nomogram was assessed in the entire LUAD dataset by the ROC and calibration curves.
Drug sensitivity analyses
In order to investigate the clinical manifestations of chemotherapeutic drugs and targeted drugs in patients with LUAD, we calculated the half-maximal inhibitory concentration (IC50) values of common drugs by using the “pRRophetic” package. And P value <0.001 was considered as statistical significance.
Association analysis between risk signature and N6-methyladenosine (m6A) regulators
The expression profile of m6A-related regulators was obtained from the TCGA database. Then, the expression of m6A-related regulators between high- and low-risk groups were visualized using the R package “ggplot2” and “ggpubr”. Pearson’s correlation analysis was subsequently implemented to investigate the correlation of anoikis-related lncRNAs and m6A-related regulators.
Competing endogenous RNA (ceRNA) regulatory network and functional enrichment analysis
The miRcode database (http://www.mircode.org/) was applied to predict the target microRNA (miRNA) of AL606489.1. Potential target messenger RNAs (mRNAs) of the miRNAs were then screened using miRDB (http://www.mirdb.org/), miRTarBase (https://mirtarbase.cuhk.edu.cn/), and TargetScan databases together. Gene Ontology (GO) analysis and Kyoto Encyclopedia of Genes and Genomes (KEGG) enrichment analysis were performed to investigate the functions and potential signaling pathways of differentially expressed mRNAs using the R package “clusterProfiler”.
Statistical analysis
The retrieval of RNA profiling and clinical information from the TCGA dataset was performed in R software (R 4.2.1) or Perl software. All statistical analyses and functional enrichment were assessed by R software. A P value <0.05 was statistically significant.
Results
Identification of anoikis-related lncRNAs in LUAD patients
RNA sequence data and clinical data of LUAD patients were downloaded from the TCGA database. There are 479 cases of LUAD patients, including 497 tumor tissues and 54 non-tumoral tissues. Firstly, a list of 27 ARGs were retrieved from published article (27). LncRNAs demonstrated close correlations with ARGs were defined as anoikis-related lncRNAs (|R|>0.4, P<0.001). A total of 1,694 anoikis-related lncRNAs were identified. The heatmap showed the anoikis-related lncRNAs between LUAD tumor tissues and adjacent noncancerous tissues (Figure S1).
Construction of an anoikis-related lncRNA risk model for LUAD patients
We established a prognostic risk model using univariate and multivariate Cox regression analyses. According to the univariate Cox analysis, a total of 16 anoikis-related lncRNAs demonstrated statistically significant by setting a P value <0.01 as the standard (Figure 1A). These 16 anoikis-related lncRNAs were subjected to multivariate Cox regression analysis to construct a prognostic risk model. According to the multivariate Cox analysis, there were seven anoikis-related lncRNAs in the prognostic risk model. As shown in Figure 1B, the forest plots indicated the independent prognostic values of these seven anoikis-related lncRNAs. Among them, six anoikis-related lncRNAs could be viewed as independent prognostic factors for LUAD patients, including AC026355.2 (P=0.001), AL606489.1 (P=7.58e−05), AL031667.3 (P<0.001), LINC01116 (P=0.02), AC018529.1 (P=0.003), and AP000844.2 (P=0.02) (Figure 1B).
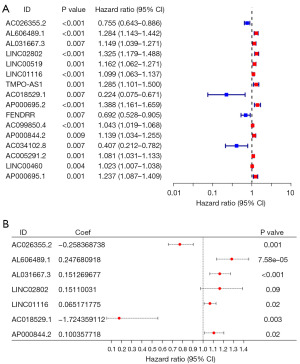
Performance of the prognostic risk model
We further verified the efficiency of the prognostic risk model. Patients were divided into high- and low-risk groups according to the risk model. As shown in Figure 2A, the Kaplan-Meier analysis demonstrated that there was significant difference between high- and low-risk groups (P<0.001). This risk model could significantly predict the risk score for LUAD patients. The distribution of risk scores (Figure 2B) and the correlation between the risk score and survival status (Figure 2C) were displayed. The expression levels of these seven anoikis-related lncRNAs are demonstrated in Figure 2D. The expression of AL606489.1, LINC02802, LINC01116, and AP000844.2 demonstrated higher expression in the high-risk group. While, the expression levels of AC026355.2, AL031667.3, and AC018529.1 were much higher in the low-risk group (Figure 2D).
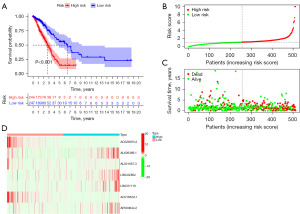
Moreover, univariate and multivariate Cox regression analyses verified the significant prognostic value of the risk signature (P<0.001, Figure 3A,3B). As shown in Figure 3C, the ROC curve of the risk model showed that the area under the curve (AUC) values at 1-, 2-, and 3-year were 0.717, 0.705, and 0.712 respectively, which suggested that the constructed prognostic model may be a good prognosis indicator for LUAD patients. We further compared the prognostic value of the risk signature with age, gender, and stage. As shown in Figure 3D, the AUC value of the risk signature in 0.717, which demonstrating better performance than other clinicopathological factors.
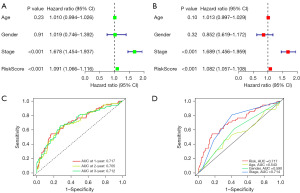
Expression and clinicopathological significance of the risk signature
The expression levels of these anoikis-related lncRNAs were compared between tumor tissues and normal tissues. Higher expression of AC026355.2 (Figure S2A, P<0.001), AL031667.3 (Figure S2B, P<0.001), AL606489.1 (Figure S2C, P<0.001), AP000844.2 (Figure S2D, P<0.001), LINC01116 (Figure S2E, P<0.001), and LINC02802 (Figure S2F, P<0.001), and lower expression of AC018529.1 (Figure S2G, P<0.001) were detected in LUAD tumor tissues.
The associations between the identified anoikis-related lncRNAs and OS of LUAD patients were analyzed using Kaplan-Meier analysis and log-rank test in R software. The Kaplan-Meier survival curves of the seven anoikis-related lncRNAs were shown in Figure 4. High expression of AL606489.1 (Figure 4A, P<0.001), LINC01116 (Figure 4B, P<0.001), and LINC02802 (Figure 4C, P<0.001) were positively correlated with poor OS. While high expression of AC018529.1 (Figure 4D, P<0.001), AC026355.2 (Figure 4E, P<0.001), and AL031667.3 (Figure 4F, P=0.002) indicated better OS for LUAD patients. Only AP000844.2 demonstrated no statistical significance in predicting the OS of LUAD patients (Figure 4G, P=0.20).
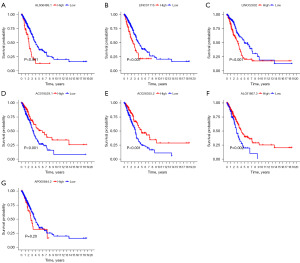
We next investigated the correlations between the risk signature and various clinicopathological factors. LUAD patients with lymph node metastasis (Figure S3A, P=1.8e−3) and advanced tumor stage (Figure S3B, P=6.3e−3) normally demonstrated higher risk score. Moreover, there were positive correlations between the risk score and RNA stemness score (RNAss) (Figure S3C, R=0.22, P=2.7e−06), as well as DNA stemness score (DNAss) (Figure S3D, R=0.13, P=0.007). However, there were no statistical significances between the risk signature and age (P=0.46), gender (P=0.056), T stage (P=0.051), and M stage (P=0.051) (Figure S4A-S4D).
Immune landscape of the risk signature
The relationships between the risk signature and immune landscape were analyzed by various aspects. There were negative correlations between the risk signature and immune score (Figure 5A, R=−0.2, P=3.5e−06) and stromal score (Figure 5B, R=−0.17, P=7.4e−05). Firstly, the immune functions, such as antigen-presenting cell (APC) co-inhibition, checkpoint, human leukocyte antigen (HLA), T cell co-inhibition, and type II interferon (IFN) response were repressed in the low-risk group (Figure 5C). We next studied the infiltration of various immune cells between high- and low-risk groups. More activated dendritic cells (aDCs), B cells, dendritic cells (DCs), interdigitating dendritic cells (iDCs), mast cells, neutrophils, plasmacytoid dendritic cells (pDCs), T helper (Th) cells, Th1 cells, and tumor-infiltrating lymphocytes (TILs) were infiltrated in the low-risk group (Figure 5D). We next investigated differential expression of 47 immune checkpoints between high- and low-risk groups. The expression level of CD276 was much higher in the high-risk group. Otherwise, the expression levels of other immune checkpoints were downregulated in the high-risk group, which including CTLA4, CD274, CD44, and IDO2 (Figure 5E). The immune microenvironment was classified into six immune subtypes. The risk scores were much higher in C1 and C2 immune subtypes. Whereas the risk score was the lowest in C4 (Figure 5F).
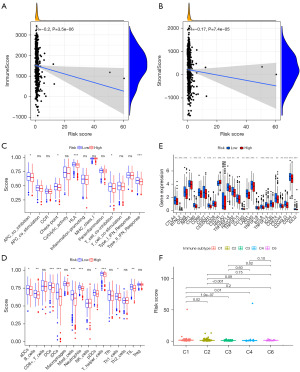
Establishment of a nomogram
A nomogram combining the risk score and clinicopathological factors is established in Figure 6A. In this nomogram, the risk score, age, T stage, N stage, and TNM stage demonstrated statistical significances. ROC curves revealed the AUC values of the nomogram in predicting 1-, 3-, and 5-year survival were 0.822, 0.837, and 0.885, respectively (Figure 6B).
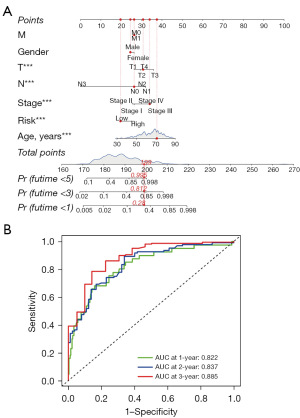
Roles of the risk signature in predicting chemotherapy and targeted therapy
For patients with advanced or metastatic who have no chance of surgical resection, chemotherapy, and targeted therapy are major treatment strategies. We next estimated IC50 values for 138 drugs in the TCGA-LUAD cohort. The findings indicated that the high-risk group exhibited a lower IC50 for pazopanib, temsirolimus, and bexarotene, suggesting that our proposed risk signature can be used as a potential indicator of drug sensitivity (Figure 7A-7C). Moreover, small molecule drugs such as NU.7441, GDC0941, and AMG.706 have demonstrated lower IC50 values in the high-risk groups, indicating their potential as novel therapeutic agents for patients afflicted with LUAD (Figure 7D-7F).
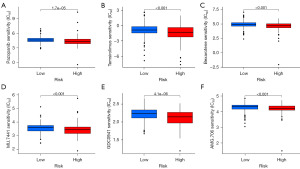
Correlation of m6A-related regulators, ceRNA network construction, and functional enrichment analysis
Increasing evidence has demonstrated the pivotal role of RNA modification in governing the expression and functionality of lncRNA. m6A emerges as the most prevalent and influential modification of RNA. The regulatory molecules involved in m6A modification exert a significant impact on LUAD by modulating diverse biological processes. To elucidate the association between m6A methylation and risk signature, we first interrogated the expression of 12 common m6a regulators in high and low-risk groups (Figure 8A). Pearson’s correlation coefficient was further conducted to analyze the relationship between m6A-related regulators and prognostic signature. As shown in Figure 8B, the m6A-related regulator RBM15 was found to be significantly correlated with the prognostic lncRNA AL606489.1. A previous study has shown that AL606489.1 has been reported as a valuable prognostic predictor in LUAD patients (29). Therefore, we believe that there is a compelling need for further investigation into the ceRNA networks associated with AL606489.1 to deepen our understanding of its potential implications. We constructed a ceRNA network through the miRcode database to explore the potential interaction miRNAs of AL606489.1. We found that there were eight miRNAs that possessed interaction positions with lncRNA AL606489.1. The target mRNAs of miRNAs which potentially interact with AL606489.1 were further screened by combining the miRDB, miRTarBase, and TargetScan databases together (Figure 8C). We conducted GO and KEGG analyses to elucidate the functional enrichment and pathways associated with the mRNAs regulated by lncRNA AL606489.1. The KEGG pathway analysis revealed that cellular senescence, cell cycle, and human T-cell leukemia virus 1 infection were significantly enriched (Figure 8D). On the other hand, the GO annotation demonstrated that the biological processes were predominantly linked to cell proliferation and DNA methylation (Figure 8E).
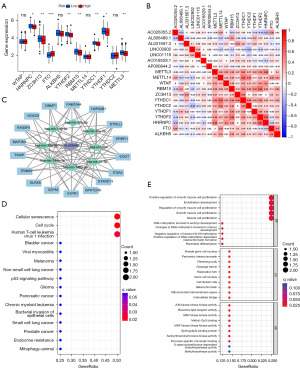
Discussion
The advancement in the next-generation sequencing (NGS) promotes the development of targeted therapy and immunotherapy for lung cancer patients (30-32). Besides, the NGS technology also leads us to learn more about the significant roles of lncRNA in lung cancer (33,34). Currently, dysregulated expression of lncRNAs has been used to diagnose and predict the outcomes of LUAD patients (33,35,36). Combination of multiple lncRNAs could enhance the specificity and accuracy of the lncRNA-based prediction model (37-39). More and more studies focus on exploring lncRNAs related to cellular functions, such as ferroptosis, pyroptosis, and immune microenvironment (24,26,40). In this study, we comprehensively analyzed anoikis-related lncRNAs in the TCGA database and established a prognostic risk model to predict the outcomes of LUAD patients.
In this study, a total of 1,694 anoikis-related lncRNAs were identified between LUAD tumor tissues and adjacent normal tissues. Among these 1,694 lncRNAs, univariate and multivariate Cox regression analyses were performed to identify seven prognostic anoikis-related lncRNAs to establish a prognostic risk model, including AC026355.2, AL031667.3, AL606489.1, AP000844.2, LINC01116, LINC02802, and AC018529.1. Except for LINC02802, the other six lncRNAs could be used as independent prognostic biomarkers for LUAD patients. The performance of the risk model was verified according to Kaplan-Meier survival analysis, ROC curve, univariate and multivariate Cox regression analyses. Our study demonstrated that this prognostic risk model demonstrates good performance than tradition clinicopathologic parameters, and with good sensitivity and specificity for LUAD patients.
Some of the anoikis-related lncRNAs in our prognostic risk model have been reported to be involved in the tumorigenesis of solid tumors, including LUAD. In accordance with our study, AL031667.3, as a cuproptosis- or ferroptosis-related lncRNA, has been reported to work as independent prognostic maker for LUAD (41,42). AL606489.1 has been identified as a prognostic lncRNA in LUAD, which is related to pyroptosis and ferroptosis (26,43). Besides, Chen et al. reported AL606489.1 as a necroptosis-related lncRNA in hepatocellular carcinoma (44). AP000844.2 expression is correlated with the prognosis of hepatocellular carcinoma and prostate cancer patients (45,46). The expression, prognosis value, and cellular functions of LINC01116 have been explored in multiple cancers, including melanoma (47), colorectal cancer (48), and hepatocellular carcinoma. LINC01116 has been reported to promote LUAD proliferation, cisplatin resistance, and metastasis (49). Ye et al. identified LINC0280 as an immune-related prognostic lncRNA in cervical cancer (50). However, AC026355.2 and AC018529.1 have not been reported and their biological functions are unclear.
Anoikis-resistance contributes to the invasion, metastasis, and drug resistance (51-53). There are many mechanisms contributing to anoikis-resistance, including the modulation of TME. There were negative correlations between the risk score and immune score, as well as stromal score. Thus, we analyzed the differences in the infiltration of immune cells, expression of immune checkpoints, as well as immune functions between high- and low-risk groups. The high-risk group demonstrated repressed immune functions, down-regulated expression of immune checkpoints, and less infiltration of immune cells, including B cells, dendritic cells, Th cells, and Th1 cells. Moreover, we observed that the risk scores were much higher in C1 and C2 immune subtypes, whereas the risk score was the lowest in C4. As we all know that C2 represents IFN-γ dominant, while C4 represents lymphocyte depleted, these results suggested that the risk signature was closely correlated to the tumor immune microenvironment. Since the functions of most of these lncRNAs are poorly understood and need further study, thus, our future work will focus on the biological functions of these lncRNAs in LUAD. In addition, we calculated the IC50 values of common drugs by using the “pRRophetic” package. Our results indicated that the high-risk group exhibited a lower IC50 for pazopanib, temsirolimus, and bexarotene, suggesting that our proposed risk signature can be used as a potential indicator of drug sensitivity.
In conclusion, we have established an anoikis-related lncRNA-based prognostic risk model for LUAD patients. Further study verified the good sensitivity and specificity of this risk model, as well as its significance related to tumor immune microenvironment. This study indicates that integrated analysis of anoikis-related lncRNA will provide novel insight to diagnose and predict the outcomes of LUAD patients.
Conclusions
This study establishes a robust prognostic signature based on seven prognostic anoikis-related lncRNAs, providing a reliable tool for predicting prognosis, delineating immune landscape, and offering profound insights for the development of personalized treatment strategies in LUAD patients.
Acknowledgments
Funding: This research was funded by
Footnote
Reporting Checklist: The authors have completed the TRIPOD reporting checklist. Available at https://tcr.amegroups.com/article/view/10.21037/tcr-24-264/rc
Peer Review File: Available at https://tcr.amegroups.com/article/view/10.21037/tcr-24-264/prf
Conflicts of Interest: All authors have completed the ICMJE uniform disclosure form (available at https://tcr.amegroups.com/article/view/10.21037/tcr-24-264/coif). The authors have no conflicts of interest to declare.
Ethical Statement: The authors are accountable for all aspects of the work in ensuring that questions related to the accuracy or integrity of any part of the work are appropriately investigated and resolved. The study was conducted in accordance with the Declaration of Helsinki (as revised in 2013).
Open Access Statement: This is an Open Access article distributed in accordance with the Creative Commons Attribution-NonCommercial-NoDerivs 4.0 International License (CC BY-NC-ND 4.0), which permits the non-commercial replication and distribution of the article with the strict proviso that no changes or edits are made and the original work is properly cited (including links to both the formal publication through the relevant DOI and the license). See: https://creativecommons.org/licenses/by-nc-nd/4.0/.
References
- Carioli G, Malvezzi M, Bertuccio P, et al. Cancer mortality in the elderly in 11 countries worldwide, 1970-2015. Ann Oncol 2019;30:1344-55. [Crossref] [PubMed]
- Bray F, Laversanne M, Sung H, et al. Global cancer statistics 2022: GLOBOCAN estimates of incidence and mortality worldwide for 36 cancers in 185 countries. CA Cancer J Clin 2024;74:229-63. [Crossref] [PubMed]
- Pankova D, Jiang Y, Chatzifrangkeskou M, et al. RASSF1A controls tissue stiffness and cancer stem-like cells in lung adenocarcinoma. EMBO J 2019;38:e100532. [Crossref] [PubMed]
- Zhang C, Zhang J, Xu FP, et al. Genomic Landscape and Immune Microenvironment Features of Preinvasive and Early Invasive Lung Adenocarcinoma. J Thorac Oncol 2019;14:1912-23. [Crossref] [PubMed]
- Theelen WSME, Peulen HMU, Lalezari F, et al. Effect of Pembrolizumab After Stereotactic Body Radiotherapy vs Pembrolizumab Alone on Tumor Response in Patients With Advanced Non-Small Cell Lung Cancer: Results of the PEMBRO-RT Phase 2 Randomized Clinical Trial. JAMA Oncol 2019;5:1276-82. [Crossref] [PubMed]
- Arrieta O, Barrón F, Padilla MS, et al. Effect of Metformin Plus Tyrosine Kinase Inhibitors Compared With Tyrosine Kinase Inhibitors Alone in Patients With Epidermal Growth Factor Receptor-Mutated Lung Adenocarcinoma: A Phase 2 Randomized Clinical Trial. JAMA Oncol 2019;5:e192553. [Crossref] [PubMed]
- Zavitsanou AM, Pillai R, Hao Y, et al. KEAP1 mutation in lung adenocarcinoma promotes immune evasion and immunotherapy resistance. Cell Rep 2023;42:113295. [Crossref] [PubMed]
- Taddei ML, Giannoni E, Fiaschi T, et al. Anoikis: an emerging hallmark in health and diseases. J Pathol 2012;226:380-93. [Crossref] [PubMed]
- Simpson CD, Anyiwe K, Schimmer AD. Anoikis resistance and tumor metastasis. Cancer Lett 2008;272:177-85. [Crossref] [PubMed]
- Dai Y, Zhang X, Ou Y, et al. Anoikis resistance--protagonists of breast cancer cells survive and metastasize after ECM detachment. Cell Commun Signal 2023;21:190. [Crossref] [PubMed]
- Kim YN, Koo KH, Sung JY, et al. Anoikis resistance: an essential prerequisite for tumor metastasis. Int J Cell Biol 2012;2012:306879. [Crossref] [PubMed]
- Yu Y, Song Y, Cheng L, et al. CircCEMIP promotes anoikis-resistance by enhancing protective autophagy in prostate cancer cells. J Exp Clin Cancer Res 2022;41:188. [Crossref] [PubMed]
- Jin L, Chun J, Pan C, et al. The PLAG1-GDH1 Axis Promotes Anoikis Resistance and Tumor Metastasis through CamKK2-AMPK Signaling in LKB1-Deficient Lung Cancer. Mol Cell 2018;69:87-99.e7. [Crossref] [PubMed]
- Wu J, Zhang Y, You G, et al. Identification of crucial anoikis-related genes as novel biomarkers and potential therapeutic targets for lung adenocarcinoma via bioinformatic analysis and experimental verification. Aging (Albany NY) 2024;16:2887-907. [Crossref] [PubMed]
- Diao X, Guo C, Li S. Identification of a novel anoikis-related gene signature to predict prognosis and tumor microenvironment in lung adenocarcinoma. Thorac Cancer 2023;14:320-30. [Crossref] [PubMed]
- Wang Y, Xie C, Su Y. A novel anoikis-related gene signature to predict the prognosis, immune infiltration, and therapeutic outcome of lung adenocarcinoma. J Thorac Dis 2023;15:1335-52. [Crossref] [PubMed]
- Kim EY, Cha YJ, Jeong S, et al. Overexpression of CEACAM6 activates Src-FAK signaling and inhibits anoikis, through homophilic interactions in lung adenocarcinomas. Transl Oncol 2022;20:101402. [Crossref] [PubMed]
- He RZ, Luo DX, Mo YY. Emerging roles of lncRNAs in the post-transcriptional regulation in cancer. Genes Dis 2019;6:6-15. [Crossref] [PubMed]
- Lin C, Wang Y, Wang Y, et al. Transcriptional and posttranscriptional regulation of HOXA13 by lncRNA HOTTIP facilitates tumorigenesis and metastasis in esophageal squamous carcinoma cells. Oncogene 2017;36:5392-406. [Crossref] [PubMed]
- Prensner JR, Chinnaiyan AM. The emergence of lncRNAs in cancer biology. Cancer Discov 2011;1:391-407. [Crossref] [PubMed]
- Rinn JL, Chang HY. Genome regulation by long noncoding RNAs. Annu Rev Biochem 2012;81:145-66. [Crossref] [PubMed]
- Pan J, Fang S, Tian H, et al. lncRNA JPX/miR-33a-5p/Twist1 axis regulates tumorigenesis and metastasis of lung cancer by activating Wnt/β-catenin signaling. Mol Cancer 2020;19:9. [Crossref] [PubMed]
- Yang J, Qiu Q, Qian X, et al. Long noncoding RNA LCAT1 functions as a ceRNA to regulate RAC1 function by sponging miR-4715-5p in lung cancer. Mol Cancer 2019;18:171. [Crossref] [PubMed]
- Yao J, Chen X, Liu X, et al. Characterization of a ferroptosis and iron-metabolism related lncRNA signature in lung adenocarcinoma. Cancer Cell Int 2021;21:340. [Crossref] [PubMed]
- Wang F, Lin H, Su Q, et al. Cuproptosis-related lncRNA predict prognosis and immune response of lung adenocarcinoma. World J Surg Oncol 2022;20:275. [Crossref] [PubMed]
- Song J, Sun Y, Cao H, et al. A novel pyroptosis-related lncRNA signature for prognostic prediction in patients with lung adenocarcinoma. Bioengineered 2021;12:5932-49. [Crossref] [PubMed]
- Sun Z, Zhao Y, Wei Y, et al. Identification and validation of an anoikis-associated gene signature to predict clinical character, stemness, IDH mutation, and immune filtration in glioblastoma. Front Immunol 2022;13:939523. [Crossref] [PubMed]
- Jiang G, Song C, Wang X, et al. The multi-omics analysis identifies a novel cuproptosis-anoikis-related gene signature in prognosis and immune infiltration characterization of lung adenocarcinoma. Heliyon 2023;9:e14091. [Crossref] [PubMed]
- Liang J, Jin W, Xu H. An efficient five-lncRNA signature for lung adenocarcinoma prognosis, with AL606489.1 showing sexual dimorphism. Front Genet 2022;13:1052092. [Crossref] [PubMed]
- Paik PK, Kim RK, Ahn L, et al. A Phase II Trial of Albumin-Bound Paclitaxel and Gemcitabine in Patients with Newly Diagnosed Stage IV Squamous Cell Lung Cancers. Clin Cancer Res 2020;26:1796-802. [Crossref] [PubMed]
- Schoenfeld AJ, Chan JM, Kubota D, et al. Tumor Analyses Reveal Squamous Transformation and Off-Target Alterations As Early Resistance Mechanisms to First-line Osimertinib in EGFR-Mutant Lung Cancer. Clin Cancer Res 2020;26:2654-63. [Crossref] [PubMed]
- Pecciarini L, Brunetto E, Grassini G, et al. Gene Fusion Detection in NSCLC Routine Clinical Practice: Targeted-NGS or FISH? Cells 2023;12:1135. [Crossref] [PubMed]
- Peng F, Wang R, Zhang Y, et al. Differential expression analysis at the individual level reveals a lncRNA prognostic signature for lung adenocarcinoma. Mol Cancer 2017;16:98. [Crossref] [PubMed]
- Morton ML, Bai X, Merry CR, et al. Identification of mRNAs and lincRNAs associated with lung cancer progression using next-generation RNA sequencing from laser micro-dissected archival FFPE tissue specimens. Lung Cancer 2014;85:31-9. [Crossref] [PubMed]
- Zhang J, Feng S, Su W, et al. Overexpression of FAM83H-AS1 indicates poor patient survival and knockdown impairs cell proliferation and invasion via MET/EGFR signaling in lung cancer. Sci Rep 2017;7:42819. [Crossref] [PubMed]
- Yu Z, Tang H, Chen S, et al. Exosomal LOC85009 inhibits docetaxel resistance in lung adenocarcinoma through regulating ATG5-induced autophagy. Drug Resist Updat 2023;67:100915. [Crossref] [PubMed]
- Yao Y, Zhang T, Qi L, et al. Comprehensive analysis of prognostic biomarkers in lung adenocarcinoma based on aberrant lncRNA-miRNA-mRNA networks and Cox regression models. Biosci Rep 2020;40:BSR20191554. [Crossref] [PubMed]
- Li J, Ma S, Lin T, et al. Comprehensive Analysis of Therapy-Related Messenger RNAs and Long Noncoding RNAs as Novel Biomarkers for Advanced Colorectal Cancer. Front Genet 2019;10:803. [Crossref] [PubMed]
- Yang L, Cui Y, Liang L, et al. Significance of cuproptosis-related lncRNA signature in LUAD prognosis and immunotherapy: A machine learning approach. Thorac Cancer 2023;14:1451-66. [Crossref] [PubMed]
- Feng Y, Zhang T, Zhang Z, et al. The super-enhancer-driven lncRNA LINC00880 acts as a scaffold between CDK1 and PRDX1 to sustain the malignance of lung adenocarcinoma. Cell Death Dis 2023;14:551. [Crossref] [PubMed]
- Mo X, Hu D, Yang P, et al. A novel cuproptosis-related prognostic lncRNA signature and lncRNA MIR31HG/miR-193a-3p/TNFRSF21 regulatory axis in lung adenocarcinoma. Front Oncol 2022;12:927706. [Crossref] [PubMed]
- Zheng Z, Zhang Q, Wu W, et al. Identification and Validation of a Ferroptosis-Related Long Non-coding RNA Signature for Predicting the Outcome of Lung Adenocarcinoma. Front Genet 2021;12:690509. [Crossref] [PubMed]
- Guo Y, Qu Z, Li D, et al. Identification of a prognostic ferroptosis-related lncRNA signature in the tumor microenvironment of lung adenocarcinoma. Cell Death Discov 2021;7:190. [Crossref] [PubMed]
- Chen M, Wu GB, Hua S, et al. Identification and validation of a prognostic model of necroptosis-related lncRNAs in hepatocellular carcinoma. Front Genet 2022;13:907859. [Crossref] [PubMed]
- Xu C, Qi X. Development and validation of a 4-lncRNA combined prediction model for patients with hepatocellular carcinoma. Adv Clin Exp Med 2022;31:1087-97. [Crossref] [PubMed]
- Liu S, Wang W, Zhao Y, et al. Identification of Potential Key Genes for Pathogenesis and Prognosis in Prostate Cancer by Integrated Analysis of Gene Expression Profiles and the Cancer Genome Atlas. Front Oncol 2020;10:809. [Crossref] [PubMed]
- Wang K, Li M, Zhang T, et al. LINC01116 Facilitates Melanoma 1 Progression Via Sequestering miR-3612 and Up-regulating GDF11 and SDC3. Arch Med Res 2022;53:44-50. [Crossref] [PubMed]
- Liang W, Wu J, Qiu X. LINC01116 facilitates colorectal cancer cell proliferation and angiogenesis through targeting EZH2-regulated TPM1. J Transl Med 2021;19:45. [Crossref] [PubMed]
- Zeng L, Lyu X, Yuan J, et al. Long non-coding RNA LINC01116 is overexpressed in lung adenocarcinoma and promotes tumor proliferation and metastasis. Am J Transl Res 2020;12:4302-13. [PubMed]
- Ye J, Chen X, Lu W. Identification and Experimental Validation of Immune-Associate lncRNAs for Predicting Prognosis in Cervical Cancer. Onco Targets Ther 2021;14:4721-34. [Crossref] [PubMed]
- Paoli P, Giannoni E, Chiarugi P. Anoikis molecular pathways and its role in cancer progression. Biochim Biophys Acta 2013;1833:3481-98. [Crossref] [PubMed]
- Jin L, Chun J, Pan C, et al. Phosphorylation-mediated activation of LDHA promotes cancer cell invasion and tumour metastasis. Oncogene 2017;36:3797-806. [Crossref] [PubMed]
- Kim H, Choi P, Kim T, et al. Ginsenosides Rk1 and Rg5 inhibit transforming growth factor-β1-induced epithelial-mesenchymal transition and suppress migration, invasion, anoikis resistance, and development of stem-like features in lung cancer. J Ginseng Res 2021;45:134-48. [Crossref] [PubMed]