Risk signature of NETosis-related subtype predicts prognosis and evaluates immunotherapy effectiveness in gastric cancer
Highlight box
Key findings
• NETosis, a recently discovered programmed cell death process, is tightly linked to the immune microenvironment of gastric cancer (GC). The NETosis-related risk signature (NRRS) effectively predicts GC patient survival.
What is known and what is new?
• GC, a highly prevalent cancer with a poor prognosis, is significantly impacted by NETosis in tumor biology.
• We discovered a correlation between NETosis subtypes and the tumor immune microenvironment in GC. We developed a validated NRRS to predict survival, immune infiltration, and drug sensitivity.
What is the implication, and what should change now?
• Our research deepens knowledge of NETosis and proposes fresh therapeutic approaches for GC.
Introduction
Gastric cancer (GC) is a widespread malignant tumor globally, ranking among the top five most common cancers. One of the challenges in managing GC is that its early clinical symptoms are often non-specific, which means patients may not experience significant discomfort or symptoms that clearly point to the disease. As a result, many patients are diagnosed at an advanced stage when the disease is already well-developed, leading to a less favorable prognosis (1-3). The primary treatment for GC continues to be surgical intervention, often supplemented by postoperative immunotherapy, chemotherapy, and other treatment modalities (4-6). Although recent advancements in medical technology have enhanced diagnostic and therapeutic options for GC, the mortality rate among patients remains high and continues to pose a significant challenge (7-9). Consequently, advancing the development of reliable biomarkers and disease models is essential for enhancing the prognosis of GC patients. These tools are vital for better patient outcomes, offering new avenues for therapeutic interventions (10-13).
Programmed cell death encompasses different types of cell death, such as pyroptosis, necroptosis, entosis, as well as ferroptosis and cuprotosis, which have been a hot research topic in recent years (14-18). NETosis is a newly defined mode of programmed cell death. Specifically, reticular DNA structures wrapped by histones, proteases, various antimicrobial proteins and cytoplasmic proteins are released by neutrophils to capture microorganisms and pathogens and are known as neutrophil extracellular traps (NETs) (19-21). NETs may be involved in various types of diseases such as autoimmune diseases, acute lung injury, sepsis and various processes of cancer (22-25).
Currently, NETosis is thought to play a crucial role in tumorigenesis (26), with prognostic features linked to certain malignancies (27-29). However, its specific function and mechanisms in GC remain unexplored, highlighting the need for further comprehensive research.
In our research, we constructed and validated a new NETosis-related risk signature (NRRS), evaluated the prognostic significance of NRRS in gastric cancer, and distinguished patients with varying degrees of sensitivity to immunotherapy, thereby laying the groundwork for enhanced patient-specific treatment strategies. We present this article in accordance with the TRIPOD reporting checklist (available at https://tcr.amegroups.com/article/view/10.21037/tcr-24-377/rc).
Methods
Data collection
In the training cohort, we obtained transcriptomic data from The Cancer Genome Atlas (TCGA, https://portal.gdc.cancer.gov/) database’s stomach adenocarcinoma (STAD) dataset, encompassing 375 tumor samples and 32 normal tissue samples. For validation, we utilized microarray gene chip data from the GSE84433 dataset on the GPL6947 platform from the Gene Expression Omnibus (GEO) database, including data from 357 patients. The study was conducted in accordance with the Declaration of Helsinki (as revised in 2013).
Consensus clustering analysis of NETosis-related subtypes
We selected 62 NETosis-related genes from previous studies, including BST1, CD93, CEACAM3, CREB5, CRISPLD2, CSF3R, CYP4F3, DYSF, CPPED1, G0S2, HPSE, CXCR1, CXCR2, KCNJ15, LILRB2, MGAM, PDE4B, S100A12, SIGLEC5, SLC22A4, SLC25A37, TECPR2, TNFRSF10C, VNN3, AKT1, AKT2, ATG7, CYBB, DNASE1, ENTPD4, HMGB1, IL1B, ITGAM, ITGB2, KCNN3, MAPK1, MAPK3, MMP9, MTOR, PTAFR, PIK3CA, RIPK1, RIPK3, SELP, SELPLG, SIGLEC14, TLR2, TLR4, TLR7, TLR8, CSF3, IL6, CTSG, MYD88, PLA2G7, GSDMD, CDK6, NOX4, AGER, MME, ALPL and CLEC6A.
We used ConsensusClusterPlus for cluster analysis. And the ideal number of clusters was established by combining statistical methods, including the consistent cumulative distribution function (CDF), area under the curve (AUC), and K-value. These techniques were utilized to ensure the stability and reliability of the clustering results.
Differentially expressed genes (DEGs) identification and analysis
Limma, a screening method rooted in generalized linear models, was utilized in this study to identify DEGs between different groups using the R package limma (version 3.40.6). Genes were considered significantly differentially expressed based on an adjusted P value <0.05 and a fold change >1.5. Furthermore, Gene Ontology (GO) and Kyoto Encyclopedia of Genes and Genomes (KEGG) analyses were conducted to compare the signaling pathways among NETosis clusters, employing statistical significance thresholds of P value and q-value <0.05.
Mutation landscape examination
Genetic mutations among STAD subtypes were explored using somatic mutation data from TCGA, visualized through waterfall plots.
Construction and validation of the NRRS
The least absolute shrinkage and selection operator (LASSO), a popular analysis approach, integrates variable selection and regularization. The non-zero coefficients derived from the LASSO regression represent the weights assigned to each gene in the final risk score model. Furthermore, we developed nomograms to estimate the survival outcomes of GC patients.
Immune cell infiltration analysis
Using the CIBERSORT algorithm, we analyzed the relative abundances of 22 distinct immune cell types. Additionally, we utilized the ESTIMATE algorithm to assess variations in Immune Score, Stromal Score, and ESTIMATE Score. To determine the correlation between the risk score and immune cells, we employed the Spearman correlation test. The Immune Score is a quantification of immune cell infiltration in a tumor microenvironment derived from gene expression data analysis. The Stromal Score assesses the presence of stromal cells within the tumor microenvironment. Lastly, the ESTIMATE Score, an algorithm combining immune and stromal scores, provides an estimation of tumor purity.
Drug sensitivity analysis
The sensitivity to chemotherapy and immunotherapy drugs is assessed by analyzing half-maximal inhibitory concentration (IC50) values. Utilizing the R software “pRRophetic”, we determined the IC50 values of various drugs in GC samples, enabling us to evaluate the association between risk levels and drug sensitivity.
Validation of NETosis-associated prognostic genes via immunohistochemical staining
Immunohistochemical staining data of GC and normal tissues were retrieved from the Human Protein Atlas (HPA, http://www.proteinatlas.org/), validating the protein expression of NETosis-related prognostic genes.
Statistical analysis
We identified genes differentially expressed between subtypes using Limma software. Kaplan-Meier curves evaluated overall survival (OS) across patient groups. LASSO regression was applied to pinpoint relevant prognostic genes. Correlation analysis examined the relationship between risk scores and immune cell infiltration, with statistical significance at P<0.05.
Results
Cluster analysis identified NETosis-related subtypes
Figure 1A illustrates that the majority of NETosis genes exhibited elevated expression levels in GC samples relative to normal tissues. Subsequent consistency clustering analysis revealed that the optimal clustering was achieved with K=2, as depicted in Figure 1B-1D. Additionally, the two identified subtypes exhibited distinct expression patterns of NETosis genes (Figure 1E,1F). Kaplan-Meier survival analysis indicated that patients in the C2 group had a more favorable prognosis (Figure 1G), with statistical significance at P=0.03.
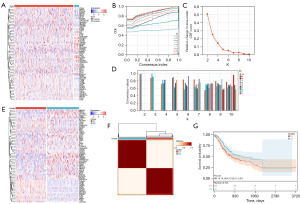
Differential expression analysis and enrichment profiling of NETosis-related subtypes
A comprehensive analysis of DEGs between the two NETosis subtypes yielded a total of 1,210 upregulated and 47 downregulated genes (Figure 2A). These genes displayed distinct expression patterns between the subtypes (Figure 2B). Further GO and KEGG enrichment analyses revealed that the majority of DEGs were involved in immune-related processes, including immune system regulation, cell migration, adaptive immune response, leukocyte migration, cytokine-cytokine receptor interaction, cancer-related pathways, and human papillomavirus infection (Figure 2C,2D).
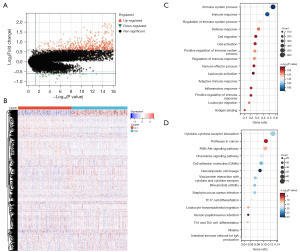
Comparison of somatic mutations, tumor microenvironment, and immune checkpoint across NETosis-related subtypes
Somatic mutations in patients from each category were examined, and a waterfall chart was generated (Figure 3A,3B). Within category C1, the genes most commonly affected by mutations were TTN, TP53, ARID1A, MUC16, and LRP1B. While in group C2, SYNE1 was the 5th most frequently mutated gene with a mutation frequency of 30.7%, which was higher than the mutation frequency of SYNE1 in group C1 (23.8%). In addition, TTN and TP53 also had much higher mutation frequencies in the C2 group (63.7%, 59.2%) than in the C1 group (47.6%, 40.2%).
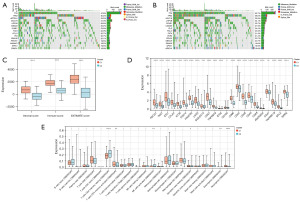
Our ESTIMATE analysis demonstrated higher scores in all three categories for group C1 compared to group C2 (Figure 3C). The evaluation of immune checkpoints (Figure 3D) showed increased expression in group C1 for most immune checkpoints. Furthermore, using CIBERSORT, we visualized the differences in immune cell infiltration between the two groups via a box-and-line plot (Figure 3E). The findings indicated greater infiltration of cells like activated CD4 memory T cells, resting dendritic cells, and neutrophils in the C1 group, while the C2 group exhibited higher infiltration of resting CD4 memory T cells and activated NK cells.
Development and verification of the NETosis-associated risk signature (NRRS)
We used the LASSO Cox method for regression analysis. Specifically, we integrate survival time, status, and gene expression data together (Figure 4A,4B). We set the lambda value to 0.055467178709963 and obtained 14 prognostic genes related to NETosis: CXCR4, NRP1, PDCD1, CTLA4, AKR1B1, SERPINE1, RGS2, SLCO2A1, TNFAIP2, RNASE1, DOC2B, APOD, ENTPD2, and CCL24. The non-zero coefficients from the LASSO regression represent the weights assigned to each gene in the final risk score model. The model formula for RiskScore is as follows: RiskScore = 0.028993043781327 × CXCR4 + 0.0314706454214018 × NRP1− 0.0941878557499203 × PDCD1 − 0.0610832396989102 × CTLA4 + 0.149627851386361 × AKR1B1 + 0.123843546915026 × SERPINE1 + 0.008120183886935 × RGS2 + 0.00732963996657683 × SLCO2A1 − 0.00734132828114282 × TNFAIP2 + 0.0387613971398363 × RNASE1 − 0.0548303763103828 × DOC2B + 0.0194583010341922 × APOD − 0.040210942582457 × ENTPD2 − 0.0107916247408867 × CCL24.
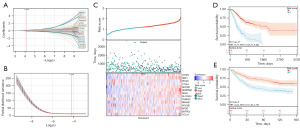
We were surprised to find that as the risk score increased, there was a significant decrease in the survival of patients. We found that CXCR4, NRP1, AKR1B1, SERPINE1, RGS2, SLCO2A1, RNASE1, APOD, and CCL24 were risk factors, their expression rose as the risk score increased. On the contrary, PDCD1, CTLA4, TNFAIP2, DOC2B, and ENTPD2 were protective factors, showing their expression decreased as the risk score increased (Figure 4C). Furthermore, we employed Kaplan-Meier analysis to ascertain the prognostic significance of the NRRS in GC patients. In the training set, the low-risk group had a better prognosis (P=7.4×−17) (Figure 4D), GSE84433 survival of the validation set showed a similar result (P=5.0×10−6) (Figure 4E).
The association of NRRS with prognosis
Our multivariate Cox regression analysis identified the NRRS as an independent prognostic factor for OS in GC patients (Figure 5A). We constructed nomograms and to improve survival predictions (Figure 5B,5C). In the receiver operating characteristic ROC analysis, the AUC values for the one-, three-, and five-year periods were 0.76, 0.81, and 0.85 (Figure 5D). Therefore, these results indicate that the NRRS is an effective tool for predicting the survival rate of GC patients.
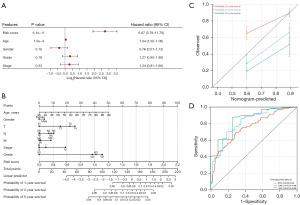
Exploration of NRRS correlation with drug sensitivity and tumor microenvironment
The risk score positively correlated with infiltrating monocytes and M2 macrophages while negatively correlating with activated CD4 memory T cells and T follicular helper cells in both the training (Figure 6A-6D) and validation sets (Figure 6E-6H).
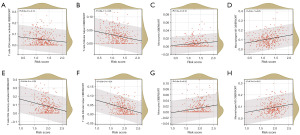
We screened compounds using predicted IC50 values, revealing drug sensitivities among patient groups (Figure 7). Low-risk patients exhibited greater sensitivity to Bcl-2 inhibitors (ABT-263), veliparib (ABT-888), Chk inhibitors (AZD7762), camptothecin, lestaurtinib (CEP-701), ATM inhibitors (KU-55933), methotrexate, B-RafV600E inhibitors (PLX4720), sunitinib, and vinblastine. Conversely, high-risk patients were more responsive to Akt inhibitors such as A-443654, AKT inhibitor VIII, saracatinib (AZD-0530), afatinib (BIBW2992), dasatinib, and elesclomol. These findings suggest that individualized drug selection may be beneficial for different groups of patients.
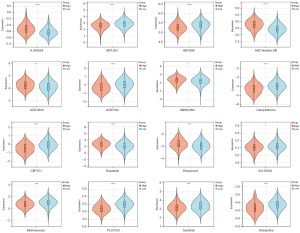
Validation of NETosis-related prognostic genes via protein levels reveals promising prognostic indicators
We examined the levels of crucial genes associated with NETosis in both GC and healthy tissues by utilizing data from HPA immunohistochemical staining (Figure 8). Our analysis revealed a notable increase in APOD levels in GC tissues, while ENTPD2 and PDCD1 showed a decrease in expression. Conversely, NRP1 and SERPINE1 displayed minimal variance between tumor and normal tissues. Regrettably, data for additional genes was unavailable for our study. Collectively, our findings suggest that these specific markers could potentially serve as significant indicators in the context of GC progression.
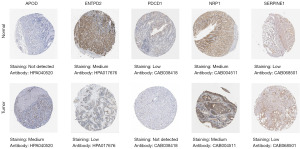
Discussion
GC remains a major threat to human health worldwide, especially in Asian countries, where morbidity and mortality remain high. Researchers are also constantly working to find tools that can help predict or improve outcomes for patients with GC. Encouragingly, various gene models related to programmed death have been developed, such as pyroptosis, necroptosis, entosis, as well as the popular ferroptosis and cuprotosis, etc. (14-18), which effectively accelerates the process of GC biological targets and potential therapies.
NETosis is a newly discovered novel mode of cell death that has been less studied. NETosis is morphologically different from apoptosis and autophagy, NETosis exhibits reticular DNA structures released by neutrophils to capture microorganisms and pathogens. There are fewer studies in this area, but the emergence of this death mechanism has provided new ideas for cancer treatment (25-29). The pathway and mechanism of its involvement in GC have not been reported. Researchers still need to study NETosis in depth.
In our research, we have identified two distinct subtypes of NETosis by analyzing the expression of 62 NETosis-related genes. Using advanced statistical methods such as limma and Cox-LASSO, we were able to pinpoint 14 genes that play a crucial role in these subtypes and constructed the NRRS. These genes are believed to be involved in cancer development and inflammation by affecting the body’s immune response. One of the key genes we identified, NRP1, has been shown to have contrasting effects on different types of cancer. Overexpression of NRP1 can enhance the growth and movement of prostate cancer cells (30), while its downregulation may contribute to bladder cancer progression (31). Another important finding is the role of arachidonic acid (ARA) when bound to APOD in regulating inflammation and oxidative stress (32). The administration of antiplatelet therapy has been observed to elevate the levels of SERPINE1 in cancer cells, which in turn has been linked to an increase in cell motility and an enhanced propensity for colon cancer metastasis (33). Based on our analysis of these 14 genes, we found that the NRRS we developed showed a better prognosis for survival. In conclusion, our research suggests that NETosis could serve as a promising therapeutic target and potentially function as a diagnostic and prognostic biomarker for GC. By understanding the intricate mechanisms of these genes and their impact on cancer development, we may pave the way for more effective treatments and personalized medicine approaches in the future.
The GO and KEGG analyses highlighted distinct immune-related processes that the different groups were engaged in. These included the regulation of immune system processes, cell migration, leukocyte activation, adaptive immune responses, leukocyte migration, and cytokine-cytokine receptor interactions. Furthermore, pathways in cancer, human papillomavirus infection, Th17 cell differentiation, and Th1 and Th2 cell differentiation were also identified. These findings shed light on the reasons behind the improved survival rates of GC patients in group C2, showcasing the importance of immune responses in patient outcomes.
The analysis of CIBERSORT results revealed a notable disparity in immune cell infiltration between group C1 and group C2. Specifically, group C1 exhibited increased levels of activated CD4 memory T cells, resting dendritic cells, and neutrophils, while group C2 showcased higher amounts of resting CD4 memory T cells and activated NK cells. Natural killer cells, known for their potent anti-cancer function in immunotherapy (34,35), were found to be more prevalent in the C2 group, emphasizing their significance in targeting cancerous cells. Memory CD4 T cells, on the other hand, play a crucial role in mounting a swift and efficient secondary immune response, thus accelerating the systemic immune reaction (36). Additionally, dendritic cells, renowned for their capacity to activate naïve T cells and facilitate effector differentiation (37,38), were also more abundant in the C2 group. This disparity in immune cell composition likely contributes to the superior survival outcomes observed in group C2. Further analysis uncovered higher expression levels of immune checkpoints in the C1 group compared to the C2 group. This suggests that immunotherapy may yield more favorable results for patients in the C1 group, aligning with previous research findings. These insights underscore the importance of understanding and leveraging the distinct immune cell profiles in guiding personalized treatment strategies for cancer patients.
Moreover, we also selected a variety of drugs based on the IC50 prediction, and the high- and low-risk groups had different sensitivities to some of these drugs, which may help patients in different groups to choose more effective and personalised drug regimens. Finally, we queried the HPA database and in turn assessed the immunohistochemical staining of NETosis-associated prognostic genes in normal tissues and GCs. It is clear that these markers are likely to play a role in GC. Taken together, the NRRS we developed can more accurately predict the survival prognosis of GC patients in advance, and has a better guiding role for diagnostic and therapeutic markers of GC.
It is acknowledged that this study is not without its limitations. Firstly, Bioinformatics analysis methods have a wide range of applications, including but not limited to drug design and screening, protein structure prediction, genome-wide chain analysis, and so on. It plays an important role in various aspects of the medical field. Nevertheless, it is our hope that in the future, we will be able to apply western blot, polymerase chain reaction (PCR), and other experiments to validate the results in this paper. Secondly, our findings are in need of further validation in an external GC queue. In conclusion, this paper presents the findings of a retrospective study. To confirm the results, further prospective, multicentre studies are required.
Conclusions
In conclusion, our study highlights the association of NETosis subtypes with GC. NRRS were also established and their effects were validated in other cohorts. This model is of great significance with GC. The aforementioned results may facilitate a more profound comprehension of NETosis and offer novel avenues for tailored therapeutic interventions.
Acknowledgments
Funding: This research was supported by
Footnote
Reporting Checklist: The authors have completed the TRIPOD reporting checklist. Available at https://tcr.amegroups.com/article/view/10.21037/tcr-24-377/rc
Peer Review File: Available at https://tcr.amegroups.com/article/view/10.21037/tcr-24-377/prf
Conflicts of Interest: All authors have completed the ICMJE uniform disclosure form (available at https://tcr.amegroups.com/article/view/10.21037/tcr-24-377/coif). The authors have no conflicts of interest to declare.
Ethical Statement: The authors are accountable for all aspects of the work in ensuring that questions related to the accuracy or integrity of any part of the work are appropriately investigated and resolved. The study was conducted in accordance with the Declaration of Helsinki (as revised in 2013).
Open Access Statement: This is an Open Access article distributed in accordance with the Creative Commons Attribution-NonCommercial-NoDerivs 4.0 International License (CC BY-NC-ND 4.0), which permits the non-commercial replication and distribution of the article with the strict proviso that no changes or edits are made and the original work is properly cited (including links to both the formal publication through the relevant DOI and the license). See: https://creativecommons.org/licenses/by-nc-nd/4.0/.
References
- Shin WS, Xie F, Chen B, et al. Updated Epidemiology of Gastric Cancer in Asia: Decreased Incidence but Still a Big Challenge. Cancers (Basel) 2023;15:2639. [Crossref] [PubMed]
- Siegel RL, Miller KD, Fuchs HE, et al. Cancer statistics, 2022. CA Cancer J Clin 2022;72:7-33. [Crossref] [PubMed]
- Yang WJ, Zhao HP, Yu Y, et al. Updates on global epidemiology, risk and prognostic factors of gastric cancer. World J Gastroenterol 2023;29:2452-68. [Crossref] [PubMed]
- Joshi SS, Badgwell BD. Current treatment and recent progress in gastric cancer. CA Cancer J Clin 2021;71:264-79. [Crossref] [PubMed]
- Ilson DH. Advances in the treatment of gastric cancer: 2022-2023. Curr Opin Gastroenterol 2023;39:517-21. [Crossref] [PubMed]
- Guan WL, He Y, Xu RH. Gastric cancer treatment: recent progress and future perspectives. J Hematol Oncol 2023;16:57. [Crossref] [PubMed]
- Song Z, Wu Y, Yang J, et al. Progress in the treatment of advanced gastric cancer. Tumour Biol 2017;39:1010428317714626. [Crossref] [PubMed]
- Siebenhüner AR, De Dosso S, Helbling D, et al. Advanced Gastric Cancer: Current Treatment Landscape and a Future Outlook for Sequential and Personalized Guide: Swiss Expert Statement Article. Oncol Res Treat 2021;44:485-94. Erratum in: Oncol Res Treat 2022;45:62. [Crossref] [PubMed]
- Tan Z. Recent Advances in the Surgical Treatment of Advanced Gastric Cancer: A Review. Med Sci Monit 2019;25:3537-41. [Crossref] [PubMed]
- Röcken C. Predictive biomarkers in gastric cancer. J Cancer Res Clin Oncol 2023;149:467-81. [Crossref] [PubMed]
- Zeng Y, Jin RU. Molecular pathogenesis, targeted therapies, and future perspectives for gastric cancer. Semin Cancer Biol 2022;86:566-82. [Crossref] [PubMed]
- Lei ZN, Teng QX, Tian Q, et al. Signaling pathways and therapeutic interventions in gastric cancer. Signal Transduct Target Ther 2022;7:358. [Crossref] [PubMed]
- Yuan L, Xu ZY, Ruan SM, et al. Long non-coding RNAs towards precision medicine in gastric cancer: early diagnosis, treatment, and drug resistance. Mol Cancer 2020;19:96. [Crossref] [PubMed]
- Obeng E. Apoptosis (programmed cell death) and its signals - A review. Braz J Biol 2021;81:1133-43. [Crossref] [PubMed]
- D’Arcy MS. Cell death: a review of the major forms of apoptosis, necrosis and autophagy. Cell Biol Int 2019;43:582-92. [Crossref] [PubMed]
- Ketelut-Carneiro N, Fitzgerald KA. Apoptosis, Pyroptosis, and Necroptosis-Oh My! The Many Ways a Cell Can Die. J Mol Biol 2022;434:167378. [Crossref] [PubMed]
- Jiang X, Stockwell BR, Conrad M. Ferroptosis: mechanisms, biology and role in disease. Nat Rev Mol Cell Biol 2021;22:266-82. [Crossref] [PubMed]
- Xie J, Yang Y, Gao Y, et al. Cuproptosis: mechanisms and links with cancers. Mol Cancer 2023;22:46. [Crossref] [PubMed]
- Rada B. Neutrophil Extracellular Traps. Methods Mol Biol 2019;1982:517-28. [Crossref] [PubMed]
- Papayannopoulos V. Neutrophil extracellular traps in immunity and disease. Nat Rev Immunol 2018;18:134-47. [Crossref] [PubMed]
- Adrover JM, McDowell SAC, He XY, et al. NETworking with cancer: The bidirectional interplay between cancer and neutrophil extracellular traps. Cancer Cell 2023;41:505-26. [Crossref] [PubMed]
- Wigerblad G, Kaplan MJ. Neutrophil extracellular traps in systemic autoimmune and autoinflammatory diseases. Nat Rev Immunol 2023;23:274-88. [Crossref] [PubMed]
- Scozzi D, Liao F, Krupnick AS, et al. The role of neutrophil extracellular traps in acute lung injury. Front Immunol 2022;13:953195. [Crossref] [PubMed]
- Zhang H, Wang Y, Qu M, et al. Neutrophil, neutrophil extracellular traps and endothelial cell dysfunction in sepsis. Clin Transl Med 2023;13:e1170. [Crossref] [PubMed]
- Olsson AK, Cedervall J. NETosis in Cancer - Platelet-Neutrophil Crosstalk Promotes Tumor-Associated Pathology. Front Immunol 2016;7:373. [Crossref] [PubMed]
- Masucci MT, Minopoli M, Del Vecchio S, et al. The Emerging Role of Neutrophil Extracellular Traps (NETs) in Tumor Progression and Metastasis. Front Immunol 2020;11:1749. [Crossref] [PubMed]
- Tu Y, Mao Z. Identification and Validation of Molecular Subtype and Prognostic Signature for Bladder Cancer Based on Neutrophil Extracellular Traps. Cancer Invest 2023;41:354-68. [Crossref] [PubMed]
- He X, Xiao Y, Liu S, et al. Predicting the Immune Microenvironment and Prognosis with a NETosis-Related lncRNA Signature in Head and Neck Squamous Cell Carcinoma. Biomed Res Int 2022;2022:3191474. [Crossref] [PubMed]
- Feng C, Li Y, Tai Y, et al. A neutrophil extracellular traps-related classification predicts prognosis and response to immunotherapy in colon cancer. Sci Rep 2023;13:19297. [Crossref] [PubMed]
- Zhang P, Chen L, Zhou F, et al. NRP1 promotes prostate cancer progression via modulating EGFR-dependent AKT pathway activation. Cell Death Dis 2023;14:159. [Crossref] [PubMed]
- Dong Y, Ma WM, Shi ZD, et al. Role of NRP1 in Bladder Cancer Pathogenesis and Progression. Front Oncol 2021;11:685980. [Crossref] [PubMed]
- Fyfe-Desmarais G, Desmarais F, Rassart É, et al. Apolipoprotein D in Oxidative Stress and Inflammation. Antioxidants (Basel) 2023;12:1027. [Crossref] [PubMed]
- Kim WT, Mun JY, Baek SW, et al. Secretory SERPINE1 Expression Is Increased by Antiplatelet Therapy, Inducing MMP1 Expression and Increasing Colon Cancer Metastasis. Int J Mol Sci 2022;23:9596. [Crossref] [PubMed]
- Preethy S, Dedeepiya VD, Senthilkumar R, et al. Natural killer cells as a promising tool to tackle cancer-A review of sources, methodologies, and potentials. Int Rev Immunol 2017;36:220-32. [Crossref] [PubMed]
- Wu SY, Fu T, Jiang YZ, Shao ZM. Natural killer cells in cancer biology and therapy. Mol Cancer. 2020;19:120. [Crossref] [PubMed]
- Künzli M, Masopust D. CD4(+) T cell memory. Nat Immunol 2023;24:903-14. [Crossref] [PubMed]
- Wculek SK, Cueto FJ, Mujal AM, et al. Dendritic cells in cancer immunology and immunotherapy. Nat Rev Immunol 2020;20:7-24. [Crossref] [PubMed]
- Patente TA, Pinho MP, Oliveira AA, et al. Human Dendritic Cells: Their Heterogeneity and Clinical Application Potential in Cancer Immunotherapy. Front Immunol 2019;9:3176. [Crossref] [PubMed]