Establishment and verification of a prognostic immune cell signature-based model for breast cancer overall survival
Highlight box
Key findings
• The study established a novel prognostic model based on immune cell signatures derived from scRNA-seq and validated it using clinical data from 95 breast cancer (BRCA) patients.
• Poor prognosis significantly and positively correlated with factors of age, tumor size, B-cell level, and CTLA4 level. Meanwhile, predictive model was negatively correlated with CD3+, CD4+, and CD8+T-cell levels; neutrophil, lymphocyte, and natural killer cell levels; TIGIT expression; and clinical stage (I/II).
What is known and what is new?
• The association between immune cell infiltration and prognosis has been understood and described.
• A predictive model for BRCA prognosis using immune cell signatures was developed.
What is the implication, and what should change now?
• Personalized treatment strategies: the model provides valuable predictive insights that can significantly enhance personalized treatment planning and stratification for patients with BRCA.
• New therapeutic targets: the identification of specific immune cell signatures and their correlation with prognosis can guide the development of new immunotherapeutic interventions and combination therapies.
• Enhanced prognostication: the model is a refined tool that can be used by clinicians to predict patient outcomes more accurately, potentially improving OS rates and quality of care in BRCA management.
Introduction
Breast cancer (BRCA) is a prevalent malignancy that impacts a considerable number of women, being responsible for around 23% of all female cancer cases (1,2). The unfortunate reality is that it is associated with one of the lowest survival rates among all malignant tumors impacting women (3). In the United States in 2019, around 276,480 women received a diagnosis of BRCA, 42,170 of whom died (3,4).
There are several medical alternatives that can be used to treat patients with BRCA, including chemotherapy, surgery, radiation therapy, and hormonal treatment (4). Despite various treatments being applied, a significant number of patients continue to experience unfavorable prognoses even when the diagnosis is made at an early stage (5,6), and predicting survival remains challenging due to the heterogeneity of BRCA. The latest research findings suggest that BRCA possesses a remarkable degree of immunogenicity, characterized by the infiltration of diverse immune cell populations (7). Therefore, there may exist a correlation between immune cell infiltration and the prognosis of individuals with BRCA. Recent studies have shown numerous risk models that can be used for predicting BRCA prognosis (8,9). Currently available prognosis prediction models for BRCA, provide valuable information but often lack the specificity and sensitivity needed for accurate long-term survival estimates (10-12).
The cancer immune microenvironment is a critical factor in the progression of BRCA (13). Tumor-infiltrating lymphocytes (TILs), which are among the known indicators of the immune ecosystem in tumorous lesions, have been comprehensively analyzed, and their correlation with the prognosis of BRCA has been examined (14,15). Recent studies suggest that TILs are not only correlated with the response to chemotherapy but also that of immunotherapy (16,17). Therefore, a comprehensive understanding of the cancer immune ecosystem may provide valuable insights into prognostic indicators and the identification of novel targets for both chemotherapy and immunotherapy in patients with BRCA (18,19).
Immunotherapy, which involves immune checkpoint blockers or adoptive cell therapy, is a promising clinical approach for the treatment progressive hematological and solid malignancies (20). The role of T cells as essential effectors against tumors and prognostic indicators is pivotal for the success of these therapies (20,21). Recent research interest in TILs has intensified as their critical role in the immune system has been increasingly recognized (22,23). Studies have identified the infiltration of inflammatory and lymphocytic cells to be vital to the tumor microenvironment (TME) (24,25). The TME, particularly the presence and activity of immune cells, has emerged as a critical factor influencing patient outcomes. Research has shown that the TME’s immunogenicity, characterized by the infiltration of diverse immune cell populations, correlates strongly with prognosis (26,27). Therefore, developing a predictive model based on TME genes could offer a more accurate and reliable method for prognosis prediction.
The development of immunotherapies marks a shift in focus from targeting cancer cells to the modulation of the immune system, particularly by targeting T cells and inhibitory receptors such as cytotoxic T lymphocyte-associated protein-4 (CTLA-4) (28,29). Recent research has investigated multiple compounds targeting diverse costimulatory or coinhibitory receptors on immune cells (T cells) for the treatment of various types of cancer (30,31). The identification of specific immune cell signatures associated with favorable or adverse prognosis holds significant implications for risk assessment, treatment stratification, and the advancement of groundbreaking immunotherapies. Additionally, the characterization of genomic alterations, immune checkpoint expression patterns, and the TME in relation to prognostic model aims clarify mechanisms underlying immune evasion and resistance to therapy in BRCA.
The interplay among TILs, tumor cells, and the surrounding microenvironment is being increasingly acknowledged as an essential determinant of clinical outcomes in BRCA. The characterization of the immune landscape within the TME can not only provide valuable insights into prognosis but also presents potential avenues for novel immunotherapeutic targets. This study aimed to examine the complexities of immune responses within BRCA, establish a predictive framework based on immune cell signatures, and assess its prognostic and therapeutic value. It is hoped that this research will contribute to the development of more effective prognostic and therapeutic strategies for managing this complex disease. We present this article in accordance with the TRIPOD reporting checklist (available at https://tcr.amegroups.com/article/view/10.21037/tcr-24-1829/rc).
Methods
The acquisition of single-cell transcriptome sequencing data
Using the keyword “breast cancer”, we retrieved the GSE169246 dataset from the Gene Expression Omnibus (GEO) database (https://www.ncbi.nlm.nih.gov/gds). This dataset contains the single-cell RNA sequencing (scRNA-seq) data from 95 individuals with BRCA. All patients with BRCA were diagnosed based on BRCA diagnostic guidelines. Among them, 20 patients were in stage I, 35 in stage II, 25 in stage III, and 15 in stage IV. The majority were Caucasian, accounting for approximately 52.63%. Follow-up data were collected within 6 years after diagnosis, recording detailed survival information. Raw sequences of cell types of were annotated according to a previous study by Zhang et al. (32).
Processing of scRNA-seq data
T-cell transcriptome sequencing data were extracted and analyzed using the Seurat software package (v. 4.1.1) in R v. 4.3.2 (The R Foundation for Statistical Computing), which is commonly used for single-cell analyses. Gene exhibiting remarkable variability was then identified through rigorous analysis of variance and was included in data scaling and centralization. Principal component analysis (PCA) was then conducted on these variable genes for linear dimension reduction. A total of 35 principal components (PCs) were selected based on the graph-based clustering (resolution =0.4) method, which can group cells into different subpopulations based on gene expression profiles. The cell population was visualized based on the unified matrix polynomial approach (UMAP) dimension reduction method. Finally, the T cells’ differentially expressed genes (DEGs) were identified by implementing an adjusted P value (Pval.adj) of 0.05 as the criterion to define significant gene expression change.
Data collection and processing
A comprehensive search for publicly accessible BRCA gene expression profiles was conducted. Samples lacking complete prognostic information were excluded. We acquired four microarray datasets from GEO (GSE65194, GSE58812, GSE20711, and GSE20685) and one RNA-seq dataset from The Cancer Genome Atlas (TCGA; TCGA-BRCA; https://www.cancer.gov/ccg/research/genome-sequencing/tcga). The gene expression data for BRCA, along with clinical information of the patients, somatic mutation status data, and tumor mutational burden (TMB) were downloaded from TCGA database. To detect amplified or deleted genes, we conducted a copy number analysis using GISTIC_2.0 (https://www.genepattern.org/modules/docs/GISTIC_2.0).
The variations in dataset platforms present a potential confounding factor that warrants careful consideration. We recognized the importance of addressing this discrepancy to ensure the robustness of our findings. To mitigate the impact of platform variations, we used standardized data preprocessing and normalization techniques tailored to each specific platform. Leveraging advanced bioinformatics tools and cross-platform normalization algorithms, we harmonized the gene expression profiles to minimize the effects of platform-specific biases. Furthermore, prior to integrative analysis, we conducted extensive validation procedures to ascertain the coherence and comparability of the datasets, thus reducing the potential confounding influence introduced by the variation in platforms.
The data preprocessing methods, including normalization, batch effect correction, and missing value imputation, closely followed established protocols as detailed in the works of Bacher et al. (33). Specifically, the quantile normalization approach was employed for RNA-seq data. Similarly, for microarray data, robust multi-array average (RMA) normalization was employed, adhering to the recommended procedures described by Bolstad et al. (34).
Pathway enrichment and immune infiltration analysis
To identify the DEGs, we applied the cluster analyzer package (v. 4.0.5 in R software) to conduct Gene Ontology (GO) annotation and Kyoto Encyclopedia of Genes and Genomes (KEGG; https://www.kegg.jp/) pathway enrichment analyses. We employed microenvironment cell population counting (MCP-Counter) to estimate immune infiltration. This method allows for the absolute quantification of ten types of immune and stromal cell populations within heterogeneous tissues from transcriptome data. To transform messenger RNA (mRNA) data into non-tumor cell infiltrating levels in the TME, we applied the “IOBR” package in R software (v. 4.3.2). Prior to MCP-Counter analysis, the gene expression profile was prepared using standard annotation files.
Clinical data
A retrospective analysis was conducted on the clinical data of 95 patients with BRCA who underwent surgical treatment from October 2018 to October 2021 at the Second Affiliated Hospital of Qiqihar Medical University. Based on their prognosis, these patients were placed into a favorable outcome group or an unfavorable outcome group. The inclusion criteria were as follows: (I) no prior radiation, chemotherapy, or endocrine therapy before surgery; (II) meeting the relevant diagnostic criteria outlined in the “Chinese Breast Cancer Screening and Early Diagnosis Guidelines”; (III) completion of at least 6–8 cycles of postoperative chemotherapy, or radiation and targeted therapy, and thorough examination and test data available; (IV) stage I–IIIA disease; (V) expected survival >3 months; (VI) age >18 years; and (VII) normal mental and cognitive function. Meanwhile, the exclusion criteria were as follows: (I) initial diagnosis of BRCA with distant metastasis; (II) significant diseases of vital organs such as the heart, brain, lungs, or kidneys; (III) a history of intracranial tumors or traumatic brain injury; (IV) a known allergy to chemotherapy drugs, targeted drugs, or contrast agents; and (V) incomplete case data. Follow-up data were collected within 6 years after diagnosis, recording detailed survival information.
Ethical statement
The study was conducted in accordance with the Declaration of Helsinki (as revised in 2013). This study was approved by the Ethics Committee of the Second Affiliated Hospital of Qiqihar Medical University (No. [2022] 0815-9). Informed consent was waived by the Ethics Committee of the Second Affiliated Hospital of Qiqihar Medical University for this retrospective study due to the exclusive use of deidentified patient data, which posed no potential harm or impact on patient care.
Data collection
Patients’ general information, including age, surgical method, and tumor staging, was retrieved through the medical record system. The overall survival (OS) of patients at 2, 4, and 6 years was calculated. Levels of T lymphocytes, B lymphocytes, natural killer (NK) cells, and lymphocytes, as well as TIGIT expression, in peripheral blood were assessed by extracting 2 mL of patient antecubital venous blood into a vacuum tube containing ethylenediaminetetraacetic acid tripotassium anticoagulant. Subsequently, 50 µL of whole blood was combined with 5 µL of monoclonal fluorescent antibodies, mixed, and incubated at room temperature in the dark for 15 minutes; 300 µL of lysing reagent was added, mixed, and left at room temperature in the dark for 10 minutes; 300 µL of phosphate-buffered saline was added and mixed, and within 2 hours, flow cytometry (Navios EX Flow Cytometer, Beckman Coulter, Brea, CA, USA) was used for detection. Absolute lymphocyte count was determined using a blood cell analyzer (XN-2000, Sysmex, Kobe, Japan). An enzyme-linked immunosorbent assay was used to measure patient serum levels of CTLA4 and neutrophils.
Statistical analysis
To compare the normality of variables between groups, we employed the Shapiro-Wilk normality test. For normally distributed variables, we performed the unpaired Student t-test, while for nonnormally distributed variables, we used the Mann-Whitney test. We applied one-way analysis of variance and the Kruskal-Wallis test to compare the two groups. Spearman rank correlation analysis was conducted to calculate correlation coefficients. Survival rates were determined via the Kaplan-Meier method, and differences in survival curves were assessed with the log-rank test. To eliminate the heterogeneity between tumor types, we recalculated the optimal threshold for every prognostic indicator using the “survminer” package in R software. In this study, both univariate and multivariate Cox proportional risk model analyses were implemented. Moreover, we assessed the prognostic model’s survival prediction accuracy using receiver operating characteristic (ROC) curve analysis and the Harrell consistency index (C-index). We completed all statistical analyses using R software v. 3.5.0 and SPSS 25 (IBM Corp., Armonk, NY, USA). A two-tailed P value <0.05 was considered to indicate statistical significance.
Results
The detection of pharmacodynamics-related genes for T cells based on BRCA scRNA-seq data
To detect predictive indicators for treatment effectiveness prior to therapy, we obtained 1,971 T cells sampled from breast tissue, which were sequenced before treatment, with their associated with response information, including partial response (PR) and stable disease (SD) also being determined. Analysis of variance identified the top 10 DEGs in each sample. We used PCA to identify genes that were highly correlated with each component (Figure 1A). Additionally, we applied the UMAP algorithm, commonly used for visualizing high-dimensional data, and linear dimensionality reduction methods to classify cell populations with greater accuracy (Figure 1B). The analysis yielded 14 different subgroups of T cells, including PR and SD cells, from patients with BRCA (Figure 1C). Furthermore, we identified 4,761 DEGs that were found in both PR and SD T cells (Figure 1D).
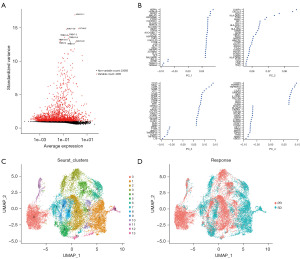
Prognostic characteristics of T-cell core pharmacodynamics-related genes were constructed in the TCGA dataset
Gene expression profiling identified 3,195 DEGs. Among these genes, 896 genes were upregulated [log fold change (FC) >1 and Pval.adj <0.05] while 2,299 genes were downregulated (logFC <–1 and Pval.adj <0.05) in BRCA tissues compared to normal control tissues (Figure 2A). To examine the association between gene expression features with the prognosis of patients with BRCA, OS analysis was conducted, which revealed 1,691 significant genes (P<0.05). We identified the DEGs that overlapped with genes associated with prognosis and T-cell pharmacodynamics-related genes to obtain 54 T-cell core pharmacodynamics-related genes (Figure 2B).
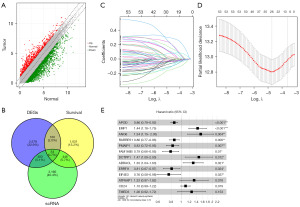
To develop a clinical prognostic model, the dimensionality of 54 prognostic genes was reduced by employing a least absolute shrinkage and selection operator (LASSO) Cox regression model. Subsequently, 26 genes were obtained, based on which a prognostic model was established through a Cox analysis of OS (Figures 2C,2D). The formula for the risk score of this model is as follows: risk score = (–0.15607676 × APOD expression) + (0.36138608 × EMP1 expression) + (0.42949380 × ANO6 expression) + (–0.15424855 × RARRES1 expression) + (–0.19074140 × PMAIP1 expression) + (–0.23465677 × FAM166B expression) + (0.38785733 × DCTPP1 expression) + (–0.26348829 × ABRACL expression) + (–0.21325435 × ERRFI1 expression) + (–0.27636725 × EIF4E3 expression) + (0.28325690 × ATP6AP1 expression) + (0.09527852 × CD24 expression) + (0.22876573 × TMED3 expression) (Figure 2E). This formula, in combination with an analysis of the expression of the included genes, could provide the risk score.
Risk model confirmation in the GEO dataset
The risk score for TCGA-BRCA was computed through use of the established model. To evaluate the capability of our model as a prognostic tool, we divided TCGA-BRCA into subgroups based on low- and high-risk scores. The surv_cutpoint function in the “survminer” R package was applied to calculate an optimal cutoff point. Individuals with high-risk scores demonstrated a shorter median survival time than did those with low-risk scores (2,447 and 6,498 days, respectively), with a P value of 4.733e−12 according to the Fisher exact test (Figure 3A). The gene expression profiles of 13 genes in both the high-risk and low-risk patient of TCGA-BRCA were also determined (Figure 3B). The high-risk group exhibited significantly unfavorable prognoses, with an increased chance of cancer-related death in TCGA-BRCA [hazard ratio (HR) =2.7, 95% confidence interval (CI): 2.2–3.3; P<0.0001; Figure 3B]. We also plotted an ROC curve, as displayed in Figure 3C, where the time-dependent ROC graph displays area under the curve (AUC) values of 0.75, 0.75, and 0.72 for the 2-, 4-, and 6-year OS predictions, respectively. In addition, we validated the risk score through GSE58812 (Figure 3D-3F), GSE20711 (Figure 4A-4C), and GSE20685 (Figure 4D-4F), obtaining similar predictive AUC values.
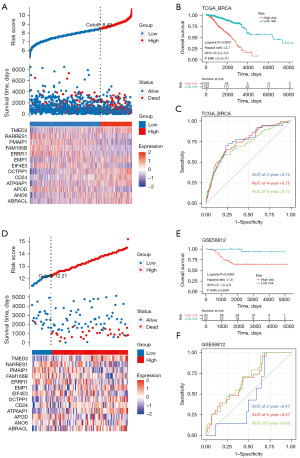
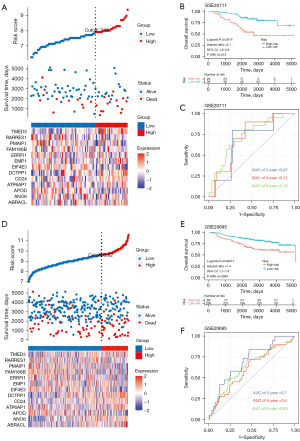
Patients’ general information
We further clinically validated the accuracy of the model. A comparison of the general information of the two patient groups revealed that the patients in the unfavorable prognosis group were significantly older than those in the favorable prognosis group (50.24±3.23 vs. 48.75±3.16; t=2.138; P=0.03) (Table 1). Compared to those in favorable prognosis group, patients in the unfavorable prognosis group exhibited significantly larger tumor size (4.25±0.87 vs. 3.84±0.76 cm; t=2.247; P=0.02), higher tumor T stage (37.5% vs. 61.9%; χ2=4.149; P=0.04), greater lymph node metastasis (40.62% vs. 65.08%; χ2=4.224; P=0.04), and higher clinical stage (34.38% vs. 68.25%; χ2=8.596; P=0.003). These findings aligned with the risk factors identified in our model, emphasizing the significant impact of age and tumor characteristics on prognosis.
Table 1
Parameter | Favorable prognosis (n=63) | Unfavorable prognosis (n=32) | t/χ2 | P |
---|---|---|---|---|
Age (years) | 48.75±3.16 | 50.24±3.23 | 2.138 | 0.03 |
BMI (kg/m2) | 23.38±3.54 | 23.87±3.29 | 0.667 | 0.50 |
Race | 0.347 | 0.56 | ||
Han | 50 (79.37) | 27 (84.38) | ||
Others | 13 (20.63) | 5 (15.62) | ||
Smoking history | 8 (12.70) | 5 (15.62) | 0.006 | 0.93 |
Alcohol history | 14 (22.22) | 9 (28.12) | 0.145 | 0.70 |
Family history of cancer | 4 (6.35) | 1 (3.12) | 0.032 | 0.85 |
Education level | 0.016 | 0.89 | ||
Junior high school and below | 22 (34.92) | 10 (31.25) | ||
Junior high school or above | 41 (65.08) | 22 (68.75) | ||
Marital status | 0.152 | 0.92 | ||
Married | 41 (65.08) | 22 (68.75) | ||
Divorce | 16 (25.40) | 7 (21.88) | ||
Other | 6 (9.52) | 3 (9.38) | ||
Breast surgery | 0.291 | 0.59 | ||
Breast conservation | 6 (9.52) | 5 (15.62) | ||
Mastectomy | 57 (90.48) | 27 (84.38) | ||
Radiotherapy | 0.034 | 0.85 | ||
Yes | 44 (69.84) | 21 (65.62) | ||
No | 19 (30.16) | 11 (34.38) | ||
Tumor size (cm) | 3.84±0.76 | 4.25±0.87 | 2.247 | 0.02 |
Tumor staging (T) | 4.149 | 0.04 | ||
T1/T2 | 39 (61.90) | 12 (37.50) | ||
T3/T4 | 24 (38.10) | 20 (62.50) | ||
Lymph node involvement | 4.224 | 0.04 | ||
N0 | 41 (65.08) | 13 (40.62) | ||
N1-3 | 22 (34.92) | 19 (59.38) | ||
Clinical staging | 8.596 | 0.003 | ||
I/II | 43 (68.25) | 11 (34.38) | ||
III/IV | 20 (31.75) | 21 (65.62) |
Data are presented as mean ± standard deviation or n (%). BMI, body mass index.
Immunity analysis
As shown in Table 2, the levels of CD3+T cells (796.28±292.16 vs. 948.73±285.34; t=2.423; P=0.01), CD4+T cells (367.38±119.25 vs. 433.26±124.36; t=2.508; P=0.01), CD8+T cells (348.69±231.47 vs. 472.35±173.49; t=2.666; P=0.01), neutrophils (2.81±1.03 vs. 3.45±1.16; t=2.728; P=0.008), lymphocytes (0.99±0.41 vs. 1.28±0.53; t=2.907; P=0.005), NK cells (20.14±3.47 vs. 22.05±3.15; t=2.614; P=0.01), and TIGIT (33.22±6.43 vs. 36.64±6.22; t=2.480; P=0.01) were significantly lower in the unfavorable prognosis group than in favorable prognosis group; meanwhile, the unfavorable prognosis group had significantly higher levels of B cells (4.96±1.16 vs. 4.35±1.03; t=2.533; P=0.01) and CTLA4 (337.38±23.35 vs. 318.36±23.03; t=3.768; P<0.001). This pattern aligned with the tendencies identified in our model, indicating the significant impact of immune cells on prognosis.
Table 2
Parameter | Favorable prognosis (n=63) | Unfavorable prognosis (n=32) | t | P |
---|---|---|---|---|
CD3+T cell (cells/μL) | 948.73±285.34 | 796.28±292.16 | 2.423 | 0.01 |
CD4+T cell (cells/μL) | 433.26±124.36 | 367.38±119.25 | 2.508 | 0.01 |
CD8+T cell (cells/μL) | 472.35±173.49 | 348.69±231.47 | 2.666 | 0.01 |
B cell (×109/L) | 4.35±1.03 | 4.96±1.16 | 2.533 | 0.01 |
Neutrophil (×109/L) | 3.45±1.16 | 2.81±1.03 | 2.728 | 0.008 |
Lymphocyte (×109/L) | 1.28±0.53 | 0.99±0.41 | 2.907 | 0.005 |
NK cell (%) | 22.05±3.15 | 20.14±3.47 | 2.614 | 0.01 |
CTLA4 (pg/mL) | 318.36±23.03 | 337.38±23.35 | 3.768 | <0.001 |
TIGIT (%) | 36.64±6.22 | 33.22±6.43 | 2.480 | 0.01 |
Data are presented as mean ± standard deviation. NK, natural killer; TIGIT, T-cell immune receptor with Ig and ITIM domains. ITIM, immunoreceptor tyrosine-based inhibitory motif.
OS
The OS prediction of patient prognosis through model construction demonstrated high predictive performance. We analyzed the OS status of the two patient groups. The results revealed that the 6-year OS of the favorable prognosis group was significantly higher than that of the unfavorable prognosis group (92.06% vs. 65.62%; P=0.005), emphasizing the potential relationship between OS and prognosis status (Table 3).
Table 3
Parameter | Favorable prognosis (n=63) | Unfavorable prognosis (n=32) | χ2 | P |
---|---|---|---|---|
2-year OS | 2 (3.17) | 4 (12.50) | None | 0.005 |
4-year OS | 3 (4.76) | 7 (21.88) | ||
6-year OS | 58 (92.06) | 21 (65.62) |
Data are presented as n (%). OS, overall survival.
Correlation analysis
The correlation analysis revealed that worsening prognosis was positively correlated with age, tumor size, B cell level, and CTLA4 level and was negatively correlated with tumor stage (T1/T2), lymph node metastasis (N0), clinical stage (I/II), CD3+T cells, CD4+T cells, CD8+T cells, neutrophils, lymphocytes, NK cells, TIGIT, and OS (Table 4). As patient prognosis deteriorated, the staging increased, indicating a significant association between prognosis and tumor characteristics and immune response.
Table 4
Parameter | r | P |
---|---|---|
Age (years) | 0.218 | 0.03 |
Tumor size (cm) | 0.237 | 0.02 |
Tumor staging (T) | −0.231 | 0.02 |
Lymph node involvement | −0.233 | 0.02 |
Clinical staging | −0.323 | 0.001 |
CD3+T cell (cells/μL) | −0.245 | 0.01 |
CD4+T cell (cells/μL) | −0.248 | 0.01 |
CD8+T cell (cells/μL) | −0.290 | 0.004 |
B cell (×109/L) | 0.264 | 0.01 |
Neutrophil (×109/L) | −0.263 | 0.01 |
Lymphocyte (×109/L) | −0.266 | 0.009 |
NK cell (%) | −0.270 | 0.008 |
CTLA4 (pg/mL) | 0.365 | <0.001 |
TIGIT (%) | −0.252 | 0.010 |
OS | −0.306 | 0.003 |
NK, natural killer; CTLA4, cytotoxic T lymphocyte-associated protein 4; TIGIT, T-cell immune receptor with Ig and ITIM domains.ITIM, immunoreceptor tyrosine-based inhibitory motif
Logistic regression analysis
The results of the logistic regression analysis identified tumor size, B cell level, age, CTLA4, tumor staging (T), lymph node metastasis, and clinical staging independent as risk factors associated with worsening prognosis, while CD3+T cells, CD4+T cells, CD8+T cells, neutrophils, lymphocytes, NK cells, and TIGIT were identified as protective factors against worsening prognosis (Table 5).
Table 5
Parameter | Odds ratio | 95% CI | Beta | P |
---|---|---|---|---|
Age (years) | 1.162 | 1.013–1.348 | 0.150 | 0.03 |
Tumor size (cm) | 1.930 | 1.111–3.569 | 0.658 | 0.02 |
Tumor staging (T) | 0.369 | 0.15–0.877 | −0.996 | 0.02 |
Lymph node involvement | 0.367 | 0.15–0.872 | −1.002 | 0.02 |
Clinical staging | 0.244 | 0.096–0.589 | −1.412 | 0.002 |
CD3+T cell (cells/μL) | 0.998 | 0.996–1.000 | −0.002 | 0.02 |
CD4+T cell (cells/μL) | 0.996 | 0.992–0.999 | −0.004 | 0.01 |
CD8+T cell (cells/μL) | 0.997 | 0.994–0.999 | −0.003 | 0.006 |
B cell (×109/L) | 1.705 | 1.137–2.655 | 0.534 | 0.01 |
Neutrophil (×109/L) | 0.592 | 0.381–0.881 | −0.524 | 0.01 |
Lymphocyte (×109/L) | 0.296 | 0.108–0.735 | −1.216 | 0.01 |
NK cell (%) | 0.837 | 0.723–0.956 | −0.178 | 0.01 |
CTLA4 (pg/mL) | 1.038 | 1.017–1.063 | 0.037 | <0.001 |
TIGIT (%) | 0.915 | 0.846–0.981 | −0.089 | 0.01 |
CI, confidence interval; NK, natural killer; TIGIT, T-cell immune receptor with Ig and ITIM domains; OS, overall survival. ITIM, immunoreceptor tyrosine-based inhibitory motif.
ROC analysis
By combining clinical parameters, we developed a composite model to predict the probability of worsening prognosis in patients with BRCA. The AUC value of the combined model was 0.898 (Figure 5), demonstrating a significantly high predictive value of the relevant clinical features in identifying the occurrence of deteriorating prognosis in patients with BRCA.
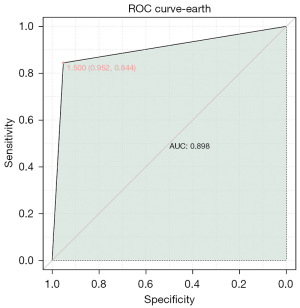
Discussion
Development of a predictive model for patients with BRCA
This study aimed to enhance the current landscape of prognostic modeling by incorporating immune cell signatures within the context of BRCA. The utility of immune cell signatures has been extensively investigated in previous studies (35,36); however, our work introduces several innovative aspects that represent a significant advancement. Notably, we integrated a multimodal approach to delineate the intricate interplay between immune cell dynamics and tumor behavior within the microenvironment. By capturing the heterogeneity and functional states of tumor-infiltrating immune cells, our objective was to provide a more sophisticated and dynamic prognostic framework, potentially revealing previously unrecognized immune subtypes and their prognostic implications. Furthermore, the incorporation of machine learning algorithms and network-based analyses enabled the identification of key immune modulatory pathways and predictive biomarkers, thus enriching the prognostic armamentarium for personalized therapeutic interventions.
The significant impact of TILs on the prognosis and response to chemotherapy and immunotherapy in the BRCA population has been extensively demonstrated through numerous investigations (37-39). In a recent study (40), researchers employed the CIBERSORT algorithm to estimate the proportions of 22 immune cell types in nearly 11,000 individuals with BRCA and discovered a significant association between immune suppressor cells and unfavorable prognosis, while T follicular helper cells exhibited a correlation with chemotherapy response in estrogen receptor-negative patients with BRCA. Similarly, another study (41) evaluated the association between TILs and response and survival in 3,771 patients with BRCA who received neoadjuvant therapy. The results indicated elevated levels of TILs were linked to a favorable outcome in human epidermal growth factor receptor 2-positive or triple-negative BRCA. Consequently, the quantification of TILs may provide innovative options for personalized treatment and enhanced prognostication for individuals with BRCA.
Our study focused on assessing the comparative quantitative levels of 1,971 immune cell signatures in an extensive BRCA sample. Furthermore, we overlapped DEGs, prognostic-related genes, and T cell pharmacodynamics-related genes to obtain 54 T-cell core pharmacodynamics-related genes. To construct a clinical prognostic model, the dimensionality of 54 prognostic genes was reduced by employing the LASSO Cox regression model. By applying the LASSO model, we identified 26 genes and established a prognostic model based on OS information through conducting Cox analysis. The reliability of the model was assessed via GEO datasets (GSE58812, GSE20711, GSE20685), and thorough analysis confirmed that the model has good predictive value for OS.
Clinical validation of the prediction model
By screening the database of patient genes and constructing a risk factor model, we identified several factors influencing patient prognosis and clinically validated them. The results revealed that age, tumor size, tumor staging (T), lymph node metastasis, clinical staging, CD3+T cells, CD4+T cells, CD8+T cells, B cells, neutrophils, lymphocytes, NK cells, TIGIT, and OS were all factors associated with a poor prognosis in patients with BRCA. These factors overlapped with the risk factors identified through genetic screening, highlighting the significant association between tumor characteristics, immune response, and patient prognosis. Additionally, we developed a combined model of clinical parameters that confirmed the diagnostic value of these risk factors for worsening prognosis in patients with BRCA.
The immune system plays a crucial role in the initiation, progression, and control of tumors. Levels of lymphocytes in the TME are correlated with the response to chemotherapy and prognosis. Immune checkpoint inhibitors, such as CTLA4 and TIGIT, work by blocking immune checkpoints on tumor cells, reducing the transmission of immune-suppressive signals and enhancing the cytotoxicity and proliferation capacity of lymphocytes. BRCA has historically been considered to have poor immunogenicity, which accounts for the slow progress in immunotherapy research in this field. As immunotherapy continues to be advanced in other types of cancer, the research on its application in BRCA is intensifying.
By combining genetic data with clinical characteristics, we developed a predictive model that integrates tumor biology outside the clinical realm, providing more refined information and target support for drug selection in modern treatment settings. Broadly speaking, the comprehensive analysis of relevant molecular markers can help facilitate the timely assessment of malignancy, the prediction of metastasis, the determination of prognosis, and ultimately, satisfactory tumor control through the selection of appropriate treatment strategies.
Limitations
It is essential to recognize that the immune cell signature-based prognostic model, although derived from robust multiomics analyses and validated across diverse patient cohorts, may have inherent constraints that warrant consideration. First, the reliance on retrospective data and publicly available datasets introduced the potential for sampling bias and factors that could distort the results, which could hinder upon the generalizability of the model to broader clinical populations. Moreover, the prognostic model’s reliance on gene expression profiles and immune cell infiltration assessment may not fully capture the dynamic and evolving nature of the TME over the course of disease progression and in response to therapeutic interventions. The variability in sources and collection methods of these data, along with the potential inclusion of various confounding factors, may reduce the reliability and efficacy of our model. Furthermore, as with any predictive model, the clinical applicability and utility of our prognostic model in informing medical decisions and improving patient outcomes should be validated in prospective research conducted in real-world clinical settings.
Conclusions
We developed a predictive model analyzing the OS and prognosis of patients with BRCA and found that this model possesses significant predictive value. The risk factors identified through genetic screening have been clinically validated. Specifically, tumor characteristics and immunotherapy are the critical factors for the prediction of prognosis in patients with BRCA.
Acknowledgments
Funding: This study was funded by
Footnote
Reporting Checklist: The authors have completed the TRIPOD reporting checklist. Available at https://tcr.amegroups.com/article/view/10.21037/tcr-24-1829/rc
Data Sharing Statement: Available at https://tcr.amegroups.com/article/view/10.21037/tcr-24-1829/dss
Peer Review File: Available at https://tcr.amegroups.com/article/view/10.21037/tcr-24-1829/prf
Conflicts of Interest: All authors have completed the ICMJE uniform disclosure form (available at https://tcr.amegroups.com/article/view/10.21037/tcr-24-1829/coif). The authors have no conflicts of interest to declare.
Ethical Statement: The authors are accountable for all aspects of the work in ensuring that questions related to the accuracy or integrity of any part of the work are appropriately investigated and resolved. The study was conducted in accordance with the Declaration of Helsinki (as revised in 2013). This study was approved by the Ethics Committee of the Second Affiliated Hospital of Qiqihar Medical University (No. [2022] 0815-9). Informed consent was waived by the Ethics Committee of the Second Affiliated Hospital of Qiqihar Medical University for this retrospective study due to the exclusive use of deidentified patient data, which posed no potential harm or impact on patient care.
Open Access Statement: This is an Open Access article distributed in accordance with the Creative Commons Attribution-NonCommercial-NoDerivs 4.0 International License (CC BY-NC-ND 4.0), which permits the non-commercial replication and distribution of the article with the strict proviso that no changes or edits are made and the original work is properly cited (including links to both the formal publication through the relevant DOI and the license). See: https://creativecommons.org/licenses/by-nc-nd/4.0/.
References
- Zhang Y, Wang Q, Yang WK, et al. Development of an immune-related prognostic biomarker for triple-negative breast cancer. Ann Med 2022;54:1212-20. [Crossref] [PubMed]
- Debien V, De Caluwé A, Wang X, et al. Immunotherapy in breast cancer: an overview of current strategies and perspectives. NPJ Breast Cancer 2023;9:7. [Crossref] [PubMed]
- Sarhangi N, Hajjari S, Heydari SF, et al. Breast cancer in the era of precision medicine. Mol Biol Rep 2022;49:10023-37. [Crossref] [PubMed]
- Kashyap D, Pal D, Sharma R, et al. Global Increase in Breast Cancer Incidence: Risk Factors and Preventive Measures. Biomed Res Int 2022;2022:9605439. [Crossref] [PubMed]
- Nassif AB, Talib MA, Nasir Q, et al. Breast cancer detection using artificial intelligence techniques: A systematic literature review. Artif Intell Med 2022;127:102276. [Crossref] [PubMed]
- Boere I, Lok C, Poortmans P, et al. Breast cancer during pregnancy: epidemiology, phenotypes, presentation during pregnancy and therapeutic modalities. Best Pract Res Clin Obstet Gynaecol 2022;82:46-59. [Crossref] [PubMed]
- Zhu SY, Yu KD. Breast Cancer Vaccines: Disappointing or Promising? Front Immunol 2022;13:828386. [Crossref] [PubMed]
- Yu H, Liu Y, Zhang W, et al. A signature of cuproptosis-related lncRNAs predicts prognosis and provides basis for future anti-tumor drug development in breast cancer. Transl Cancer Res 2023;12:1392-410. [Crossref] [PubMed]
- Ying Y, Yang M, Chen J, et al. Identification and evaluation of a risk model predicting the prognosis of breast cancer based on characteristic signatures. Transl Cancer Res 2023;12:1441-51. [Crossref] [PubMed]
- Yuan J, Li J, Zhao Z. A model for predicting clinical prognosis based on brain metastasis-related genes in patients with breast cancer. Transl Cancer Res 2023;12:3453-70. [Crossref] [PubMed]
- Hu H, Yuan S, Fu Y, et al. Eleven inflammation-related genes risk signature model predicts prognosis of patients with breast cancer. Transl Cancer Res 2024;13:3652-67. [Crossref] [PubMed]
- Layman RM, Lin H, Gutierrez Barrera AM, et al. Clinical outcomes and Oncotype DX Breast Recurrence Score® in early-stage BRCA-associated hormone receptor-positive breast cancer. Cancer Med 2022;11:1474-83. [Crossref] [PubMed]
- Wang S, Xiong Y, Zhang Q, et al. Clinical significance and immunogenomic landscape analyses of the immune cell signature based prognostic model for patients with breast cancer. Brief Bioinform 2021;22:bbaa311. [Crossref] [PubMed]
- Zheng G, Jia L, Yang AG. Roles of HLA-G/KIR2DL4 in Breast Cancer Immune Microenvironment. Front Immunol 2022;13:791975. [Crossref] [PubMed]
- Zaakouk M, Van Bockstal M, Galant C, et al. Inter- and Intra-Observer Agreement of PD-L1 SP142 Scoring in Breast Carcinoma-A Large Multi-Institutional International Study. Cancers (Basel) 2023;15:1511. [Crossref] [PubMed]
- Nalio Ramos R, Missolo-Koussou Y, Gerber-Ferder Y, et al. Tissue-resident FOLR2(+) macrophages associate with CD8(+) T cell infiltration in human breast cancer. Cell 2022;185:1189-1207.e25. [Crossref] [PubMed]
- Wu M, Li H, Zhang C, et al. Silk-Gel Powered Adenoviral Vector Enables Robust Genome Editing of PD-L1 to Augment Immunotherapy across Multiple Tumor Models. Adv Sci (Weinh) 2023;10:e2206399. [Crossref] [PubMed]
- Seung E, Xing Z, Wu L, et al. A trispecific antibody targeting HER2 and T cells inhibits breast cancer growth via CD4 cells. Nature 2022;603:328-34. [Crossref] [PubMed]
- Nakhjavani M, Shigdar S. Natural Blockers of PD-1/PD-L1 Interaction for the Immunotherapy of Triple-Negative Breast Cancer-Brain Metastasis. Cancers (Basel) 2022;14:6258. [Crossref] [PubMed]
- Yang F, Xiao Y, Ding JH, et al. Ferroptosis heterogeneity in triple-negative breast cancer reveals an innovative immunotherapy combination strategy. Cell Metab 2023;35:84-100.e8. [Crossref] [PubMed]
- Barzaman K, Moradi-Kalbolandi S, Hosseinzadeh A, et al. Breast cancer immunotherapy: Current and novel approaches. Int Immunopharmacol 2021;98:107886. [Crossref] [PubMed]
- Franzoi MA, Romano E, Piccart M. Immunotherapy for early breast cancer: too soon, too superficial, or just right? Ann Oncol 2021;32:323-36. [Crossref] [PubMed]
- Li J, Zhang D, Liu Z, et al. The combined effect and mechanism of antiangiogenic drugs and PD-L1 inhibitor on cell apoptosis in triple negative breast cancer. Ann Transl Med 2023;11:83. [Crossref] [PubMed]
- Goff SL, Danforth DN. The Role of Immune Cells in Breast Tissue and Immunotherapy for the Treatment of Breast Cancer. Clin Breast Cancer 2021;21:e63-73. [Crossref] [PubMed]
- Dieci MV, Miglietta F, Guarneri V. Immune Infiltrates in Breast Cancer: Recent Updates and Clinical Implications. Cells 2021;10:223. [Crossref] [PubMed]
- Bilotta MT, Antignani A, Fitzgerald DJ. Managing the TME to improve the efficacy of cancer therapy. Front Immunol 2022;13:954992. [Crossref] [PubMed]
- Peng C, Xu Y, Wu J, et al. TME-Related Biomimetic Strategies Against Cancer. Int J Nanomedicine 2024;19:109-35. [Crossref] [PubMed]
- Hosseini A, Gharibi T, Marofi F, et al. CTLA-4: From mechanism to autoimmune therapy. Int Immunopharmacol 2020;80:106221. [Crossref] [PubMed]
- Pu Y, Ji Q. Tumor-Associated Macrophages Regulate PD-1/PD-L1 Immunosuppression. Front Immunol 2022;13:874589. [Crossref] [PubMed]
- van der Leun AM, Thommen DS, Schumacher TN. CD8(+) T cell states in human cancer: insights from single-cell analysis. Nat Rev Cancer 2020;20:218-32. [Crossref] [PubMed]
- Huseni MA, Wang L, Klementowicz JE, et al. CD8(+) T cell-intrinsic IL-6 signaling promotes resistance to anti-PD-L1 immunotherapy. Cell Rep Med 2023;4:100878. [Crossref] [PubMed]
- Zhang Y, Chen H, Mo H, et al. Single-cell analyses reveal key immune cell subsets associated with response to PD-L1 blockade in triple-negative breast cancer. Cancer Cell 2021;39:1578-1593.e8. [Crossref] [PubMed]
- Bacher R. Normalization for Single-Cell RNA-Seq Data Analysis. Methods Mol Biol 2019;1935:11-23. [Crossref] [PubMed]
- Bolstad BM, Irizarry RA, Astrand M, et al. A comparison of normalization methods for high density oligonucleotide array data based on variance and bias. Bioinformatics 2003;19:185-93. [Crossref] [PubMed]
- Kuraoka M, Yeh CH, Bajic G, et al. Recall of B cell memory depends on relative locations of prime and boost immunization. Sci Immunol 2022;7:eabn5311. [Crossref] [PubMed]
- Soerens AG, Künzli M, Quarnstrom CF, et al. Functional T cells are capable of supernumerary cell division and longevity. Nature 2023;614:762-6. [Crossref] [PubMed]
- Fassler DJ, Torre-Healy LA, Gupta R, et al. Spatial Characterization of Tumor-Infiltrating Lymphocytes and Breast Cancer Progression. Cancers (Basel) 2022;14:2148. [Crossref] [PubMed]
- Feng J, Li J, Huang X, et al. Nomogram to Predict Tumor-Infiltrating Lymphocytes in Breast Cancer Patients. Front Mol Biosci 2021;8:761163. [Crossref] [PubMed]
- Grandal B, Evrevin C, Laas E, et al. Impact of BRCA Mutation Status on Tumor Infiltrating Lymphocytes (TILs), Response to Treatment, and Prognosis in Breast Cancer Patients Treated with Neoadjuvant Chemotherapy. Cancers (Basel) 2020;12:3681. [Crossref] [PubMed]
- Ali HR, Chlon L, Pharoah PD, et al. Patterns of Immune Infiltration in Breast Cancer and Their Clinical Implications: A Gene-Expression-Based Retrospective Study. PLoS Med 2016;13:e1002194. [Crossref] [PubMed]
- Denkert C, von Minckwitz G, Darb-Esfahani S, et al. Tumour-infiltrating lymphocytes and prognosis in different subtypes of breast cancer: a pooled analysis of 3771 patients treated with neoadjuvant therapy. Lancet Oncol 2018;19:40-50. [Crossref] [PubMed]