Construction and validation of prognostic model for colorectal mucinous adenocarcinoma patients and identification of a new prognosis related gene FAM174B
Highlight box
Key findings
• A four-gene prognostic model was constructed and validated to predict the survival of patients with mucinous adenocarcinoma (MAC).
• FAM174B was identified as potentially correlating with mucin production in MAC.
What is known and what is new?
• MAC is a subtype of colorectal cancer (CRC) characterized by high mucin production and generally poorer prognosis compared to non-specific adenocarcinoma (AC).
• This study identified FAM174B, along with CREB3L1, SPDEF, and RAP1GAP, as key genes in a prognostic model that can predict the survival of MAC patients. FAM174B’s correlation with mucin production was highlighted, suggesting its role in MAC pathology.
What is the implication, and what should change now?
• The prognostic model and the identification of FAM174B provide valuable tools for better predicting patient outcomes and understanding MAC pathology.
• Clinicians should consider incorporating this four-gene model into practice to improve prognosis accuracy and tailor treatment strategies for MAC patients. Further research should explore the biological functions of these genes, particularly FAM174B, in MAC.
Introduction
Colorectal cancer (CRC) ranks among the most prevalent malignancies and stands as the foremost cause of cancer-related mortality worldwide (1,2). CRC comprises several pathological subtypes, of which the most common is non-specific adenocarcinoma (AC). Mucinous adenocarcinoma (MAC) is a unique and rare histology subtype of CRC, accounting for 10–15% of all CRCs (3-5). The pathologic diagnostic criterion of MAC is that the extracellular mucus components account for at least 50% of the tumor volume (6,7). Numerous studies have demonstrated that the clinical characteristics, molecular characteristics, chemotherapy/radiotherapy response, and prognosis of MAC are distinct from those of AC. Compared with AC, MAC is more common in the proximal colon, has a higher tumor stage, poorer tumor differentiation, and is more likely to have lymph node metastasis (8-12). Regarding molecular characteristics, MAC exhibits higher BRAF mutation, microsatellite instability, and CpG island methylation phenotypes, but only a few molecules can be used for MAC identification (13,14).
Most studies have found that MAC is associated with poor prognosis (15-17); however, the prognostic indicator of MAC in CRC remains rare (16,18,19). Accurately predicting the prognosis of MAC patients can help clinicians provide more effective treatment strategies and follow-up management, potentially improving patients’ prognoses. Abnormal expression of mucin-related molecules such as MUC2, MUC5B, and MUC5AC is a distinctive feature and key biomarker of MAC, but they have limited prognostic value in MAC patients (20,21). Thus, finding new biomarkers or models that can distinguish MAC and AC and predict prognosis has excellent clinical value in stratifying CRC patients and providing personalized treatment for MAC.
Therefore, in this study, we analyzed and screened out the differential genes related to the prognosis of CRC between MAC and AC, intended to explore potential prognostic indicators of MAC, as well as the biological functions of this critical biomarker. By doing so, we have constructed and validated a four-gene prognostic model to predict the survival of MAC and tentatively found that FAM174B might correlate with mucin production in MAC. We present this article in accordance with the TRIPOD reporting checklist (available at https://tcr.amegroups.com/article/view/10.21037/tcr-24-347/rc).
Methods
Data collection
We retrieved RNA sequencing data from The Cancer Genome Atlas (TCGA) database (which was downloaded on 25 December 2023, https://portal.gdc.cancer.gov/), encompassing 86 tissues of colorectal MAC and 612 tissues of non-specific AC, along with corresponding clinical information (Table 1). The eligibility criteria for samples included in the TCGA project were primarily based on the availability of tumor samples from patients diagnosed with CRC and samples were typically obtained from patients who had undergone surgical resection of their tumors as part of their clinical care. The study was conducted in accordance with the Declaration of Helsinki (as revised in 2013).
Table 1
Characteristics | Adenocarcinoma (N=543) | Mucinous adenocarcinoma (N=75) |
---|---|---|
Gender, n (%) | ||
Male | 292 (47.2) | 37 (6.0) |
Female | 251 (40.6) | 38 (6.1) |
Age (years), n (%) | ||
≥65 | 324 (52.4) | 43 (7.0) |
<65 | 219 (35.4) | 32 (5.2) |
Pathological stage, n (%) | ||
Stage I | 95 (15.9) | 10 (1.6) |
Stage II | 196 (32.7) | 30 (4.9) |
Stage III | 152 (24.6) | 26 (4.2) |
Stage IV | 81 (13.1) | 8 (1.3) |
Vital status, n (%) | ||
Alive | 489 (79.1) | 66 (10.7) |
Dead | 54 (8.7) | 9 (1.5) |
Discovery of key differentially expressed genes (DEGs) in MAC tissues
The RNA sequencing data underwent differential expression analysis using the R package limma (version 3.40.6). Significantly differentially expressed genes between MAC and AC were identified based on a P value <0.05 and |log(fold change)| ≥1.2 criteria.
Kyoto Encyclopedia of Genes and Genomes (KEGG) functional enrichment analysis
Enrichment analyses of KEGG were performed using the R software package clusterProfiler (version 3.14.3) to evaluate the outcomes (22). Statistical significance was defined as a false discovery rate (FDR) <0.01.
Weighted gene co-expression network analysis (WGCNA) and identification of hub genes
The gene expression profile from TCGA was utilized to eliminate genes with standard deviation of zero across samples. Employing the R software package (version R- 4.3.1) WGCNA, the function good samples. Genes were applied to eliminate outliers and samples (23). Subsequently, WGCNA was executed to construct a scale-free coexpression network. The significance of the gene was determined based on the mediating P value (gene significance = lgP) in the linear regression linking gene expression and clinical characteristics. Module eigengenes were defined as the primary principal component within each gene module, representing the overall gene expression within a specific module. Module membership was determined based on the association between module eigengene and a gene expression profile. Hub genes associated with MAC were identified by calculating module membership and gene significance values, using criteria of |module membership| >0.8 and |gene significance| >0.1 as the cut-off.
Construction of the prognostic model
The least absolute shrinkage and selection operator (LASSO) regression model is an innovative algorithm that addresses collinearity issues. Our investigation employed LASSO to Minimize redundancy in factors and pinpoint the most influential survival-relevant hub genes. the R software package glmnet was utilized to integrate gene expression data, overall survival (OS) status, and OS time, employing the LASSO-Cox method for regression analysis.
Evaluation of the robustness and validity of the prognostic model
The prognostic disparity between these groups was analyzed using the survfit function within the R software package (24). The risk score was determined using the following formula: risk score = (gene 1 expression level × coefficient) + (gene 2 expression level × coefficient) + ... + (gene n expression level × coefficient), and MAC patients are classified into high-risk and low-risk groups based on the magnitude of their risk scores. The high and low-risk groups were defined based on the median value of the risk scores calculated from our prognostic model. Kaplan-Meier survival curves and log-rank tests were employed to evaluate the statistical significance of the prognosis in both high- and low-risk groups.
Clinical significance of the hub genes
Patients were divided into high- (≥50%) and low-expression (<50%) groups based on hub gene expression levels. Kaplan-Meier survival curves and ROC analyses were then conducted. The relationship between hub gene expression and clinical characteristics was assessed using Wilcoxon and Kruskal-Wallis tests.
LinkedOmics analysis
Linkedomic (https://www.linkedomics.org/) was employed to conduct gene set enrichment analysis (GSEA) and evaluate the pathways and molecular mechanisms associated with FAM174B (24). The criteria for ranking included a FDR <0.05, and the analysis involved 500 simulations.
Statistical analysis
Initially, we identified the distinct genes between MAC and AC via differential analysis. Next, we utilized WGCNA to pinpoint 5 hub genes from the pool of differential genes. Subsequently, LASSO regression was applied to these 5 hub genes, culminating in a prognostic model comprising 4 genes. The prognostic model’s performance was assessed through receiver operating characteristic (ROC) curves, from which the area under the curve (AUC), sensitivity, and specificity were derived. Other analysis was conducted using SPSS 26.0 (SPSS, Inc., Chicago, IL, USA) and GraphPad Prism 8 (GraphPad, Inc., CA, USA). The distinction between the two groups was determined through either the paired two-tailed Student’s t-test or the Mann-Whitney-Wilcoxon test. Statistical significance was established at P<0.05.
Results
Discovery of dysregulated genes in MAC
The analytical flowchart of this study is depicted in Figure 1. We obtained the transcriptomic data of MAC and AC samples from the TCGA-colon adenocarcinoma (COAD) and TCGA-rectal adenocarcinoma (READ) datasets and identified DEGs between MAC and AC. Results showed that 12,282 DEGs were upregulated and 3,357 DEGs were downregulated in MAC tissues compared with the AC tissues (|fold change| ≥1.2, P<0.05). According to the expression of DEGs, MAC tissues can be obviously distinguished from AC tissues. Furthermore, these DEGs were shown in the Volcano plot (Figure 2A), and the top 50 up- and down-regulated DEGs of MAC were displayed in the heatmap (Figure 2B). Subsequently, we performed a KEGG pathway enrichment analysis on the DEGs. The most enriched pathways that these differentially expressed genes were involved in were olfactory transduction, protein digestion and absorption, and cytokine-cytokine receptor interaction (Figure 2C).
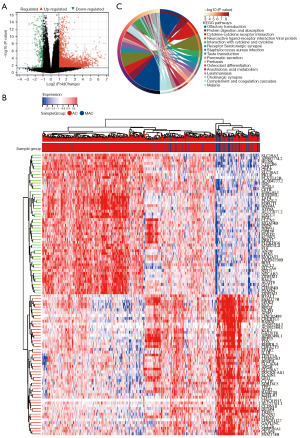
WGCNA and identification of crucial gene modules for MAC
After being initially screened, we then identified the critical gene modules related to MAC by performing WGCNA on the DEGs. Taking a soft-thresholding power of 3 (scale-free R2 =0.95), we discovered 19 gene modules (Figure 3A-3D). Comparing the correlation heatmaps of module eigengenes with MAC (Figure 3E), the cyan module had the highest positive correlation with MAC (correlation coefficient =0.4, P<0.001). In addition, the cyan module scatter plot showed a significant positive correlation between its module membership and gene significance for MAC (correlation coefficient =0.77, P<0.001, Figure 3F). Hence, we extracted hub genes within the cyan module and ultimately identified five hub genes (FAM174B, CREB3L1, SPDEF, RAP1GAP, and B3GNT6) through screening.
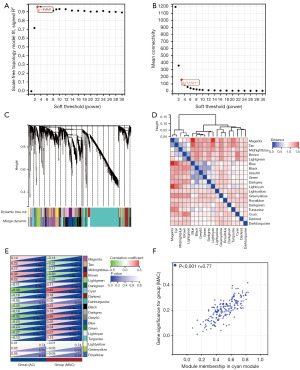
Establishment and verification of a prognostic model for patients with MAC
Then, based on the initially identified hub genes, we used LASSO regression analysis to screen genes strongly associated with the OS time and constructed a risk assessment signature model in MAC patients. The results indicated that when the lambda coefficients were 0.2218, the prognostic signature of 4 hub genes was finally identified: FAM174B, CREB3L1, SPDEF, and RAP1GAP (Figure 4A,4B). Subsequently, we divided MAC patients into low-risk and high-risk groups based on the risk scores model from the four hub genes. The survival analysis further indicated that MAC patients’ OS time significantly decreased after the risk score increased (Figure 4C). Subsequently, Kaplan-Meier survival analysis revealed that patients in the high-risk category exhibited significantly shorter OS compared to those in the low-risk category (P=0.007, Figure 4D). In addition, we assessed the performance of the four hub genes prognostic model. The AUCs for 1-, 3-, and 5-year OS were 0.61 [95% confidence interval (CI): 0.73–0.49], 0.69 (95% CI: 0.76–0.63), and 0.77 (95% CI: 0.83–0.71), respectively. Thus, the ROC curve analysis indicated that the four genes model had an excellent prognostic prediction capacity for MAC patients (Figure 4E). Moreover, these four hub genes exhibited close correlation in messenger RNA (mRNA) expression levels, suggesting potential functional interrelationships (Figure 4F).
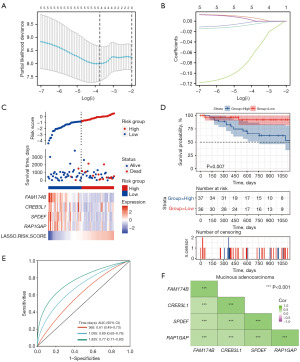
Evaluation of the clinical relevance of the key genes
After the entire model assessment, the prognostic value of the four independent hub genes was also evaluated through Kaplan-Meier curves. Results showed that only FAM174B was closely associated with OS (Figure 4D, Figure S1). According to the expression level of FAM174B, patients were divided into FAM174B low- (<50%) and high-expression groups (≥50%). The OS of the FAM174B high-expression group was better than that of the FAM174B low-expression group (P=0.02, Figure 5A). The AUC values for FAM174B predicting 1-, 3-, and 5-year OS were 0.73 (95% CI: 0.55–0.92), 0.82 (95% CI: 0.64–0.99), and 0.76 (95% CI: 0.56–0.96), respectively (Figure 5B). Subsequently, we explored the relationship between FAM174B expression levels and the clinical features of CRC patients. The findings indicated that FAM174B expression was significantly associated with MAC histology, but there was no noticeable difference in pT stages, Nodal stages, M stages, and pathological tumor, node, metastasis (pTNM) stages (Figure 5C-5G).
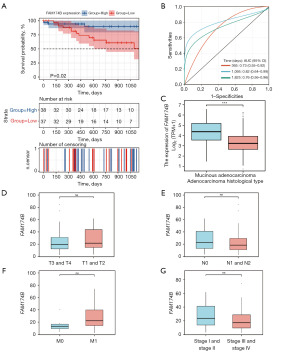
Assessment of the biological function of the FAM174B
To explore the role of FAM174B in MAC, we first performed GSEA to study the potential biological function of FAM174B in MAC. Based on the FAM174B expression level in 73 cases of MAC, cases were categorized and analyzed by FAM174B low and high expression groups. Differential analysis revealed 30 genes, each upregulated or downregulated in association with FAM174B (Figure 6A,6B). Analysis of differential gene enrichment showed that FAM174B expression was positively associated with mucin-type O-glycolipid biosynthesis and amino sugar and nucleotide sugar metabolism (Figure 6C).
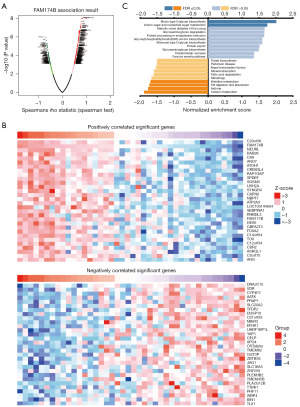
The relationship of FAM174B and mucin production
The hallmark of MAC is abundant extracellular mucin in tumor tissue and mucin-type O-glycolipid biosynthesis is critical for mucin formation. Therefore, we speculate that FAM174B is important for mucin formation in MAC. Correlation analyses were performed between expression levels of FAM174B and MAC key mucin proteins to identify this hypothesis. Results showed that FAM174B expression was positively correlated with MUC2 (P<0.001, Pearson r =0.39), MUC5AC (P=0.004, Pearson r =0.33) and MUC5B (P<0.001, Pearson r =0.43) (Figure 7A-7C). These results suggest that FAM174B may be a potential molecule associated with MAC mucin production.
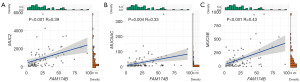
Discussion
MAC is a pathologic type of CRC that differs from AC in many aspects, such as clinical characteristics, molecular characteristics, and chemotherapy/radiotherapy response. Multiple studies have shown that patients with MAC patients exhibit a poorer prognosis relative to individuals diagnosed with AC, but the poor prognosis of MAC remains controversial (16,25,26). Therefore, there is an urgent need to find biomarkers that are related to more prognostic features and can distinguish MAC and AC to help evaluate and predict the MAC patients. By screening the differential genes of MAC and AC, we can find reliable prerequisites for potential biomarkers to identify MAC and AC.
Based on the TCGA dataset, this study identified 15,639 differentially expressed genes in MAC samples compared with AC samples, including 12,282 upregulated genes and 3,357 down-regulated genes. MAC correlation analysis of DEGs by WGCNA identified cyan modules with the highest correlation with MAC. Then, LASSO regression analysis was employed to screen the four key genes, namely FAM174B, CREB3L1, SPDEF, and RAP1GAP. Currently, the biomarkers that discriminate between MAC and AC are predominantly mucins. The hub genes we screened by analyzing the differential genes of MAC and AC all have the potential to be biomarkers for discriminating between MAC and AC.
In addition, we established a risk score model based on these four hub genes. The ROC curve results showed that the model can accurately predict the prognosis of MAC patients, with AUCs of 3- and 5-year survival times of 0.69 and 0.77, respectively. The K-M plot results showed that only FAM174B was closely associated with OS, which indicates that FAM174B has a higher correlation with MAC prognosis than other hub genes and a closer relationship with MAC. The prognostic model we constructed is expected to provide clinical decisions and suggest predictive indicators of MAC survival. Still, due to the limited number of MAC patients, more patient data are needed for verification.
FAM174B is a hub pivotal gene that we screened for prognostic relevance and promises to be an essential gene in differentiating MAC from AC. In exploring the association between FAM174B and clinical characteristics, it was found that high expression of FAM174B in the T stage was more biased towards the early stage; in the N stage, it was more biased towards the absence of lymph node metastasis; in M stage, it was more biased towards the absence of distant metastasis. Likewise, pathological staging also favored early stages. This indicated that the hub gene FAM174B we screened may play a protective role in MAC. Subsequently, we conducted GSEA analysis in MAC and classified FAM174B into a high and low expression group. KEGG enrichment results showed that the high expression of FAM174B was significantly positively correlated with Mucin type O-glycan biosynthesis, which indicates that FAM174B may be involved in the O-glycan biosynthesis. Abnormal expression of mucin is one of the most striking features of MAC.
Because O-glycan biosynthesis is related to mucin synthesis, we attempted to explore the correlation between FAM174B and mucin. FAM174B showed a positive correlation with the essential genes MUC5AC, MUC5B, and MUC2 in mucin, further suggesting that FAM174B may be related to MAC mucus production. Under normal circumstances, mucin acts as a protective barrier for epithelial cells (20,27). In addition, aberrant expression of mucin has been associated with tumor growth, invasion, metastasis, apoptosis resistance, and chemotherapy resistance (28,29). Currently, the mucoid characteristics of MAC suggest that it originates from MUC2-expressing cells. Mutations in the MUC2 gene are an essential cause of aberrant mucus production (30). Secondly, MUC2 is also associated with the inactivation of tumor suppressor genes, such as TP53 (31-33). In addition, abnormal activation of the Wnt/β-Catenin signaling pathway is a vital switch supporting MUC2 expression (13,34,35). Therefore, the association between FAM174B and MUC2 is a direction for further exploration in our research. However, there are some limitations in this study. First, the incidence of MAC is lower than that of AC, so not many data have been collected; second, in order to include a sufficient number of genes, 1.2 times the differential genes were selected, which affected the significance of the difference; third, this study was conducted only through data analysis and verification, we will choose IHC tissue for verification as the next step.
Conclusions
We have developed and validated a four-gene prognostic model to predict the survival of MAC. Additionally, we found that FAM174B might correlate with mucin production in MAC.
Acknowledgments
Funding: This work was supported by
Footnote
Reporting Checklist: The authors have completed the TRIPOD reporting checklist. Available at https://tcr.amegroups.com/article/view/10.21037/tcr-24-347/rc
Peer Review File: Available at https://tcr.amegroups.com/article/view/10.21037/tcr-24-347/prf
Conflicts of Interest: All authors have completed the ICMJE uniform disclosure form (available at https://tcr.amegroups.com/article/view/10.21037/tcr-24-347/coif). The authors have no conflicts of interest to declare.
Ethical Statement:
Open Access Statement: This is an Open Access article distributed in accordance with the Creative Commons Attribution-NonCommercial-NoDerivs 4.0 International License (CC BY-NC-ND 4.0), which permits the non-commercial replication and distribution of the article with the strict proviso that no changes or edits are made and the original work is properly cited (including links to both the formal publication through the relevant DOI and the license). See: https://creativecommons.org/licenses/by-nc-nd/4.0/.
References
- Siegel RL, Miller KD, Wagle NS, et al. Cancer statistics, 2023. CA Cancer J Clin 2023;73:17-48. [Crossref] [PubMed]
- Haggar FA, Boushey RP. Colorectal cancer epidemiology: incidence, mortality, survival, and risk factors. Clin Colon Rectal Surg 2009;22:191-7. [Crossref] [PubMed]
- Viganò L, Russolillo N, Ferrero A, et al. Resection of liver metastases from colorectal mucinous adenocarcinoma: is this a different disease? Results of a case-control study. Ann Surg 2014;260:878-84; discussion 884-5. [Crossref] [PubMed]
- Hugen N, Brown G, Glynne-Jones R, et al. Advances in the care of patients with mucinous colorectal cancer. Nat Rev Clin Oncol 2016;13:361-9. [Crossref] [PubMed]
- Kakar S, Aksoy S, Burgart LJ, et al. Mucinous carcinoma of the colon: correlation of loss of mismatch repair enzymes with clinicopathologic features and survival. Mod Pathol 2004;17:696-700. [Crossref] [PubMed]
- Nagtegaal ID, Odze RD, Klimstra D, et al. The 2019 WHO classification of tumours of the digestive system. Histopathology 2020;76:182-8. [Crossref] [PubMed]
- Hugen N, van Beek JJ, de Wilt JH, et al. Insight into mucinous colorectal carcinoma: clues from etiology. Ann Surg Oncol 2014;21:2963-70. [Crossref] [PubMed]
- Leopoldo S, Lorena B, Cinzia A, et al. Two subtypes of mucinous adenocarcinoma of the colorectum: clinicopathological and genetic features. Ann Surg Oncol 2008;15:1429-39. [Crossref] [PubMed]
- Lupinacci RM, Mello ES, Coelho FF, et al. Prognostic implication of mucinous histology in resected colorectal cancer liver metastases. Surgery 2014;155:1062-8. [Crossref] [PubMed]
- Ahnen DJ, Wade SW, Jones WF, et al. The increasing incidence of young-onset colorectal cancer: a call to action. Mayo Clin Proc 2014;89:216-24. [Crossref] [PubMed]
- Okuno M, Ikehara T, Nagayama M, et al. Mucinous colorectal carcinoma: clinical pathology and prognosis. Am Surg 1988;54:681-5. [PubMed]
- Green JB, Timmcke AE, Mitchell WT, et al. Mucinous carcinoma--just another colon cancer? Dis Colon Rectum 1993;36:49-54. [Crossref] [PubMed]
- Hugen N, Simons M, Halilović A, et al. The molecular background of mucinous carcinoma beyond MUC2. J Pathol Clin Res 2014;1:3-17. [Crossref] [PubMed]
- Inamura K, Yamauchi M, Nishihara R, et al. Prognostic significance and molecular features of signet-ring cell and mucinous components in colorectal carcinoma. Ann Surg Oncol 2015;22:1226-35. [Crossref] [PubMed]
- Catalano V, Loupakis F, Graziano F, et al. Mucinous histology predicts for poor response rate and overall survival of patients with colorectal cancer and treated with first-line oxaliplatin- and/or irinotecan-based chemotherapy. Br J Cancer 2009;100:881-7. [Crossref] [PubMed]
- Verhulst J, Ferdinande L, Demetter P, et al. Mucinous subtype as prognostic factor in colorectal cancer: a systematic review and meta-analysis. J Clin Pathol 2012;65:381-8. [Crossref] [PubMed]
- Börger ME, Gosens MJ, Jeuken JW, et al. Signet ring cell differentiation in mucinous colorectal carcinoma. J Pathol 2007;212:278-86. [Crossref] [PubMed]
- Park JS, Huh JW, Park YA, et al. Prognostic comparison between mucinous and nonmucinous adenocarcinoma in colorectal cancer. Medicine (Baltimore) 2015;94:e658. [Crossref] [PubMed]
- Hogan J, Burke JP, Samaha G, et al. Overall survival is improved in mucinous adenocarcinoma of the colon. Int J Colorectal Dis 2014;29:563-9. [Crossref] [PubMed]
- Imai Y, Yamagishi H, Fukuda K, et al. Differential mucin phenotypes and their significance in a variation of colorectal carcinoma. World J Gastroenterol 2013;19:3957-68. [Crossref] [PubMed]
- Kufe DW. Mucins in cancer: function, prognosis and therapy. Nat Rev Cancer 2009;9:874-85. [Crossref] [PubMed]
- Yu G, Wang LG, Han Y, et al. clusterProfiler: an R package for comparing biological themes among gene clusters. OMICS 2012;16:284-7. [Crossref] [PubMed]
- Langfelder P, Horvath S. WGCNA: an R package for weighted correlation network analysis. BMC Bioinformatics 2008;9:559. [Crossref] [PubMed]
- Shakur AH, Huang S, Qian X, et al. SURVFIT: Doubly sparse rule learning for survival data. J Biomed Inform 2021;117:103691. [Crossref] [PubMed]
- Mekenkamp LJ, Heesterbeek KJ, Koopman M, et al. Mucinous adenocarcinomas: poor prognosis in metastatic colorectal cancer. Eur J Cancer 2012;48:501-9. [Crossref] [PubMed]
- Ott C, Gerken M, Hirsch D, et al. Advanced Mucinous Colorectal Cancer: Epidemiology, Prognosis and Efficacy of Chemotherapeutic Treatment. Digestion 2018;98:143-52. [Crossref] [PubMed]
- Li L, Huang PL, Yu XJ, et al. Clinicopathological Significance of Mucin 2 Immuno-histochemical Expression in Colorectal Cancer: A Meta-Analysis. Chin J Cancer Res 2012;24:190-5. [Crossref] [PubMed]
- Reynolds IS, Fichtner M, McNamara DA, et al. Mucin glycoproteins block apoptosis; promote invasion, proliferation, and migration; and cause chemoresistance through diverse pathways in epithelial cancers. Cancer Metastasis Rev 2019;38:237-57. [Crossref] [PubMed]
- Luo C, Cen S, Ding G, et al. Mucinous colorectal adenocarcinoma: clinical pathology and treatment options. Cancer Commun (Lond) 2019;39:13. [Crossref] [PubMed]
- Tawiah A, Cornick S, Moreau F, et al. High MUC2 Mucin Expression and Misfolding Induce Cellular Stress, Reactive Oxygen Production, and Apoptosis in Goblet Cells. Am J Pathol 2018;188:1354-73. [Crossref] [PubMed]
- Park SY, Lee HS, Choe G, et al. Clinicopathological characteristics, microsatellite instability, and expression of mucin core proteins and p53 in colorectal mucinous adenocarcinomas in relation to location. Virchows Arch 2006;449:40-7. [Crossref] [PubMed]
- Tozawa E, Ajioka Y, Watanabe H, et al. Mucin expression, p53 overexpression, and peritumoral lymphocytic infiltration of advanced colorectal carcinoma with mucus component: is mucinous carcinoma a distinct histological entity? Pathol Res Pract 2007;203:567-74. [Crossref] [PubMed]
- Jang KT, Chae SW, Sohn JH, et al. Coexpression of MUC1 with p53 or MUC2 correlates with lymph node metastasis in colorectal carcinomas. J Korean Med Sci 2002;17:29-33. [Crossref] [PubMed]
- Gundamaraju R, Chong WC. Consequence of distinctive expression of MUC2 in colorectal cancers: How much is actually bad? Biochim Biophys Acta Rev Cancer 2021;1876:188579. [Crossref] [PubMed]
- O'Connell E, Reynolds IS, McNamara DA, et al. Resistance to Cell Death in Mucinous Colorectal Cancer-A Review. Cancers (Basel) 2021;13:1389. [Crossref] [PubMed]