Up-regulation of MSMO1 was associated with poor survival in cervical cancer
Highlight box
Key findings
• Methylsterol monooxygenase 1 (MSMO1) was increased and was associated with poor prognosis in cervical squamous cell carcinoma (CESC).
What is known and what is new?
• MSMO1 is increased and up-regulation of MSMO1 is correlated with progression of some tumor. But the expression pattern of MSMO1 in CESC is unknown.
• MSMO1 expression is increased in CESC. High MSMO1 had strong diagnostic power. Up-regulation of MSMO1 was related to poor prognosis.
What is the implication, and what should change now?
• In the screening and diagnosis of cervical cancer, detecting the expression of MSMO1 may help improve the diagnostic rate. MSMO1 is associated with poor prognosis and may serve as a prognostic marker and potential therapeutic target in the future.
Introduction
Cervical cancer is the fourth leading cancer in female and causes about 4,250 death every year around the world (1,2). The treatment of localized cervical cancer includes surgery, radiotherapy, chemotherapy, targeted therapy, immunotherapy or the combination of the above treatments (3,4). However, there are no standard treatment strategy for patients with advanced stage disease. Though immunotherapy have become the new treatment for patients with metastatic or recurrent cervical cancer, and many trials exploring new strategies for the management of metastatic and recurrent cervical cancer are ongoing (5), the prognosis of these patients is poor. The 5-year survival rate of advanced stage patients is 16.5% and the median survival time of these patients is 8–13 months (6,7). Exploring the biological mechanisms of cervical cancer and identifying new therapeutic targets are important for improving the prognosis of advanced-stage disease. Prognostic biomarkers based on biomolecules have attracted much attention in recent years (8). For example, a molecular staging strategy was evaluated in colon cancer patients. The molecular staging, including 43 genes, showed a stronger power than Duck’s staging in predicting survival of colon cancer patients (8). At present, the prognosis of advanced cervical cancer is still poor, and early diagnosis is one of the key ways to reduce its incidence rate and mortality. Screening modalities, such as cervical cytology (Pap test), human papillomavirus (HPV) test, and co-testing with HPV and cytology, has been used widely, but not all patients benefit from these screening methods. For this reason new biomarkers need to be developed to undertake timely diagnosis and effective therapies (9).
Cholesterol is an important component of cell membrane that helps to maintain the normal function of the cell member (10). The cellular cholesterol metabolism is regulated by complex molecular networks (11). In recent year, increasing evidence shows that cholesterol metabolism plays vital roles in the development of tumor (12,13). It is indicated that cholesterol can activate some important pathways and promote the development of tumors, including the estrogen-related receptor α pathway (14), the hedgehog signaling pathway (15), the canonical Wnt signaling pathway (16), the mitogen-activated protein kinase (MAPK) pathway (17), etc.
Methylsterol monooxygenase 1 (MSMO1), also named as sterol-C4-methyl oxidase (SC4MOL), catalyzes C4-methylsterols demethylation in cholesterol biosynthesis pathway (18). Increased MSMO1 expression promotes the migration, invasion and proliferation capacity of tumor cell (19). It has been revealed that MSMO1 is increased in several types of cancers and up-regulation of MSMO1 is correlated with drug resistance, tumor cells growth and apoptosis inhibitions (20-22). However, there is few research on the relation between MSMO1 and cervical cancer. Only one study showed that high MSMO1 was related to worse overall survival (OS) in cervical cancer (23). The expression pattern of MSMO1 in cervical cancer and its correlation to clinical characteristics remains unknown. We hypothesize that MSMO1 also has certain diagnostic and prognostic value in cervical cancer. Thus, in this research, we used The Cancer Genome Atlas (TCGA) database to study the role of MSMO1 in cervical cancer. We present this article in accordance with the REMARK reporting checklist (available at https://tcr.amegroups.com/article/view/10.21037/tcr-24-243/rc).
Methods
Data source and software
Cervical cancer patients and non-tumor cases from the TCGA database [TCGA-cervical squamous cell carcinoma (CESC) project] (https://portal.gdc.cancer.gov/) and Gene Expression Omnibus (GEO) database (https://www.ncbi.nlm.nih.gov/geo/) were included. The transcriptome data and corresponding clinical data of cervical cancer patients and non-tumor cases were downloaded from TCGA database and GEO database (reference number GSE30760). Transcriptome data of non-tumor cervical tissues were obtained from Genotype-Tissue Expression Project (GTEx) database (https://www.genome.gov/Funded-Programs-Projects/Genotype-Tissue-Expression-Project). Patients with incomplete survival information were excluded from the survival analysis. We used the toil algorithm to normalize the gene expression data (24). Software (R v3.6.3, https://www.r-project.org/) was used to analysis the data.
Analysis of the correlation between MSMO1 and clinical characteristics
Totally 306 cervical cancer cases and 13 non-tumor cases were included. Gene expression data of MSMO1 in cervical cancer and non-tumor cervical tissues were extracted and compared. The media MSMO1 expression value was calculated. Patients were classified into MSMO1 high and MSMO1 low groups. We used the median level of MSMO1 expression as the cut-off value. Patients were classified as MSMO1 high group if the MSMO1 expression was higher than the cut-off value. Otherwise, the patients were classified as MSMO1 low group. Correlation of MSMO1 expression with clinical characteristics was analyzed using the logistic regression analysis. Using a “pROC” package of R software, we plotted the receiver operating characteristic (ROC) curve and calculated the area under the curve (AUC) (25). Patient survivals in MSMO1 high group and MSMO1 low group were compared by Kaplan-Meier (KM) method. Cox regression analysis was used to determine the impact of MSMO1 expression as well as clinicopathological features to patient survival. Data from KM-plotter database (https://kmplot.com/analysis/), Gene Expression Profiling Interactive Analysis (GEPIA) (http://gepia2.cancer-pku.cn/#index) and GEO (https://www.ncbi.nlm.nih.gov/geo/) database were used to validate the prognostic value of MSMO1. The interaction between proteins was analyzed using the STRING software (https://www.string-db.org/). We also detected the relation between T cell infiltration and MSMO1 expression using the Xiantao software (https://www.xiantao.love). The study was conducted in accordance with the Declaration of Helsinki (as revised in 2013).
Statistical analysis
The comparison of MSMO1 expression between normal tissue and cancer sample was tested by Wilcoxon test. Correlation of MSMO1 expression to clinical features was tested using Kruskal-Wallis test, logistic regression analysis and Cox regression analysis. The comparison of survival was tested by Log-rank test. R software (v 3.6.3) was applied to perform the statistical analysis. Results were considered statistically significant when P<0.05.
Results
MSMO1 expression patterns in cervical cancer patients
MSMO1 expression information was extracted from TCGA database and 306 cervical cancer cases and 13 non-tumor cases were included. Firstly, we compared MSMO1 expression in non-tumor cervical tissue samples and cervical cancer samples. MSMO1 expression was significantly increased in cervical cancer (P<0.001) (Figure 1A). Then, we made a comparison of MSMO1 expression in various subgroup patients. MSMO1 expression level in patients with stage III–IV disease was higher than that patients with stage I–II disease (P=0.04) (Figure 1B). Patients who did not achieve complete response to primary treatments also had a higher MSMO1 level than those who had a complete response (P<0.001) (Figure 1C). MSMO1 expression level did not show any difference in patients with different Tumor Node Metastasis (TNM) stage, body weight, body mass index (BMI), histological type, menstrual state and contraceptive drug used history (Figure S1). Logistic regression analysis found that MSMO1 expression was correlated to age (Chi-squared test, P=0.01; Wilcoxon test, P=0.003) and patient response to primary therapy (P<0.001) (Table 1).
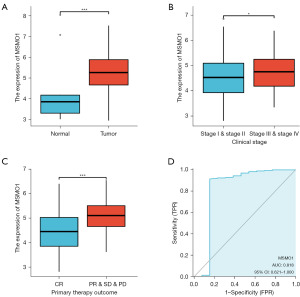
Table 1
Characteristics | Total (N) | OR (95% CI) | P value |
---|---|---|---|
Clinical stage (stage III & IV vs. stage I & II) | 299 | 1.454 (0.845–2.524) | 0.18 |
Age (>50 vs. ≤50 years) | 306 | 1.845 (1.160–2.953) | 0.01 |
Smoker (yes vs. no) | 263 | 1.532 (0.941–2.506) | 0.09 |
Histological type (squamous cell carcinoma vs. adenosquamous) | 306 | 1.147 (0.634–2.085) | 0.65 |
Primary therapy outcome (PD & SD & PR vs. CR) | 219 | 4.421 (2.000–10.847) | <0.001 |
MSMO1, methylsterol monooxygenase 1; OR, odds ratio; CI, confidence interval; PD, progressive disease; SD, stable disease; PR, partial response; CR, complete response.
The diagnostic power of MSMO1 in CESC
To analyze the diagnostic power of MSMO1, we plotted the ROC curve and calculated the AUC. The diagnostic sensitivity was 0.912 and specificity was 0.846. The AUC was 0.818 (Figure 1D) in the TCGA-CESC cohort. The above results suggested that MSMO1 expression levels were higher in cervical cancer and were correlated with advanced stage and poor response to primary therapy. MSMO1 had high diagnostic value in CESC.
Up-regulation of MSMO1 was related to poor prognosis in CESC in TCGA database
Patients were classified into MSMO1 high and MSMO1 low expression groups based on the median expression value of MSMO1. Patient characteristics are shown in Table 2. The OS, disease-specific survival (DSS) and progression-free survival (PFS) in patients with high MSMO1 and low MSMO1 was compared. High MSMO1 expression patients had a lower OS [hazard ratio (HR) =2.05, 95% confidence interval (CI): 1.25–3.37, P=0.004], DSS (HR =2.36, 95% CI: 1.32–4.23, P=0.004) and PFS (HR =2.17, 95% CI: 1.32–3.57, P=0.002) (Figure 2A-2C). Between MSMO1 high and low patients, subgroup analysis showed statistically significant differences in OS (HR =2.47, 95% CI: 1.42–4.28, P=0.001), DSS (HR =2.90, 95% CI: 1.50–5.60, P=0.002), and PFS (HR =2.36, 95% CI: 1.35–4.12, P=0.003) in the squamous cell carcinoma subgroup (Figure 2D-2F). But in adenocarcinoma subgroup, OS, DSS and PFS between the two groups did not show any difference (Figure S2). Univariate analysis indicated that advanced clinical stage (for OS, HR =2.369, 95% CI: 1.457–3.854, P<0.001; for DSS, HR =2.675, 95% CI: 1.550–4.615, P<0.001; for PFS, HR =1.847, 95% CI: 1.117–3.054, P=0.02) and high MSMO1 expression (for OS, HR =2.054, 95% CI: 1.251–3.372, P=0.004; for DSS, HR =2.362, 95% CI: 1.320–4.229, P=0.004; for PFS, HR =2.173, 95% CI: 1.324–3.567, P=0.002) were related to poor OS, DSS and PFS. Multivariate analysis found that advanced clinical stage was a risk factor to OS (HR =2.225, 95% CI: 1.365–3.627, P=0.001) and DSS (HR =2.487, 95% CI: 1.439–4.301, P=0.001). And high MSMO1 expression was an independent risk factor to OS (HR =1.902, 95% CI: 1.156–3.129, P=0.01), DSS (HR =2.172, 95% CI: 1.210–3.897, P=0.009) and PFS (HR =1.975, 95% CI: 1.189–3.282, P=0.009) (Table 3). High MSMO1 expression level was correlated to poor prognosis in CESC.
Table 2
Characteristic | Low expression of MSMO1 (n=153) | High expression of MSMO1 (n=153) | P value | Method |
---|---|---|---|---|
T stage, n (%) | 0.14 | Fisher.test | ||
T1 | 83 (34.2) | 57 (23.5) | ||
T2 | 34 (14.0) | 38 (15.6) | ||
T3 | 10 (4.1) | 11 (4.5) | ||
T4 | 3 (1.2) | 7 (2.9) | ||
N stage, n (%) | 0.62 | Chisq.test | ||
N0 | 77 (39.5) | 57 (29.2) | ||
N1 | 32 (16.4) | 29 (14.9) | ||
M stage, n (%) | 0.53 | Fisher.test | ||
M0 | 66 (52.0) | 50 (39.4) | ||
M1 | 5 (3.9) | 6 (4.7) | ||
Clinical stage, n (%) | 0.15 | Chisq.test | ||
Stage I | 89 (29.8) | 73 (24.4) | ||
Stage II | 31 (10.4) | 38 (12.7) | ||
Stage III | 22 (7.4) | 24 (8.0) | ||
Stage IV | 7 (2.3) | 15 (5.0) | ||
Primary therapy outcome, n (%) | <0.001 | Fisher.test | ||
PD | 4 (1.8) | 19 (8.7) | ||
SD | 3 (1.4) | 3 (1.4) | ||
PR | 1 (0.5) | 7 (3.2) | ||
CR | 100 (45.7) | 82 (37.4) | ||
Age, n (%) | 0.01 | Chisq.test | ||
≤50 years | 105 (34.3) | 83 (27.1) | ||
>50 years | 48 (15.7) | 70 (22.9) | ||
Histological type, n (%) | 0.76 | Chisq.test | ||
Adenosquamous | 28 (9.2) | 25 (8.2) | ||
Squamous cell carcinoma | 125 (40.8) | 128 (41.8) | ||
Smoker, n (%) | 0.11 | Chisq.test | ||
No | 77 (29.3) | 67 (25.5) | ||
Yes | 51 (19.4) | 68 (25.9) | ||
Age (years), median [IQR] | 45 [37, 54] | 48 [41, 61] | 0.003 | Wilcoxon |
MSMO1, methylsterol monooxygenase 1; PD, progressive disease; SD, stable disease; PR, partial response; CR, complete response; IQR, interquartile range.
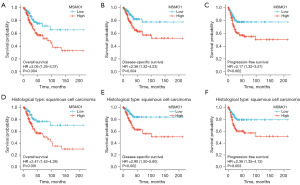
Table 3
Characteristics | Total (N) | Univariate | Multivariate | |||
---|---|---|---|---|---|---|
HR (95% CI) | P value | HR (95% CI) | P value | |||
Overall survival | ||||||
Age (>50 vs. ≤50 years) | 306 | 1.289 (0.810–2.050) | 0.28 | |||
Clinical stage (stage III & IV vs. stage I & II) | 299 | 2.369 (1.457–3.854) | <0.001 | 2.225 (1.365–3.627) | 0.001 | |
Histological type (adenosquamous vs. squamous cell carcinoma) | 306 | 0.968 (0.508–1.843) | 0.92 | |||
Smoker (yes vs. no) | 263 | 1.505 (0.924–2.451) | 0.10 | |||
MSMO1 (high vs. low) | 306 | 2.054 (1.251–3.372) | 0.004 | 1.902 (1.156–3.129) | 0.01 | |
Disease-specific survival | ||||||
Age (>50 vs. ≤50 years) | 302 | 1.295 (0.761–2.204) | 0.34 | |||
Clinical stage (stage III & IV vs. stage I & II) | 295 | 2.675 (1.550–4.615) | <0.001 | 2.487 (1.439–4.301) | 0.001 | |
Histological type (adenosquamous vs. squamous cell carcinoma) | 302 | 1.017 (0.497–2.081) | 0.96 | |||
Smoker (yes vs. no) | 259 | 1.355 (0.777–2.363) | 0.28 | |||
MSMO1 (high vs. low) | 302 | 2.362 (1.320–4.229) | 0.004 | 2.172 (1.210–3.897) | 0.009 | |
Progression-free survival | ||||||
Age (>50 vs. ≤50 years) | 306 | 1.577 (0.993–2.504) | 0.053 | 1.255 (0.773–2.037) | 0.36 | |
Clinical stage (stage III & IV vs. stage I & II) | 299 | 1.847 (1.117–3.054) | 0.02 | 1.637 (0.977–2.743) | 0.06 | |
Histological type (adenosquamous vs. squamous cell carcinoma) | 306 | 1.255 (0.699–2.251) | 0.45 | |||
Smoker (yes vs. no) | 263 | 1.025 (0.633–1.659) | 0.92 | |||
MSMO1 (high vs. low) | 306 | 2.173 (1.324–3.567) | 0.002 | 1.975 (1.189–3.282) | 0.009 |
HR, hazard ratio; CI, confidence interval; MSMO1, methylsterol monooxygenase 1.
Up-regulation of MSMO1 was related to poor prognosis in CESC in independent databases
To validate the prognostic value of MSMO1, we analyzed the correlation of MSMO1 expression to OS in KM-plotter database and GEPIA database. Totally, 304 CESC patients form KM-plotter database were included in analysis. There were152 patients assigned to the MSMO1 high group and another 152 patients into MSMO1 low group. High MSMO1 expression patients showed worse OS (HR =2.18, 95% CI: 1.32–3.60, P=0.002) (Figure 3A); 292 CESC patients from GEPIA database were included in the survival analysis; 146 cases were included into high MSMO1 group and 146 patients into low MSMO1 group. OS was compared and results showed that high MSMO1 expression patients had worse prognosis than low MSMO1 expression patients (HR =2.0, P=0.004) (Figure 3B). The results were consistent with the those of TCGA database and indicated that MSMO1 was a poor prognosis factor in CESC. GEO dataset GSE30760 was also used to further verify the MSMO1 prognostic value. In the total cervical cancer patient cohort, patient survival in MSMO1 high group and MSMO1 low group did not show significant difference (Figure 3C). We further analyzed the survival of patient in squamous cell carcinoma subgroup. There was a trend that low MSMO1 patients had better prognosis than high MSMO1 patients, though the result did not reach statistical significance (Figure 3D).
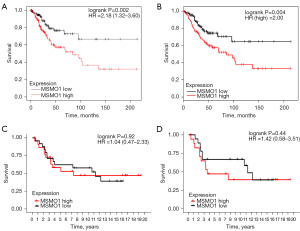
Pathways and functions enrichment analyses in cervical cancer
We used the STRING software to analyze the functional protein associated networks. Results showed that MSMO1 and CYP51A1, SQLE, SC5D, FDFT1, LSS, NSDHL, HMGCS1, C14orf1, HSD17B7, TM7SF2 showed a co-expression relationship (Figure 4A). The functional enrichments in the network showed that the steroid biosynthesis pathway (hsa00100) and metabolic pathways (hsa01100) were enriched. The active interaction genes were enriched in the three network clusters: cholesterol biosynthesis, and fatty acid hydroxylase (CL:14229), cholesterol biosynthesis (CL:14232) and CK syndrome, and tinea pedis (CL:14235) (Table 4).
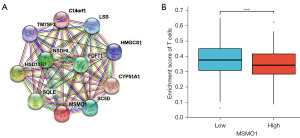
Table 4
Term ID | Term description | Matching proteins in your network (labels) | P value |
---|---|---|---|
hsa00100 | Steroid biosynthesis | CYP51A1, HSD17B7, MSMO1, SC5D, SQLE, TM7SF2, NSDHL, LSS, FDFT1 | <0.001 |
hsa01100 | Metabolic pathways | CYP51A1, HSD17B7, MSMO1, SC5D, SQLE, TM7SF2, HMGCS1, NSDHL, LSS, FDFT1 | <0.001 |
CL:14229 | Cholesterol biosynthesis, and Fatty acid hydroxylase | CYP51A1, HSD17B7, C14orf1, MSMO1, SC5D, SQLE, TM7SF2, HMGCS1, NSDHL, LSS, FDFT1 | <0.001 |
CL:14232 | Cholesterol biosynthesis | CYP51A1, HSD17B7, MSMO1, SC5D, SQLE, TM7SF2, HMGCS1, NSDHL, LSS, FDFT1 | <0.001 |
CL:14235 | CK syndrome, and tinea pedis | CYP51A1, MSMO1, SQLE, NSDHL, LSS | <0.001 |
MSMO1, methylsterol monooxygenase 1.
T cell infiltration comparison
To analyze whether MSMO1 is related to infiltrating T cells in tumor, we compared the T cell infiltration level in MSMO1 high and MSMO1 low patients. It was indicated that T cell infiltration in MSMO1 high group was lower than that in MSMO1 low group (Figure 4B).
Discussion
MSMO1 is involved in regulating the biological behavior of tumor cells. Inactivation of MSMO1 and its partner increases sensitivity of tumor cell to epidermal growth factor receptor (EGFR) inhibitors by promoting EGFR degradation (20). Another study showed that MSMO1 was associated with radioresistance in pancreatic cancer (26). In this research, we showed that MSMO1 was up-regulated in cervical cancer. Up-regulation of MSMO1 was related to unfavorable survival. The result was consistent with previous study (23). MSMO1 was an independent prognostic biomarker for cervical cancer. We further examined the prognostic values of MSMO1 to OS in KM-plotter and GEPIA database and the results were consistent. The result was validated using dataset from the GEO database. Results from GEO database also showed a trend that squamous cell cervical carcinoma patients with high MSMO1 expression had unfavorable prognosis. The results did not show statistical differences, probably because the sample size of the dataset was too small. The results should be further validated with in vitro and in vivo assays. We also investigated the potential interacting proteins using function and pathway enrichment analyses. Results showed that MSMO1 co-expressed with proteins related to cholesterol synthesis, including CYP51A1, SQLE, SC5D, FDFT1, LSS, NSDHL, HMGCS1, C14orf1, HSD17B7, and TM7SF2. Function and pathway enrichment analyses also indicated that steroid biosynthesis pathway and cholesterol biosynthesis, and fatty acid hydroxylase network were enriched in the MSMO1 high phenotype.
Previous study indicated that cholesterol or derivatives is required to T cell activation and proliferation (27). MSMO1 plays an important role in cholesterol synthesis (28). To find out whether MSMO1 was correlated with T cell infiltration, we compared the T cell infiltration level between MSMO1 high and low group and found that T cell infiltration in MSMO1 high expression group was lower.
More and more evidence showed that cholesterol metabolism took part in the development of tumors by affecting cell proliferation, invasion and migration (29-31). Increased cholesterol synthesis and uptake has been observed in many cancers. When lipids or oxygens is limited, SREBP2 and RORγ, two important transcription factors in cholesterol synthesis pathway, are upregulated (32,33). Cholesterol synthesis is also involved in the maintenance of cancer stem cells by activating the downstream pathways of Notch, sonic hedgehog and receptor tyrosine kinases (34). Some types of cancer, such as anaplastic large cell lymphoma, rely on cholesterol uptake rather than cholesterol synthesis, due to loss of rate-limiting enzyme of cholesterol synthesis (35). Another feature of cholesterol metabolism in cancer is enrichment of cholesterol derivatives, including cholesteryl esters and oxysterols. Cholesterol acyltransferases 1 (ACAT1) is the key enzyme that transforms cholesterol into cholesteryl esters (36). Inhibition of ACAT1 decreases the migration capability, impedes tumor progression and represses tumor growth (37-39). Oxysterols is another cholesterol derivative. Treatment to increase functional oxysterols promotes cell proliferation, tumor growth, tumor cell invasion and metastasis (40-42).
In this study, we showed that MSMO1 was an independent prognostic biomarker and was correlated to decreased T cell infiltration in cervical cancer. On the basis of clinical staging, combined with the expression level of MSMO1 help to better determine the prognosis of cervical cancer patients. MSMO1 may be one of the potential therapeutic targets in the future. However, it should be noted that there are some limitations in this study. Firstly, we used MSMO1 messenger ribonucleic acid (mRNA) in the current study to investigate the correlation of MSMO1 and cervical cancer. Though there is an overall positive correlation between protein and mRNA expression, it is not a perfect method to use mRNA to predict protein (43), because post transcriptional modification is not taken into consideration. Secondly, the current study was based on TCGA databased and the result should be verified clinically, and the mechanism needs further study to validation with in vitro and in vivo assays.
Conclusions
Our research showed that MSMO1 was increased and was associated with poor prognosis in cervical cancer. However, this study primarily relied on bioinformatics analysis and clinical data, without conducting biomedical experiments to investigate the underlying mechanisms of MSMO1 on cervical cancer. Therefore, future investigations incorporating functional experiments to elucidate the role of MSMO1 in cervical cancer would be highly valuable and evaluate if MSMO1 may potentially serve as a prognostic biomarker in cervical cancer.
Acknowledgments
Funding: None.
Footnote
Reporting Checklist: The authors have completed the REMARK reporting checklist. Available at https://tcr.amegroups.com/article/view/10.21037/tcr-24-243/rc
Peer Review File: Available at https://tcr.amegroups.com/article/view/10.21037/tcr-24-243/prf
Conflicts of Interest: All authors have completed the ICMJE uniform disclosure form (available at https://tcr.amegroups.com/article/view/10.21037/tcr-24-243/coif). The authors have no conflicts of interest to declare.
Ethical Statement: The authors are accountable for all aspects of the work in ensuring that questions related to the accuracy or integrity of any part of the work are appropriately investigated and resolved. The study was conducted in accordance with the Declaration of Helsinki (as revised in 2013).
Open Access Statement: This is an Open Access article distributed in accordance with the Creative Commons Attribution-NonCommercial-NoDerivs 4.0 International License (CC BY-NC-ND 4.0), which permits the non-commercial replication and distribution of the article with the strict proviso that no changes or edits are made and the original work is properly cited (including links to both the formal publication through the relevant DOI and the license). See: https://creativecommons.org/licenses/by-nc-nd/4.0/.
References
- Siegel RL, Miller KD, Jemal A. Cancer statistics, 2019. CA Cancer J Clin 2019;69:7-34. [Crossref] [PubMed]
- Chaichian S, Moazzami B, Sadoughi F, et al. Functional activities of beta-glucans in the prevention or treatment of cervical cancer. J Ovarian Res 2020;13:24. [Crossref] [PubMed]
- Li H, Wu X, Cheng X. Advances in diagnosis and treatment of metastatic cervical cancer. J Gynecol Oncol 2016;27:e43. [Crossref] [PubMed]
- Abu-Rustum NR, Yashar CM, Bean S, et al. NCCN Guidelines Insights: Cervical Cancer, Version 1.2020. J Natl Compr Canc Netw 2020;18:660-6. [Crossref] [PubMed]
- D'Oria O, Bogani G, Cuccu I, et al. Pharmacotherapy for the treatment of recurrent cervical cancer: an update of the literature. Expert Opin Pharmacother 2024;25:55-65. [Crossref] [PubMed]
- van Meir H, Kenter GG, Burggraaf J, et al. The need for improvement of the treatment of advanced and metastatic cervical cancer, the rationale for combined chemo-immunotherapy. Anticancer Agents Med Chem 2014;14:190-203. [Crossref] [PubMed]
- Ferlay J, Steliarova-Foucher E, Lortet-Tieulent J, et al. Cancer incidence and mortality patterns in Europe: estimates for 40 countries in 2012. Eur J Cancer 2013;49:1374-403. [Crossref] [PubMed]
- Eschrich S, Yang I, Bloom G, et al. Molecular staging for survival prediction of colorectal cancer patients. J Clin Oncol 2005;23:3526-35. [Crossref] [PubMed]
- Ferrari F, Giannini A. Approaches to prevention of gynecological malignancies. BMC Womens Health 2024;24:254. [Crossref] [PubMed]
- Espinosa G, López-Montero I, Monroy F, et al. Shear rheology of lipid monolayers and insights on membrane fluidity. Proc Natl Acad Sci U S A 2011;108:6008-13. [Crossref] [PubMed]
- Chang TY, Chang CC, Ohgami N, et al. Cholesterol sensing, trafficking, and esterification. Annu Rev Cell Dev Biol 2006;22:129-57. [Crossref] [PubMed]
- Xu H, Zhou S, Tang Q, et al. Cholesterol metabolism: New functions and therapeutic approaches in cancer. Biochim Biophys Acta Rev Cancer 2020;1874:188394. [Crossref] [PubMed]
- Kuzu OF, Noory MA, Robertson GP. The Role of Cholesterol in Cancer. Cancer Res 2016;76:2063-70. [Crossref] [PubMed]
- Wei W, Schwaid AG, Wang X, et al. Ligand Activation of ERRα by Cholesterol Mediates Statin and Bisphosphonate Effects. Cell Metab 2016;23:479-91. [Crossref] [PubMed]
- Xiao X, Tang JJ, Peng C, et al. Cholesterol Modification of Smoothened Is Required for Hedgehog Signaling. Mol Cell 2017;66:154-162.e10. [Crossref] [PubMed]
- Sheng R, Kim H, Lee H, et al. Cholesterol selectively activates canonical Wnt signalling over non-canonical Wnt signalling. Nat Commun 2014;5:4393. [Crossref] [PubMed]
- Wang C, Li P, Xuan J, et al. Cholesterol Enhances Colorectal Cancer Progression via ROS Elevation and MAPK Signaling Pathway Activation. Cell Physiol Biochem 2017;42:729-42. [Crossref] [PubMed]
- Kalay Yildizhan I, Gökpınar İli E, Onoufriadis A, et al. New Homozygous Missense MSMO1 Mutation in Two Siblings with SC4MOL Deficiency Presenting with Psoriasiform Dermatitis. Cytogenet Genome Res 2020;160:523-30. [Crossref] [PubMed]
- Liu M, Li J, Wang Y, et al. MAGEA6 positively regulates MSMO1 and promotes the migration and invasion of oesophageal cancer cells. Exp Ther Med 2022;23:204. [Crossref] [PubMed]
- Sukhanova A, Gorin A, Serebriiskii IG, et al. Targeting C4-demethylating genes in the cholesterol pathway sensitizes cancer cells to EGF receptor inhibitors via increased EGF receptor degradation. Cancer Discov 2013;3:96-111. [Crossref] [PubMed]
- He P, Sun L, Zhu D, et al. Knock-Down of Endogenous Bornavirus-Like Nucleoprotein 1 Inhibits Cell Growth and Induces Apoptosis in Human Oligodendroglia Cells. Int J Mol Sci 2016;17:435. [Crossref] [PubMed]
- Simigdala N, Gao Q, Pancholi S, et al. Cholesterol biosynthesis pathway as a novel mechanism of resistance to estrogen deprivation in estrogen receptor-positive breast cancer. Breast Cancer Res 2016;18:58. [Crossref] [PubMed]
- Xu HH, Wang HL, Xing TJ, et al. A Novel Prognostic Risk Model for Cervical Cancer Based on Immune Checkpoint HLA-G-Driven Differentially Expressed Genes. Front Immunol 2022;13:851622. [Crossref] [PubMed]
- Vivian J, Rao AA, Nothaft FA, et al. Toil enables reproducible, open source, big biomedical data analyses. Nat Biotechnol 2017;35:314-6. [Crossref] [PubMed]
- Robin X, Turck N, Hainard A, et al. pROC: an open-source package for R and S+ to analyze and compare ROC curves. BMC Bioinformatics 2011;12:77. [Crossref] [PubMed]
- Souchek JJ, Baine MJ, Lin C, et al. Unbiased analysis of pancreatic cancer radiation resistance reveals cholesterol biosynthesis as a novel target for radiosensitisation. Br J Cancer 2014;111:1139-49. [Crossref] [PubMed]
- Aguilar-Ballester M, Herrero-Cervera A, Vinué Á, et al. Impact of Cholesterol Metabolism in Immune Cell Function and Atherosclerosis. Nutrients 2020;12:2021. [Crossref] [PubMed]
- Ershov P, Kaluzhskiy L, Mezentsev Y, et al. Enzymes in the Cholesterol Synthesis Pathway: Interactomics in the Cancer Context. Biomedicines 2021;9:895. [Crossref] [PubMed]
- Ding X, Zhang W, Li S, et al. The role of cholesterol metabolism in cancer. Am J Cancer Res 2019;9:219-27. [PubMed]
- Wang Y, Liu C, Hu L. Cholesterol regulates cell proliferation and apoptosis of colorectal cancer by modulating miR-33a-PIM3 pathway. Biochem Biophys Res Commun 2019;511:685-92. [Crossref] [PubMed]
- Liu Z, Liu X, Liu S, et al. Cholesterol promotes the migration and invasion of renal carcinoma cells by regulating the KLF5/miR-27a/FBXW7 pathway. Biochem Biophys Res Commun 2018;502:69-75. [Crossref] [PubMed]
- Lewis CA, Brault C, Peck B, et al. SREBP maintains lipid biosynthesis and viability of cancer cells under lipid- and oxygen-deprived conditions and defines a gene signature associated with poor survival in glioblastoma multiforme. Oncogene 2015;34:5128-40. [Crossref] [PubMed]
- Cai D, Wang J, Gao B, et al. RORγ is a targetable master regulator of cholesterol biosynthesis in a cancer subtype. Nat Commun 2019;10:4621. [Crossref] [PubMed]
- Kim WY. Therapeutic targeting of lipid synthesis metabolism for selective elimination of cancer stem cells. Arch Pharm Res 2019;42:25-39. [Crossref] [PubMed]
- Garcia-Bermudez J, Baudrier L, Bayraktar EC, et al. Squalene accumulation in cholesterol auxotrophic lymphomas prevents oxidative cell death. Nature 2019;567:118-22. [Crossref] [PubMed]
- Wang YJ, Bian Y, Luo J, et al. Cholesterol and fatty acids regulate cysteine ubiquitylation of ACAT2 through competitive oxidation. Nat Cell Biol 2017;19:808-19. [Crossref] [PubMed]
- Antalis CJ, Uchida A, Buhman KK, et al. Migration of MDA-MB-231 breast cancer cells depends on the availability of exogenous lipids and cholesterol esterification. Clin Exp Metastasis 2011;28:733-41. [Crossref] [PubMed]
- Geng F, Cheng X, Wu X, et al. Inhibition of SOAT1 Suppresses Glioblastoma Growth via Blocking SREBP-1-Mediated Lipogenesis. Clin Cancer Res 2016;22:5337-48. [Crossref] [PubMed]
- Jiang Y, Sun A, Zhao Y, et al. Proteomics identifies new therapeutic targets of early-stage hepatocellular carcinoma. Nature 2019;567:257-61. [Crossref] [PubMed]
- Nelson ER, Wardell SE, Jasper JS, et al. 27-Hydroxycholesterol links hypercholesterolemia and breast cancer pathophysiology. Science 2013;342:1094-8. [Crossref] [PubMed]
- Raza S, Ohm JE, Dhasarathy A, et al. The cholesterol metabolite 27-hydroxycholesterol regulates p53 activity and increases cell proliferation via MDM2 in breast cancer cells. Mol Cell Biochem 2015;410:187-95. [Crossref] [PubMed]
- Shen Z, Zhu D, Liu J, et al. 27-Hydroxycholesterol induces invasion and migration of breast cancer cells by increasing MMP9 and generating EMT through activation of STAT-3. Environ Toxicol Pharmacol 2017;51:1-8. [Crossref] [PubMed]
- Guo Y, Xiao P, Lei S, et al. How is mRNA expression predictive for protein expression? A correlation study on human circulating monocytes. Acta Biochim Biophys Sin (Shanghai) 2008;40:426-36. [Crossref] [PubMed]