PYGL regulation of glycolysis and apoptosis in glioma cells under hypoxic conditions via HIF1α-dependent mechanisms
Highlight box
Key findings
• Functional assays revealed that glycogen phosphorylase L (PYGL) promotes glioma cell proliferation, migration, and invasion, while its knockdown reduces viability and glycolysis while increasing apoptosis.
• Hypoxia was found to induce PYGL expression through hypoxia-inducible factor 1-alpha (HIF1α), linking it to tumor adaptation under low oxygen conditions.
What is known and what is new?
• Previous research has identified various differentially expressed genes and signaling pathways linked to glioma, but the specific roles of these genes in glioma pathogenesis and their potential as therapeutic targets remain underexplored.
• This study identified PYGL as a key regulator in glioma. Differential expression analysis of glioma-related datasets revealed that PYGL is upregulated in glioma, which is associated with poor survival. Functional assays showed that PYGL promotes glioma cell proliferation, colony formation, invasion, and migration, while its knockdown increases apoptosis and reduces glycolysis. Additionally, hypoxia induces PYGL expression through a HIF1α-dependent mechanism, linking metabolic changes to tumor progression.
What is the implication, and what should change now?
• The findings establish PYGL as a critical regulator of glioma progression and glycolysis. Future research should focus on developing PYGL inhibitors or modulators and evaluating their efficacy in preclinical models of glioma.
Introduction
Glioma is a malignant primary brain tumor originating in the central nervous system, including the spinal cord and brain (1). Based on the World Health Organization (WHO) categorization, gliomas are classified from grade I to IV based on their degree of differentiation, with grade I representing the most differentiated and least malignant tumors (2). The exact etiology of gliomas remains under investigation, but genetic factors, environmental exposures, and occupational hazards are considered potential contributors (3). Gliomas represent the most frequently occurring brain tumors in adults, particularly affecting individuals over the age of 40 years (4). The progression of gliomas is often accompanied by complications such as seizures, neurological deficits, infections, and thrombosis (5). Presently available forms of treatments include radiotherapy, surgical resection, chemotherapy, and targeted therapies (6). However, the prognosis of patients with glioma remains particularly poor despite advancements in treatment, which emphasizes the need for a better understanding of glioma biology. Recent research has focused on the metabolic adaptations of glioma cells, particularly the roles of key enzymes in glycolysis, glucose transporters, signaling pathways, and transcription factors (7). These studies offer new possibilities in developing therapeutic strategies aimed at targeting the metabolic vulnerabilities of glioma cells.
Glycolysis is a metabolic process that occurs in the cytoplasm of cells under both aerobic and anaerobic conditions (8). In gliomas, tumor cells often grow rapidly, exceeding the oxygen supply provided by blood vessels, which leads to regions becoming hypoxic (9). This hypoxic environment prompts tumors to rely more heavily on glycolysis for energy production. Glycolysis rapidly generates adenosine triphosphate (ATP), which supports the fast growth and proliferation of tumor cells (10). Reuss et al. investigated the mechanisms underlying the glycolytic switch in malignant gliomas, focusing on the regulation performed by key factors such as hypoxia-inducible factor 1-alpha (HIF1α), the metabolic symbiosis model of the lactate shuttle, and the relationship between glycolysis and tumor immunity (11). Additionally, glycogen phosphorylase L (PYGL) plays a crucial role in glycogen metabolism by producing glucose 1-phosphate from glycogen, which is then transformed into glucose 6-phosphate, providing essential substrates for glycolysis (12). Research by Ji et al. demonstrated that in pancreatic ductal adenocarcinoma (PDAC), PYGL is closely connected to epithelia-mesenchymal transition (EMT) (13). PYGL facilitates tumor cell migration and invasion through hypoxia induction and glycolysis, correlating with malignant features and poor prognosis in PDAC. These findings highlight the importance of exploring the relationship between glycolysis and PYGL in gliomas to understand the metabolic adaptations of these tumors better.
Although considerable advancements have been achieved in clarifying the metabolic alterations in glioma, the specific role of glycogen metabolism and its regulatory enzymes remain inadequately explored. Glycolysis has been established as a critical pathway in glioma progression, yet the contribution of PYGL, a key enzyme in glycogen metabolism, to glioma pathogenesis is not well understood. PYGL is responsible for breaking down glycogen into glucose 1-phosphate, which feeds into the glycolytic pathway, thereby playing a pivotal role in cellular energy production. Previous studies have identified the importance of glycolysis-related genes, but a comprehensive analysis of the impact of PYGL on glioma cell behavior is lacking (14,15). This lack in knowledge highlights the need for detailed investigations into the functional role of PYGL and its regulation under hypoxic conditions, particularly through HIF1α-dependent mechanisms. Clarifying this subject will provide deeper insights into glioma metabolism and uncover potential therapeutic targets for inhibiting tumor progression.
Previous studies have highlighted the regulatory mechanisms of glycolysis in malignant gliomas, focusing on key factors such as HIF1α and their impact on tumor metabolism and progression (16,17). PYGL, an essential enzyme in glycogen metabolism, has been implicated in tumor growth and metastasis, particularly through its role in glycolysis. In this study, we attempted to clarify the function of PYGL in glioma pathology, investigating its effects on glycolysis, cell proliferation, and apoptosis. Understanding the involvement of PYGL in these processes may help identify therapeutic targets for glioma treatment.
Gliomas, as the most aggressive primary brain tumors, have a heterogeneous nature and complex metabolic alterations, making their prognosis particularly poor despite various treatment options (18). Accurate prognostic prediction is crucial for personalized treatment plans and better patient management (19). Numerous prognostic models have been developed, including clinical feature-based models and those incorporating molecular markers such as gene expression profiles from The Cancer Genome Atlas (TCGA), isocitrate dehydrogenase (IDH) mutation status, 1p/19q chromosomal deletions, and MGMT promoter methylation status (20). While these models have made progress in risk stratification, there is still a need for improvement to enhance predictive accuracy and clinical applicability (21).
PYGL, an enzyme pivotal in glycogen metabolism, has been widely studied for its role in various cancers (22). Its expression levels in glioma have been associated with tumor aggressiveness, proliferation, and glycolytic activity (23). However, the potential value of PYGL in existing prognostic models remains underexplored (24). Our study aims to enhance the prognostic prediction model for glioma by incorporating PYGL. The inclusion of PYGL may improve the accuracy of predictions for several reasons: it serves as a metabolic reprogramming biomarker closely related to tumor cell metabolism, which may influence tumor growth and response to treatment; its expression levels have been shown to be independently associated with the survival rate of glioma patients, suggesting its potential as an independent prognostic factor; PYGL may affect the sensitivity of tumors to radiotherapy and chemotherapy, making its expression level a potential predictor of treatment response; and its expression may be related to immune cell infiltration and angiogenesis in the tumor microenvironment, providing an additional dimension for prognostic assessment (25).
Therefore, we believe that incorporating PYGL into the prognostic prediction model could enhance the accuracy of predictions, aiding in a better understanding of the biological characteristics of glioma and offering more personalized treatment recommendations for patients (26). This improvement could significantly impact clinical decision-making and provide new perspectives for future research and therapeutic strategy development (27). We present this article in accordance with the TRIPOD and MDAR reporting checklists (available at https://tcr.amegroups.com/article/view/10.21037/tcr-24-1974/rc).
Methods
Overview of the research process
By examining the gene expression data of glioma samples and corresponding normal tissue samples in the TCGA and Gene Expression Omnibus (GEO) databases, we identified differentially expressed genes (DEGs) associated with gliomas using the ‘Limma’ package in R software. Common DEGs between the two datasets were subsequently identified using the Venn diagram tool, and glycolysis-related genes from the Gene Set Enrichment Analysis (GSEA) database were integrated to further dissect the interactions between these genes and the glycolytic pathway. Key genes associated with glioma prognosis were identified by Cox regression analysis, which led to the construction of nomograms predicting the probability of patient survival. Human brain microvascular endothelial cells (HBMECs) and glioma cell lines were cultured in the experimental phase, and the cells were subjected to hypoxic conditions and 2-deoxy-D-glucose (2-DG) treatment to investigate the effects of these stressors on PYGL expression and cellular metabolism. Using quantitative reverse transcription polymerase chain reaction (qRT-PCR) and Western blotting, PYGL expression levels in cells were monitored, and various cellular functions, including viability, proliferation, clonogenicity, migration, invasion, and apoptosis, were assessed. The results suggest that PYGL is a key regulator of glycolysis in gliomas, and targeting PYGL and its associated metabolic pathways may provide novel therapeutic strategies for glioma treatment (Figure S1).
Data acquisition and differential expression analysis
A total of 666 glioma samples and 5 adjacent normal samples were acquired from TCGA (https://tcga-data.nci.nih.gov/tcga/) database. The GSE67089 dataset, including 19 glioma stem cell samples and 3 normal neurosphere samples, was obtained from the GEO (https://www.ncbi.nlm.nih.gov/gds/). Differential gene expression analysis was conducted on these two datasets via the “Limma” package in R software (The R Foundation for Statistical Computing, Vienna, Austria). Genes were filtered according to the fold change (FC) criterion, with FC >2 indicating DEGs that were elevated and FC <0.5 indicating downregulated DEGs. P<0.05 was applied as the significance criterion. This study was conducted in accordance with the Declaration of Helsinki (revised 2013).
Identification of intersection genes and glycolysis-related analysis
To identify the intersecting genes between the DEGs from TCGA glioma dataset and the GSE67089 dataset, the Venn online graph tool (https://bioinformatics.psb.ugent.be/webtools/Venn/) was used for topological analysis. This tool facilitated the detection of the intersection of upregulated and downregulated DEGs across both datasets. To further clarify the relationship between DEGs and glycolysis, a set of 267 glycolysis-related genes was retrieved from the GSEA (https://www.gsea-msigdb.org/gsea/msigdb/human/genesets.jsp) database. The intersecting genes of the upregulated and downregulated DEGs were then analyzed in conjunction with the glycolysis-related gene set using the Venn online graph tool to identify key intersection genes.
Cox regression analysis, prognostic modelling and validation of the independent prognostic role of PYGL
In this study, we performed univariate and multivariate Cox regression analyses to identify key prognostic indicators for gliomas using the R package ‘survival’. Through these analyses, we extracted 95% confidence intervals (CIs) (12), hazard ratios (HRs), and P values for each variable. In order to visualise the association between these factors and survival, we drew forest plots using the ‘forestplot’ software package in R. Based on the results of the multivariate Cox regression analysis, we constructed a nomogram using the R package ‘rms’ to predict the overall survival probability of glioma patients at 1, 3 and 5 years. In addition, we generated calibration plots to assess the accuracy of the survival probabilities predicted by the nomogram and compared the predicted survival rates with the actual results.
To validate the independent prognostic role of PYGL, we first performed univariate Cox regression analyses of PYGL expression levels with other clinicopathological parameters (e.g., age, gender, tumour grade, etc.) to assess their correlation with prognosis. Subsequently, we included PYGL together with other significant clinicopathological parameters in a multivariate Cox regression model to further assess the independent prognostic value of PYGL. To ensure the stability and predictive accuracy of the model, we used the bootstrap method for internal validation. We calculated the C-index to assess the predictive performance of the model.
We used clinical samples and prognostic results from The TCGA and the GEO database. From the TCGA database, we obtained 666 glioma samples and 5 adjacent normal samples, covering different gliomas grades from I to IV. We acquired the GSE67089 dataset from the GEO database, including 19 glioma stem cell samples and 3 normal neurosphere samples. In both databases, we collected prognostic information about patients, including survival time and survival status (alive or dead).
Cell lines and culture conditions
HBMECs (cat. No. 1000) were purchased from ScienCell Research Laboratories (Carlsbad, CA, USA). Glioma cell lines, including U138 (cat. No. TCHu138), U251 (cat. No. TCHu251), U87 (cat. No. TCHu87), and T98G (cat. No. SCSP-5274), were obtained from the Cell Bank of the Chinese Academy of Sciences (Shanghai, China). Additionally, 10% fetal bovine serum (FBS) and 1% penicillin/streptomycin-supplemented Dulbecco’s Modified Eagle Medium (DMEM) were applied to cultivate all cell lines. The cells were kept at 37 ℃ with 5% CO2 in a humidified incubator.
Cell transfection
In six-well plates, 5×105 cells per well were employed to seed U251 and U87 cells, which were then cultured until they reached 70–80% confluence. According to the manufacturer’s instructions, transfection was conducted using 10 µL of Lipofectamine 2000 (Invitrogen, Thermo Fisher Scientific, Waltham, MA, USA). Cells were transfected with specific small interfering RNAs (siRNAs) targeting either a negative control siRNA (si-NC) or PYGL (si-PYGL-1, si-PYGL-2, and si-PYGL-3). Additionally, specific siRNAs targeting HIF1α (si-HIF1α-1 and si-HIF1α-2) were used. For overexpression experiments, cells were transfected with a PYGL overexpression vector, while cells transfected with negative controls were provided by an empty vector. After 48 hours of transfection, cells were cultured for further analysis.
Hypoxic treatment
To examine the impact of hypoxia on PYGL expression, U251 and U87 cells were seeded at a density of 5×105 cells per well in six-well plates and cultured until they reached 70–80% confluence. The cells were then exposed to conditions of hypoxia (1% O2, 5% CO2, and 94% N2) various time intervals of 0, 6, 12, 24, 48, and 72 hours at 37 ℃. During the hypoxic treatment, the trigas incubator (Heal Force, Shanghai, China) was used to maintain a stable low-oxygen environment. At each time point, cells were immediately harvested and placed on ice for subsequent RNA and protein extraction and analysis.
Treatment with 2-DG
To evaluate the impact of 2-DG on cell metabolism and PYGL expression, U87 and U251 cells were seeded at a density of 5×105 cells per well in six-well plates and cultured until they reached 70–80% confluence. After receiving 2 mM of 2-DG treatment, transfected cells were incubated for 24 hours. After the treatment period, cells were immediately harvested and placed on ice for subsequent RNA and protein extraction and analysis. The 2-DG treatment aimed to inhibit glycolysis, thereby allowing for the study of its effects on cellular metabolism and gene expression.
Quantitative reverse transcription-polymerase chain reaction
Total RNA from U251 and U87 cells was retrieved with TRIzol reagent (Tiangen Biotech, Beijing, China) in accordance with the manufacturer’s instructions. A PrimeScript reagent kit (Takara Bio, Kusatsu, Japan) was employed to synthesize complement DNA (cDNA). SYBR Green PCR Master Mix (Vazyme, Nanjing, China) was applied for qRT-PCR via the StepOnePlus Real-Time PCR System (WcGene Biotech, Shanghai, China). In addition, relative levels were assessed with the 2−ΔΔCt method (28) via the internal reference gene, β-actin. Table 1 includes a list of the primer sequences used in qRT-PCR.
Table 1
Target | Direction | Primer sequence (5'-3') |
---|---|---|
PYGL | Forward | TGATGTCCAGGTGAAGAGGA |
Reverse | AATGGAAGACAGCTGACGGT | |
PKM2 | Forward | ATGTCGAAGCCCCATAGTGAA |
Reverse | TGGGTGGTGAATCAATGTCCA | |
LDHA | Forward | ACGTCAGCAAGAGGGAGAAA |
Reverse | CGCTTCCAATAACACGGTTT | |
HIF1α | Forward | CCACAGGACAGTACAGGATG |
Reverse | TCAAGTCGTGCTGAATAATACC | |
β-actin | Forward | AGGATTCCTATGTGGGCGACGCGAC |
Reverse | ATAGCACAGCCTGGATAGCAA |
Western blot assay
Protease and phosphatase inhibitors were added to RIPA lysis solution (Beyotime, Shanghai, China) to facilitate the preparation of protein lysates from U251 and U87 cells. A BCA Protein Assay Kit (Beyotime) was used to ascertain the protein content. Protein was separated in equal parts via sodium dodecyl-sulfate polyacrylamide gel electrophoresis (SDS-PAGE) and then placed onto polyvinylidene fluoride (PVDF) membranes. The membranes were subsequently incubated with primary antibodies targeting PYGL (1:2,000; cat. No. 66769-1-Ig; RRID: AB_2882115), Bcl-2 (1:2000; cat. No. 12789-1-AP, RRID: AB_2227948), caspase-3 (1:1,000; cat. No. 19677-1-AP; RRID: AB_10733244), Bax (1:2,000; cat. No. 50599-2-Ig; RRID: AB_2061561), PKM2 (1:2,000, cat. No; 15822-1-AP; RRID: AB_1851537), LDHA (1:1,000; cat. No. 66287-1-Ig; RRID: AB_2881670), and HIF1α (1:2,000; cat. No. 66730-1-Ig; RRID: AB_2882080), all of which were obtained from Wuhan Sanying Biotechnology (Wuhan, China). β-actin (1:4,000; cat. No. 20536-1-APl RRID: AB_10700003) was used as the loading control for cytoplasmic proteins. After incubation with horseradish peroxidase (HRP)-conjugated secondary antibodies (Wuhan Sanying Biotechnology), the protein bands were detected with an enhanced chemiluminescence detection device (Beyotime) and captured on ImageJ software (ImageJ, US National Institutes of Health, Bethesda, MD, USA).
Cell Counting Kit 8 (CCK-8) assay
Cell viability was assessed via CCK-8 assay (cat No. CK04; Dojindo Laboratories, Mashiki, Japan). In 96-well plates, U251 and U87 cells were cultivated at a density of 5×103 cells per well. After the indicated treatments, CCK-8 reagent was planted in each well. After 12, 24, and 48 hours, a microplate reader (Thermo Fisher Scientific) was employed to measure the absorbance at 450 nm.
Colony formation
Colony formation was assessed via plate colony-formation assay. Initially, 200 cells from each group were seeded into each well of a six-well plate for 10 days. After this time of incubation, the cells were washed with phosphate-buffered saline (PBS), stained with alkaline nitro cyanate tetrazolium chloride, and fixed. Colony images were captured, and colonies were tallied under a microscope (Olympus).
Transwell assay
A cell suspension was prepared in a serum-free medium, and 500 µL of serum-containing media was introduced to the lower chamber of a 5 µm Costar Transwell system (Corning, Corning, NY, USA) in order to conduct the migration test. The top chamber received 200 µL of the cell suspension, which contained approximately 5×104 cells. After a 24-hour incubation, the chamber was removed, and the upper chamber medium was aspirated. A cotton swab was used to carefully wipe the membrane’s top surface. Cells on the bottom surface of the membrane were 4’,6-diamidino-2-phenylindole (DAPI)-stained at room temperature for 15 minutes. Following staining, the membrane was washed with PBS and dried by air, and cells were counted at 200× magnification using an inverted microscope in five randomly chosen fields. Images were captured for analysis. In the invasion assay, the process mirrored that of the migration assay, but the Transwell chamber membrane was coated with Matrigel (BD Biosciences, Franklin Lakes, NJ, USA) beforehand. All other steps remained the same as those described for the migration assay.
Flow cytometry analysis
Flow cytometry analysis was applied to evaluate cell apoptosis in glioma cells. U251 and U87 cells were initially plated at a density of 1×104 cells per well on 24-well plates for 24 hours. For apoptosis analysis, trypsin-EDTA was applied to dissociate the cells, which was followed by a PBS wash and an incubation period of 15 minutes at room temperature with 5 µL of propidium iodide (PI) and 5 µL of Annexin Vimentin-fluorescein isothiocyanate (V-FITC) solution. The staining was then assessed using a flow cytometer (Jiyuan, Guangzhou, China), and the FlowJo program (BD Biosciences) was employed to analyze the data.
Extracellular acidification rate (ECAR) analysis
The Seahorse XFp Extracellular Flux Analyzer (Agilent Technologies, Santa Clara, CA, USA) was used to conduct the ECAR measurements in accordance with manufacturer protocols. Briefly, U87 and U251 cells were placed onto Seahorse XFp cell culture microplates at a density of 1×104 cells/well for 24 hours. Subsequently, after the cells were washed in assay media supplemented with 2 mM of glutamine, they were allowed to acclimate for 2 hours at 37 ℃ in a non-CO2 incubator. Following baseline ECAR calibration, 10 mM of glucose, 1 µM of oligomycin, and 50 mM of 2-DG were subsequently injected into the detection wells at predefined intervals. A Seahorse XF96 analyzer (Agilent Technologies) was employed to measure and visualize the ECAR data.
ATP content assay
The ATP assay was performed using an ATP detection kit (Beyotime) as per the directions provided by the manufacturer. Briefly, 4×105 cells per well of U251 and U87 cells were sown in six-well plates. Following the incubation period, each well received 200 µL of cell lysate, which was then centrifuged at 12,000 ×g for 10 minutes at 4 ℃ to extract the supernatant. Following this, 10 µL of the supernatant was added for detection after the samples were incubated with 100 µL of ATP detection buffer in order to eliminate the ATP background. The Infinite M200 PRO microplate reader (Tecan, Männedorf, Switzerland) was used to record the optical density measurements, and ATP levels were determined by converting the readings based on a standard curve.
Lactate production assay
We employed commercial detection kits (Nanjing Jiancheng Bio, Nanjing, China) to evaluate lactate production. To promote cell adhesion and proliferation, U251 and U87 cells were cultivated for 24 hours at 37 ℃ after being planted in six-well plates at a density of 4×105 cells. To simulate metabolic stress conditions, cells were exposed to serum deprivation after incubation by substituting the medium with DMEM without FBS. A lactate detection kit was employed to examine the lactate levels as per manufacturer’s instructions. In brief, specific reagents were added to the culture supernatants, and the optical density was calculated using a microplate reader at 540 nm. Lactate concentrations were then determined according to the kit’s instructions.
Statistical analysis
R software was employed to conduct the statistical analyses. Each experiment was performed in triplicate to ensure precision and reliability, with each set of three repetitions representing technical replicates. The results were expressed as the mean ± standard deviation (29). The significance of the differences between several groups was evaluated via one-way analysis of variance (ANOVA). The Tukey post hoc test was applied for pairwise comparisons. Two-way ANOVA was performed for analyses containing multiple variables, and post hoc tests were adjusted via the Bonferroni correction for multiple comparisons. All statistical tests were performed using two-sided, P<0.05 was considered to indicate statistical significance. No sample was excluded from any of the analyses. In the constructed prognostic risk model, the C-index was calculated to assess the predictive accuracy of the model. The C-index is a value between 0.5 and 1, where 0.5 indicates that the predictive performance of the model is equivalent to random guessing and 1 indicates that the model has perfect predictive ability. The C-index for the prognostic risk model is 0.752 (95% CI: 0.707–0.797), which indicates that the model has good predictive accuracy (30). Typically, a C-index value greater than 0.7 is considered a clinically significant predictive model.
Results
DEGs in TCGA and GSE67089 glioma datasets
Differential expression analysis was conducted on TCGA glioma dataset and the GEO dataset GSE67089 with the “limma” R package. In the TCGA glioma dataset, a total of 1,255 upregulated and 1,867 downregulated DEGs were identified (Figure 1A). In the GSE67089 dataset, 326 upregulated and 628 downregulated DEGs were identified (Figure 1B). Further analysis revealed 170 intersecting DEGs between the upregulated and downregulated genes in both TCGA and GSE67089 datasets (Figure 1C). Notably, among these intersection genes, 4 overlapped with 267 glycolysis-related genes (Figure 1D).
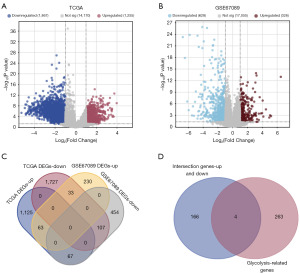
Construction of a prognostic risk model and validation of key genes
The risk model based on univariate and multivariate Cox regression analyses indicated that GUSB, PYGL, and age were significantly associated with survival rates. However, PRKACB, VLDLR, and radiotherapy did not show significant correlations with survival (Figure 2A,2B). The nomogram demonstrated high predictive accuracy for 1-year survival, with satisfactory predictions for 3- and 5-year survival (Figure 2C). The calibration plots were closely aligned with the diagonal, demonstrating excellent calibration performance (Figure 2D). Further expression analysis of GUSB and PYGL in both TCGA-glioma dataset and the GSE67089 dataset showed elevated expression levels in the tumor group (Figure 2E,2F). Given the crucial role of PYGL in glycogen metabolism, this study focused on investigating the mechanisms by which PYGL and glycolysis contribute to glioma pathogenesis.
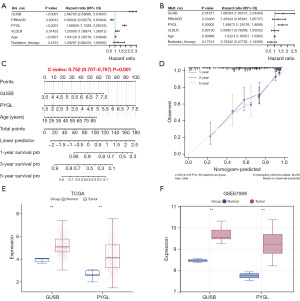
Silencing PYGL expression inhibited glioma cell proliferation
The levels of PYGL in normal cells (HBMEC) and glioma cells (U251, U138, U87, and T98G) were assessed using qRT-PCR and Western blotting. A significant upregulation of PYGL in glioma cells, particularly in U87 and U251 cells (Figure 3A-3C), was found. Subsequently, the transfection efficacy of three PYGL knockdown plasmids was examined via qRT-PCR and Western blotting in U251 and U87 cells, revealing that si-PYGL-1 and si-PYGL-2 had higher knockdown efficiencies (Figure 3D-3F). CCK-8 assay demonstrated a marked decline in the viability of U251 and U87 cells following PYGL knockdown (Figure 3G,3H).
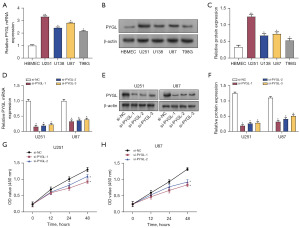
PYGL knockdown suppressed glioma cell colony formation, migration, and invasion
The impact of PYGL knockdown on colony formation in U251 and U87 cells was evaluated using a colony formation assay. Results indicated a significant reduction in colony numbers compared to those in the control groups (Figure 4A,4B). Additionally, Transwell assays demonstrated that the migration and invasion capacity of U251 and U87 cells were markedly reduced following the knockdown of PYGL (Figure 4C,4D). These findings suggest that PYGL knockdown effectively inhibits the invasive and migratory capacities of glioma cells.
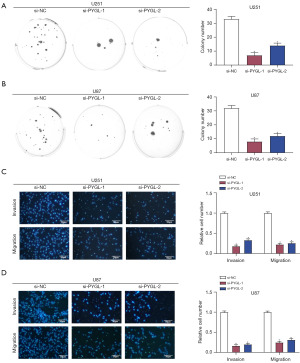
PYGL knockdown enhanced apoptosis in glioma cells through modulation of apoptotic protein expression
To investigate the impacts of PYGL knockdown on apoptosis in glioma cells, si-NC, si-PYGL-1, and si-PYGL-2 were transfected into U251 and U87 cells. Flow cytometry analysis showed a notable rise in the apoptosis rate in both U251 and U87 cells transfected with si-PYGL-1 and si-PYGL-2 in contrast to the si-NC group (Figure 5A,5B). The levels of the apoptosis-related proteins in glioma cells were investigated via Western blot analysis. PYGL knockdown led to a notable decline in Bcl-2 and a rise in Bax and caspase-3 in the glioma cell lines (Figure 5C-5E). These discoveries revealed that PYGL knockdown promotes apoptosis through the regulation of key apoptosis-related proteins.
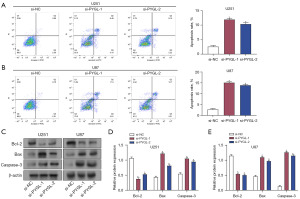
PYGL knockdown influenced the glucose metabolism and glycolysis in glioma cells
To characterize the impact of PYGL knockdown on glycolysis in glioma, glycogen levels in U87 and U251 cells were assessed via a glycogen detection kit. The results showed that PYGL knockdown increased glycogen levels in these cells, indicating reduced glucose utilization in PYGL knockdown cells (Figure 6A). Subsequent measurement of ECAR showed that ECAR was reduced in glioma cells after PYGL knockdown after the addition of glucose, oligomycin, and 2-DG, indicating reduced glycolytic activity (Figure 6B,6C). In addition, the relative ATP levels in PYGL-knockdown U251 and U87 cells were considerably lower than those in the control group, indicating impaired energy production (Figure 6D). Similarly, lactate production in PYGL-knockdown cells was significantly reduced in both cell lines, further confirming the reduction in glycolytic flux (Figure 6E). qRT-PCR and Western blot analysis demonstrated that the expression of PKM2 and LDHA were reduced after PYGL knockdown (Figure 6F-6J). These results indicate that PYGL knockdown significantly affects glucose metabolism and glycolysis in glioma cells by reducing glucose utilization, glycolytic activity, ATP production, lactate production, and the expression of the key glycolytic enzymes PKM2 and LDHA.
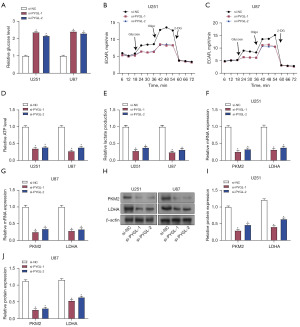
Effects of PYGL overexpression and 2-DG on glycogen levels and ECAR in glioma cells
The transfection efficacy of the PYGL overexpression plasmids in U87 and U251 cells was assessed using qRT-PCR and Western blotting (Figure 7A-7C). Glycogen levels in these cells were measured using a glycogen detection kit to determine the effects of PYGL overexpression and 2-DG. It was found that PYGL overexpression resulted in a decline in glycogen levels in U87 and U251 cells, while 2-DG increased glycogen levels. Notably, when PYGL overexpression and 2-DG were combined, 2-DG effectively reversed the glycogen-reducing effect of PYGL overexpression (Figure 7D,7E). Additionally, the impact of PYGL overexpression and 2-DG on ECAR was evaluated. Overexpression of PYGL increased the ECAR in both U251 and U87 cells, whereas 2-DG reduced the ECAR. Furthermore, 2-DG was able to inhibit the increase in the ECAR caused by PYGL overexpression (Figure 7F,7G). These findings indicate that PYGL overexpression and 2-DG exert significant effects on the glycogen metabolism and glycolytic activity of glioma cells.
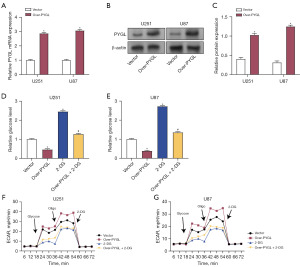
Impact of PYGL overexpression and 2-DG on ATP, lactate levels, and glycolysis-related proteins in glioma cells
PYGL overexpression and 2-DG treatment were assessed in terms of their effects on ATP and lactate levels in glioma cells. Overexpression of PYGL resulted in increased ATP and lactate levels in these cells, while 2-DG reduced both ATP and lactate levels. Interestingly, 2-DG was able to counteract the increase in ATP and lactate induced by PYGL overexpression (Figure 8A-8D). Additionally, the influence of PYGL overexpression and 2-DG on glycolysis-related enzymes was evaluated using qRT-PCR and Western blotting. Overexpression of PYGL led to enhanced levels of PKM2 and LDHA in U87 and U251 cells. In contrast, 2-DG decreased the expression of PKM2 and LDHA. When both treatments were combined, 2-DG effectively inhibited the PYGL-induced upregulation of PKM2 and LDHA (Figure 8E-8I). These results suggest that PYGL overexpression promotes glycolysis in glioma cells, and this effect can be modulated by 2-DG.
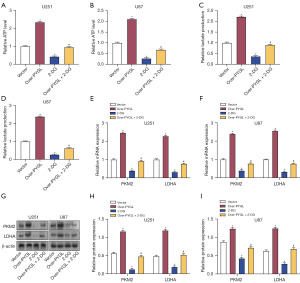
Effects of PYGL overexpression and 2-DG on cell viability and apoptosis in glioma cells
The effect of PYGL overexpression and 2-DG on cell viability in glioma was evaluated via CCK-8 assay. Overexpression of PYGL increased cell viability, whereas 2-DG decreased it. The combination of PYGL overexpression and 2-DG effectively counteracted the enhancement of cell viability induced by PYGL overexpression (Figure 9A,9B). Flow cytometry demonstrated that PYGL overexpression suppressed apoptosis in U251 and U87 cells, while 2-DG promoted apoptosis. When combined, 2-DG reversed the antiapoptotic effect of PYGL overexpression (Figure 9C,9D). Western blot assay further demonstrated that PYGL overexpression resulted in elevated Bcl-2 expression and reduced Bax and caspase-3 expression. Treatment with 2-DG significantly reduced Bcl-2 expression and increased Bax and caspase-3 expression. PYGL overexpression combined with 2-DG treatment resulted in the restoration of protein expression to levels between those of the control group and the 2-DG-alone group (Figure 9E-9G). These findings suggest that PYGL overexpression stimulates cell division and prevents apoptosis in glioma cells, whereas 2-DG treatment has the opposite effect. PYGL overexpression combined with 2-DG treatment partially reversed these changes, suggesting a complex interaction between PYGL and glycolysis inhibition in regulating glioma cell growth and survival.
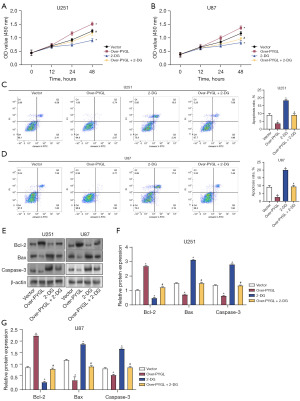
Hypoxia-induced expression of PYGL in glioma cells was mediated by HIF1α
To clarify the influence of hypoxia on PYGL expression, U251 and U87 cells were subjected to hypoxia at 0, 12, 24, 48, and 72 hours. qRT-PCR revealed that hypoxia significantly increased PYGL messenger RNA (mRNA) levels in U251 and U87 cells in a time-dependent manner (Figure 10A,10B). Western blot analysis confirmed that PYGL protein expression in glioma cell lines was also upregulated under hypoxia, especially at 12 hours (Figure 10C-10E). The effects of HIF1α knockdown on PYGL expression at 12 hours under normoxic and hypoxic conditions were further analyzed. qRT-PCR showed that HIF1α knockdown significantly reduced HIF1α mRNA levels under normoxic and hypoxic conditions in glioma cells (Figure 10F,10G). Correspondingly, PYGL mRNA levels were significantly reduced in HIF1α-knockdown cells under normoxic and hypoxic conditions compared with si-NC cells. Western blot analysis further confirmed that HIF1α knockdown notably reduced the protein expression of HIF1α and PYGL in glioma cells under normoxic and hypoxic conditions (Figure 10H-10J). These findings show that HIF1α is a key regulator of PYGL expression in glioma cells, especially under hypoxic conditions. Hypoxia-induced PYGL expression through HIF1α, and knockdown of HIF1α significantly reduced PYGL levels, emphasizing the key role of HIF1α in regulating glycolytic enzymes under hypoxic conditions.
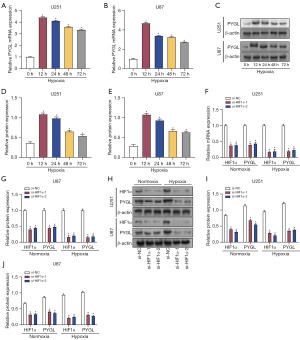
Discussion
Gliomas are among the most malignant types of central nervous system cancers, and despite improvements in treatment approaches, the prognosis for patients with high-grade gliomas, such as glioblastoma multiforme, remains poor (18). The blood-brain barrier complicates the delivery of many antitumor agents, limiting their efficacy (19). Recent studies have expanded our understanding of glioma pathogenesis and the intricate interactions within the tumor microenvironment. For instance, Gao et al. demonstrated that trametinib effectively prevents the growth, migration, and invasion of glioma cells by suppressing the PKM2/c-myc pathway, ultimately reducing glycolysis levels both in vivo and in vitro (20). Our study complements these observations by identifying four glycolysis-related genes through differential expression analysis of glioma datasets. Among them, GUSB and PYGL were identified as key prognostic genes and were significantly overexpressed in glioma tissues. Xu et al. confirmed that the level of GUSB is correlated with glioma prognosis and may influence the malignant progression of the disease (21). Similarly, Zhao et al. demonstrated that elevated expression of PYGL is linked to increased malignancy in gliomas and is an independent predictor of poor prognosis (22). This is consistent with the findings of Peng et al. who found that high expression of TXNDC11 also predicted a poor prognosis for glioma (31). Additionally, elevated levels of PYGL in tumor-associated macrophages and malignant cells underscore support its potential role in glioma progression. These findings suggest that targeting PYGL and related glycolytic pathways may offer new therapeutic approaches for glioma management.
PYGL, an enzyme responsible for glycogen breakdown and regulation of blood glucose levels, plays a critical role in modulating glycolysis and overall energy metabolism by influencing glycogenolysis rates (23). The enzyme activity directly affects the supply of glucose 6-phosphate, a key intermediate in glycolysis (24,25). Prior research has demonstrated a connection between PYGL and cancer progression; for instance, the study by Guan et al. demonstrated that PYGL encourages the metastasis, chemoresistance, and development of head-and-neck squamous cell carcinoma via the GSH/ROS/p53 pathway (26). Despite an abundance of findings for other cancer types, research into the role of PYGL in glioma remains limited. Our study identified PYGL as a hub gene in glioma and suggests its significant involvement in tumor biology. We discovered that glioma cells had an increased level of PYGL, and its knockdown notably impaired cell proliferation, colony formation, migration, and invasion. Additionally, PYGL knockdown enhanced apoptosis by decreasing Bcl-2 and increasing caspase-3 and Bax expression, underscoring its pivotal role in glioma cell behavior. Furthermore, PYGL knockdown increased intracellular glycogen levels while reducing ECAR, ATP, and lactate levels. It also decreased the levels of proteins involved in glycolysis (LDHA and PKM2), indicating that PYGL inhibition suppresses glycolysis in glioma cells. These findings emphasize the close relationship between PYGL and glycolysis in glioma.
Glycolysis, which may occur in both normoxic and hypoxic environments, is the process that turns glucose into pyruvate and is necessary for glioma cell proliferation (27). Even when oxygen is present, the primary energy source for gliomas is glycolysis, which facilitates their rapid growth (32). Lactate is produced during this process, which causes the tumor microenvironment to become more acidic and encourages invasion and metastasis (33). The rate at which metabolic byproducts acidify the medium provides a precise measure of the amount of glycolysis occurring within cells. PKM2, which catalyzes the conversion of phosphoenolpyruvate to pyruvate while making ATP, and LDHA, which transforms lactate from pyruvate and contributes to the buildup of lactate and further acidification, are important enzymes in this pathway and enhance the survival and invasion of tumor cells (34). Park et al. identified elevated PKM2 expression in gliomas and demonstrated that PKM2 inhibitors, such as shikonin and compound 3K, enhance late-stage apoptosis in U87MG glioma cells via autophagic mechanisms (35). Furthermore, Du et al. demonstrated that ANXA2P2 stimulates glycolysis and proliferation in glioma cells by competing with miR-9 for binding sites, thereby reversing the suppressive impact of miR-9 on LDHA expression (36). In our study, PYGL overexpression markedly elevated glycogen levels, ATP, and lactate in glioma cells, thereby stimulating glycolysis. The glycolysis inhibitor 2-DG effectively reversed these effects. 2-DG functions as a glucose mimic, inhibiting hexokinase, disrupting glycolysis, reducing ATP production, and increasing apoptosis. This inhibition also reduces cell viability and counteracts the metabolic advantages conferred by PYGL overexpression. These findings underscore the pivotal role of PYGL in regulating glycolysis in glioma cells and suggest that targeting PYGL in conjunction with glycolysis inhibitors such as 2-DG may represent a potentially effective treatment approach for gliomas.
Previous research has indicated that tumors reprogram glycogen metabolism under hypoxic conditions to adapt to harsh environments, with glycogen breakdown by PYGL being critical for maintaining the optimal function of the pentose phosphate pathway (37). The absence of PYGL leads to glycogen accumulation, increased reactive oxygen species levels and p53-dependent senescence, significantly suppressing tumor formation (38). Our study confirmed that PYGL expression in glioma cells is induced by hypoxia and gradually decreases as hypoxia persists. HIF1α, a transcription factor activated under hypoxia conditions, enhances glycolytic capacity by upregulating glycolysis-related genes (39). Research by Ding et al. revealed that HIF1α is notably upregulated in gliomas and is related to cell cycle regulation, DNA repair, DNA replication, and the tumor immune microenvironment (40). Additionally, Wang et al. found that in a hypoxic microenvironment, the HIF1α/HIF2α/Sox2 network promotes glioma stem cell formation through dedifferentiation, contributing to chemotherapy resistance (41). This suggests that HIF1α and HIF2α, as upstream regulators of Sox2, play crucial roles in the malignant progression of gliomas via dedifferentiation. Furthermore, the study by Yang et al. highlighted the role of complement and coagulation cascades in the prognosis and immune microenvironment of low-grade gliomas, which may be related to our finding that the expression of HIF1α and PYGL in gliomas is associated with immune cell infiltration (42). Our study further demonstrated that HIF1α depletion under both normoxic and hypoxic conditions results in a considerable reduction in PYGL expression in glioma cells. This indicates that hypoxia induces PYGL expression in a HIF1α-dependent manner. Our findings emphasize the critical function of hypoxia and HIF1α in regulating PYGL expression and underscore the importance of targeting these pathways to inhibit glioma progression. Our results support the findings of Liu et al. that mitochondria-associated gene signatures predict prognosis and immune status in gliomas, which further emphasises the important role of mitochondrial metabolism in glioma development (43).
This study integrated data from TCGA and GSE67089 datasets, providing robust identification of DEGs and demonstrating the clinical value of PYGL as prognostic markers. Experimental validation confirmed the role of PYGL in glioma cell metabolism and behavior. However, the reliance on in vitro experiments limited the direct applicability of these findings to in vivo conditions. Further validation in larger, more diverse patient cohorts is required to assess the generalizability of the results. Additionally, the exact molecular mechanisms by which PYGL influences glioma progression need further elucidation.
Research limitations
Although the present study provides valuable insights into the role of PYGL in glycolysis and apoptosis in glioma cells, some limitations need to be addressed in future studies. First, our study relied heavily on in vitro experiments. Although these experiments gave us a preliminary understanding of PYGL function and mechanism, they may not fully mimic the complex environment and interactions in vivo. Therefore, our results need to be validated in in vivo models to confirm the role of PYGL in glioma development and to assess its potential as a therapeutic target. Furthermore, although we have identified the expression and function of PYGL in glioma cells, the exact molecular mechanisms by which it affects glioma progression remain unclear. In particular, how PYGL interacts with HIF1α and other glycolysis-associated proteins and how these interactions affect the behavior of glioma cells require further studies to elucidate. Second, our study sample was mainly from populations of European origin. This sample selection may limit the generalisability of our findings, as there may be genetic and environmental differences in populations of different ethnic and geographic backgrounds that may affect PYGL expression and function. Therefore, future studies need to include more diverse populations to ensure the consistency and relevance of our findings across populations. Finally, our study focused on the effects of PYGL on glioma cell metabolism without directly assessing its impact on patient clinical outcomes. Therefore, the clinical relevance of PYGL as a potential therapeutic target needs to be further assessed in subsequent clinical studies.
Given these limitations, we suggest that future studies should include the following directions: first, animal model studies to validate the role of PYGL in glioma development and assess its potential as a therapeutic target. Second, the use of advanced molecular biology techniques, such as proteomics and genomics, to identify the proteins and genes regulated by PYGL interactions and how these interactions affect the behavior of glioma cells. Finally, the range of samples should be expanded to include populations of different ethnic and geographic backgrounds to increase the generalisability and clinical relevance of the findings, and clinical studies should be conducted to assess the potential and efficacy of PYGL as a therapeutic target. Through these future studies, we hope to not only overcome the limitations of current research, but also provide a more comprehensive understanding of the role of PYGL in gliomas and provide a scientific basis for developing new therapeutic strategies.
Conclusions
Our research underscores the pivotal function of PYGL in glioma progression, which exerts its effect various mechanisms. Bioinformatics analysis identified PYGL as a hub gene with strong predictive performance and significant expression in glioma datasets and glycolysis-related gene sets. Elevated PYGL expression correlated with increased glioma cell proliferation, migration, and invasion. PYGL knockdown suppressed these aggressive behaviors and enhanced apoptosis by modulating key apoptotic proteins. Additionally, PYGL knockdown impaired glycolysis, evidenced by increased glycogen levels and reduced ATP and lactate levels. Conversely, overexpression of PYGL promoted glycolysis and cell viability, which could be counteracted by 2-DG. Furthermore, hypoxia-induced PYGL expression was found to be HIF1α dependent, highlighting the impact of the hypoxia microenvironment on glioma metabolism and progression. These outcomes indicated that targeting PYGL could be a possible remedy strategy for glioma, emphasizing the need for further exploration into the role of PYGL in glioma metabolism and its interactions with hypoxia.
Acknowledgments
Funding: None.
Footnote
Reporting Checklist: The authors have completed the TRIPOD and MDAR reporting checklists. Available at https://tcr.amegroups.com/article/view/10.21037/tcr-24-1974/rc
Data Sharing Statement: Available at https://tcr.amegroups.com/article/view/10.21037/tcr-24-1974/dss
Peer Review File: Available at https://tcr.amegroups.com/article/view/10.21037/tcr-24-1974/prf
Conflicts of Interest: Both authors have completed the ICMJE uniform disclosure form (available at https://tcr.amegroups.com/article/view/10.21037/tcr-24-1974/coif). The authors have no conflicts of interest to declare.
Ethical Statement: The authors are accountable for all aspects of the work in ensuring that questions related to the accuracy or integrity of any part of the work are appropriately investigated and resolved. The study was conducted in accordance with the ethical principles set out in the Declaration of Helsinki (revised 2013).
Open Access Statement: This is an Open Access article distributed in accordance with the Creative Commons Attribution-NonCommercial-NoDerivs 4.0 International License (CC BY-NC-ND 4.0), which permits the non-commercial replication and distribution of the article with the strict proviso that no changes or edits are made and the original work is properly cited (including links to both the formal publication through the relevant DOI and the license). See: https://creativecommons.org/licenses/by-nc-nd/4.0/.
References
- Sobti RC, Sugimura H, Sobti A. Molecular Biomarkers for Cancer Diagnosis and Therapy. Springer; 2024.
- Komori T. Grading of adult diffuse gliomas according to the 2021 WHO Classification of Tumors of the Central Nervous System. Lab Invest 2022;102:126-33. [Crossref] [PubMed]
- Li W, Wang W. Causal effects of exposure to ambient air pollution on cancer risk: Insights from genetic evidence. Sci Total Environ 2024;912:168843. [Crossref] [PubMed]
- Pellerino A, Caccese M, Padovan M, et al. Epidemiology, risk factors, and prognostic factors of gliomas. Clin Transl Imaging 2022;10:467-75. [Crossref]
- Das AK, Makhdoomi MJ, Bhat ZH, et al. Neuro-oncology, Surgical Oncology, and Chemotherapy: Symptoms, Causes, and Therapy. Evidence‐Based Neurological Disorders: Jenny Stanford Publishing; 2024:267-310.
- Weller M, Wen PY, Chang SM, et al. Glioma. Nat Rev Dis Primers 2024;10:33. [Crossref] [PubMed]
- Shah P, Pallavali RR, Guda DR. Molecular landscape of glucose metabolism in glioblastoma and the normal human brain: A narrative review. Glioma 2024;7:10-24. [Crossref]
- Schurr A. How the 'Aerobic/Anaerobic Glycolysis' Meme Formed a 'Habit of Mind' Which Impedes Progress in the Field of Brain Energy Metabolism. Int J Mol Sci 2024;25:1433. [Crossref] [PubMed]
- Mosteiro A, Pedrosa L, Ferrés A, et al. The Vascular Microenvironment in Glioblastoma: A Comprehensive Review. Biomedicines 2022;10:1285. [Crossref] [PubMed]
- Xu S, Liao J, Liu B, et al. Aerobic glycolysis of vascular endothelial cells: a novel perspective in cancer therapy. Mol Biol Rep 2024;51:717. [Crossref] [PubMed]
- Reuss AM, Groos D, Buchfelder M, et al. The Acidic Brain-Glycolytic Switch in the Microenvironment of Malignant Glioma. Int J Mol Sci 2021;22:5518. [Crossref] [PubMed]
- Llavero F, Zugaza JL. The importance of muscle glycogen phosphorylase in glial cells function. Biochem Soc Trans 2024;52:1265-74. [Crossref] [PubMed]
- Ji Q, Li H, Cai Z, et al. PYGL-mediated glucose metabolism reprogramming promotes EMT phenotype and metastasis of pancreatic cancer. Int J Biol Sci 2023;19:1894-909. [Crossref] [PubMed]
- Yang C, Wang H, Shao M, et al. Brain-Type Glycogen Phosphorylase (PYGB) in the Pathologies of Diseases: A Systematic Review. Cells 2024;13:289. [Crossref] [PubMed]
- Cai X, Chen Z, Huang C, et al. Development of a novel glycolysis-related genes signature for isocitrate dehydrogenase 1-associated glioblastoma multiforme. Front Immunol 2022;13:950917. [Crossref] [PubMed]
- Elzakra N, Kim Y. HIF-1α Metabolic Pathways in Human Cancer. Adv Exp Med Biol 2021;1280:243-60. [Crossref] [PubMed]
- Infantino V, Santarsiero A, Convertini P, et al. Cancer Cell Metabolism in Hypoxia: Role of HIF-1 as Key Regulator and Therapeutic Target. Int J Mol Sci 2021;22:5703. [Crossref] [PubMed]
- Qu S, Qiu O, Hu Z. The prognostic factors and nomogram for patients with high-grade gliomas. Fundamental Research 2021;1:824-8. [Crossref]
- Narsinh KH, Perez E, Haddad AF, et al. Strategies to Improve Drug Delivery Across the Blood-Brain Barrier for Glioblastoma. Curr Neurol Neurosci Rep 2024;24:123-39. [Crossref] [PubMed]
- Gao M, Yang J, Gong H, et al. Trametinib Inhibits the Growth and Aerobic Glycolysis of Glioma Cells by Targeting the PKM2/c-Myc Axis. Front Pharmacol 2021;12:760055. [Crossref] [PubMed]
- Xu J, Guo Y, Ning W, et al. Comprehensive Analyses of Glucose Metabolism in Glioma Reveal the Glioma-Promoting Effect of GALM. Front Cell Dev Biol 2022;9:717182. [Crossref] [PubMed]
- Zhao CY, Hua CH, Li CH, et al. High PYGL Expression Predicts Poor Prognosis in Human Gliomas. Front Neurol 2021;12:652931. [Crossref] [PubMed]
- Migocka-Patrzałek M, Elias M. Muscle Glycogen Phosphorylase and Its Functional Partners in Health and Disease. Cells 2021;10:883. [Crossref] [PubMed]
- Zois CE, Hendriks AM, Haider S, et al. Liver glycogen phosphorylase is upregulated in glioblastoma and provides a metabolic vulnerability to high dose radiation. Cell Death Dis 2022;13:573. [Crossref] [PubMed]
- Żołnierkiewicz O, Rogacka D. Hyperglycemia - A culprit of podocyte pathology in the context of glycogen metabolism. Arch Biochem Biophys 2024;753:109927. [Crossref] [PubMed]
- Guan J, Xu X, Qiu G, et al. Cellular hierarchy framework based on single-cell/multi-patient sample sequencing reveals metabolic biomarker PYGL as a therapeutic target for HNSCC. J Exp Clin Cancer Res 2023;42:162. [Crossref] [PubMed]
- Malla A, Gupta S, Sur R. Glycolytic enzymes in non-glycolytic web: functional analysis of the key players. Cell Biochem Biophys 2024;82:351-78. [Crossref] [PubMed]
- Sindhuja S, Amuthalakshmi S, Nalini CN. A review on PCR and POC-PCR - a boon in the diagnosis of COVID-19. Curr Pharm Anal 2022;18:745-64. [Crossref]
- Puthdee N, Sriswasdi S, Pisitkun T, et al. The LIN28B/TGF-β/TGFBI feedback loop promotes cell migration and tumour initiation potential in cholangiocarcinoma. Cancer Gene Ther 2022;29:445-55. [Crossref] [PubMed]
- Zhou D, Liu X, Wang X, et al. A prognostic nomogram based on LASSO Cox regression in patients with alpha-fetoprotein-negative hepatocellular carcinoma following non-surgical therapy. BMC Cancer 2021;21:246. [Crossref] [PubMed]
- Peng P, Cheng F, Dong Y, et al. High expression of TXNDC11 indicated unfavorable prognosis of glioma. Transl Cancer Res 2021;10:5040-51. [Crossref] [PubMed]
- Garcia JH, Jain S, Aghi MK. Metabolic Drivers of Invasion in Glioblastoma. Front Cell Dev Biol 2021;9:683276. [Crossref] [PubMed]
- Gao Y, Zhou H, Liu G, et al. Tumor Microenvironment: Lactic Acid Promotes Tumor Development. J Immunol Res 2022;2022:3119375. [Crossref] [PubMed]
- Zhu S, Guo Y, Zhang X, et al. Pyruvate kinase M2 (PKM2) in cancer and cancer therapeutics. Cancer Lett 2021;503:240-8. [Crossref] [PubMed]
- Park JH, Lee JS, Oh Y, et al. PKM2 Is Overexpressed in Glioma Tissues, and Its Inhibition Highly Increases Late Apoptosis in U87MG Cells With Low-density Specificity. In Vivo 2022;36:694-703. [Crossref] [PubMed]
- Du P, Liao Y, Zhao H, et al. ANXA2P2/miR-9/LDHA axis regulates Warburg effect and affects glioblastoma proliferation and apoptosis. Cell Signal 2020;74:109718. [Crossref] [PubMed]
- Iessi E, Vona R, Cittadini C, et al. Targeting the Interplay between Cancer Metabolic Reprogramming and Cell Death Pathways as a Viable Therapeutic Path. Biomedicines 2021;9:1942. [Crossref] [PubMed]
- Khan T, Sullivan MA, Gunter JH, et al. Revisiting Glycogen in Cancer: A Conspicuous and Targetable Enabler of Malignant Transformation. Front Oncol 2020;10:592455. [Crossref] [PubMed]
- Shi Y, Lin X, Wang J, et al. Advances of HIF-1α/glycolysis axis in non-small cell lung cancer. Oncology Reports 2024;51:1-11. [Crossref]
- Ding Z, Zhang J, Li L, et al. Prognostic biomarker HIF1α and its correlation with immune infiltration in gliomas. Oncol Lett 2024;27:193. [Crossref] [PubMed]
- Wang P, Gong S, Liao B, et al. HIF1α/HIF2α induces glioma cell dedifferentiation into cancer stem cells through Sox2 under hypoxic conditions. J Cancer 2022;13:1-14. [Crossref] [PubMed]
- Yang J, Shen L, Yang J, et al. Complement and coagulation cascades are associated with prognosis and the immune microenvironment of lower-grade glioma. Transl Cancer Res 2024;13:112-36. [Crossref] [PubMed]
- Liu Y, Cai L, Wang H, et al. Novel mitochondrial-related gene signature predicts prognosis and immunological status in glioma. Transl Cancer Res 2024;13:3338-53. [Crossref] [PubMed]
(English Language Editor: J. Gray)