The role of SEC14L4 in esophageal squamous cell cancer: insights into clinical relevance and molecular pathways
Highlight box
Key findings
• High expression of Saccharomyces cerevisiae-like 4 (SEC14L4) indicates poor clinical outcomes, suggesting its potential as a therapeutic target and providing evidence for its involvement in the molecular mechanisms underlying esophageal squamous cell cancer (ESCC).
What is known and what is new?
• Esophageal cancer caused around 544,000 deaths in 2020, making it the sixth highest cause in cancer-related mortality and the seventh most prevalent cancer. Due to it mainly being diagnosed in the advanced stages, the overall 5-year survival rate for ESCC ranges from 15% to 25%, and the 5-year survival rate after surgery for patients with resectable ESCC ranges from 35% to 45%. SEC14L4 represents mammalian Golgi dynamics proteins, and SEC14L4 proteins are uniquely found in all eukaryotic genomes. Alterations in lipid metabolism and glycerophospholipids in cancer cells can affect the immune system response.
• High expression of SEC14L4 serves as a prognostic indicator of poor outcomes in patients with ESCC. It thus has potential as a therapeutic target and may be involved in the molecular mechanisms underlying ESCC.
What is the implication, and what should change now?
• Our results indicate that increased SEC14L4 expression is associated with unfavorable clinical prognosis in patients with ESCC, emphasizing its potential as a target for treatment and providing insights into its contribution to ESCC.
Introduction
The rapid increase in cancer incidence and mortality represents a significant global healthcare burden. In 2020, esophageal cancer caused around 544,000 deaths, the sixth highest cause in cancer-related mortality and the seventh most prevalent cancer (1,2). Although esophageal adenocarcinoma is the predominant histologic subtype of esophageal cancer in high-income countries, esophageal squamous cell cancer (ESCC) still represents over 90% of all esophageal cancer cases globally (1,3). Surgical resection remains a cornerstone for the treatment of early-stage ESCC, while adjuvant therapy or definitive chemoradiotherapy are often necessary for patients in the advanced stage upon diagnosis (4,5). However, treatments have demonstrated limited effectiveness and can lead to the development of drug resistance (6-9). The 5-year survival rate for individuals with ESCC is generally between 15% and 25%, primarily because many cases are diagnosed at an advanced stage. In contrast, for patients with resectable ESCC, the 5-year survival rate following surgery increases to approximately 35% to 45% (10). Therefore, it is essential to clarify the underlying mechanisms of ESCC and to develop novel and effective treatments to improve survival.
Several prognostic and therapeutic biomarkers have been reported by researchers (11,12). Using a bioinformatics approach, we identified the Saccharomyces cerevisiae-like 4 (SEC14L4) gene as an adverse prognostic indicator in ESCC patients from The Cancer Genome Atlas (TCGA) database. Compared to the numerous previous researches on esophageal cancer, the role of SEC14L4 in this disease has not yet been reported. This gene encodes a protein that belongs to the SEC14 cytosolic factor family, the members of which all contain an SEC14 domain (13,14). The SEC14 family (Saccharomyces cerevisiae-like) is one of the most well-studied lipid transport protein families. Proteins in this family possess a tripartite functional domain that binds cellular retinoids, playing a crucial role in regulating the metabolism and transport of lipids, including phosphatidylcholine, phosphatidylinositol, and phosphatidylethanolamine, primarily within the Golgi apparatus (15). Due to the complexity of its pathogenesis, esophageal cancer continues to be a focus of active research. Dysregulated lipid metabolism and metabolic reprogramming are key biological hallmarks of malignant tumors and are closely linked to the initiation and progression of esophageal cancer. These processes drive uncontrolled cancer cell proliferation, survival, invasion, and resistance to antineoplastic therapies (16). Several genes of the SEC14 superfamily have been studied in regard to their relationship with malignant tumors, specifically as it relates to genesis and development (17-19). However, the expression of the SEC14L4 gene in ESCC has not been investigated.
In this study, transcriptome and clinical data were obtained from TCGA, and analyses were performed to characterize the relationship between SEC14L4 and ESCC. More specifically, we aimed to investigate the clinical significance and potential molecular mechanisms related to SEC14L4’s involvement in ESCC using a bioinformatics approach. Furthermore, the drug resistance and sensitivity associated with SEC14L4 were examined, and the prognostic role and signaling pathways associated with SEC14L4 in ESCC were clarified, with the goal of identifying novel prognostic biomarkers and therapeutic targets for ESCC. We present this article in accordance with the TRIPOD reporting checklist (available at https://tcr.amegroups.com/article/view/10.21037/tcr-24-1657/rc).
Methods
Data acquisition and differential analysis
The database of TCGA (https://portal.gdc.cancer.gov/) is the largest repository of cancer-related genomic information, containing various types of genetic data. We obtained the primary messenger RNA (mRNA) expression and single-nucleotide polymorphism (SNP) data for ESCC. In total, 99 specimens were collected, comprising 3 samples from the normal group and 96 samples from the tumor group. Subsequently, we conducted differential expression analysis of SEC14L4. Additionally, we retrieved the data file GSE196756 from the National Center for Biotechnology Information (NCBI) Gene Expression Omnibus (GEO) public database and used the expression profiles of six patients for single-cell data analysis. The study was conducted in accordance with the Declaration of Helsinki (as revised in 2013).
Nomogram model analysis
A nomogram model was established based on gene expression and clinical symptoms. In the nomogram, line segments were drawn on the same plane, each representing a definite percentage, to reflect the relationship between each variable by the prediction model derived from regression analysis. A multivariate regression model was created, assigning a score to each value level of every contributing factor based on its impact on the outcome variable as determined by the regression coefficient size. Subsequently, these scores were summed to generate a total score, which was then used to calculate the value of prediction.
Co-expression analysis
A co-expression analysis of the SEC14L4 gene in ESCC was conducted. This analysis applied a correlation index filter of 0.3 and a significance threshold of P<0.05. Following the identification of genes exhibiting the most significant co-expression with SEC14L4, visual representations including circle diagrams and heat maps were generated using the “corrplot” and “circlize” packages in R (The R Foundation of Statistical Computing) to visualize the correlation patterns.
Gene set variation analysis (GSVA)
GSVA is a nonparametric and unsupervised approach for assessing the enrichment of gene sets in the transcriptome. GSVA translates alterations in individual genes into alterations in pathways and evaluates the biological role of the sample through a thorough evaluation of the specific genes involved. For this study, we obtained gene collections from the Molecular Signatures Database (MSigDB). The algorithm of GSVA was employed to thoroughly evaluate each gene set, with latent changes in biological function being assessed across different cases.
Gene set enrichment analysis (GSEA)
Patients were stratified into two groups (high and low expression groups) based on SEC14L4 expression levels. GSEA was employed to assess discrepancies in signaling pathways between the high and low expression categories. The gene sets used for analysis were obtained from the MSigDB version 7.0, with a focus on the annotated gene set for subtype pathways. Different pathway expression among subtypes was assessed, and gene sets that were significantly enriched (adjusted P value <0.05) were ranked according to their consistency scores.
Weighted gene correlation network analysis (WGCNA)
We conducted WGCNA to identify co-expressed gene modules. We clarified the association between the genetic network and SEC14L4, which included the identification of key genes in the network. The co-expression network of all genes was established using the “WGCNA” package in R. The program identified the top 5,000 genes in terms of the highest variability for inclusion in subsequent investigation. The threshold value was adjusted to 5 for a softer setting. The weighted adjacency matrix was converted into a topological overlap matrix (TOM) in order to assess network connectivity. The cluster tree construction of the TOM matrix was established using the hierarchical clustering technique, with various branches and colors of the cluster tree representing distinct gene modules. Genes were grouped into modules based on their genetic patterns according to the weighted correlation coefficient. Genes with analogous patterns were classified into modules, leading to the formation of multiple modules according to gene expression patterns.
Gene module functional enrichment analysis
To elucidate the biological functions and cellular pathways associated with the primary modules identified in the WGCNA, particularly the red module which showed the strongest correlation with SEC14L4 expression, we performed a functional analysis of the genes within this module. This analysis was conducted using the Metascape database (www.metascape.org), providing a comprehensive assessment of their functional correlations. Gene Ontology (GO) pathway analysis was conducted for the identified genes. A minimum overlap of ≥3 and significance threshold of P≤0.01 were deemed to indicate statistical significance.
Drug susceptibility analysis
The “pRRophetic” package in R software was used to predict the chemotherapy response of each tumor sample based on the Genomics of Drug Sensitivity in Cancer (GDSC) pharmacogenomics database (available at https://www.cancerrxgene.org/). Regression analysis was employed to obtain the half maximal inhibitory concentration (IC50) estimates for every specific chemotherapy drug treatment. The accuracy of regression and prediction were evaluated used 10-fold cross-validation with the GDSC training set. Default settings were selected for all arguments, including “combat” to mitigate mass effects, and the average of duplicate gene expression was used.
Single-cell analysis
The profile of gene expression was processed using the “Seurat” package in R, and genes with low expression were filtered out. The data were then subjected to normalization and homogenization, followed by the determination of positional relationships among clusters through t-distributed stochastic neighbor embedding (tSNE) analysis. Clusters were annotated through the notation file supplied in the “celldex” package in R, and cells with significant implications for disease occurrence were identified.
Cell culture
Human normal esophageal epithelial cells (HEECs) and esophageal human squamous cell carcinoma cells (KYSE520 and KYSE150) were acquired from the Cell Bank of the Chinese Academy of Sciences (Shanghai, China). Cells were grown in six-well dishes in a moist environment with 5% CO2 with Dulbecco’s Modified Eagle Medium (DMEM) and 10% fetal bovine serum (cat. no. C0232; Gibco, Thermo Fisher Scientific, Waltham, MA, USA) and preserved at 37 ℃.
Quantitative real-time polymerase chain reaction (qRT-PCR)
Total RNA was extracted from cells using TRIzol reagent (Merck KGaA, Darmstadt, Germany). RNA concentration was determined with a NanoDrop microspectrophotometer (Thermo Fisher Scientific). Reverse transcription of mRNA was carried out by means of a Revert Aid First Strand cDNA Synthesis Kit (Thermo Fisher Scientific). qRT-PCR was conducted using 2× SYBR Green Mix (Thermo Fisher Scientific) with an ABI StepOne Plus system (Applied Biosystems, Thermo Fisher Scientific). Levels of gene expression were tested using the 2−ΔΔCt technique. Each sample was subjected to triplicate treatments in three independent experiments. All primers are listed in Table 1.
Table 1
Gene | Primer sequence |
---|---|
SEC14L4 | F: 5'-TATGACTCGGGTGGTCTTTGT-3' |
R: 5'-GGTCGAGGGACCCAATGATG-3' | |
GAPDH | F: 5'-TGACTTCAACAGCGACACCCA-3' |
R: 5'-CACCCTGTTGCTGTAGCCAAA-3' |
qRT-PCR, quantitative real-time polymerase chain reaction; F, forward; R, reverse; SEC14L4, Saccharomyces cerevisiae-like 4; GAPDH, glyceraldehyde-3-phosphate dehydrogenase.
Statistical analysis
R version 4.3.0 (https://www.R-project.org) was used for bioinformatics statistical analysis, while GraphPad Prism 9.4.0 (GraphPad Software, Inc., La Jolla, CA, USA) was employed to analyze the gene expression of SEC14L4. Differences in outcomes among patients with ESCC were evaluated using Kaplan-Meier curve and log-rank analysis. Prognosis was assessed using the Cox proportional hazards regression model. Variables between groups were compared with the Student t-test, while Spearman correlation coefficient was used to evaluate correlations between continuous variables. A P<0.05 was deemed to indicate a statistical difference in all analyses.
Results
High expression of SEC14L4 in advanced ESCC
ESCC-related expression data were obtained from TCGA database, and a significant upregulation of SEC14L4 expression in tumor samples compared with that in normal tissues was observed (P<0.001; Figure 1A). In addition, the correlation between SEC14L4 expression and clinical parameters was investigated. The analysis revealed a significant association between SEC14L4 expression and advanced tumor-node-metastasis (TNM) stage (P=0.03; Figure 1B) as well as T stage (P=0.005; Figure 1C). No significant differences in SEC14L4 expression were observed with respect to lymph node metastasis (P=0.23; Figure 1D), gender (P=0.36; Figure 1E), or metastasis (P=0.54; Figure 1F).
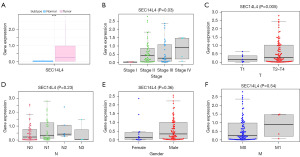
SEC14L4 as an indicator of poor prognosis in patients with advanced ESCC
Survival analysis based on TCGA database showed that there was a significant difference in survival outcomes between individuals with high and low expression levels of the SEC14L4 gene (P=0.045; Figure 2A). Subgroup analysis of survival based on T stage and TNM stage was also performed. The P values in the subgroups of T3–T4, T1–T2, TNM stage III–IV, and TNM stage I–II were 0.051, 0.84, 0.04, and 0.27, respectively (Figure 2B-2E). The P value (0.051), indicating potential significance in the T3–T4 subgroup, was close to 0.05. We constructed univariate and multivariate Cox regression models and forest plots based on clinical data and SEC14L4 gene expression (Figure 2F,2G).
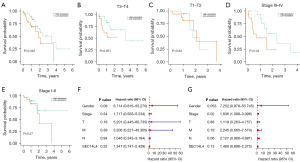
Nomogram model analysis of SEC14L4
Nomogram model analysis demonstrated that different clinical parameters of ESCC, along with SEC14L4 expression, contributed to the scoring in varying degrees across all samples (Figure 3A). Additionally, prediction analyses for 1- and 2-year overall survival (OS) were performed (Figure 3B). The results demonstrated consistency between predicted and observed OS, indicating good predictive efficacy of the nomogram model.
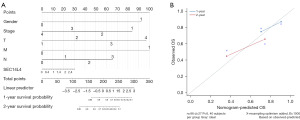
Mutation and correlation analysis of SEC14L4
A comparison was made between the mutant gene variations in the SNP-related data of the two groups. The top 30 genes in terms of mutation rate were selected for visualization. The mutation map was displayed (Figure 4A). The findings indicated a significantly higher rate of TP53 and mutations in other genes in the high expression group compared to the low expression group. Furthermore, through correlation analysis, we characterized the network of co-expression of SEC14L4 based on the expression profiles of patients with ESCC downloaded from TCGA. A total of 698 genes significantly corelated with SEC14L4 expression were identified. The heat map of the top 10 genes in terms of positive or negative correlation coefficients is displayed in Figure 4B, and the co-expression correlation circle map can be found in Figure 4C.
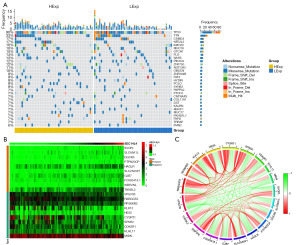
WGCNA
To investigate the regulatory network of SEC14L4 in ESCC, we constructed a WGCNA network based on ESCC data. A soft threshold (β) of 5 was applied, followed by detection of gene modules based on the TOM. A total of 14 gene modules were identified in ESCC, as shown in Figure 5A and represented by various colors and corresponding gene counts. Subsequently, we examined the correlation between modules and traits, which indicated that the red module exhibited the closest relationship with SEC14L4 (correlation coefficient =0.26; P=0.009; Figure 5B). Furthermore, pathway analysis was performed using genes from the red module, demonstrating enrichment primarily in pathways related to the regulation of DNA metabolic processes, transcription factor binding, and other pathways (Figure 5C).
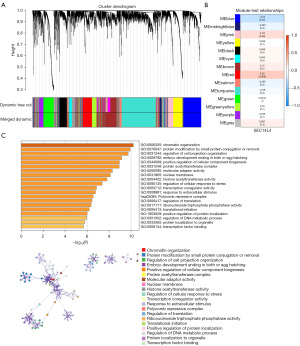
The pathways involved the high expression of SEC14L4
We next investigated the special signaling pathways associated with SEC14L4 and examined the underlying molecular processes through which SEC14L4 affects cancer progression. The results of GSVA revealed that apoptosis, the IL6/JAK/STAT3 (interleukin-6/Janus kinase/signal transducer and activator of transcription 3) signaling pathway, the reactive oxygen species pathway, and other signaling pathways were enriched in patients with elevated SEC14L4 expression (Figure 6A). Additionally, GSEA results demonstrated that SEC14L4 is associated with enrichment in the pentose phosphate pathway, DNA replication pathway, and glutathione metabolism signaling pathway (Figure 6B,6C).
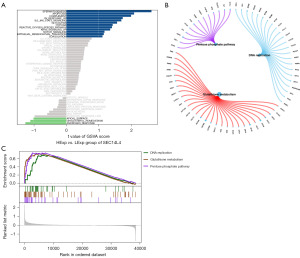
Drug sensitivity associated with the high expression of SEC14L4
Early-stage ESCC exhibits a pronounced treatment response to a combination of surgery and chemotherapy. Using drug sensitivity information from the GDSC database, we employed the “pRRophetic” R package to predict the response to chemotherapy for individual tumor cases. We then examined the relationship between SEC14L4 expression and sensitivity to standard chemotherapy medications. This analysis identified a close relationship between SEC14L4 expression and ESCC sensitivity to the AICAR, BMS.708163, GNF.2, Nutlin.3a, PD.0325901, and RDEA119 chemotherapeutic drugs (Figure 7A-7F).
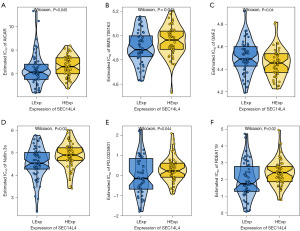
Single-cell analysis results
The single-cell dataset GSE196756 was obtained from the NCBI GEO public database, and 23 subtypes were obtained using tSNE (Figure 8A). All clusters were annotated into three cell categories: natural killer (NK) cells, monocytes, and B cells (Figure 8B). Additionally, SEC14L4 gene expression was examined in NK cells, monocytes, and B cells at the individual cell level (Figure 8C), with the highest expression of SEC14L4 being observed in monocytes.
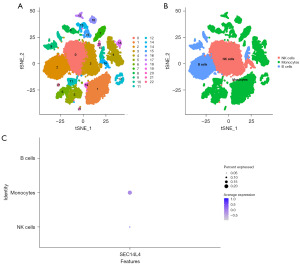
qRT-PCR analysis of SEC14L4 expression
The expression of SEC14L4 was higher in KYSE520 and KYSE150 compared to HEECs (Figure 9).
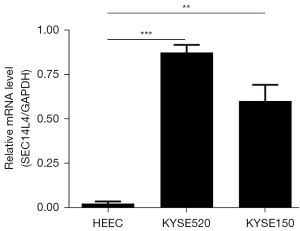
Discussion
In our study, the expression of SEC14L4 was found to be elevated in tumor tissue, particularly in patients with late-stage ESCC. The high expression of SEC14L4 may serve as an indicator of poor prognosis in those with ESCC (P=0.045), particularly those with locally advanced disease (TNM stage III and IV: P=0.04; T3 and T4: P=0.051). The expression of SEC14L4 and various clinical indicators contributed differently to ESCC prognosis according to the results of the nomogram model, which indicated that the nomogram model exhibited good efficacy in the prediction of survival. Subsequently, SNPs and co-expressed genes of SEC14L4 were identified and the pathways associated with SEC14L4 explored, including DNA metabolic processes, transcription factor binding, apoptosis, and the IL6/JAK/STAT3 signaling pathway, among others. In the single-cell analysis, the expression of SEC14L4 was predominantly observed in monocytes. Subsequent analyses identified an association between SEC14L4 expression and sensitivity to BMS.708163, GNF.2, Nutlin.3a, PD.0325901, RDEA119, and AICAR. The high expression of SEC14L4 was confirmed in KYSE520 and KYSE150.
The 5-year survival rate of patients ESCC remains unsatisfactory because this disease is often diagnosed at an advanced stage, even with comprehensive treatments such as surgery supplemented with neoadjuvant chemoradiotherapy, postoperative adjuvant chemoradiotherapy, endoscopic treatment, and immunotherapy (20). In such cases, predicting prognosis is critical. The significance of prognostic biomarkers in ESCC patients has garnered widespread attention in recent years. Various biomarkers, including liquid biopsy markers such as circulating tumor cells and circulating tumor DNA, genetic biomarkers such as microsatellite instability and SNPs, epigenetic biomarkers such as microRNAs and long noncoding RNAs, and proteomics, have emerged in clinical practice or attracted research interest (11,12). However, single biomarkers often fail to meet clinical needs comprehensively. Combining multiple prognostic markers can provide a more reliable prognosis for patients with esophageal cancer. In our study, SEC14L4 emerged as a prognostic indicator of adverse outcome in patients with ESCC. Research on SEC14L4 in cancer is limited, and its role in esophageal cancer has not been explored previously. To our knowledge, this study is the first to investigate the involvement of SEC14L4 in esophageal cancer. Further investigation into the prognostic significance of SEC14L4 in ESCC is warranted.
The SEC14 superfamily encodes yeast phosphatidylinositol transfer proteins, which regulate crucial interfaces between membrane trafficking pathways and lipid metabolism (21). Yeast SEC14P is the original member of the SEC14 domain superfamily, and the NCBI database includes 1,551 SEC14 domains (www.ncbi.nlm.nih.gov) (13). SEC14L4 is associated with mammalian Golgi dynamics proteins, and SEC14-like proteins are uniquely found in all eukaryotic genomes (22). Alterations in lipid metabolism and glycerophospholipids in cancer cells can affect the immune system response. Hence, there is a possibility of identifying biomarkers for treatments and diagnoses that target the lipid composition of cell membranes and extracellular vesicles in cancer cells (23). Some studies have investigated the SEC14 family in cancers. For example, the expression of SEC14L1 was discovered to be positive in gynecologic malignancies (17). Zhang et al. reported that the high expression of SEC14L2 suggests a poor prognosis, and changes in the competing endogenous RNA-based SEC14L2-SLC6A1 axis may impact the tumor immune microenvironment in hepatocellular carcinoma (24). However, the relationship between SEC14L4 and ESCC remains understudied. Thus, in this study, we elucidated the correlation between SEC14L4 and various genes and pathways.
The top 30 SNP-related genes, including TP53, TTN, CSMD3, NOTCH1, and NFE2L2, were identified. Genes co-expressed with SEC14L4 were also investigated and visualized. Signaling pathways including glutathione metabolism signaling pathways, DNA metabolic processes, transcription factor binding pathways, apoptosis, and reactive oxygen species pathway were associated with the high expression of SEC14L4. Moreover, the results of qRT-PCR showed that expression of SEC14L4 in human ESCC cells was higher than that in HEECs. In recent years, the tumor microenvironment has been investigated through single-cell transcriptomic analysis by many researchers in ESCC. Immune cell types including B cells, T cells, mast cells, epithelial cells, monocytes, NK cells, fibroblasts, and other immune cells have been examined by single-cell transcriptomic analysis (25-28). Therefore, we aimed to clarify how SEC14L4 influences the tumor microenvironment in ESCC. In our study, all clusters were annotated into NK cells, monocytes, and B cells, with the highest expression of SEC14L4 being found in monocytes.
The evolution of early ESCC is influenced by a complex interplay between genetic alterations and the immune microenvironment. Genetic mutations and chromosomal changes play a pivotal role in the early stages of ESCC. These mutations can disrupt normal cell growth and regulation, leading to tumor initiation. Key genes and genetic pathways implicated in early ESCC include: TP53 mutations, Notch pathway mutations, PI3K/AKT pathway, chromosomal instability (CIN) and so on (29). The immune microenvironment in early ESCC plays a critical role in determining whether the immune system can effectively suppress tumor growth or whether the tumor can evade immune surveillance and progress, such as: tumor-infiltrating lymphocytes (TILs), regulatory T cells (Tregs) and myeloid-derived suppressor cells (MDSCs), immune checkpoints, tumor-associated macrophages (TAMs), cytokines and chemokines (30). Genetic alterations and the immune microenvironment are interdependent in shaping the evolution of early ESCC. Mutations in certain genes can alter the immune landscape of the tumor by modulating immune evasion mechanisms, altering cytokine production and impacting tumor antigenicity (31). In the tumor microenvironment, monocytes and their differentiated forms, known as TAMs, are often reprogrammed by tumor cells to suppress T cell activity, thereby facilitating tumor growth and metastasis (32). These cells secrete various factors that promote angiogenesis [such as vascular endothelial growth factory (VEGF)], immunosuppression (such as IL-10), and tissue remodeling [such as matrix metalloproteinases (MMPs)], enabling tumors to evade immune surveillance. The role of monocytes within the immune microenvironment is highly complex. On the one hand, they can initiate and modulate immune responses to combat pathogens and tumor cells. On the other hand, under certain conditions, they may support tumor progression and metastasis (33). A more comprehensive understanding of the diverse roles of monocytes in the immune microenvironment will be crucial for developing novel therapeutic strategies, particularly in the context of cancer immunotherapy (34). These indicated valuable insights into the molecular mechanisms underlying the association between SEC14L4 and ESCC, as well as potential therapeutic strategies.
SEC14 domain proteins not only affect the development of antifungals but also influence their virulence (15). SEC14L5 was reported to be a drug resistance-related gene in lung cancer (35). However, as a member of the SEC family, SEC14L4 does not serve as a predictor of immunotherapy response in lung adenocarcinoma (18). The chemotherapy drug sensitivity appears to be particularly important due to the vital role of chemotherapy in preventing metastasis in ESCC patients after surgery. Based on the drug sensitivity information obtained from the GDSC database, we explored the sensitivity of ESCC to common chemotherapy drugs in patients with high SEC14L4 expression. We discovered that SEC14L4 was significantly associated with sensitivity to AICAR, BMS.708163, GNF.2, Nutlin.3a, PD.0325901, and RDEA119. These drugs may have potential as chemotherapeutic agents in treating ESCC.
Our study involved certain limitations that should be acknowledged. First, we did not conduct additional in vitro and in vivo tests with clinical specimens. Second, additional exploratory experiments are required to fully elucidate the functions of SEC14L4 in ESCC. These limitations present opportunities for future research into the function of SEC14L4 in ESCC.
Conclusions
Our results indicate that increased SEC14L4 expression is associated with unfavorable clinical prognosis in patients ESCC, emphasizing its potential as a target for drug development and providing insights into its molecular mechanisms in ESCC.
Acknowledgments
Funding: None.
Footnote
Reporting Checklist: The authors have completed the TRIPOD reporting checklist. Available at https://tcr.amegroups.com/article/view/10.21037/tcr-24-1657/rc
Data Sharing Statement: Available at https://tcr.amegroups.com/article/view/10.21037/tcr-24-1657/dss
Peer Review File: Available at https://tcr.amegroups.com/article/view/10.21037/tcr-24-1657/prf
Conflicts of Interest: All authors have completed the ICMJE uniform disclosure form (available at https://tcr.amegroups.com/article/view/10.21037/tcr-24-1657/coif). A.S. reports his consulting or advisory board role with AstraZeneca, Bristol-Myers Squibb, Merck, Exelixis, Pfizer, Xilio therapeutics, Taiho, Amgen, Autem therapeutics, KAHR medical, and Daiichi Sankyo; and he also receives institutional research funding from AstraZeneca, Bristol-Myers Squibb, Merck, Clovis, Exelixis, Actuate therapeutics, Incyte Corporation, Daiichi Sankyo, Five prime therapeutics, Amgen, Innovent biologics, Dragonfly therapeutics, Oxford Biotherapeutics, Arcus therapeutics, and KAHR medical, outside the submitted work. The other authors have no conflicts of interest to declare.
Ethical Statement: The authors are accountable for all aspects of the work in ensuring that questions related to the accuracy or integrity of any part of the work are appropriately investigated and resolved. The study was conducted in accordance with the Declaration of Helsinki (as revised in 2013).
Open Access Statement: This is an Open Access article distributed in accordance with the Creative Commons Attribution-NonCommercial-NoDerivs 4.0 International License (CC BY-NC-ND 4.0), which permits the non-commercial replication and distribution of the article with the strict proviso that no changes or edits are made and the original work is properly cited (including links to both the formal publication through the relevant DOI and the license). See: https://creativecommons.org/licenses/by-nc-nd/4.0/.
References
- Sung H, Ferlay J, Siegel RL, et al. Global Cancer Statistics 2020: GLOBOCAN Estimates of Incidence and Mortality Worldwide for 36 Cancers in 185 Countries. CA Cancer J Clin 2021;71:209-49. [Crossref] [PubMed]
- Lagergren J, Smyth E, Cunningham D, et al. Oesophageal cancer. Lancet 2017;390:2383-96. [Crossref] [PubMed]
- Morgan E, Soerjomataram I, Rumgay H, et al. The Global Landscape of Esophageal Squamous Cell Carcinoma and Esophageal Adenocarcinoma Incidence and Mortality in 2020 and Projections to 2040: New Estimates From GLOBOCAN 2020. Gastroenterology 2022;163:649-658.e2. [Crossref] [PubMed]
- Domper Arnal MJ, Ferrández Arenas Á, Lanas Arbeloa Á. Esophageal cancer: Risk factors, screening and endoscopic treatment in Western and Eastern countries. World J Gastroenterol 2015;21:7933-43. [Crossref] [PubMed]
- Ikebe M, Morita M, Yamamoto M, et al. Neoadjuvant therapy for advanced esophageal cancer: the impact on surgical management. Gen Thorac Cardiovasc Surg 2016;64:386-94. [Crossref] [PubMed]
- Yang H, Liu H, Chen Y, et al. Neoadjuvant Chemoradiotherapy Followed by Surgery Versus Surgery Alone for Locally Advanced Squamous Cell Carcinoma of the Esophagus (NEOCRTEC5010): A Phase III Multicenter, Randomized, Open-Label Clinical Trial. J Clin Oncol 2018;36:2796-803. [Crossref] [PubMed]
- Ilson D, Lordick F. Definitive or neoadjuvant chemoradiotherapy for squamous cell oesophageal cancer? Lancet Oncol 2018;19:1285-6. [Crossref] [PubMed]
- Luan S, Zeng X, Zhang C, et al. Advances in Drug Resistance of Esophageal Cancer: From the Perspective of Tumor Microenvironment. Front Cell Dev Biol 2021;9:664816. [Crossref] [PubMed]
- Puhr HC, Prager GW, Ilhan-Mutlu A. How we treat esophageal squamous cell carcinoma. ESMO Open 2023;8:100789. [Crossref] [PubMed]
- Huang FL, Yu SJ. Esophageal cancer: Risk factors, genetic association, and treatment. Asian J Surg 2018;41:210-5. [Crossref] [PubMed]
- Zhou N, Hofstetter WL. Prognostic and therapeutic molecular markers in the clinical management of esophageal cancer. Expert Rev Mol Diagn 2020;20:401-11. [Crossref] [PubMed]
- Yang W, Han Y, Zhao X, et al. Advances in prognostic biomarkers for esophageal cancer. Expert Rev Mol Diagn 2019;19:109-19. [Crossref] [PubMed]
- Bankaitis VA, Mousley CJ, Schaaf G. The Sec14 superfamily and mechanisms for crosstalk between lipid metabolism and lipid signaling. Trends Biochem Sci 2010;35:150-60. [Crossref] [PubMed]
- Chinen K, Takahashi E, Nakamura Y. Isolation and mapping of a human gene (SEC14L), partially homologous to yeast SEC14, that contains a variable number of tandem repeats (VNTR) site in its 3' untranslated region. Cytogenet Cell Genet 1996;73:218-23. [Crossref] [PubMed]
- Holič R, Šťastný D, Griač P. Sec14 family of lipid transfer proteins in yeasts. Biochim Biophys Acta Mol Cell Biol Lipids 2021;1866:158990. [Crossref] [PubMed]
- Jiao R, Jiang W, Xu K, et al. Lipid metabolism analysis in esophageal cancer and associated drug discovery. J Pharm Anal 2024;14:1-15. [Crossref] [PubMed]
- Banet N, Masnick M, Quddus MR. Evaluation of Saccharomyces cerevisiae -like 1 (SEC14L1) in Gynecologic Malignancies Shows Overexpression in Endometrial Serous Carcinoma. Int J Gynecol Pathol 2023;42:136-42. [Crossref] [PubMed]
- Huang X, Feng Y, Li Y, et al. A novel transcriptomic signature associated with lymphovascular invasion predicts clinical outcomes, tumor microenvironment, and therapeutic response in lung adenocarcinoma. Int Immunopharmacol 2024;127:111286. [Crossref] [PubMed]
- Kempna P, Ricciarelli R, Azzi A, et al. Alternative splicing and gene polymorphism of the human TAP3/SEC14L4 gene. Mol Biol Rep 2010;37:3503-8. [Crossref] [PubMed]
- Watanabe M, Otake R, Kozuki R, et al. Recent progress in multidisciplinary treatment for patients with esophageal cancer. Surg Today 2020;50:12-20. [Crossref] [PubMed]
- Schaaf G, Ortlund EA, Tyeryar KR, et al. Functional anatomy of phospholipid binding and regulation of phosphoinositide homeostasis by proteins of the sec14 superfamily. Mol Cell 2008;29:191-206. [Crossref] [PubMed]
- Ghosh R, Bankaitis VA. Phosphatidylinositol transfer proteins: negotiating the regulatory interface between lipid metabolism and lipid signaling in diverse cellular processes. Biofactors 2011;37:290-308. [Crossref] [PubMed]
- Sarmento MJ, Llorente A, Petan T, et al. The expanding organelle lipidomes: current knowledge and challenges. Cell Mol Life Sci 2023;80:237. [Crossref] [PubMed]
- Zhang DD, Shi Y, Liu JB, et al. Construction of a Myc-associated ceRNA network reveals a prognostic signature in hepatocellular carcinoma. Mol Ther Nucleic Acids 2021;24:1033-50. [Crossref] [PubMed]
- Chen Y, Zhu S, Liu T, et al. Epithelial cells activate fibroblasts to promote esophageal cancer development. Cancer Cell 2023;41:903-918.e8. [Crossref] [PubMed]
- Zhang X, Peng L, Luo Y, et al. Dissecting esophageal squamous-cell carcinoma ecosystem by single-cell transcriptomic analysis. Nat Commun 2021;12:5291. [Crossref] [PubMed]
- Dinh HQ, Pan F, Wang G, et al. Integrated single-cell transcriptome analysis reveals heterogeneity of esophageal squamous cell carcinoma microenvironment. Nat Commun 2021;12:7335. [Crossref] [PubMed]
- Sui X, Chen C, Zhou X, et al. Integrative analysis of bulk and single-cell gene expression profiles to identify tumor-associated macrophage-derived CCL18 as a therapeutic target of esophageal squamous cell carcinoma. J Exp Clin Cancer Res 2023;42:51. [Crossref] [PubMed]
- Zheng S, Liu B, Guan X. The Role of Tumor Microenvironment in Invasion and Metastasis of Esophageal Squamous Cell Carcinoma. Front Oncol 2022;12:911285. [Crossref] [PubMed]
- Li R, Huang B, Tian H, et al. Immune evasion in esophageal squamous cell cancer: From the perspective of tumor microenvironment. Front Oncol 2023;12:1096717. [Crossref] [PubMed]
- Baba Y, Nomoto D, Okadome K, et al. Tumor immune microenvironment and immune checkpoint inhibitors in esophageal squamous cell carcinoma. Cancer Sci 2020;111:3132-41. [Crossref] [PubMed]
- Coillard A, Segura E. In vivo Differentiation of Human Monocytes. Front Immunol 2019;10:1907. [Crossref] [PubMed]
- Olingy CE, Dinh HQ, Hedrick CC. Monocyte heterogeneity and functions in cancer. J Leukoc Biol 2019;106:309-22. [Crossref] [PubMed]
- Carroll TM, Chadwick JA, Owen RP, et al. Tumor monocyte content predicts immunochemotherapy outcomes in esophageal adenocarcinoma. Cancer Cell 2023;41:1222-1241.e7. [Crossref] [PubMed]
- Zhu L, Chen P, Wang H, et al. Analysis of prognostic and therapeutic values of drug resistance-related genes in the lung cancer microenvironment. Transl Cancer Res 2022;11:339-57. [Crossref] [PubMed]