Exploring a specific type of tissue-resident natural killer cell involved in the anti-tumor and immunotherapy response in human papillomavirus-positive head and neck squamous cell carcinoma using scRNA-seq
Highlight box
Key findings
• We identified a specific type of tissue-resident natural killer (trNK) cell involved in the anti-tumor and immunotherapy response in human papillomavirus (HPV)-positive head and neck squamous cell carcinoma (HNSCC) patients.
What is known and what is new?
• HPV-positive HNSCC is a common malignancy with a complex immune microenvironment that includes natural killer (NK) cells, which are known for their roles in tumor surveillance and the immune response.
• This study was the first to identify a specific trNK cell subset (trNK-0) in HPV-positive HNSCC that may contribute to anti-tumor immunity and could serve as a predictor of the immunotherapy response. Our findings provide a new perspective for targeted-treatment strategies.
What is the implication, and what should change now?
• The implications of our findings are significant. There is an urgent need to explore the role of NK cells in immunotherapy for HPV-positive HNSCC. Immediate actions should focus on accelerating clinical trials that leverage NK cell activity, enhancing research into the mechanisms of NK cell interactions with HPV-positive tumors, and integrating this knowledge into medical education to refine therapeutic approaches.
Introduction
Head and neck squamous cell carcinomas (HNSCCs) are invasive and highly heterogeneous carcinomas, which can develop from various mucosal epithelium sites in the upper airway and digestive tract (1). Increasingly, oropharyngeal HNSCC is closely associated with oncogenic human papillomavirus (HPV) strains, primarily the high-risk HPV-16 genotypes, which contribute to over 70% of new cases of oropharyngeal HNSCCs in North American and Europe. Additionally, HPV-18, -31, -33, and -52 genotypes, and other strains that have been detected in a small proportion of patients (2).
It is well established that high-risk HPV infections play a carcinogenic role by integrating viral DNA into host cells, and promoting E6 and E7 oncogene expression (2). Over recent decades, the epidemiology of HNSCC has changed significantly, such that there has been a decrease in smoking-related cancers, and an increase in HPV-positive cancers (3). With this epidemiological shift, it has become clear that in addition to having varied outcomes, HPV-positive and HPV-negative HNSCC are characterized by distinctly different gene expression, mutational, and immune profiles; for example, a seminal analysis showed that the overall survival of HPV-positive HNSCC patients with no history of smoking was significantly improved compared to that of HPV-negative HNSCC patients (4). While, overall survival is improved in HPV-positive HNSCC, there remain patients who do not respond well to treatment. Therefore, there is an urgent need to explore the underlying tumor progression mechanism and to develop useful prognostic indicators for treatment and interventions for HPV-positive HNSCC (5).
The tumor microenvironment (TME) of HNSCC comprises a heterogeneous and complex mix of cancer cells and stromal cells, including various immune cells, endothelial cells, and cancer-associated fibroblasts. In addition, the TME of HNSCC can display impaired tumor-infiltrating lymphocyte (TIL) function, as the tumor cells produce interleukin (IL)-10 and transforming growth factor beta (TGF-β) cytokines, which promote immunosuppressive M2 macrophage polarization (6). Natural killer (NK) cells, which comprise cluster of differentiation CD3−CD16+CD56dim lymphocytes, are a crucial component of the immune system, and are also referred to as conventional natural killer (cNK) cells. cNK cells account for about 5–15% of circulating lymphocytes (7). In fighting solid tumors, NK cells may have a number advantages over T cells; for example, NK cells may enhance radioimmunotherapy and chemotherapy response through the direct cytotoxicity of granzymes and perforin, or the secretion of cytokines and chemokines [tumor necrosis factor alpha (TNF-α) and interferon gamma (IFN-γ)] against tumor cells (8). A study showed that NK cells with different origins exhibit distinct phenotypes and behavior characteristics, while recent research has shown that tissue-resident natural killer (trNK) cells are unevenly distributed at different sites (e.g., at the mucosal barrier) (9). Such findings have greatly improved our understanding of NK cell diversity, and shown that NK cells share common markers and unique tissue-specific features that may be shaped by local TME factors (9). In general, HPV-positive HNSCC tumors have high infiltration levels of immune cells. Thus, exploring the unique features of the trNK population may improve the clinical prognosis of patients.
With the continuous development of high-throughput sequencing technology, the pace of tumor molecular profiling and the identification of useful prognostic biomarkers have both increased dramatically. Single-cell RNA-sequencing (scRNA-seq) holds significant value in exploring tumor heterogeneity, offering insights into the complexity of the TME and revealing the roles of immune cells within it. It can identify and classify immune cells, uncover their evasion mechanisms, and drug resistance, which is crucial for the development of targeted therapies. Moreover, scRNA-seq can characterize tumor-specific subpopulations that may affect prognosis and treatment strategies (10). A number of HNSCC biomarkers have been proposed, among which, aldehyde dehydrogenase 1 (ALDH1), cluster of differentiation 44 (CD44), and cluster of differentiation 133 (CD133) have been the most extensively validated and have been shown to have observable prognostic significance (11). In the present study, we performed a comprehensive scRNA-seq analysis of HPV-positive HNSCC, including pairs of peripheral blood mononuclear cell (PBMC) and TIL samples, to explore the immune and clinicopathological features of the trNK cell population. Univariate and multivariate analyses were performed to confirm the cancer progression-related clinicopathological factors, and immune-infiltration and immune-checkpoint analyses were conducted to provide a basis for choosing the optimal immunotherapy. We present this article in accordance with the MDAR reporting checklist (available at https://tcr.amegroups.com/article/view/10.21037/tcr-24-1535/rc).
Methods
Data acquisition and processing of scRNA-seq data
The scRNA-seq dataset (GSE139324) was obtained from the Gene Expression Omnibus (GEO) database (https://www.ncbi.nlm.nih.gov/geo/). The dataset comprised the data of 8 HPV-positive HNSCC patients, and each patient had pairs of PBMC and TIL samples, forming a total of 16 samples. The “Seurat” R package was used to process the single-cell data, and the “Harmony” R package was used to remove the batch effects between the samples (10,12). The cells with 200–9,000 gene numbers that were expressed in at least 3 cells and those with less than 5% mitochondrial genes were retained (13). After filtering, these cell data were normalized to identify the highly variable genes (HVGs) using the FindVariableFeatures function of the “Seurat” package. A principal component analysis of these HVGs was then performed to identify the significant principal components. Next, the top 50 principal components were used for the cell-clustering analysis (with an initial resolution of 0.1, and a reunion resolution of NK cells of 0.4). The FindAllMarkers function was used to determine the marker genes of each cell cluster (adjusted P value <0.05). The “SingleR” package was used for the cell type annotation (14). The Cancer Genome Atlas Head-Neck Squamous Cell Carcinoma (TCGA-HNSC) dataset comprising RNA-seq data and clinical information was downloaded from the Genomic Data Commons (GDC) data portal (https://portal.gdc.cancer.gov/). The HPV subtype information of TCGA-HNSC samples was collected from the cBioPortal database (https://www.cbioportal.org/). The bulk RNA-seq data of the HNSCC GSE23036, GSE40774, and GSE65858 datasets containing clinical information were downloaded from the GEO database. The hallmark gene sets were downloaded from the Molecular Signatures Database (MSigDB; https://www.gsea-msigdb.org/gsea/msigdb) (15). The study was conducted in accordance with the Declaration of Helsinki (as revised in 2013).
Cell-communication and pathway-enrichment analyses
The “cellphonedb” Python package was used to perform the cell-communication analysis based on the standardized expression matrix of “Seurat”, using the default parameters. The “clusterProfiler” R package was used for the gene set enrichment analysis (GSEA) of the hallmark-related pathways (16).
Immune-infiltration and escape analyses
The immune cell infiltration and function analyses were performed using the “gene set variation analysis” (“GSVA”) R package. The Tumor Immune Dysfunction and Exclusion (TIDE) analysis was conducted using the TIDE score tool (http://tide.dfci.harvard.edu/). The Wilcoxon rank-sum test was used to compare differences in the immune-infiltration score, immune function score, and TIDE score between the samples (17). In addition, we obtained the immunotherapy GSE123813 dataset, comprising scRNA-seq data and cell annotation information, from the Tumor Immune Single-Cell Hub (TISCH; http://tisch.comp-genomics.org/home/), and the trNK scores were then calculated, and the differences in the scores were compared before and after treatment.
Statistical analysis
Unless otherwise indicated, all the statistical analyses and plots were completed using the R software (version 4.1.3), and a P value <0.05 was considered statistically significant. The “survival” and “survminer” packages were used for the log-rank test and to plot the survival curves (18). The Wilcoxon rank-sum test was used to compare the continuous variables between two groups, and the Kruskal-Wallis test was used to compare the continuous variables between three or more groups. Fisher’s exact test or the Chi-square test was used for comparisons between subtypes.
Results
The single-cell landscape of HNSCC
After cell filtration and unbiased clustering, a total of 30,562 cells were classified into 9 main cell clusters. Based on the expression of gene markers (as detailed in Table S1), the cell clusters were annotated and sorted into six primary cell types, illustrated in Figure 1A: T cells were identified by CD3D; NK cells and natural killer T (NKT) cells were identified by NKG7; B cells and plasma cells were identified by CD79A; NK cells were also characterized by GNLY; and macrophages were identified by CD68 (as shown in Figure 1B). Interestingly, a further analysis revealed that the PBMC samples had higher proportions of NK cells and macrophages, while the TIL samples had a higher proportion of B cells (Figure 1C). Additionally, the top 10 HVGs demonstrated significant variation across the distinct cell types (Figure 1D).
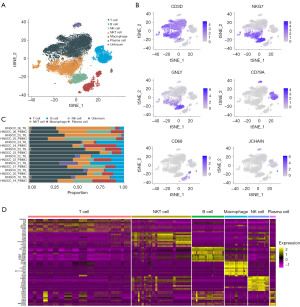
The heterogeneous patterns and functions of identified trNK cells
To examine the heterogeneity of the NK cells in HNSCC, a total of 5 NK cell subgroups were obtained after the dimensionality reduction clustering of NK cells (Figure 2A). Notably, 3 NK subgroups had a higher proportion of TIL samples and were defined as trNK cells (i.e., trNK 0, 1, and 2), while the 2 other NK subgroups were defined as tumor-associated NK cells (i.e., taNK 0 and 1) (Figure 2A). Subsequently, we examined the correlation between the gene expression profiles and the NK cell clusters, but did not find any significant correlation between the trNK subgroups (Figure 2B), which suggested high heterogeneity among the trNK subpopulations. The marker genes of the trNK cell subgroups are shown in Figure 2C. These trNK subgroups also exhibited diverse hallmark activity differences; for example, trNK-1 had a higher IFN-γ response, and the phosphatidylinositol 3-kinase-protein kinase B-mechanistic target of rapamycin (PI3K-Akt-mTOR) and mTOR complex 1 (mTORC1) signaling pathway activity, while most hallmark pathways (e.g., allograft rejection and Myc target V1) in trNK-0 and -2 were inhibited, which suggests that these trNK cells may exert anti-tumor effects (Figure 2D). Meanwhile, the taNK cells were highly enriched in epithelial mesenchymal transition and TNF-α signaling via nuclear factor-kappa B (NF-κB) (Figure 2D), which suggests taNK cells have some oncogenic features.
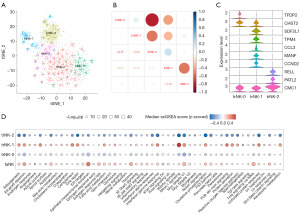
Functional trNK cells may be activated by macrophage crosstalk
Utilizing CellPhoneDB, we explored the crosstalk characteristics of trNK cells within the TME. Our analysis revealed that trNK cells were extensively engaged in immune crosstalk with a variety of immune cells, as depicted in Figure 3A. The trNK cells expressed a suite of receptors pivotal for this interaction, including annexin A1 (ANXA1) paired with its receptor formyl peptide receptor 1 (FPR1), bone marrow stromal antigen 2 (BST2) interacting with leukocyte immunoglobulin-like receptor A4 (LILRA4), C-C motif chemokine ligand 3 (CCL3) binding to its receptor C-C motif chemokine receptor 1 (CCR1), CD47 known to interact with signal regulatory protein alpha (SIRPα), CD99 associated with paired immunoglobulin-like type 1 receptor A (PILRA), and low-density lipoprotein-related protein 1 (LRP1) partnering with sortilin 1 (SORT1). These receptor-ligand pairs facilitated the recruitment of macrophages, which in turn, through a complex network of interactions involving amyloid precursor protein (APP) and sortilin-related receptor 1 (SORL1), intercellular adhesion molecule 1 (ICAM1) and integrin alpha L (ITGAL), transforming growth factor beta 1 (TGFB1) and its receptor transforming growth factor beta receptor type 3 (TGFBR3), the SPP1 integrin α4β1 complex, and CD93 and interferon-gamma receptor 1 (IFNGR1), captured the trNK cells, thereby activating their immune killing function, as illustrated in Figure 3A. The multitude of receptor-ligand interactions underscored the susceptibility of trNK cells to modulation by T cells through diverse interaction pathways, as shown in Figure 3B. In addition, the GSEA indicated that signaling pathways responsive to both interferon-gamma (IFN-γ) and the IL-2-STAT5 signaling axis were significantly activated (Figure 3C). These results are consistent with the interactions between trNK cells and macrophages, which are mediated through the signaling axes involving CD93 and the IFNGR1, as well as the SPP1 integrin α4β1 complex.
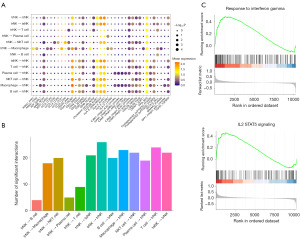
The trNK-0 score was an independent and valuable prognostic indicator
To assess the prognostic value of the trNK cells, we calculated the trNK score of each patient using the single-sample GSEA method in 520 TCGA-HNSC bulk RNA-seq samples based on the specific marker expression of the trNK cell subgroups. The patients were divided into high- and low-score groups based on the median value. The results revealed that the trNK-0 marker score was significantly correlated with outcome, such that patients with a high trNK-0 score had a better prognosis (Figure 4A). The excellent prognostic value of trNK-0 was also validated in another independent dataset (GSE65858) (Figure 4B).
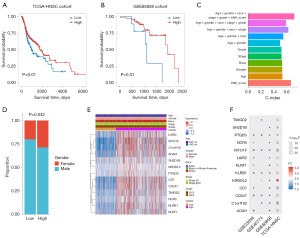
Subsequently, we conducted univariate and multivariate Cox analyses based on the trNK-0 score and other clinical factors and found that the trNK score was a significant independent prognostic factor (P<0.01, Table 1). Further, the trNK score also had the highest concordance index (C-index) value in the univariate analysis (Figure 4C), which showed the good prognostic ability of the trNK score. We then demonstrated that the model incorporating the trNK variable achieved the highest C-index value (Figure 4C). The clinical feature difference analysis of the trNK score revealed that the low-score group had a significantly higher proportion of males (P<0.05; Figure 4D). No significant difference was observed in the expression of the trNK cell marker between the groups in terms of age, gender, race and stage (Figure 4E). However, in the other 3 datasets (i.e., GSE23036, GSE40774, and GSE65858), these genes had similar expression patterns (Figure 4F), which suggests that the trNK marker gene could serve as a reliable classifier and has significant prognostic value.
Table 1
Variables | Univariate analysis | Multivariate analysis | |||
---|---|---|---|---|---|
HR (95% CI) | P value | HR (95% CI) | P value | ||
trNK score (high vs. low) | 0.70 (0.53–0.93) | 0.01 | 0.66 (0.49–0.88) | 0.004 | |
Gender (male vs. female) | 0.74 (0.56–0.99) | 0.043 | 0.70 (0.51–0.94) | 0.02 | |
Age (>60 vs. ≤60 years) | 1.26 (0.96–1.65) | 0.09 | 1.20 (0.91–1.58) | 0.21 | |
Race (Black or African American vs. Asian) | 1.42 (0.49–4.12) | 0.52 | 1.59 (0.55–4.63) | 0.40 | |
Race (White vs. Asian) | 0.88 (0.33–2.37) | 0.80 | 0.92 (0.34–2.48) | 0.87 | |
Stage (III/IV vs. I/II) | 1.16 (0.84–1.6) | 0.37 | 1.19 (0.85–1.65) | 0.31 | |
Grade (G3/4 vs. G1/2) | 0.83 (0.62–1.12) | 0.22 | 0.86 (0.64–1.17) | 0.33 |
TCGA-HNSC, The Cancer Genome Atlas Head-Neck Squamous Cell Carcinoma; HR, hazard ratio; CI, confidence interval; trNK, tissue-resident natural killer.
A higher trNK score was associated with a better immunotherapy effect
The immune-infiltration analysis showed that the patients in TCGA-HNSC cohort with high trNK scores had significantly higher immune-infiltration levels than those with low trNK scores (P<0.05), including higher levels of activated dendritic cells, B cells, and macrophages (Figure 5A). These patients also had higher immune abilities in terms of cytolytic activity, inflammation-promoting activity, parainflammation, and the Type I IFN response (Figure 5B). In addition, the patients with low trNK-0 scores had significantly higher TIDE scores (Figure 5C), and lower immune-checkpoint expression, including CD244, CD274, CTLA4, PDCD1LG2, and PDCD1 expression (Figure 5D), which suggests that these patients are more prone to immune escape and may suffer setbacks from immunotherapy. We calculated and compared the pre- and post-treatment trNK scores in the GSE123813 sample, and found that the responder patients had significantly higher trNK scores after treatment, while the non-responder patients had significantly lower rNK scores (P<0.05; Figure 5E). This provides further evidence that the trNK score is a favorable prognostic factor.
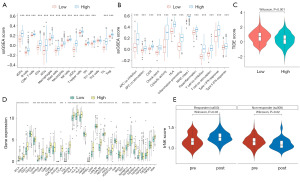
Discussion
In terms of prognosis, HNSCC exhibits enormous heterogeneity in its tumor behavior and treatment response that can substantially impact the outcomes of patients with similar disease stages. Traditional clinicopathological indicators, including tumor-node-metastasis (TNM) stage, and histological features, including the site, vascular invasion, size of positive lymph nodes, and extracapsular extension, have been shown to be inadequate in predicting individual outcomes, as no one-size-fits-all treatment strategy for HPV-positive HNSCC patients appears to be effective (19). HPV-positive HNSCC cases possess a distinct genetic profile and tumor mutational burden, marking them as a unique subset (20). Consequently, exploring the underlying immune-activation mechanism and prognostic markers that reflect tumor biological characteristics at the molecular level could be of great significance in the individualized treatment and the development of interventions for HPV-positive HNSCC patients. In this study, we performed an scRNA-seq analysis and identified a specific trNK cell population that was closely associated with HNSCC progression. Its specific marker genes could serve as an independent prognostic classifier for predicting treatment effects.
In humans, NK cells are classified into two distinct subgroups: (I) highly cytotoxic CD16+CD56dim NK cells, which are predominant in peripheral circulation; and (II) CD16+/–CD56bright NK cells, which are primarily localized to tissues and some lymphoid organs (21). The second subgroup is defined as trNK. It was first identified in the murine liver and later found in other tissues, including skin, salivary gland, mucosa and kidney tissues (22). The roles of these distinct NK cell subsets in the TME vary. For instance, CD56dim NK cells are major executors of the anti-tumor immune response due to their potent cytotoxicity. While CD56bright NK cells have lower cytotoxicity, they play a role in immune regulation, modulating the behavior of other immune cells by secreting cytokines, thereby enhancing the overall anti-tumor response. The results of the scRNA-seq analysis showed that there was no significant quantitative difference in the T cells between the tissue and blood samples. Upreti et al. also reported that there was no quantitative difference in the proportion of T cell subsets derived from HNSCC- and healthy control-PBMC samples, but did note that there was a qualitative difference (23); PBMC-HNSCC T cells generate a significantly weaker IFN‐γ recall response under viral antigen stimulation, showing the systemic immunosuppressive condition of HNSCC patients (23). This suggests that the cell immune-state is crucial in the anti-tumor response.
These trNK cells play a significant role in the TME, especially in complex HNSCC. These trNK cells have diverse phenotypes shaped by the TME, characterized by a lack of migratory receptors and limited entry into blood circulation (24). These cells directly target tumor cells by secreting cytokines and chemokines, such as TNF-α and IFN-γ (25). The distribution of trNK cells within the TME is uneven, and their positioning at specific tissue sites like mucosal barriers may be crucial for defending against tumor cell invasion. In the TME of HNSCC, trNK cells exhibit unique phenotypes, including the expression of immune-suppressive molecules such as programmed death-ligand 1 (PD-L1), which may lead to the dysfunction of NK cells and is associated with the immune evasion of tumor cells. Thus, research on this trNK cell subset is warranted to ascertain its potential role in HNSCC treatment. In this study, the defined trNK-1 population exhibited high cancer hallmark activity, including angiogenesis, fatty-acid metabolism, glycolysis, mTORC1 signaling, and PI3K-Akt-mTOR signaling. In a hypoxic microenvironment, angiogenesis is essential for tumor growth, metastasis, and therapy resistance (26). New blood vessels not only supply nutrients and oxygen, they also engage in crosstalk with the TME, including tumor-derived cytokines IL-4, IL-10, and TGF-β, and facilitate immunosuppression (27). Enhanced glycolysis and fatty-acid metabolism are also hallmarks of cancer progression, and can provide sufficient energy supply and metabolic intermediates, such as dihydroxyacetone phosphate, nucleotides and lipids, supporting the rapid division and proliferation of cancer cells (28). The PI3K-Akt-mTOR signaling pathway is involved in tumor growth, proliferation, and survival, and is often overactive in malignancies (29). Conversely, the activity of this pathway in the trNK-0 was inhibited, which suggests an anti-tumor phenotype. Based on the phenotypes of immune cells, a batch of molecular signatures, incorporating diverse markers, have been considered effective classifiers in HNSCC. An enriched proinflammatory M1 macrophage signature exhibited enhanced anti-HPV infection, cytolytic activity, and high immune cell infiltration (30). Brooks et al. developed a hypoxia-immune prognostic transcriptional classifier (31). Further, immune cell subsets related to IFN-γ and a PD-L1 expression classifier were shown to be useful in predicting the prognosis of HPV-positive HNSCC patients (32).
The crosstalk analysis revealed that these trNK cells engaged in abundant interactions with other immune cells. ANXA1_FPR1, CCL3_CCR1, and CD47-SIRPα, are the classical axes of macrophage (type M2) recruitment and were highly expressed in the trNK cells. In the ANXA1_FPR1 axis, AnxA1 activated the FPRs via paracrine, autocrine, and juxtacrine ways to relieve inflammation. The CCL3 chemokine was observed to be highly expressed in carcinogenesis and to promote anti-inflammatory cell recruitment. CD47 was observed to be aberrantly expressed and to regulate the SIRPα in macrophages to promote tumor immune escape (33). These tumor-promoting properties are consistent with the properties of trNK-1 and taNK-0, which activated the cancer-related hallmark pathways. Conversely, we identified a specific trNK(-0) cell subtype that showed a markedly different immune-activation phenotype and had gene markers that were closely associated with prognosis and the immunotherapy response. Thus, trNK(-0) may be an effective diagnosis and treatment indicator in HNSCC in clinical practice.
This study has potential clinical implications for evaluating the prognosis of and selecting the optimal treatments for HPV-positive HNSCC patients; however, it also had a number of limitations. First, this retrospective study was based on public datasets, and the prognostic efficiency of the trNK(-0) needs to be validated in the future in clinical practice. Second, we assessed the potential immunotherapy responses of patients; however, spatial and time differences of the transcriptome might have led to deviations in the actual results. and scRNA-seq faces challenges such as data complexity and sensitivity limitations. Thus, future validation with a larger dataset and more clinical factors is required.
Conclusions
This study identified a specific trNK cell cluster that is closely associated with HPV-positive HNSCC progression. The results revealed the underlying association between this specific NK cell subtype and immunological TME changes in HPV-positive HNSCC patients. These observations could promote the development of immunotherapy-based interventions, which in turn could improve the prognosis of HPV-positive HNSCC patients. These findings could also increase understandings of the role and diversity of NK cells in HPV-positive HNSCC progression.
Acknowledgments
Funding: This work was supported by
Footnote
Reporting Checklist: The authors have completed the MDAR reporting checklist. Available at https://tcr.amegroups.com/article/view/10.21037/tcr-24-1535/rc
Peer Review File: Available at https://tcr.amegroups.com/article/view/10.21037/tcr-24-1535/prf
Conflicts of Interest: All authors have completed the ICMJE uniform disclosure form (available at https://tcr.amegroups.com/article/view/10.21037/tcr-24-1535/coif). The authors have no conflicts of interest to declare.
Ethical Statement: The authors are accountable for all aspects of the work in ensuring that questions related to the accuracy or integrity of any part of the work are appropriately investigated and resolved. The study was conducted in accordance with the Declaration of Helsinki (as revised in 2013).
Open Access Statement: This is an Open Access article distributed in accordance with the Creative Commons Attribution-NonCommercial-NoDerivs 4.0 International License (CC BY-NC-ND 4.0), which permits the non-commercial replication and distribution of the article with the strict proviso that no changes or edits are made and the original work is properly cited (including links to both the formal publication through the relevant DOI and the license). See: https://creativecommons.org/licenses/by-nc-nd/4.0/.
References
- Chow LQM. Head and Neck Cancer. N Engl J Med 2020;382:60-72. [Crossref] [PubMed]
- Lechner M, Liu J, Masterson L, et al. HPV-associated oropharyngeal cancer: epidemiology, molecular biology and clinical management. Nat Rev Clin Oncol 2022;19:306-27. [Crossref] [PubMed]
- Zhou C, Nan X. Application of indocyanine green in the management of oral cancer: a literature review. Oncologie 2023;25:605-17. [Crossref]
- Liberale C, Soloperto D, Marchioni A, et al. Updates on Larynx Cancer: Risk Factors and Oncogenesis. Int J Mol Sci 2023;24:12913. [Crossref] [PubMed]
- Westphaln KK, Imagawa KK, Smith LE, et al. Risk Factors for Abuse in Children with Congenital Heart Disease Presenting at a Pediatric Tertiary Care Hospital. Congenital Heart Disease 2024;18:657-70. [Crossref]
- Ruffin AT, Li H, Vujanovic L, et al. Improving head and neck cancer therapies by immunomodulation of the tumour microenvironment. Nat Rev Cancer 2023;23:173-88. [Crossref] [PubMed]
- Vivier E, Rebuffet L, Narni-Mancinelli E, et al. Natural killer cell therapies. Nature 2024;626:727-36. [Crossref] [PubMed]
- Kirchhammer N, Trefny MP, Natoli M, et al. NK cells with tissue-resident traits shape response to immunotherapy by inducing adaptive antitumor immunity. Sci Transl Med 2022;14:eabm9043. [Crossref] [PubMed]
- Zhou J, Tian Z, Peng H. Tissue-resident NK cells and other innate lymphoid cells. Adv Immunol 2020;145:37-53. [Crossref] [PubMed]
- Zhang M, Hu S, Min M, et al. Dissecting transcriptional heterogeneity in primary gastric adenocarcinoma by single cell RNA sequencing. Gut 2021;70:464-75. [Crossref] [PubMed]
- Roy S, Kar M, Roy S, et al. Inhibition of CD44 sensitizes cisplatin-resistance and affects Wnt/β-catenin signaling in HNSCC cells. Int J Biol Macromol 2020;149:501-12. [Crossref] [PubMed]
- Voigt FF, Kirschenbaum D, Platonova E, et al. The mesoSPIM initiative: open-source light-sheet microscopes for imaging cleared tissue. Nat Methods 2019;16:1105-8. [Crossref] [PubMed]
- Zulibiya A, Wen J, Yu H, et al. Single-Cell RNA Sequencing Reveals Potential for Endothelial-to-Mesenchymal Transition in Tetralogy of Fallot. Congenital Heart Disease 2024;18:611-25. [Crossref]
- Hao Y, Stuart T, Kowalski MH, et al. Dictionary learning for integrative, multimodal and scalable single-cell analysis. Nat Biotechnol 2024;42:293-304. [Crossref] [PubMed]
- Liberzon A, Birger C, Thorvaldsdóttir H, et al. The Molecular Signatures Database (MSigDB) hallmark gene set collection. Cell Syst 2015;1:417-25. [Crossref] [PubMed]
- Yu G, Wang LG, Han Y, et al. clusterProfiler: an R package for comparing biological themes among gene clusters. OMICS 2012;16:284-7. [Crossref] [PubMed]
- Hänzelmann S, Castelo R, Guinney J. GSVA: gene set variation analysis for microarray and RNA-seq data. BMC Bioinformatics 2013;14:7. [Crossref] [PubMed]
- Liu TT, Li R, Huo C, et al. Identification of CDK2-Related Immune Forecast Model and ceRNA in Lung Adenocarcinoma, a Pan-Cancer Analysis. Front Cell Dev Biol 2021;9:682002. [Crossref] [PubMed]
- Lee YG, Kang EJ, Keam B, et al. Treatment strategy and outcomes in locally advanced head and neck squamous cell carcinoma: a nationwide retrospective cohort study (KCSG HN13-01). BMC Cancer 2020;20:813. [Crossref] [PubMed]
- Kitamura N, Sento S, Yoshizawa Y, et al. Current Trends and Future Prospects of Molecular Targeted Therapy in Head and Neck Squamous Cell Carcinoma. Int J Mol Sci 2020;22:240. [Crossref] [PubMed]
- Sojka DK, Yang L, Yokoyama WM. Uterine Natural Killer Cells. Front Immunol 2019;10:960. [Crossref] [PubMed]
- Spits H, Artis D, Colonna M, et al. Innate lymphoid cells--a proposal for uniform nomenclature. Nat Rev Immunol 2013;13:145-9. [Crossref] [PubMed]
- Upreti D, Zhang ML, Bykova E, et al. Change in CD3ζ-chain expression is an independent predictor of disease status in head and neck cancer patients. Int J Cancer 2016;139:122-9. [Crossref] [PubMed]
- Peng H, Tian Z. Diversity of tissue-resident NK cells. Semin Immunol 2017;31:3-10. [Crossref] [PubMed]
- Freud AG, Mundy-Bosse BL, Yu J, et al. The Broad Spectrum of Human Natural Killer Cell Diversity. Immunity 2017;47:820-33. [Crossref] [PubMed]
- Schito L. Hypoxia-Dependent Angiogenesis and Lymphangiogenesis in Cancer. Adv Exp Med Biol 2019;1136:71-85. [Crossref] [PubMed]
- Rahma OE, Hodi FS. The Intersection between Tumor Angiogenesis and Immune Suppression. Clin Cancer Res 2019;25:5449-57. [Crossref] [PubMed]
- Fukushi A, Kim HD, Chang YC, et al. Revisited Metabolic Control and Reprogramming Cancers by Means of the Warburg Effect in Tumor Cells. Int J Mol Sci 2022;23:10037. [Crossref] [PubMed]
- Tian T, Li X, Zhang J. mTOR Signaling in Cancer and mTOR Inhibitors in Solid Tumor Targeting Therapy. Int J Mol Sci 2019;20:755. [Crossref] [PubMed]
- Chen YP, Wang YQ, Lv JW, et al. Identification and validation of novel microenvironment-based immune molecular subgroups of head and neck squamous cell carcinoma: implications for immunotherapy. Ann Oncol 2019;30:68-75. [Crossref] [PubMed]
- Brooks JM, Menezes AN, Ibrahim M, et al. Development and Validation of a Combined Hypoxia and Immune Prognostic Classifier for Head and Neck Cancer. Clin Cancer Res 2019;25:5315-28. [Crossref] [PubMed]
- Feng B, Shen Y, Pastor Hostench X, et al. Integrative Analysis of Multi-omics Data Identified EGFR and PTGS2 as Key Nodes in a Gene Regulatory Network Related to Immune Phenotypes in Head and Neck Cancer. Clin Cancer Res 2020;26:3616-28. [Crossref] [PubMed]
- Sugimura-Nagata A, Koshino A, Inoue S, et al. Expression and Prognostic Significance of CD47-SIRPA Macrophage Checkpoint Molecules in Colorectal Cancer. Int J Mol Sci 2021;22:2690. [Crossref] [PubMed]
(English Language Editor: L. Huleatt)