Integrative multi-omics and machine learning approach reveals tumor microenvironment-associated prognostic biomarkers in ovarian cancer
Highlight box
Key findings
• An integrative multi-omics and machine learning study of the ovarian cancer tumor microenvironment (TME) identifies a prognostic model using 700 TME-related genes, with a focus on immune cell dynamics.
What is known and what is new?
• The TME’s complexity and its role in ovarian cancer progression and treatment response, particularly the impact of immune cells, are well-acknowledged.
• This manuscript reveals the specific roles of exhausted CD8+ T cells and macrophages/monocytes in high-risk ovarian cancer. It introduces a prognostic model integrating TME genes with immune cell data, enhancing prediction accuracy.
What is the implication, and what should change now?
• The study underscores the need for personalized medicine in ovarian cancer, recommending treatment strategies that target the TME’s immune component. It advocates reinvigorating exhausted T cells. These insights are crucial for clinicians and researchers to refine therapeutic approaches and outcomes.
Introduction
Ovarian cancer (OC) represents a significant challenge in women’s health globally, characterized by its high incidence and mortality rates (1). This cancer type is particularly prevalent in high-income countries, ranking as the eighth most common cancer among women (2). However, its impact is even more pronounced in lower-income countries, where it is the third most severe form of cancer in women, following breast and cervical cancers (3). The disparities in its prevalence and severity can be linked to various factors, including lifestyle, genetic predispositions, differences in diagnostic capabilities, and access to medical resources (4). Notably, in populous nations like China, the incidence of OC has been increasing, now surpassing uterine cancer as the second leading cause of death from gynecological malignancies (5). This alarming trend underscores the urgent need for enhanced prevention, screening, and treatment strategies for OC (6).
Currently, the standard treatment for OC involves surgical intervention combined with platinum-based paclitaxel chemotherapy (7,8). However, this approach faces considerable challenges, notably high recurrence rates. About 75% of patients treated experience disease recurrence, often developing resistance to platinum drugs (9). This situation highlights the limitations of current therapies and the pressing need for more effective treatment strategies (10).
The recent shift in focus to the molecular biology of OC has brought the tumor microenvironment (TME) into the spotlight (11,12). The TME in OC comprises a complex network of tumor cells, immune cells, the extracellular matrix, blood vessels, lymphatic vessels, and other cellular components. Its role in tumor development, progression, metastasis, and response to treatment is increasingly recognized as pivotal (13,14). The composition and functional state of immune cells within the TME, in particular, have been shown to correlate closely with the prognosis of OC patients (15,16). For instance, the presence of certain types of immune cell infiltrates, such as activated T cells, may be associated with better survival rates, while others, like immunosuppressive cells, are linked with poorer outcomes (17-19).
Given the lack of effective early screening and prevention methods for OC, most patients are diagnosed at advanced stages (20,21). This complicates treatment and reduces the chances of successful outcomes. Therefore, a thorough study of the TME is significant for developing new therapeutic strategies. For example, targeted therapies against specific immune cell subgroups or immunosuppressive pathways in the TME could offer new opportunities to improve the effectiveness of existing treatments and reduce drug resistance.
In this study, we aimed to deepen our understanding of OC’s molecular biology and the dynamic changes within the TME. To achieve this, we conducted extensive analyses of single-cell RNA sequencing (scRNA-seq) on both normal and OC samples. Our approach involved the annotation and identification of 700 genes related to the TME and a detailed examination of immune infiltration at the single-cell level. We paid particular attention to analyzing T cell subgroups, combining differential gene expression (DEG) analysis and Gene Ontology (GO) analysis, to explore their potential roles in OC development and progression.
Simultaneously, we leveraged bulk RNA-seq data from The Cancer Genome Atlas (TCGA) OC cohort to establish a prognostic model based on TME characteristic genes. This model was optimized for predictive performance using the least absolute shrinkage and selection operator (LASSO) algorithm and was validated using another dataset (GSE140082, containing 380 OC samples). Our analyses also extended to drug sensitivity within the TCGA-OC cohort, aiming to provide scientific support for personalized treatment of OC.
The ultimate goal of this research is to reveal the associations between the TME and OC prognosis and immune status by analyzing molecular characteristics at both single-cell and bulk transcriptomic levels. This includes evaluating different risk subgroups’ responses to immune checkpoint inhibitors (ICIs) treatment, as well as their sensitivity to first-line drugs for OC, including tyrosine kinase inhibitors and mechanistic target of rapamycin (mTOR) inhibitors. Through the lens of predictive, preventive, and personalized medicine (PPPM), we hope to guide personalized prognosis prediction and treatment, thereby enhancing the survival rate and quality of life for OC patients (22). This research design and methodology are outlined in detail in Figure 1, providing a roadmap for our investigative approach. We present this article in accordance with the REMARK reporting checklist (available at https://tcr.amegroups.com/article/view/10.21037/tcr-24-539/rc).
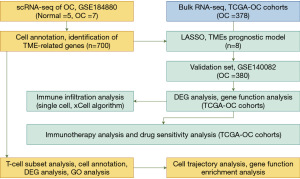
Methods
Data collection and quality control
OC scRNA-seq data were acquired from the Gene Expression Omnibus (GEO) database, accession number GSE184880. After downloading, preliminary quality control was conducted in the R environment (version 4.2.1) (23,24). All variables were cleared (rm(list = ls())), and automatic conversion of strings to factor types was disabled (options(stringsAsFactors = FALSE)). Quality control parameters included excluding cells with mitochondrial gene expression over 10% and removing cells with abnormal total gene expression, ensuring the quality of single-cell data for downstream analysis.
Data normalization and variance feature identification
Data normalization and scaling were conducted using the Seurat package. Normalized expression metrics were obtained via the NormalizeData function, followed by identification of highly variable genes using FindVariableFeatures. The ScaleData function was then applied for standardizing gene expression data, ensuring comparability across cells (25).
Dimensionality reduction and cluster analysis
Data dimensionality was reduced through principal component analysis (PCA) to reveal inherent data structures. The optimal number of principal components was determined using the ElbowPlot function. Batch effects were integrated using the Harmony algorithm, followed by dimensionality reduction via t-distributed stochastic neighbor embedding (t-SNE) and Uniform Manifold Approximation and Projection (UMAP) algorithms (RunTSNE and RunUMAP functions) for visualization (26).
Cell clustering and phenotypic annotation
Cells were clustered based on expression profiles using FindNeighbors and FindClusters functions, with results displayed using the DimPlot function. Clusters were annotated based on known marker gene expression patterns, visualized through FeaturePlot and DotPlot.
Statistical analysis and data integration
Using the Seurat package, individual scRNA-seq datasets were merged using the merge function, ensuring consistency in cell annotation across batches. This integrated dataset was used for all subsequent analyses (27).
Single-sample gene set enrichment analysis (ssGSEA)
T cells were extracted from the overall scRNA-seq dataset based on specific cell type annotations. The expression matrix for these T cells was converted to a format compatible with the gene set variation analysis (GSVA) package (28). ssGSEA was then conducted to compute exhaustion scores for T cells in OC and normal samples using predefined gene sets. The results were integrated with metadata and visualized using ggplot2. Statistical comparisons between T cells in OC and normal samples were performed using the Wilcoxon rank-sum test to identify significant differences in functional pathways.
Construction of TME-related genes prognostic signature
OC patients from TCGA were randomly divided into training and testing sets at an 8:2 ratio. To identify key genes influencing OC patient prognosis, we utilized the LASSO algorithm based on 700 TME-related genes in the training set. Subsequently, using stepwise multivariate COX regression analysis, we constructed a prognostic model for OC patients based on the key genes identified by LASSO. Using the prognostic model based on TME-related genes, we calculated the risk scores of OC patients in the training, testing, and validation sets, and distinguished high- and low-risk OC patients based on the median value. The Kaplan-Meier (KM) survival curve was plotted using the “survival” R package. The receiver operating characteristic (ROC) curve was plotted using the “timeROC” R package (29).
Drug sensitivity prediction
Chemotherapy drug sensitivity was predicted using the pRRopheticPredict function of the pRRophetic package. Half-maximal inhibitory concentration (IC50) values were calculated for each sample and correlated with survival risk scores derived from the machine learning model (30).
Tumor immune dysfunction and exclusion (TIDE) analysis
To evaluate the potential responsiveness of OC patients to immunotherapy, we conducted a TIDE analysis. The TIDE algorithm is designed to predict immune checkpoint blockade (ICB) response based on the expression signatures of T cell dysfunction and exclusion. The analysis helps in identifying tumors that are likely to respond to immunotherapy by assessing the dysfunction of T cells in tumors with high cytotoxic T lymphocyte (CTL) infiltration and the exclusion of T cells from tumors with low CTL infiltration. The TIDE scores were calculated for each sample using the TIDE R package. Higher TIDE scores indicate a higher likelihood of immune evasion and poorer response to ICB therapy. This analysis allows us to stratify patients based on their potential response to immunotherapy, providing valuable insights into the immune landscape of OC (31).
Pseudo-time trajectory analysis
Single-cell developmental trajectories were constructed using the monocle package, performing pseudo-temporal ordering of cells. DEG analysis identified genes with significant changes along trajectories, providing insights into dynamic gene expression changes during cell differentiation (32).
Visualization and data presentation
All data visualizations were generated using ggplot2 and ComplexHeatmap packages. Survival curves, heatmaps, and feature plots were produced to intuitively display relationships between gene expression patterns, drug sensitivity, and cell phenotypic annotation (33).
Data availability
All processed and analyzed data, along with corresponding R code, will be made publicly available in a permanent repository, adhering to scientific standards of transparency and reproducibility.
Software and reproducibility
All analyses were performed in R (version 4.2.1), with script version control managed through Git (version 2.39.2). To ensure reproducibility, detailed codes, including random seeds settings for machine learning algorithms.
Ethical compliance
All patient-derived data were processed in accordance with ethical guidelines and regulations for research using human samples, with appropriate de-identification measures taken to ensure privacy. The study was conducted in accordance with the Declaration of Helsinki (as revised in 2013).
Results
scRNA-seq of OC: extraction of TME genes and comparative ssGSEA analysis of T cells
Our workflow is illustrated in Figure 1. From the GEO dataset GSE184880, gene expression data were obtained for 64,659 cells across seven OC samples. To ensure the data’s quality, we implemented stringent quality control standards: cells were selected based on their RNA features, with a threshold set at nFeature_RNA >500 and nFeature_RNA <5,000, and the proportion of mitochondrial genes was required to be less than 15%. Post filtering and quality control, 39,337 cells expressing 700 TME-related genes were analyzed. Quality control involved dual-threshold filtering based on gene expression counts and the percentage of mitochondrial and ribosomal genes.
Clustering was performed using the k-nearest neighbors (KNN) algorithm, followed by nonlinear dimensionality reduction (t-SNE), resulting in cell distribution maps for OC and normal tissues (Figure 2A). Utilizing the FindMarkers command, differential expression genes distinguishing each cell type were identified, serving as biological markers for those cell types (Figure 2B). After annotating cell clusters with cell markers from the CellMakers website, we identified 8 cell subclusters (Figure 2C). The visualization of each subcluster’s proportions in normal and OC samples was conducted using the ggplot2 package (Figure 2D), revealing a higher proportion of T cells in OC patients and a greater prevalence of fibroblasts in normal tissues. Additionally, the gene expression profiles and key pathways specific to each cell subgroup within OC samples were highlighted, including seven key TME markers (Figure 2E), providing deeper insights into the molecular mechanisms driving OC progression.
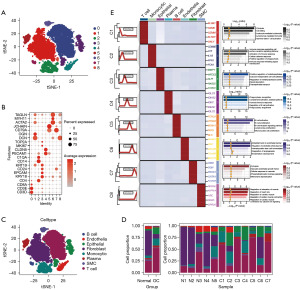
Given the significant role of T cells observed in Figure 2, we further analyzed their functional states within the OC microenvironment. We performed ssGSEA on the gene expression profiles of normal T cells and OC tumor (OCT) cells. These cells were extracted from the overall dataset and processed to ensure compatibility with the GSVA package. ssGSEA scores were calculated for predefined gene sets related to various functional pathways. Differential analysis revealed significant disparities (Wilcoxon rank-sum test, P<0.001), with OCT cells showing reduced activation/effector functions and T cell receptor (TCR) signaling activity, but elevated exhaustion markers and stress response signatures. These findings suggest a compromised immune response in the OC microenvironment, potentially facilitating tumor immune evasion. Visualization using ggplot2 highlighted these significant differences in gene set scores between the two cell types (Figure 3).
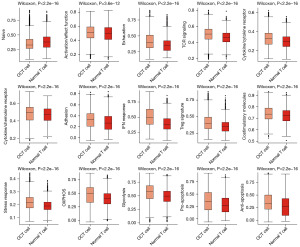
Our analysis, code, and visualization methods adhere to robust scientific standards, enhancing our understanding of the OC TME. We anticipate that these findings will contribute significantly to targeted therapy and personalized treatment strategies in OC.
Development of a prognostic model based on key TME genes
To explore the prognostic value of key genes in OC, we combined LASSO regression with machine learning algorithms to construct a prognostic model based on TME genes (Figure 4A,4B). The criteria for dividing patients into high- and low-risk groups were established based on the LASSO algorithm, which identified eight key genes (as shown in Figure 4C). A multivariate Cox regression model was constructed, and each patient’s risk score was calculated according to the model. Patients were then stratified into high or low-risk groups based on the median value of the risk scores.
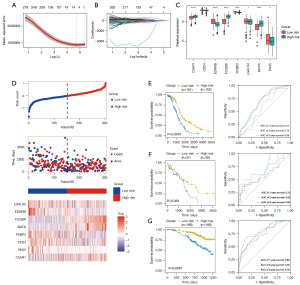
Each sample’s risk score was calculated using z-score normalization, dividing patients into high-risk and low-risk groups. The grouping was determined based on the optimum cutoff value derived from the risk scores. The heatmap illustrates feature genes that are highly expressed in both high-risk and low-risk groups. The low-risk group exhibited higher expression of genes such as EDNRB, FKBP2, and LGALS2, while the high-risk group showed higher expression of FCGBP, MATK, and C5AR1 (Figure 4C,4D). Figure 4C highlights statistically significant differentially expressed genes between the two risk groups within the model.
To further validate the performance of the prognostic risk model, risk scores were calculated for each patient in the TCGA-OC cohort. Patients were then classified into high-risk and low-risk groups based on the optimal risk score cutoff, with a comparison of their survival. KM survival curves indicated significant differences in survival between the two risk groups (P<0.001). Time-dependent ROC curves were plotted to assess the accuracy of the prognostic risk model, using the area under the curve (AUC) metric (Figure 4E). Patients in the low-risk group exhibited markedly better survival than those in the high-risk group. The prognostic model demonstrated an AUC of 0.57 at 1 year, 0.70 at 2 years, and 0.69 at 3 years. Both internal and external validations of the model yielded results consistent with those from the training set, showing significantly better survival for the low-risk group in the validation cohort (P=0.03, P<0.001) (Figure 4F,4G).
Differential analysis and enrichment analysis between high-risk and low-risk groups
PCA was conducted on high- and low-risk groups, revealing distinct gene expression profiles between the two groups (Figure 5A). DEG analysis identified 623 differentially expressed genes between the high- and low-risk samples (Figure 5B,5C), with 401 genes significantly upregulated and 222 genes significantly downregulated. Among these genes, examples include FCGBP, ADCY7, CX3CR1, and WDR81, which were significantly upregulated in the high-risk group, while genes like EDNRB, PET100, HIST1H3F, and BEX5 were significantly downregulated.
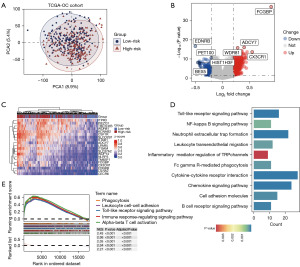
To elucidate the role of these genes in the pathogenesis and progression of OC, we performed enrichment analysis on the identified differentially expressed genes. Kyoto Encyclopedia of Genes and Genomes (KEGG) enrichment analysis revealed that these genes are primarily associated with several pathways, including the inflammatory mediator regulation of transient receptor potential (TRP) channels (Figure 5D). Gene set enrichment analysis (GSEA) enrichment analysis identified significant enrichment peaks for the differentially expressed genes in pathways such as phagocytosis, leukocyte cell-cell adhesion, toll-like receptor signaling pathway, immune response-regulating signaling pathway, and alpha-beta T cell activation, all of which were statistically significant (P<0.001) (Figure 5E). The enrichment analysis results suggest a significant correlation between OC and changes in immune function.
Immune infiltration analysis
To further investigate the immune landscape of OC, we conducted an immune infiltration analysis. We began by mapping the expression of core risk genes across various cell subpopulations. As depicted in Figure 6A, LGALS2 and C5AR1 predominantly expressed within monocytic cells, while MATK was primarily expressed in T cells. The box plots outline the cellular infiltration in the OC microenvironment; as shown in Figure 6B, the expression levels of macrophages, mast cells, and monocytes are significantly higher in the high-risk group compared to the low-risk group. Conversely, plasma cells and Th2 cells show significantly lower expression levels in the high-risk group, with these differences being statistically significant.
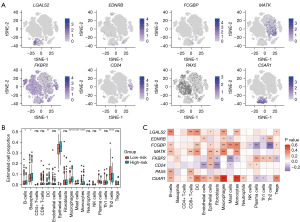
Building on this, we analyzed the correlation between core risk genes and the cellular infiltration profile. The results, as illustrated in Figure 6C, demonstrate significant correlations between core risk genes and the expression of various cells. Notably, C5AR1 exhibits a strong positive correlation with macrophages and monocytes, while FCGBP shows a strong negative correlation with Th2 cells.
Analysis of immune therapy responsiveness and drug sensitivity prediction between high-risk and low-risk groups
To investigate the disparities in immune therapy response between high-risk and low-risk groups, we first performed a TIDE analysis. The results, as depicted in Figure 7A, indicated that the TIDE scores were significantly higher in the high-risk group (P<0.001), suggesting a lower response to immune therapy. Correlation analysis (Figure 7B) revealed a positive relationship between TIDE scores and risk scores (R=0.33, P<0.001).
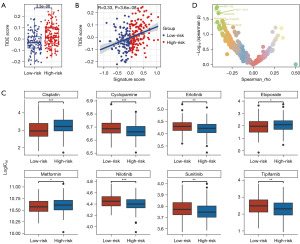
Subsequently, we assessed the relationship between risk scores and IC50 values of eight Food and Drug Administration (FDA)-approved chemotherapeutic and immune drugs. As shown in Figure 7C, compared to the low-risk group, the high-risk group was more sensitive to cisplatin, etoposide, and metformin, while demonstrating reduced sensitivity to cyclopamine, erlotinib, nilotinib, sunitinib, and tipifarnib, with each difference holding statistical significance.
To further explore potential therapeutic strategies for high-risk OC patients, we analyzed the correlation between drug response and risk scores using data from the Genomics of Drug Sensitivity in Cancer (GDSC) database. The selected drugs included the chemotherapeutic agents rapamycin, sorafenib, and MG-132, as well as the immunomodulatory agents ACY-1215, crizotinib, cediranib, VE821, and GSK2830371. Combining our statistical results with findings from other studies (34-39), we identified these drugs as potentially effective for OC treatment. As shown in Figure 7D, Spearman correlation analysis revealed significant positive correlations between the IC50 values of these drugs and the high-risk scores, suggesting that these drugs might be more effective in treating high-risk OC patients.
Analysis of T cell subgroups
To further investigate the role of T cells in the development of OC, we analyzed T cell subpopulations. After log-normalization, dimensionality reduction, and cell annotation, we identified five T cell subgroups: naive T, Treg, exhausted CD8, natural killer T (NKT), and cytotoxic CD8 (Figure 8A). Analysis of the proportions of each T cell subgroup in normal and OC samples (Figure 8B) revealed that the exhausted CD8 subset was more prevalent in OC samples, while cytotoxic CD8 cells were less common compared to normal samples.
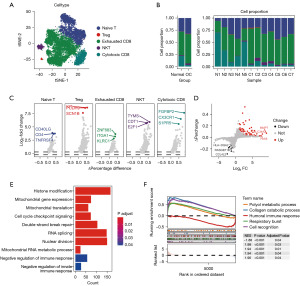
Figure 8C presents the marker genes highly expressed in each T cell subgroup. DEG analysis between normal and OC T cells (Figure 8D) showed an upregulation of 54 genes and a downregulation of nine genes in OC samples. Examples of upregulated genes include MMP7, SLC34A2, and PAX8, while genes like HLA-DQA2, RASGEF18, and CCL4L2 were among the downregulated. Further enrichment analysis of the differential genes (Figure 8E,8F) indicated that these genes are predominantly enriched in pathways such as histone modification, RNA splicing, nuclear division, and double-strand break repair. High enrichment levels were also seen in pathways such as collagen catabolic process and cell recognition, whereas the association with the humoral immune response was comparatively weak.
Pseudotime analysis of T cells
To delineate the developmental trajectory of T cell subpopulations in OC, we conducted pseudotime analyses across various T cell subgroups. As illustrated in Figure 9A-9C, naive T cells predominantly represent the initial stage of T cell development. These cells progressively differentiate into subgroups, mainly composed of NKT cells, with exhausted CD8 T cells emerging at later stages of development. The trajectory culminates in a diversified landscape characterized by a mix of NKT cells, exhausted CD8 T cells, and naive T cells. Throughout this developmental continuum, MATK consistently exhibited high levels of expression. In Figure 9A-9C, the significance of numbers 1, 2, 3, and 4 represents the key branching points in cell differentiation. These numbers help illustrate the progression through different stages of T cell differentiation: (I) initial differentiation stage predominantly consisting of naive T cells; (II) intermediate stage with increasing presence of Treg and NKT cells; (III) advanced differentiation stage with a mix of exhausted CD8 and cytotoxic CD8 cells; and (IV) final stage showing a diversified landscape with all subgroups.
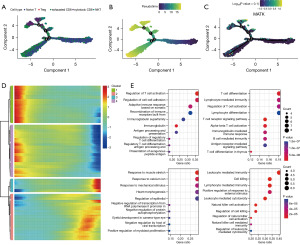
Four gene modules associated with subgroup differentiation were extracted along the T cell evolutionary trajectory. Differential expression analysis of these modules (Figure 9D) revealed distinct expression patterns. Module 1 genes were primarily enriched in pathways related to the regulation of T cell activation and cell-cell adhesion. Module 2 genes were predominantly involved in T cell differentiation and lymphocyte-mediated immunity. Module 3 genes were enriched in pathways responsive to muscle stretch and calcium ion, while Module 4 genes were focused on leukocyte-mediated immunity. The modules in Figure 9E correspond to the clustering results in Figure 9D, identifying the GO terms significantly enriched in each cluster.
Discussion
In our study, we have meticulously charted the landscape of the TME in OC through the lens of scRNA-seq, a vantage point that has allowed us to uncover the complexity and dynamism of this crucial biological niche. By focusing on the TME, our research aligns with the burgeoning interest in understanding how the microenvironment influences cancer progression, metastasis, and response to treatment. The intricacies of the TME, composed of a diverse array of cell types, including immune cells, stromal cells, and cancer cells, play pivotal roles in the tumor’s evolution and its interaction with the host’s immune system.
The development of a prognostic model based on TME characteristics stands as a significant advancement in the field of OC research (12,40). The ability of this model to stratify patients into distinct risk categories based on molecular profiles paves the way for more personalized and targeted therapeutic strategies. This approach is especially critical in OC, where the heterogeneity of the disease often leads to varied responses to standard treatments and prognoses. The predictive prowess of our model, as indicated by KM survival analyses and the area under the curve (AUC) metrics from ROC analyses, demonstrates its potential utility as a clinical tool. Such tools are indispensable in the era of precision medicine, where treatment decisions are increasingly informed by detailed molecular and genetic information (41,42).
One of the most striking findings of our study is the increased prevalence of T cells in the OC TME. This observation is particularly intriguing as it suggests an active immune response against the tumor, yet this response seems insufficient to halt disease progression in many cases. The role of T cells in cancer has been a focus of intense research, especially in the context of immunotherapies that seek to harness the body’s immune system to fight cancer (43). The presence of these cells in the TME of OC patients could have significant implications for the development of such therapies.
Additionally, our study has cast light on the roles of macrophages and monocytes in high-risk OC patients. These cells are known to play dual roles in cancer: they can attack tumor cells, but they can also support tumor growth and metastasis. The association of elevated levels of these cells with high-risk patients suggests their potential role in tumor progression and immune evasion, a finding that could be leveraged to develop novel therapeutic strategies aimed at modulating the immune component of the TME.
Our research also underscores the importance of T cell subgroups, particularly exhausted CD8+ T cells, in OC. The prevalence of these cells in high-risk patient samples is a critical discovery, as these cells are often associated with weakened immune responses in cancer. Understanding the mechanisms that lead to T cell exhaustion in the OC TME could open new therapeutic avenues, such as treatments designed to reinvigorate these exhausted T cells. Known studies have demonstrated that high programmed cell death 1 (PD-1) expression in OC correlates with poor clinical outcomes and resistance to conventional therapies (44-47). Additionally, Lag3, another inhibitory receptor expressed on exhausted CD8 T cells, has been linked to advanced disease stages and poor prognosis in OC patient (48). Targeting these immune checkpoint pathways may offer novel therapeutic opportunities for patients with OC, especially those exhibiting significant CD8 T cell exhaustion.
The differential drug sensitivities we observed among patient groups underscore the need for personalized treatment approaches in OC. The development of tailored therapeutic regimens, informed by comprehensive genomic profiling, could significantly improve treatment efficacy and patient outcomes. This personalized approach is particularly crucial in managing a heterogeneous disease like OC, where a one-size-fits-all treatment strategy is often inadequate. In our study, we identified several non-frontline drugs that show significant sensitivity in high-risk OC patients. By leveraging the GDSC database, we found that certain novel drugs could potentially serve as effective second-line treatments for refractory OC. These findings are promising as they provide new therapeutic options for patients who do not respond well to standard frontline treatments. We did not focus on frontline drugs such as carboplatin, paclitaxel, bevacizumab, and poly(ADP-ribose) polymerase (PARP) inhibitors in detail because these drugs are already established as effective in both high-risk and low-risk groups, with no significant statistical differences between these groups. Existing literature consistently demonstrates that these drugs significantly improve progression-free survival (PFS) and overall survival (OS) across different risk categories (49-51). This evidence supports the reliability of frontline drugs and explains our emphasis on exploring new potential therapies for situations where first-line treatments are limited or fail.
Our results indicate a higher presence of Th2 cells in the low-risk population. This observation is particularly interesting given recent studies that have shown a correlation between Th2 cell infiltration in high-grade serous OC (HGSOC) and poor prognosis in patients treated with chemotherapy (52,53). Th2 cells are known to be involved in humoral immunity and can contribute to an immunosuppressive TME, which may affect the efficacy of chemotherapy. The increased presence of Th2 cells in the low-risk group in our study suggests that these patients might have a different immune landscape compared to the high-risk group. Further investigation into the role of Th2 cells in OC, particularly in the context of treatment response and patient outcomes, is warranted. Understanding these dynamics could lead to improved stratification of patients and more personalized therapeutic strategies.
One important aspect of personalized treatment in OC is the consideration of genetic mutations, particularly in the BRCA1 and BRCA2 genes. Given the therapeutic importance of BRCA mutations in DNA repair mechanisms, it is well known that BRCA alterations are frequently observed in OC patients. These alterations have significant therapeutic implications as patients with BRCA mutations are eligible for PARP inhibitor therapy (54). Considering this, we examined the BRCA status of the patients included in our study. A substantial proportion of our patient cohort exhibited BRCA mutations, consistent with previous reports in OC literature. This finding underscores the potential benefit of PARP inhibitor therapy for this subset of patients. Incorporating BRCA status into our analysis further enhances the clinical relevance of our study, highlighting the need for personalized treatment strategies based on genetic profiling. Future research should focus on integrating comprehensive BRCA mutation screening into routine clinical practice for OC patients. This would facilitate the identification of patients who could benefit from PARP inhibitors and improve overall treatment outcomes through personalized medicine approaches.
Despite these significant findings, there are limitations in this study that must be acknowledged. The insights gleaned from our single-cell sequencing data, derived from a relatively small and specific cohort of HGSOC patients, may not be broadly applicable across all subtypes of OC. Future studies involving larger, more diverse patient populations are needed to validate and generalize our findings. Additionally, the risk genes identified in our prognostic model warrant further exploration to understand their biological functions and interactions within the TME.
Looking ahead, the field of OC research stands at a promising juncture. As we continue to unravel the complexities of the TME and its myriad interactions with cancer cells and the host immune system, new therapeutic targets and strategies are likely to emerge. The integration of advanced genomic and transcriptomic technologies with clinical data will be crucial in this endeavor, allowing for a more nuanced understanding of this disease and paving the way for more effective and personalized treatments.
In conclusion, our study adds a vital chapter to the growing body of knowledge on the TME in OC. By illuminating the cellular and molecular dynamics within the TME, we not only enhance our understanding of this complex disease but also open the door to novel therapeutic possibilities. As we move forward, the continued exploration of the TME, its constituents, and their interactions will be key to developing more effective strategies to combat OC, ultimately improving the prognosis and quality of life for patients suffering from this challenging disease.
Conclusions
Our study represents a significant advancement in the understanding of OC by employing an integrative multi-omics and machine learning approach to decode the complexities of the TME. The unique insights garnered into the molecular dynamics of the OC TME have underscored the pivotal role of TME-associated biomarkers in refining patient prognosis and guiding therapeutic strategies. Notably, the development of a robust prognostic model based on key TME genes, validated against independent datasets, provides a powerful tool for stratifying patients into risk-specific groups. This stratification facilitates personalized treatment plans, potentially enhancing survival rates and quality of life for OC patients.
Our findings also shed light on the differential immune cell infiltration patterns within the OC TME, emphasizing the dualistic nature of immune responses in this setting. The prevalence of exhausted CD8+ T cells in high-risk patients highlights the need for innovative therapeutic approaches that might reinvigorate these compromised immune cells. Furthermore, the observed variability in drug sensitivity among patient subgroups underlines the necessity of personalized medicine in OC treatment.
While our study offers groundbreaking insights into OC’s molecular landscape and opens new avenues for targeted therapy, it is imperative to acknowledge its limitations, including the scope of the patient cohort and the generalizability of the results. Future research should aim to validate these findings across larger and more diverse populations and explore the biological functions of the identified risk genes in greater depth.
In conclusion, this research marks a critical step forward in the pursuit of precision medicine in OC treatment. By elucidating the intricate relationship between the TME and cancer progression, our study not only enhances the understanding of OC’s biological complexity but also paves the way for the development of more effective, personalized therapeutic interventions.
Acknowledgments
Funding: None.
Footnote
Reporting Checklist: The authors have completed the REMARK reporting checklist. Available at https://tcr.amegroups.com/article/view/10.21037/tcr-24-539/rc
Peer Review File: Available at https://tcr.amegroups.com/article/view/10.21037/tcr-24-539/prf
Conflicts of Interest: All authors have completed the ICMJE uniform disclosure form (available at https://tcr.amegroups.com/article/view/10.21037/tcr-24-539/coif). The authors have no conflicts of interest to declare.
Ethical Statement: The authors are accountable for all aspects of the work in ensuring that questions related to the accuracy or integrity of any part of the work are appropriately investigated and resolved. The study was conducted in accordance with the Declaration of Helsinki (as revised in 2013).
Open Access Statement: This is an Open Access article distributed in accordance with the Creative Commons Attribution-NonCommercial-NoDerivs 4.0 International License (CC BY-NC-ND 4.0), which permits the non-commercial replication and distribution of the article with the strict proviso that no changes or edits are made and the original work is properly cited (including links to both the formal publication through the relevant DOI and the license). See: https://creativecommons.org/licenses/by-nc-nd/4.0/.
References
- Webb PM, Jordan SJ. Global epidemiology of epithelial ovarian cancer. Nat Rev Clin Oncol 2024;21:389-400. [Crossref] [PubMed]
- Mazidimoradi A, Momenimovahed Z, Khani Y, et al. Global patterns and temporal trends in ovarian cancer morbidity, mortality, and burden from 1990 to 2019. Oncologie 2023;25:641-59. [Crossref]
- Zhang Y, Luo G, Li M, et al. Global patterns and trends in ovarian cancer incidence: age, period and birth cohort analysis. BMC Cancer 2019;19:984. [Crossref] [PubMed]
- Wang L, Ding Y, Zhang Y, et al. The association between neuropsychological impairment, self-perceived cognitive deficit, symptoms, and health related quality of life in newly diagnosed ovarian cancer patients. Asia Pac J Oncol Nurs 2024;11:100447. [Crossref] [PubMed]
- Sun K, Han B, Zeng H, et al. Incidence and Mortality of Cancers in Female Genital Organs - China, 2022. China CDC Wkly 2024;6:195-202. [Crossref] [PubMed]
- MacArthur E, Long Roche K, Sakran J, et al. Salpingectomy for ovarian cancer prevention: Video education for the surgeon. Gynecol Oncol Rep 2024;53:101368. [Crossref] [PubMed]
- Yang ST, Chang WH, Chou FW, et al. Front-line chemo-immunotherapy for treating epithelial ovarian cancer: Part I CA125 and anti-CA125. Taiwan J Obstet Gynecol 2023;62:802-8. [Crossref] [PubMed]
- Abdallah R, Atallah D, Bitar N, et al. Consensus on the management of platinum-sensitive high-grade serous epithelial ovarian cancer in Lebanon. Gynecol Oncol Rep 2023;47:101186. [Crossref] [PubMed]
- Romero D. Mirvetuximab soravtansine has activity in platinum-sensitive epithelial ovarian cancer. Nat Rev Clin Oncol 2024;21:402. [Crossref] [PubMed]
- Kumar S, Acharya S, Karthikeyan M, et al. Limitations and potential of immunotherapy in ovarian cancer. Front Immunol 2024;14:1292166. [Crossref] [PubMed]
- Lim JHC. High resolution analysis of the tumour microenvironment of high grade serous ovarian cancer (HGSOC) using single cell transcriptomics and quantitative histopathological examination. 2024 Mar 29. Available online: https://era.ed.ac.uk/handle/1842/41682
- Gao L, Wei Z, Ying F, et al. Glutamine metabolism prognostic index predicts tumour microenvironment characteristics and therapeutic efficacy in ovarian cancer. J Cell Mol Med 2024;28:e18198. [Crossref] [PubMed]
- Prayudi PKA, Winata IGS, Mahendra INB, et al. The Mechanism and Dynamic Regulation of Epithelial to Mesenchymal Transition in Ovarian Cancer. Clin Exp Obstet Gynecol 2023;50:272. [Crossref]
- Jazwinska DE, Kulawiec DG, Zervantonakis IK. Cancer-mesothelial and cancer-macrophage interactions in the ovarian cancer microenvironment. Am J Physiol Cell Physiol 2023;325:C721-30. [Crossref] [PubMed]
- Jin X, Chen Y, Hu Q. Relationships of SIGLEC family-related lncRNAs with clinical prognosis and tumor immune microenvironment in ovarian cancer. Sci Rep 2024;14:7593. [Crossref] [PubMed]
- Yang B, Dai M. High-dimensional deconstruction of ovarian cancer at single-cell precision reveals HEBP2 that reshape the TIME and drive carboplatin resistance. Transl Oncol 2024;44:101917. [Crossref] [PubMed]
- Magarifuchi N, Iwasaki T, Katayama Y, et al. Gene amplification of chromatin remodeling factor SMARCC2 and low protein expression of ACTL6A are unfavorable factors in ovarian high-grade serous carcinoma. Oncol Lett 2024;27:196. [Crossref] [PubMed]
- Fei Q, Zhang X, Wang S, et al. A pan-cancer characterization of immune-related NFIL3 identifies potential predictive biomarker. J Cancer 2024;15:1271-86. [Crossref] [PubMed]
- Bate T, Martin RM, Yarmolinsky J, et al. Investigating the association between genetically proxied circulating levels of immune checkpoint proteins and cancer survival: protocol for a Mendelian randomisation analysis. BMJ Open 2024;14:e075981. [Crossref] [PubMed]
- Boussios S, Samartzis EP, Pavlidis N, et al. Molecular Genetics and Emerging Therapies for Epithelial Ovarian Cancer: Basic Research and Clinical Perspectives. Frontiers Media SA; 2023:348.
- Watson R, Ulmer K, Gabrilovich S, et al. Prediction of ovarian cancer with deep machine-learning and alternative splicing (2167). Gynecologic Oncology 2023;176:S236. [Crossref]
- Tavares V, Marques IS. Paradigm Shift: A Comprehensive Review of Ovarian Cancer Management in an Era of Advancements. International Journal of Molecular Sciences 2024;25:1845. [Crossref] [PubMed]
- Xu J, Fang Y, Chen K, et al. Single-Cell RNA Sequencing Reveals the Tissue Architecture in Human High-Grade Serous Ovarian Cancer. Clin Cancer Res 2022;28:3590-602. [Crossref] [PubMed]
- Slovin S, Carissimo A, Panariello F, et al. Single-Cell RNA Sequencing Analysis: A Step-by-Step Overview. Methods Mol Biol 2021;2284:343-65. [Crossref] [PubMed]
- Stuart T, Butler A, Hoffman P, et al. Comprehensive Integration of Single-Cell Data. Cell 2019;177:1888-1902.e21. [Crossref] [PubMed]
- Korsunsky I, Millard N, Fan J, et al. Fast, sensitive and accurate integration of single-cell data with Harmony. Nat Methods 2019;16:1289-96. [Crossref] [PubMed]
- Tabula Muris Consortium. Overall coordination; Logistical coordination; Single-cell transcriptomics of 20 mouse organs creates a Tabula Muris. Nature 2018;562:367-72. [Crossref]
- Hänzelmann S, Castelo R, Guinney J. GSVA: gene set variation analysis for microarray and RNA-seq data. BMC Bioinformatics 2013;14:7. [Crossref] [PubMed]
- Bailey MH, Tokheim C, Porta-Pardo E, et al. Comprehensive Characterization of Cancer Driver Genes and Mutations. Cell 2018;173:371-385.e18. [Crossref] [PubMed]
- Barretina J, Caponigro G, Stransky N, et al. The Cancer Cell Line Encyclopedia enables predictive modelling of anticancer drug sensitivity. Nature 2012;483:603-7. [Crossref] [PubMed]
- Jiang P, Gu S, Pan D, et al. Signatures of T cell dysfunction and exclusion predict cancer immunotherapy response. Nat Med 2018;24:1550-8. [Crossref] [PubMed]
- Deng Q, Ramsköld D, Reinius B, et al. Single-cell RNA-seq reveals dynamic, random monoallelic gene expression in mammalian cells. Science 2014;343:193-6. [Crossref] [PubMed]
- Gu Z, Eils R, Schlesner M. Complex heatmaps reveal patterns and correlations in multidimensional genomic data. Bioinformatics 2016;32:2847-9. [Crossref] [PubMed]
- Ali ES, Mitra K, Akter S, et al. Recent advances and limitations of mTOR inhibitors in the treatment of cancer. Cancer Cell Int 2022;22:284. [Crossref] [PubMed]
- Devapatla B, Sharma A, Woo S. CXCR2 Inhibition Combined with Sorafenib Improved Antitumor and Antiangiogenic Response in Preclinical Models of Ovarian Cancer. PLoS One 2015;10:e0139237. [Crossref] [PubMed]
- Yoo J, Jeon YH, Lee DH, et al. HDAC6-selective inhibitors enhance anticancer effects of paclitaxel in ovarian cancer cells. Oncol Lett 2021;21:201. [Crossref] [PubMed]
- Santiago-O'Farrill JM, Blessing Bollu A, Yang H, et al. Crizotinib Enhances PARP Inhibitor Efficacy in Ovarian Cancer Cells and Xenograft Models by Inducing Autophagy. Mol Cancer Res 2024;22:840-51. [Crossref] [PubMed]
- Lee JM, Moore RG, Ghamande S, et al. Cediranib in Combination with Olaparib in Patients without a Germline BRCA1/2 Mutation and with Recurrent Platinum-Resistant Ovarian Cancer: Phase IIb CONCERTO Trial. Clin Cancer Res 2022;28:4186-93. [Crossref] [PubMed]
- Huntoon CJ, Flatten KS, Wahner Hendrickson AE, et al. ATR inhibition broadly sensitizes ovarian cancer cells to chemotherapy independent of BRCA status. Cancer Res 2013;73:3683-91. [Crossref] [PubMed]
- Sun R, Li S, Ye W, Lu Y. Development of a Prognostic Model Based on Lysosome-Related Genes for Ovarian Cancer: Insights into Tumor Microenvironment, Mutation Patterns, and Personalized Treatment Strategies [Internet]. 2024. Available online: https://www.researchsquare.com/article/rs-3865563/v1
- Yoshikawa N, Kubokawa M, Matsukawa T, et al. 304 Exploring mean platelet volume as a survival indicator in ovarian clear cell carcinoma: a retrospective analysis and random forest model assessment. International Journal of Gynecological Cancer 2024; [Crossref]
- Cui Y, Zhang W, Lu W, et al. An exosome-derived lncRNA signature identified by machine learning associated with prognosis and biomarkers for immunotherapy in ovarian cancer. Front Immunol 2024;15:1228235. [Crossref] [PubMed]
- Drakes M, Mehrotra S, Kertowidjojo E, et al. 1420 Understanding racial differences in the ovarian cancer tumor microenvironment. J Immunother Cancer [Internet]. 2023;11(Suppl 1). Available online: https://jitc.bmj.com/content/11/Suppl_1/A1579
- Hamanishi J, Mandai M, Ikeda T, et al. Safety and Antitumor Activity of Anti-PD-1 Antibody, Nivolumab, in Patients With Platinum-Resistant Ovarian Cancer. J Clin Oncol 2015;33:4015-22. [Crossref] [PubMed]
- Chardin L, Leary A. Immunotherapy in Ovarian Cancer: Thinking Beyond PD-1/PD-L1. Front Oncol 2021;11:795547. [Crossref] [PubMed]
- Zhang T, Zheng S, Liu Y, et al. DNA damage response and PD-1/PD-L1 pathway in ovarian cancer. DNA Repair (Amst) 2021;102:103112. [Crossref] [PubMed]
- Yin Z, Yu M, Ma T, et al. Mechanisms underlying low-clinical responses to PD-1/PD-L1 blocking antibodies in immunotherapy of cancer: a key role of exosomal PD-L1. J Immunother Cancer 2021;9:e001698. [Crossref] [PubMed]
- Huo JL, Wang YT, Fu WJ, et al. The promising immune checkpoint LAG-3 in cancer immunotherapy: from basic research to clinical application. Front Immunol 2022;13:956090. [Crossref] [PubMed]
- Hall M, Bertelli G, Li L, et al. Role of front-line bevacizumab in advanced ovarian cancer: the OSCAR study. Int J Gynecol Cancer 2020;30:213-20. [Crossref] [PubMed]
- Suh YJ, Lee B, Kim K, et al. Bevacizumab versus PARP-inhibitors in women with newly diagnosed ovarian cancer: a network meta-analysis. BMC Cancer 2022;22:346. [Crossref] [PubMed]
- Pujade-Lauraine E, Hilpert F, Weber B, et al. Bevacizumab combined with chemotherapy for platinum-resistant recurrent ovarian cancer: The AURELIA open-label randomized phase III trial. J Clin Oncol 2014;32:1302-8. [Crossref] [PubMed]
- Su H, Jin Y, Tao C, et al. Th2 cells infiltrating high-grade serous ovarian cancer: a feature that may account for the poor prognosis. J Gynecol Oncol 2023;34:e48. [Crossref] [PubMed]
- Cao W, Wang C, Zhang Y, et al. Identification of the prognostic value of LACTB2 and its correlation with immune infiltrates in ovarian cancer by integrated bioinformatics analyses. Eur J Med Res 2024;29:166. [Crossref] [PubMed]
- Lord CJ, Ashworth A. BRCAness revisited. Nat Rev Cancer 2016;16:110-20. [Crossref] [PubMed]