A lncRNA signature associated with endoplasmic reticulum stress supports prognostication and prediction of drug resistance in acute myelogenous leukemia
Highlight box
Key findings
• A prognostic signature was constructed using 227 endoplasmic reticulum (ER) stress-related long non-coding RNAs (lncRNAs) to develop a unique prognostic marker in acute myeloid leukemia (AML).
What is known and what is new?
• AML-specific lncRNAs have been identified and found to be aberrantly expressed in leukemic cells. They have been shown to contribute to leukemogenesis, AML progression, and response to treatment. However, the precise association between AML and ER stress-related lncRNAs remains unclear, calling for additional exploration.
• A unique prognostic marker linked to ER stress is developed, providing valuable perspectives on the correlation between lncRNAs associated with ER stress and the immune microenvironment and prognostic prediction of AML.
What is the implication, and what should change now?
• The signature not only serves as a valuable resource for deciphering the immune responses, somatic mutational landscape, drug resistance and potential biological functions in AML patients but also provides promising insights for developing accurate therapeutic strategies for them.
Introduction
Acute myeloid leukemia (AML) is a fast-developing hematological malignancy that is identified by the excessive growth of myeloid cells in the bone marrow (1). AML has a significant global incidence rate and carries a high mortality burden. The exact mechanisms leading to AML development are still being investigated. Advances in targeted therapies, such as inhibitors targeting specific genetic mutations, have shown promising results in improving outcomes for specific subsets of AML patients (2). The survival rate for AML over a period of 5 years is relatively poor, and it is subject to variation based on multiple factors such as the age of the patient, their overall health condition, the stage of the disease, and genetic traits (3-5). Hence, acquiring a thorough comprehension of the etiology and advancement mechanisms of AML is vital in order to offer innovative treatment strategies and enhance the outlook for individuals with AML.
Endoplasmic reticulum (ER) stress is a cellular response to perturbations in the ER, resulting in unfolded or misfolded protein accumulation within the cell (6). When the protein folding capacity of the ER is overwhelmed, ER stress is triggered, initiating a complex signaling network known as the unfolded protein response (UPR) (7). In recent years, emerging studies have shed light on the relationship between ER stress and cancer. ER stress dysregulation has been observed in multiple tumor types (8-10). Altered expression or activity of key molecules involved in the UPR pathway can contribute to tumor initiation, progression, and therapeutic resistance. In terms of the immune system, ER stress can influence immune cell function and modulate inflammatory responses (11). Xie et al. has reported increased ER stress markers and UPR activation in AML cells (12), highlighting the importance of further investigating the role of ER stress in AML pathogenesis and treatment.
Long non-coding RNAs (lncRNAs) are non-coding RNA molecules that play vital regulatory roles in numerous cellular processes, despite their inability to encode proteins (13). Their involvement in chemotherapy resistance and tumor immune evasion has established them as appealing targets for the development of innovative cancer therapies. AML-specific lncRNAs have been identified and found to be aberrantly expressed in leukemic cells (14). They have been shown to contribute to leukemogenesis, AML progression, and response to treatment (15). Hence, lncRNAs demonstrate immense potential as diagnostic markers and therapeutic targets for AML.
However, the precise association between AML and ER stress-related lncRNAs remains unclear, calling for additional exploration. This study aims to elucidate the relationship between ER stress-related lncRNAs and AML. Furthermore, it aims to examine the functional and biological pathways influenced by ER stress and study its effect on the immune microenvironment in AML. Moreover, the assessment is made regarding the potential of lncRNAs associated with ER stress as prognostic indicators for drug responsiveness and immune response in clinical settings. The findings of this research may offer innovative insights for the clinical prognosis and treatment of AML, providing novel ideas and approaches to enhance patient outcomes. We present this article in accordance with the TRIPOD reporting checklist (available at https://tcr.amegroups.com/article/view/10.21037/tcr-24-722/rc).
Methods
Dataset collection and preparation
This study was conducted in accordance with the Declaration of Helsinki (as revised in 2013). To obtain the necessary data, including RNA transcriptome sequence, mutation, and clinical data, we downloaded information from Genotype-Tissue Expression (GTEx) (https://xenabrowser.net/hub/) and The Cancer Genome Atlas (TCGA) database (https://portal.gdc.cancer.gov/). Subsequently, annotation files from the Ensembl database (https://asia.ensembl.org/) were utilized to distinguish the lncRNAs (16). A total of 488 samples (337 healthy samples, 151 AML samples) were enrolled for analysis. These samples are all from the bone marrow and contain both leukemia cells and immune cells.
For the identification of ER stress-related lncRNA prognostic signature, we utilized a curated list of 295 acknowledged ER stress-related genes from previous publications (17,18). These genes were subjected to analysis, and the results are available at: https://cdn.amegroups.cn/static/public/10.21037tcr-24-722-1.docx (19-21). To identify differentially expressed lncRNAs between healthy and AML samples, we employed the R package “limma”. The “limma” package was also utilized to generate a heatmap and volcano plot. Additionally, we utilized the “pheatmap” package to create the heatmap and volcano plot.
Construction of the ER stress-related lncRNA signature
We initially employed univariate logistic regression to preliminarily identify lncRNAs associated with ER stress that possess prognostic value. Afterwards, we employed the least absolute shrinkage and selection operator (LASSO) regression technique to identify the most informative predictive characteristics. This approach effectively fitted a generalized linear model and mitigated the risk of overfitting (22). The multivariate logistic regression analysis incorporated lncRNAs with non-zero LASSO regression coefficients. Visual representations of the logistic regression results were created through forest plots using the R package “ggplot2”. Subsequently, a prognostic signature was developed based on these findings. In the training cohort, each sample was assigned a risk score using the multivariate logistic regression analysis formula. The samples were then divided into high and low-risk groups based on the median expression level of the training risk score. To evaluate the effectiveness of the model, Kaplan-Meier survival analysis was performed. To further validate the prognostic model, it underwent rigorous testing in an independent testing cohort. This validation process ensured the reliability and applicability of the model beyond the initial training cohort.
Construction of the nomogram and calibration curves
The nomogram is a widely utilized predictive tool in the fields of oncology and medicine (23). It provides a graphical representation of a statistical prediction model, allowing clinicians to estimate the probability of a specific clinical outcome for an individual patient. We developed a predictive nomogram by incorporating the risk score and clinical features to estimate the 1-, 2-, and 3-year overall survival (OS) rates. To evaluate its accuracy in clinical prognosis, we constructed calibration curves. These curves assess the agreement between the predicted and observed outcomes, providing valuable insights into the performance of the nomogram.
Enrichment analyses of function and biological pathways
Genes that showed differential expression in the high- and low-risk groups were determined using the conditions of |log2 fold change (FC) >1| and a false discovery rate (FDR) <0.05. Bar plots and bubble plots were utilized for the visualization of Gene Ontology (GO) and Kyoto Encyclopedia of Genes and Genomes (KEGG) pathways. For pathway enrichment analysis, the gene set named ‘c2.cp.kegg.Hs.symbols’ was acquired from the MSigDB database (http//www.gsea-msigdb.org/gsea/msigdb/index.jsp). Furthermore, the biological pathways were investigated using gene set enrichment analysis (GSEA) (24). To elucidate the primary functions of the distinct genes, we employed the “clusterProfiler” package to conduct KEGG analysis.
Correlation analysis of mutation and tumor mutation burden (TMB) analysis
Somatic mutation data extracted from the TCGA “Masked Somatic Mutation” dataset were processed using the R package “maftools” to visualize the genetic mutational landscape in AML patients. Subsequently, we examined the mutation patterns across different groups and presented the top 20 genes with the highest mutation frequencies. TMB, a quantitative biomarker, was calculated as the number of somatic mutations detected per megabase in tumor samples. The median TMB value was utilized as a threshold to classify patients into TMB-high or TMB-low groups for Kaplan-Meier survival curve analysis.
Evaluation between ER stress-related lncRNA signature and immune characteristics
To assess the relative abundance of immune cell infiltrates in the different risk groups, we utilized the R packages to estimate the proportions of immune cells and stromal cells in a single sample. Furthermore, to delve into the disparities in immune characteristics between the high- and low-risk groups, we utilized the CIBERSORT algorithm to quantify the proportions of infiltrating immune cells (25). We assessed the distribution of immune cells, explored the functionality of the immune-related factors, and evaluated the expression levels of checkpoint genes in AML samples, aiming to understand their correlation with the risk scores. Furthermore, survival analysis was conducted to examine the potential impact of these factors on patient outcomes. We further analyzed the variations between the two groups by plotting multiple box plots.
Establishment of consensus cluster based on ER stress-related lncRNAs
Using the ER stress-related lncRNAs, we conducted unsupervised cluster analysis employing the R package “ConsensusClusterPlus” to identify distinct consensus clusters. The consensus clustering algorithm was applied with 1,000 iterations to ensure the robustness of the clustering results (26). To further validate the distinct clusters obtained, principal component analysis (PCA) was performed. Subsequently, we assessed the intricate association between immune characteristics and the identified clusters.
Potential drug response value evaluation
We retrieved the expression matrix and drug handling information from the Cancer Genome Project (CGP) program, a comprehensive database containing data on 138 anticancer drugs targeting 727 cell lines. Subsequently, we employed the R package “oncoPredict” to analyze the correlation between the risk score and the half-maximal inhibitory concentration (IC50) of each cell line to specific drugs (27). We utilized the Genomics of Drug Sensitivity in Cancer database (GDSC, http://www.cancerrxgene.org/downloads) to analyze the pathways targeted by the aforementioned drugs (28).
Statistical analysis
Statistical analyses in this study were conducted using R x64-4.1.3. The t-test was employed to compare differences in quantitative data and normally distributed variables, while the Wilcoxon rank-sum test was utilized for comparing differences in non-normally distributed variables. To compare differences across more than two groups of variables, the one-way analysis of variance and the Kruskal-Wallis test were applied. Spearman analysis was used for correlation analysis. A significance level of P<0.05 was considered statistically significant. All statistical tests were two-sided.
Results
Construction of ER stress-related lncRNA prognostic signature
In order to create the predictive pattern for ER stress-related lncRNAs, we initially acquired a sum of 295 ER stress-related mRNAs from publicly available databases. Through correlation analysis (|cor| >0.6, P<0.001), we identified 404 non-redundant ER stress-related lncRNAs (available online: https://cdn.amegroups.cn/static/public/10.21037tcr-24-722-2.docx). These lncRNAs are closely correlated with ER stress-related mRNAs and are potentially closely related to the ER stress process in AML disease.
Afterwards, a differential analysis was conducted on these lncRNAs, leading to the identification of 227 lncRNAs associated with ER stress (available online: https://cdn.amegroups.cn/static/public/10.21037tcr-24-722-3.docx) that displayed varying expression levels between normal and tumor samples (|log2FC| >1 and P<0.05). Among these differentially expressed lncRNAs, 136 were upregulated in tumor samples, while 91 were downregulated (Figure 1A,1B).
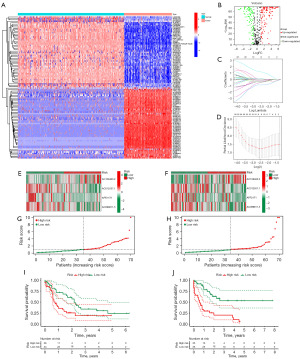
Next, we integrated the differentially expressed lncRNA data with patient survival information to assess the relationship between expression levels and survival time. A subset of 41 lncRNAs, which were differentially expressed and related to ER stress, were identified through univariate Cox analysis. As shown in Table S1, these lncRNAs showed close associations with prognostic outcomes of patients with AML (P<0.05).
In order to enhance the accuracy of our results, we conducted LASSO analysis and multivariate Cox regression analysis (Figure 1C,1D). Afterwards, we chose four lncRNAs (AC108463.2, AC012557.1, AFF2-IT1, and AC099811.5) for the development of the prognostic signature associated with ER stress. The scoring formula was as follows: risk score = AC108463.2 × 2.1761 − AC012557.1 × 1.3870 − AFF2-IT14 × 0.8159 − AC099811.5 × 1.1651.
Based on the median values, the patients were categorized into groups of high risk and low risk. In the training cohort, AC108463.2 showed an increase in expression in the high-risk group, whereas AC012557.1, AFF2-IT1, and AC099811.5 showed a decrease in expression. These findings were consistent in the validation cohort (Figure 1E,1F). Afterwards, patients with AML were sorted based on their risk scores, and the prognostic model was organized in increasing order of risk score (Figure 1G,1H). In both the training and validation cohorts, the Kaplan-Meier survival curves demonstrated a notable disparity in OS among the two groups. In Figure 1I,1J, the survival rate of AML patients in the high-risk category was notably lower than that of patients in the low-risk category. The results indicate that the prognostic signature associated with ER stress has significant potential in forecasting the prognosis of AML.
The association between the prognostic signature of ER stress-related lncRNA and clinical characteristic
Figure 2A shows the execution of univariate Cox regression analysis to examine if clinical characteristics can function as autonomous risk factors in AML patients. The findings showed strong links between the ER stress-related pattern and age (P<0.001) and risk score (P<0.001) among AML individuals. Subsequently, a nomogram incorporating age, gender, race, classification (based on the leukemia morphology code), and risk score was developed to assist in predicting the prognosis of AML patients. Figure 2B displays a nomogram that enables the estimation of the prognosis for patients over 1, 2, and 3 years. Figure 2C show a strong correlation between the projected and real OS rates at 1, 2, and 3 years, as indicated by the calibration curve. The area under the curve (AUC) of 0.811, 0.718, and 0.816 for 1-, 3-, and 5-year survival rates, respectively, indicate a favorable predictive performance (Figure 2D). Furthermore, with regards to the association between clinical characteristics and the predictive model, Figure 2E demonstrates notable variations in OS between AML groups categorized as high- and low-risk across various age brackets (P<0.05). Among individuals aged 55 or younger, the group at high risk demonstrates a significantly lower rate of survival in comparison to the low-risk group. On the other hand, for patients aged above 55, the outcomes are opposite, as the high-risk category exhibits a significantly greater rate of survival compared to the low-risk category (Figure 2F,2G).
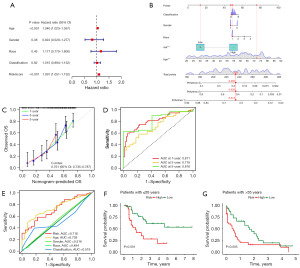
Analysis of the prognostic signature for ER stress-related lncRNA involves enrichment and TMB assessment
In order to examine the biological mechanisms linked to lncRNAs related to ER stress, we performed enrichment analyses using GO and KEGG pathway (Figure 3A,3B). In terms of biological processes (BP), ER stress-related lncRNAs were mainly involved in positively regulating cellular component biogenesis and protein processing regulation. In cellular components (CC), these lncRNAs were primarily enriched in myofibrils and striated muscle thin filaments. Concerning molecular functions (MF), ER stress-related lncRNAs were predominantly associated with growth factor binding and fibronectin binding. In relation to the analysis of KEGG pathway enrichment, we discovered numerous noteworthy pathways associated with the advancement and progression of cancer, including cancer-related transcriptional misregulation, phosphatidylinositol-3-kinase (PI3K)/protein kinase B (AKT) and p53 signaling pathway. Furthermore, we employed GSEA to further elucidate the roles of these genes (Figure 3C). The findings indicated that these genes were highly concentrated in different terms related to cytokine interaction and the immune system.
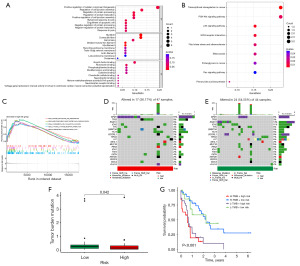
Next, we conducted an extensive analysis of somatic mutations in AML samples. A comparison was made between the distribution of somatic mutations in two risk groups using a waterfall plot. Analysis was conducted on the 20 driver genes with the greatest mutation frequencies. The findings indicated that the group at greater risk displayed a generally elevated frequency of mutations in comparison to the group at lower risk. In the high-risk group, nucleophosmin 1 (NPM1) mutation was observed in 15% of cases, whereas in the low-risk group, it was present in 9% (Figure 3D,3E). Moreover, individuals classified as high-risk exhibited a decreased TMB compared to those in the low-risk category, and this dissimilarity was determined to be statistically significant (Figure 3F, P=0.04). Analysis of the TMB survival curve revealed that patients who had both high-risk scores and high TMB experienced a worse survival outcome (Figure 3G, P<0.001). The results indicate that the risk score has the capacity to function as a predictive marker, and when combined with TMB, it could augment the precision of prognostic forecasts for patients with AML.
Association between ER stress-related lncRNA risk model and infiltration of immune cells
A strong correlation was found between the risk model of ER stress-related lncRNA and the presence of immune cells in the tumor microenvironment (Figure 4A). Negative correlation coefficients were observed for various immune cell types, including T cell CD4+ Th1_XCELL and Macrophage M1_XCELL, indicating that patients with a higher classifier index experienced immunosuppression. T cell CD8+_XCELL, NK cell resting_CIBERSORT, and T cell regulatory (Tregs)_QUANTISEQ also demonstrated negative correlations with the classifier index. Regarding patient survival, individuals at high risk demonstrated notably greater OS rates compared to those at lower risk for various immune cell types, such as mast cells, NK cells, common myeloid progenitors, CD4+ T cell memory subsets, activated mast cells, resting mast cells, and uncharacterized cells (Figure 4B-4I). Conversely, high-risk patients demonstrated lower OS rates for plasmacytoid dendritic cells and M2 macrophages (Figure 4J,4K).
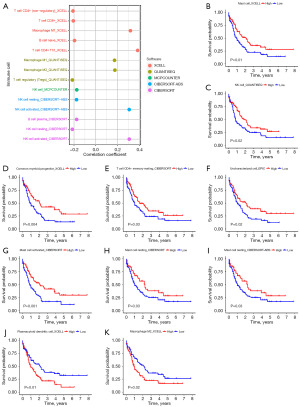
In order to assess the penetration of immune cells in a more comprehensive manner, we performed GSEA on individual samples to compare the scores of immune cells and the reactions of immune response. Figure 5A displayed significant variations in the immunocyte composition between the high- and low-risk groups, as indicated by the findings. In particular, the group at high risk showed elevated levels of pDCs, Tfh, and Tregs in comparison to the group at low risk. In addition, in relation to immune response reactions, we noticed heightened antigen-presenting cells (APC) co-stimulation, chemokine C-C-Motif receptor (CCR) signaling, immune checkpoint regulation, major histocompatibility complex (MHC) class 1 antigen processing and presentation, and type 2 interferon (IFN) response in the high-risk group (Figure 5B). Additionally, we observed significant disparities in the expression levels of genes involved in immune regulation between the two groups with different risk profiles (Figure 5C).
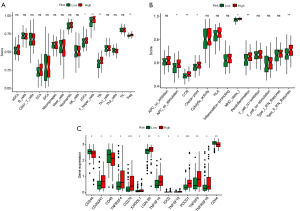
Establishment of consensus cluster based on ER stress-related lncRNAs
We utilized non-negative matrix factorization (NMF) consistent clustering to establish clusters and classify AML samples based on ER stress-related lncRNAs, aiming to identify consensus clusters (Figure 6A). PCA analysis revealed the presence of three separate clusters, specifically referred to as cluster 1, cluster 2, and cluster 3, exhibiting distinct population distributions (Figure 6B). Furthermore, significant differences were noted between the groups at high and low risk in relation to different aspects (Figure 6C). Cluster 1 predominantly comprised high-risk patients, while cluster 3 mainly consisted of low-risk patients (Figure 6D). It is worth mentioning that there was a notable variation in OS between patients belonging to the three subcategories (Figure 6E, P=0.001). Subsequently, we compared the ESTIMATE score and Immune score among the three consensus clusters. Cluster 3 displayed the least favorable scores in relation to both the ESTIMATE score and Immune score when compared to the remaining two clusters (Figure 6F,6G). Given the significance of immunotherapy, we delved deeper into the variations in the expression of immune checkpoint molecules within the three distinct subcategories. Significant differences were observed in the expression of immune checkpoint molecules among the three clusters, as shown in Figure 6H.
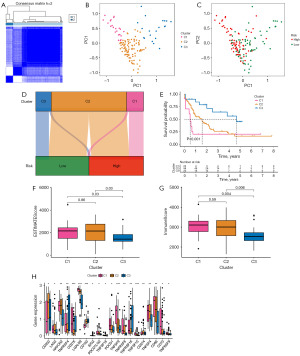
Potential drug response value evaluation of the ER stress-related lncRNA prognostic signature
To examine the influence of a predictive pattern on drug reaction, we performed Spearman correlation analysis to evaluate the connection between the risk score of cellular lines and the IC50 of particular medications. A total of 18 drugs were found to be correlated with the risk scores (Figure 7A). Within this group, 9 medications displayed sensitivity to drugs linked with the risk score, which encompassed Dactolisib (P<0.0001), AZD2014 (P<0.0001), and PF-4708671 (P<0.0001). In contrast, 9 medications exhibited resistance to drugs linked to the risk score, including Doramapimod (P<0.0001), JQ1 (P<0.001), and Entinostat (P<0.001). Significantly, dactolisib displayed the greatest sensitivity to the drug among the low-risk group, whereas doramapimod showed the highest drug sensitivity among the high-risk group (Figure 7B,7C). Figure 7D illustrates the signaling pathways that these drugs aim to target. Our observation revealed that drugs exhibiting sensitivity, which had a positive correlation with high-risk scores, had a tendency to focus on the wingless/integrated (WNT) signaling, c-Jun N-terminal kinases (JNK), and p38 signaling pathway. Conversely, drugs associated with low-risk scores were inclined to target the receptor tyrosine kinase (RTK) signaling, PI3K/mechanistic target of rapamycin (MTOR) signaling, and extracellular signal-regulated protein kinase (ERK)/mitogen-activated protein kinase (MAPK) signaling pathway. Furthermore, we analyzed the differences in drug sensitivity among the three clusters. The results revealed significant variations among the clusters. Cluster 1 exhibited the least responsiveness to Dactolisib, while cluster 3 exhibited the greatest sensitivity, suggesting a potentially more robust reaction to the drug in cluster 3 (Figure 7E). Conversely, cluster 1 exhibited the highest sensitivity to Doramapimod treatment (Figure 7F).
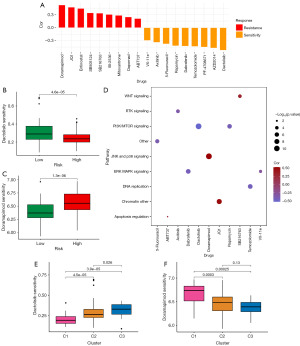
Discussion
AML is a complicated and diverse disorder, with different genetic and molecular changes playing a role in its growth and advancement. The understanding of the underlying mechanisms and biomarkers associated with AML is crucial for improving diagnosis, treatment strategies, and patient outcomes. Recent studies have suggested a potential link between AML and ER stress (29,30). ER stress has been shown to play a role in AML pathogenesis by influencing the immune microenvironment, which is crucial for disease progression and therapeutic responses. Therefore, we believed that lncRNAs could emerge as key players in modulating gene expression and cellular processes, including those involved in ER stress and immunomodulation within the AML microenvironment.
To verify this hypothesis, we performed a screening of differential lncRNAs using 337 healthy samples and 151 AML samples. Afterwards, we utilized LASSO and Cox analysis to create a prognostic signature for ER stress-related lncRNA. The utilization of this signature has the capacity to anticipate the outlook of individuals with AML, assisting in their medical treatment and decision-making procedure. According to a study, manipulating lncRNA H19 has been found to amplify the suppressive impacts of resveratrol on the growth and movement of cancer cells by controlling ER stress (31). In hematologic malignancies, Cui et al. reported the regulation of the miR-320a/XBP1 axis through LINC00963 to modulate ER stress and autophagy in diffuse large B-cell lymphoma (32). By utilizing these advanced statistical methods, we identified four key lncRNAs associated with ER stress that could serve as valuable prognostic markers in AML. The risk value of the signature was assessed through the utilization of training and test cohorts. The results demonstrated a robust predictive capability of the prognostic signature. Significantly, individuals categorized as high-risk demonstrated decreased OS rates in comparison to those in the low-risk category, emphasizing the influence of ER stress as a contributing factor to the prognosis of AML. These findings provide further evidence of the significant role played by ER stress in influencing AML outcomes. The AUC at 1, 3, and 5 years were 0.811, 0.718, and 0.816, respectively, which further illustrate the accuracy of the signature. In addition, a nomogram was developed by integrating known risk factors with the risk score to accurately predict the survival probability of patients with AML. The findings from the nomogram and receiver operating characteristic (ROC) curve analysis suggest a notable disparity in age between the two risk categories. In particular, individuals over the age of 55 exhibit a considerably decreased likelihood of survival among the high-risk category.
Through the analysis of GO and KEGG enrichment, it was discovered that lncRNAs related to ER stress were connected to diverse biological processes. Afterwards, GSEA was additionally used to enhance the function annotation of these genes, including the interaction of various cytokines and terms associated with the immune system. It goes without saying that ER stress is intricately linked to the tumor microenvironment and immune reactions. Inactivation of protein kinase R-like endoplasmic reticulum kinase (PERK), an ER stress kinase, induces paraptosis and the generation of type I IFN, resulting in the augmentation of anti-cancer T cell reactions (33). The atypical stimulation of the ER stress receptors and their subsequent signaling pathways has become a crucial controlling element in the development of tumors, spread of cancer cells, and the reaction to chemotherapy, targeted treatment, and immunotherapy (11). Afterwards, the features illustrating the tumor microenvironment and the map of somatic mutations were examined in a hierarchical manner for the patients with AML. Somatic analysis revealed a high frequency of NPM1 mutations in the high-risk group, while runt-related transcription factor 1 (RUNX1) mutations were highly prevalent in both the low-risk group. Cocciardi et al. investigated the clonal evolution patterns of AML with NPM1 gene mutations (34). Preudhomme et al. discovered a significant occurrence of RUNX1 biallelic modification in AML resulting from familial platelet disorder (35). The findings underscore the possibility of focusing on ER stress as a significant therapeutic objective and offer direction for the creation of innovative treatment approaches. According to our investigation, the low-risk group exhibited a considerably elevated TMB in comparison to the high-risk group. Importantly, the combination of high-risk status and high TMB resulted in a significantly lower OS rate when compared to the other three groups. To some degree, these findings indicated that the examined signature had a comparable functional role to TMB.
Most immune cells showed an inverse association with risk scores in relation to immune responses. The survival analysis results revealed that patients in different groups exhibited varying survival capacities in relation to different immune cell types. Research has suggested that LILRB3 plays a supportive role in the development of AML and regulates T-cell antitumor immune responses (36). Furthermore, it has been found that memory-like natural killer cells induced by cytokines demonstrate heightened reactions towards myeloid leukemia (37). Single sample gene set enrichment analysis (ssGSEA) results also showed that many immunocytes are differing in high- and low-risk groups. These findings highlight the potential prognostic significance of these specific cell types in determining the survival outcomes of patients with different risk levels. Next, consensus clusters were established to classify the AML samples based on ER stress-related lncRNAs. Considering the requirement for personalized immunotherapy approaches in treating patients with AML, the development of a novel AML clustering system is of paramount importance and should be prioritized. AML patients were categorized into three clusters based on the findings of lncRNAs associated with ER stress. Cluster 1 primarily consisted of patients at high risk, while Cluster 3 mainly comprised patients at low risk. There was a notable disparity in the operating system among the patients in the three subcategories. Compared to the other two clusters, Cluster 3 displayed the least favorable scores in relation to the ESTIMATE score and immune score. Given the significance of immunotherapy, our investigation persisted in examining the variations in the expression of immune checkpoint molecules within the three distinct subcategories. Significant variations were observed in the expression of immune checkpoint molecules among the three clusters, as indicated by the results.
Drug resistance is one of the significant challenges in the treatment of AML, as it limits the effectiveness of therapy and negatively impacts patient survival rates. Long et al. summarized the genetic biomarkers of drug resistance in AML, highlighting that the emergence of multidrug resistance significantly impedes the effectiveness of chemotherapy and determines adverse prognosis (38). Hence, it is of significant importance to examine the correlation between drug responsiveness and individuals categorized as high- and low-risk groups, which can greatly impact the therapy of AML patients. In our study, we identified 18 drugs that showed significant correlation with the risk score. Dactolisib showed the greatest sensitivity to drugs in the low-risk category, while Doramapimod displayed the highest drug sensitivity in the high-risk category. Moreover, we also analyzed the difference of the “conventional” drug, Methotrexate, in the treatment of AML between the high- and low-risk groups. Unfortunately, there was no significant difference between the two groups (P>0.05). The sensitivity of conventional drugs to the high-risk and low-risk groups still needs to be further improved by adjusting the signature. The drug sensitivity among the three clusters varies significantly for both Dactolisib and Doramapimod. Dactolisib can inhibit tumor cell growth and metastasis, preventing tumor formation. It achieves this by modulating cellular signaling pathways, promoting tumor cell apoptosis, and suppressing tumor cell proliferation, thereby preventing the occurrence and metastasis of cancer (39). It has been demonstrated that Doramapimod, an inhibitor of p38 mitogen-activated protein kinase (MAPK), hinders the phosphorylation of B-cell CLL/lymphoma 2 (BCL2) mediated by p38 MAPK. Consequently, it boosts the responsiveness to the BCL2 inhibitor venetoclax and heightens the drug sensitivity in AML (40). Furthermore, our investigation also revealed that medications whose responsiveness was associated with an elevated risk score commonly focused on the WNT signaling, JNK, and p38 signaling pathways. Conversely, medications linked to a minimal-risk rating had a higher probability of focusing on the RTK signaling, PI3K/MTOR signaling, and ERK MAPK signaling pathways.
Conclusions
To summarize, utilizing ER stress-related lncRNAs, we developed a unique prognostic marker linked to ER stress. Our data not only serves as a valuable resource for deciphering the immune responses, somatic mutational landscape, drug resistance and potential biological functions in AML patients but also provides promising insights for developing accurate therapeutic strategies for them. Nevertheless, our study has certain limitations. The findings were primarily derived from bioinformatics analysis and should be validated through subsequent experiments such as single-cell sequencing. Single-cell sequencing can be used to cluster the cells and study the functions of the cell populations independently. Nonetheless, our findings were predominantly derived from bioinformatics analysis and require subsequent experimental verification. Nevertheless, in spite of these constraints, our discoveries provide valuable perspectives on the correlation between lncRNAs associated with ER stress and the immune microenvironment of AML.
Acknowledgments
Funding: None.
Footnote
Reporting Checklist: The authors have completed the TRIPOD reporting checklist. Available at https://tcr.amegroups.com/article/view/10.21037/tcr-24-722/rc
Peer Review File: Available at https://tcr.amegroups.com/article/view/10.21037/tcr-24-722/prf
Conflicts of Interest: All authors have completed the ICMJE uniform disclosure form (available at https://tcr.amegroups.com/article/view/10.21037/tcr-24-722/coif). The authors have no conflicts of interest to declare.
Ethical Statement: The authors are accountable for all aspects of the work in ensuring that questions related to the accuracy or integrity of any part of the work are appropriately investigated and resolved. This study was conducted in accordance with the Declaration of Helsinki (as revised in 2013).
Open Access Statement: This is an Open Access article distributed in accordance with the Creative Commons Attribution-NonCommercial-NoDerivs 4.0 International License (CC BY-NC-ND 4.0), which permits the non-commercial replication and distribution of the article with the strict proviso that no changes or edits are made and the original work is properly cited (including links to both the formal publication through the relevant DOI and the license). See: https://creativecommons.org/licenses/by-nc-nd/4.0/.
References
- Shimony S, Stahl M, Stone RM. Acute myeloid leukemia: 2023 update on diagnosis, risk-stratification, and management. Am J Hematol 2023;98:502-26. [Crossref] [PubMed]
- Wang B, Reville PK, Yassouf MY, et al. Comprehensive characterization of IFNγ signaling in acute myeloid leukemia reveals prognostic and therapeutic strategies. Nat Commun 2024;15:1821. [Crossref] [PubMed]
- Perna F, Espinoza-Gutarra MR, Bombaci G, et al. Immune-Based Therapeutic Interventions for Acute Myeloid Leukemia. Cancer Treat Res 2022;183:225-54. [Crossref] [PubMed]
- Tosic N, Marjanovic I, Lazic J. Pediatric acute myeloid leukemia: Insight into genetic landscape and novel targeted approaches. Biochem Pharmacol 2023;215:115705. [Crossref] [PubMed]
- Chen CY. Chromothripsis in myeloid malignancies. Ann Hematol 2024; [Crossref] [PubMed]
- Chen X, Shi C, He M, et al. Endoplasmic reticulum stress: molecular mechanism and therapeutic targets. Signal Transduct Target Ther 2023;8:352. [Crossref] [PubMed]
- Groenendyk J, Agellon LB, Michalak M. Calcium signaling and endoplasmic reticulum stress. Int Rev Cell Mol Biol 2021;363:1-20. [Crossref] [PubMed]
- Salvagno C, Mandula JK, Rodriguez PC, et al. Decoding endoplasmic reticulum stress signals in cancer cells and antitumor immunity. Trends Cancer 2022;8:930-43. [Crossref] [PubMed]
- Ajoolabady A, Kaplowitz N, Lebeaupin C, et al. Endoplasmic reticulum stress in liver diseases. Hepatology 2023;77:619-39. [Crossref] [PubMed]
- Celik C, Lee SYT, Yap WS, et al. Endoplasmic reticulum stress and lipids in health and diseases. Prog Lipid Res 2023;89:101198. [Crossref] [PubMed]
- Chen X, Cubillos-Ruiz JR. Endoplasmic reticulum stress signals in the tumour and its microenvironment. Nat Rev Cancer 2021;21:71-88. [Crossref] [PubMed]
- Xie F, Qu J, Lin D, et al. Reduced Proteolipid Protein 2 promotes endoplasmic reticulum stress-related apoptosis and increases drug sensitivity in acute myeloid leukemia. Mol Biol Rep 2023;51:10. [Crossref] [PubMed]
- Bridges MC, Daulagala AC, Kourtidis A. LNCcation: lncRNA localization and function. J Cell Biol 2021;220:e202009045. [Crossref] [PubMed]
- Li P, Li J, Wen F, et al. A novel cuproptosis-related LncRNA signature: Prognostic and therapeutic value for acute myeloid leukemia. Front Oncol 2022;12:966920. [Crossref] [PubMed]
- Izadirad M, Jafari L, James AR, et al. Long noncoding RNAs have pivotal roles in chemoresistance of acute myeloid leukemia. Drug Discov Today 2021;26:1735-43. [Crossref] [PubMed]
- Martin FJ, Amode MR, Aneja A, et al. Ensembl 2023. Nucleic Acids Res 2023;51:D933-41. [Crossref] [PubMed]
- Xin T, Sun Y, Meng H, et al. Identification of endoplasmic reticulum stress-related lncRNAs in lung adenocarcinoma by bioinformatics and experimental validation. Ann Med 2023;55:2251500. [Crossref] [PubMed]
- Zheng Y, Yue X, Fang C, et al. A Novel Defined Endoplasmic Reticulum Stress-Related lncRNA Signature for Prognosis Prediction and Immune Therapy in Glioma. Front Oncol 2022;12:930923. [Crossref] [PubMed]
- Gong T, Liu Y, Tian Z, et al. Identification of immune-related endoplasmic reticulum stress genes in sepsis using bioinformatics and machine learning. Front Immunol 2022;13:995974. [Crossref] [PubMed]
- Zhang Q, Guan G, Cheng P, et al. Characterization of an endoplasmic reticulum stress-related signature to evaluate immune features and predict prognosis in glioma. J Cell Mol Med 2021;25:3870-84. [Crossref] [PubMed]
- Li F, Ma J, Yan C, et al. ER stress-related mRNA-lncRNA co-expression gene signature predicts the prognosis and immune implications of esophageal cancer. Am J Transl Res 2022;14:8064-84. [PubMed]
- Friedman J, Hastie T, Tibshirani R. Regularization Paths for Generalized Linear Models via Coordinate Descent. J Stat Softw 2010;33:1-22. [Crossref] [PubMed]
- Iasonos A, Schrag D, Raj GV, et al. How to build and interpret a nomogram for cancer prognosis. J Clin Oncol 2008;26:1364-70. [Crossref] [PubMed]
- Subramanian A, Tamayo P, Mootha VK, et al. Gene set enrichment analysis: a knowledge-based approach for interpreting genome-wide expression profiles. Proc Natl Acad Sci U S A 2005;102:15545-50. [Crossref] [PubMed]
- Chen B, Khodadoust MS, Liu CL, et al. Profiling Tumor Infiltrating Immune Cells with CIBERSORT. Methods Mol Biol 2018;1711:243-59. [Crossref] [PubMed]
- Wilkerson MD, Hayes DN. ConsensusClusterPlus: a class discovery tool with confidence assessments and item tracking. Bioinformatics 2010;26:1572-3. [Crossref] [PubMed]
- Maeser D, Gruener RF, Huang RS. oncoPredict: an R package for predicting in vivo or cancer patient drug response and biomarkers from cell line screening data. Brief Bioinform 2021;22:bbab260. [Crossref] [PubMed]
- Yang W, Soares J, Greninger P, et al. Genomics of Drug Sensitivity in Cancer (GDSC): a resource for therapeutic biomarker discovery in cancer cells. Nucleic Acids Res 2013;41:D955-61. [Crossref] [PubMed]
- Philippe C, Jaud M, Féral K, et al. Pivotal role of the endoplasmic reticulum stress-related XBP1s/miR-22/SIRT1 axis in acute myeloid leukemia apoptosis and response to chemotherapy. Leukemia 2024;38:1764-76. [Crossref] [PubMed]
- Turos-Cabal M, Sánchez-Sánchez AM, Puente-Moncada N, et al. FLT3-ITD regulation of the endoplasmic reticulum functions in acute myeloid leukemia. Hematol Oncol 2024;42:e3281. [Crossref] [PubMed]
- Li T, Zhang X, Cheng L, et al. Modulation of lncRNA H19 enhances resveratrol-inhibited cancer cell proliferation and migration by regulating endoplasmic reticulum stress. J Cell Mol Med 2022;26:2205-17. [Crossref] [PubMed]
- Cui Y, Xu H, Yang Y, et al. The regulation of miR-320a/XBP1 axis through LINC00963 for endoplasmic reticulum stress and autophagy in diffuse large B-cell lymphoma. Cancer Cell Int 2021;21:305. [Crossref] [PubMed]
- Mandula JK, Chang S, Mohamed E, et al. Ablation of the endoplasmic reticulum stress kinase PERK induces paraptosis and type I interferon to promote anti-tumor T cell responses. Cancer Cell 2022;40:1145-1160.e9. [Crossref] [PubMed]
- Cocciardi S, Dolnik A, Kapp-Schwoerer S, et al. Clonal evolution patterns in acute myeloid leukemia with NPM1 mutation. Nat Commun 2019;10:2031. [Crossref] [PubMed]
- Preudhomme C, Renneville A, Bourdon V, et al. High frequency of RUNX1 biallelic alteration in acute myeloid leukemia secondary to familial platelet disorder. Blood 2009;113:5583-7. [Crossref] [PubMed]
- Wu G, Xu Y, Schultz RD, et al. LILRB3 supports acute myeloid leukemia development and regulates T-cell antitumor immune responses through the TRAF2-cFLIP-NF-κB signaling axis. Nat Cancer 2021;2:1170-84. [Crossref] [PubMed]
- Romee R, Rosario M, Berrien-Elliott MM, et al. Cytokine-induced memory-like natural killer cells exhibit enhanced responses against myeloid leukemia. Sci Transl Med 2016;8:357ra123. [Crossref] [PubMed]
- Long L, Assaraf YG, Lei ZN, et al. Genetic biomarkers of drug resistance: A compass of prognosis and targeted therapy in acute myeloid leukemia. Drug Resist Updat 2020;52:100703. [Crossref] [PubMed]
- Youssef M, Moussa N, W, Helmy M, et al. Unraveling the therapeutic potential of GANT61/Dactolisib combination as a novel prostate cancer modality. Med Oncol 2022;39:143. [Crossref] [PubMed]
- Kurtz SE, Eide CA, Kaempf A, et al. Associating drug sensitivity with differentiation status identifies effective combinations for acute myeloid leukemia. Blood Adv 2022;6:3062-7. [Crossref] [PubMed]