Identification of the CD8+ T-cell exhaustion signature of hepatocellular carcinoma for the prediction of prognosis and immune microenvironment by integrated analysis of bulk- and single-cell RNA sequencing data
Highlight box
Key findings
• A 13-gene CD8+ T cell exhaustion (TEX) signature was developed for hepatocellular carcinoma (HCC) prognosis prediction.
• The signature showed strong predictive power for overall survival across different datasets and populations.
• The risk score derived from the signature was an independent predictor of HCC prognosis.
• Significant differences in clinical characteristics, functional analysis, mutation landscape, chemotherapy sensitivity, and immune cell infiltration were observed between risk groups.
What is known and what is new?
• CD8+ TEX plays a crucial role in anti-tumor immunity and correlates with patient prognosis in HCC.
• This study introduces a novel CD8+ TEX-based signature using integrated analysis of bulk and single-cell RNA sequencing data for HCC prognosis and immune microenvironment prediction.
What is the implication, and what should change now?
• The CD8+ TEX signature could be used to stratify HCC patients for personalized treatment strategies.
• The model's ability to predict targeted drug sensitivity and immune cell infiltration suggests its potential in guiding immunotherapy and chemotherapy decisions.
• Further clinical validation and integration of this signature into HCC management protocols should be considered to improve patient outcomes.
Introduction
Liver cancer is the eighth most common type of cancer and the third leading cause of death among cancer-related ailments. Hepatocellular carcinoma (HCC) accounts for 80% of liver cancer cases. In 2019, approximately 747,000 instances of HCC were recorded worldwide. Additionally, HCC led to roughly 480,000 fatalities. The outlook for HCC patients is typically bleak, with a median survival period ranging from 6 to 10 months (1). Traditional HCC treatment methods include surgical interventions (resection and liver transplantation), radiofrequency ablation, microwave ablation, radiotherapy, and transcatheter arterial chemoembolization (TACE). In the past decade, researchers have begun to pay more attention to targeted therapy and immunotherapy in patients with HCC (2).
The compromised anticancer immunity in the cancer microenvironment is a hallmark of cancer because it allows cancer cells to grow and spread unchecked (3). Immunotherapy is a promising approach in the field of antitumor therapy as it uses the body’s own immune system to fight cancer. One of the key players in this therapy is CD8+ T cells, which are responsible for killing tumor cells. These cells can be activated by tumor-related antigens, and once activated, they can specifically target and destroy cancer cells (4). The quantity and function of CD8+ T cells largely determine the anti-tumor effect and affect the prognosis. Research has shown that patients with higher levels of CD8+ T cells in tumors tend to have better outcomes and longer survival rates (5,6); however, under the continuous stimulation of tumor antigens and inflammatory factors, the proliferation ability of CD8+ T cells is gradually weakened, and the anti-tumor effect is gradually decreased, which is called the CD8+ T cells exhaustion (7). Although exhausted CD8+ T cells are unable to effectively clear tumor cells and promote tumor progression, CD8+ T cell exhaustion (TEX) is not irreversible (8). By understanding the mechanisms of CD8+ TEX, researchers hope to identify pathways that can be targeted to revive exhausted cells and enhance the immune response against cancer cells. This provides a theoretical basis for the development of new immunotherapy strategies that could improve patient outcomes.
Over the past decade, RNA sequencing (RNA-seq) has become an indispensable tool for transcriptome-wide analysis of differential gene expression (9). Single-cell RNA sequencing (scRNA-seq) overcomes the limitations of bulk RNA-seq. Bulk RNA-seq technology provides the transcription profile of a population of cells or the average expression level of tissues but cannot reveal the gene expression patterns of individual cells (10). The application of scRNA-seq can be used to analyze the transcription profile at the single-cell level and obtain more detailed information about the immune microenvironment around the tumor (11).
Our study confirmed that CD8+ TEX was evident in HCC using scRNA-seq. Combined with bulk RNA-seq analysis, we constructed a risk prediction model for predicting CD8+ TEX with good performance. This model is of great significance for guiding immune checkpoint blockade therapies. CD8+ TEX is an important factor promoting the escape of tumor immunity, and the intervention of CD8+ TEX is expected to become a new effective treatment against HCC. We present this article in accordance with the TRIPOD reporting checklist (available at https://tcr.amegroups.com/article/view/10.21037/tcr-24-650/rc).
Methods
Acquisition and processing of scRNA-seq and bulk RNA-seq
The scRNA-seq data of 34,413 HCC cells and 28,686 normal cells in 10 samples were obtained from the GSE149614 dataset in the Gene Expression Omnibus (GEO) database. Bulk RNA-seq data of 365 HCC samples of training dataset were obtained from The Cancer Genome Atlas (TCGA) database. Bulk RNA-seq data of 221 HCC samples from the GSE14520 dataset in the GEO database and 232 HCC samples from the ICGC-LIRI-JP dataset in the International Cancer Genome Consortium (ICGC) database were treated as external validation datasets. In this study, HCC samples from patients with a survival time <30 days, uncertain survival status, or unclear clinicopathological characteristics were excluded.
scRNA-seq data clustering dimension reduction
First, we normalized the data through log-normalization and found the top 2,000 highly variable genes through the FindVariableFeatures function. All genes were scaled using the ScaleData function, and the RunPCA function was used to reduce the dimensions of the top 2,000 highly variable genes screened. We clustered the cells through the “FindNeighbors” (dim =1:20) and found the cell clusters through the “FindClusters” (resolution = 0.5). Next, we selected the top 20 principal components (PCs) to further reduce dimensionality using the uniform manifold approximation and projection (UMAP) method (12). Finally, we screened the marker genes of 29 subgroups through the “FindAllMarkers” (logfc =0.25 and Minpct =0.25). Finally, we used the corrected P<0.05 to screen the marker gene.
CD8+ TEX model construction and validation
CD8+ T cell-related genes associated with prognosis were identified by univariate analysis, least absolute shrinkage and selection operator (LASSO), and multivariate analysis of differentially expressed genes (DEGs). The training cohort (TCGA dataset) was used to construct the risk-score model. External validation cohorts (GEO and ICGC datasets) were then used to verify the reliability of the risk score model. Risk score = Σ coefficient mRNAn × expression level mRNAn. The samples were divided into high- and low-risk groups based on the median risk score. The risk score distribution was plotted using the R package of “time ROC (receiver operating characteristic)”. The log-rank test was used to compare survival differences between the two groups. The overall survival (OS) of each group was determined using the Kaplan-Meier (KM) survival curve.
Independent prognostic analysis and nomogram construction
Based on the TCGA cohort, clinicopathologic variables [e.g., age, sex, tumor grade and Tumor-Node-Metastasis (TNM) staging system] and CD8+ T cells signature were separately incorporated into univariate and multivariate analyses. Subsequently, prognostic variables were combined into a nomogram for predicting 1-, 3- and 5-year OS. ROC curves and calibration curves were used to evaluate the predictive performance and accuracy of the nomogram.
Functional enrichment analysis
We used the “ClusterProfiler” R package to analyze the Gene Ontology (GO) and Kyoto Encyclopedia of Genes and Genomes (KEGG) pathways and “circlize” R package for visualization of the GO result. We also performed KEGG analysis by Gene Set Enrichment Analysis (GSEA) algorithm using “c2.cp.kegg.Hs.symbols.gmt” to compare the differences in enrichment pathways between different risk groups.
Somatic mutation analysis
We utilized maftools to evaluate the somatic mutation data of HCC samples saved in Mutation Annotation Format (MAF) (13). We calculated the tumor mutation burden (TMB) score for each HCC patient and investigated the relationship between TMB and risk score. The TMB score was calculated using the formula: (total number of mutations/total covered bases) × 106 (14). We employed KM analysis to explore the prognostic value of TMB in HCC.
Chemotherapy sensitivity analysis
We estimated the half-maximal inhibitory concentration (IC50) of commonly used chemotherapy drugs for HCC using the “prophytic” R package based on the public pharmacology website “Genomics of Drug Sensitivity in Cancer” (GDSC).
Correlation analysis of TEX signature and immune microenvironment
We calculated the immune score, stromal score and tumor purity of each sample through the ‘ESTIMATE’ package and presumed infiltration of immune cells by CIBERSORT package. CIBERSORT is a method based on a matrix of gene expression to estimate the relative proportions of various cell subsets in tissues (15,16). This study leveraged the CIBERSORT assay to evaluate differences in immune cell populations among distinct groups. TIMER, CIBERSORT, CIBERSORT-ABS, QUANTISEQ, MCPCOUNTER, XCELL, and EPIC algorithms were used to analyze the correlations between the risk score and the infiltration of CD8+ T cells.
Statistical analysis
Continuous variables were expressed as the mean ± standard deviation. The χ2 test and t-test/variance analysis were used separately to compare the distribution of dichotomous and continuous variables. KM statistics and log-rank tests were used for survival analysis. All statistical analyses were carried out using R and Perl, and statistical significance was set at P<0.05.
Ethical statement
The study was conducted in accordance with the Declaration of Helsinki (as revised in 2013) and was approved by the Ethics Committee of the Mengchao Hepatobiliary Hospital of Fujian Medical University (No. AF/SW-10/02.0). Written informed consent are not required.
Results
scRNA-seq data clustering dimension reduction
A schematic representation of the study design is shown in Figure 1. Principal component analysis (PCA) was used for the preliminary dimensionality reduction of the scRNA-seq data. The top 20 PCs with significant differences were selected for further analysis. The cells were aggregated into 29 clusters according to the UMAP algorithm (Figure 2A). The data for the top 20 marker genes are listed in Table S1. Clusters were annotated through the ‘SingleR’ package based on marker genes (Table S2). Clusters 0, 1, 4, 12, 19, 23 were T cells. Clusters 2, 13, 25, 26, and 27 are monocytes. Clusters 3, 6, and 22 were macrophages. Clusters 5, 18, and 28 were natural killer (NK) cells. Clusters 7, 8, 14, 16, 17, 20, 21, 24 were hepatocytes. Clusters 9 and 15 contained B-cells. Cluster 10 were endothelial cells. Cluster 11 were tissue stem cells (Figure 2B). We found that cluster 0 cells were the most significantly reduced among all cells in HCC tissues compared to those in normal tissues (Figure 2A). Cluster 0 cells were identified as CD8+ T cells by using a cell dot map and marker genes (Figure 2C).
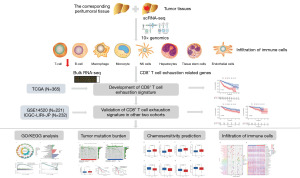
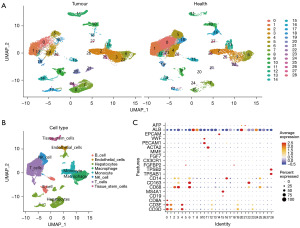
Construction and verification of CD8+ T cells exhaustion model
DEGs of each cluster were identified, and volcano plots of the DEGs in each cluster are shown in the supplementary material. We identified 797 DEGs of CD8+ T cells in normal and HCC tissues by analyzing the TCGA dataset. Volcano plots of the DEGs are shown in Figure 3A. Univariate Cox regression analysis was used to screen for survival-related CD8+ T cells genes (Figure S1). LASSO Cox regression analysis was performed to reduce multicollinearity, and the number of genes was narrowed down to 30 (Figure 3B,3C). Multivariate Cox analysis identified the following 13 genes (HSPD1, UBB, DNAJB4, CALM1, LGALS3, BATF, COMMD3, IL7R, FDPS, DRAP1, RPS27L, PAPOLA, GPR171) and their correlation coefficients, which were used for subsequent model construction (Figure 3D). The risk score was calculated as follows: risk score = (0.327885223854173 × expression of HSPD1) + (−0.721356816880481 × expression of UBB) + (0.331440322615168 × expression of DNAJB4) + (0.602486359882098 × expression of CALM1) + (0.15669643850664 × expression of LGALS3) + (0.146585622608913 × expression of BATF) + (0.539731037131079× expression of COMMD3) + (−0.357375447327156 × expression of IL7R) + (0.192222102819627 × expression of FDPS) + (0.363979655423306 × expression of DRAP1) + (−0.435854620712225 × expression of RPS27L) + (0.507842889478542 × expression of PAPOLA) + (−0.579485721648165 × expression of GPR171). Based on the median score calculated using the risk score formula, 365 patients from the training cohort were equally divided into low-risk and high-risk groups. Patients in the high-risk group had a higher death rate and shorter survival time than those in the low-risk group. A notable difference in OS time was detected between the low group and high-risk group (P<0.001, Figure 4A). Patients from the GSE14520 and ICGC-LIRI-JP cohorts were used as the validation sets. KM analysis indicated a significant difference in the survival rate between the low-risk group and high-risk group in the GSE14520 cohort (P=0.008, Figure 4B) and the ICGC-LIRI-JP cohort (P=0.003, Figure 4C). Time-dependent ROC analysis was applied to evaluate the sensitivity and specificity of the prognostic model, yielding an area under the curve (AUC) of 0.813 for 1-year, 0.768 for 3-year, and 0.783 for 5-year survival in the TCGA cohort (Figure 4D). ROC curve analysis of the GSE14520 cohort showed that our model had good predictive efficacy for 1-year (AUC =0.670), 3-year survival (AUC =0.639), and 5-year survival (AUC =0.656) (Figure 4E). The ICGC-LIRI-JP cohort showed that the model had good predictive efficacy for 1-year (AUC =0.663) and 3-year survival (AUC =0.742) (Figure 4F). Using different databases, we found that patients in the high-risk group had a higher mortality rate than those in the low-risk group (Figure S2A-S2C).
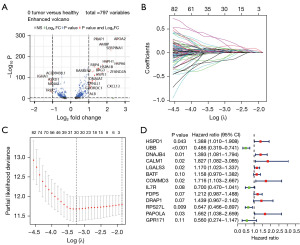
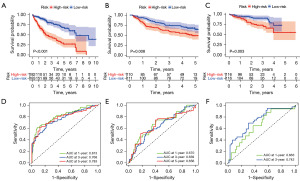
The distribution of clinical features in different risk groups
We analyzed the expression of 13 CD8+ TEX genes in both the low-risk group and the high-risk group, as well as the distribution of different clinical subtypes (Figure 5A). Then, we used bar graphs to compare the proportions of different clinical subtypes in the high-risk group and the low-risk group (Figure 5B-5H). The results indicate that there is no correlation between the risk score and age, gender, N stage, or M stage. However, there is a significant positive correlation between the risk score and grade, stage, and T stage. It suggests that the higher the risk score, the more malignant the tumor is. This highlights the importance of risk assessment in determining the severity of cancer.
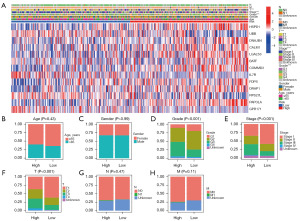
Survival analysis of high-risk and low-risk group in different subgroups of HCC patients
To test the robustness of the CD8+ TEX gene model, we conducted tests on different subgroups of HCC patients. Based on the clinical features, we classified HCC patients into five subgroups. These were age (≤65 and >65 years), gender (female and male), pathological grade (G1–2 and G3–4), pathological stage (1–2 and 3–4) and T stage (T1–2 and T3–4). The data analysis reveals that in comparison to low-risk group, high-risk group have significantly shorter survival times across all subgroups (Figure 6A-6J). This was done to ensure that the model is reliable and effective in predicting outcomes across diverse patient populations.
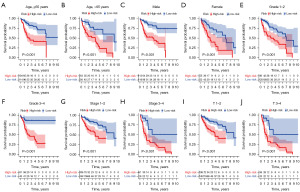
Construction of nomogram based on CD8+ T cell TEX signature combined with clinical characteristics
The results of the univariate and multivariate Cox regression analyses were shown in Figure 7A,7B. Multivariate Cox regression analysis indicated that the risk score was an independent factor for OS in HCC [hazard ratio (HR) =1.163, 95% confidence interval (CI): 1.111–1.218, Figure 7B]. Four prognostic factors were combined to establish a nomogram for predicting the 1-, 3- and 5-year OS (Figure 7C). The AUC for predicting 1-, 3- and 5-year OS were 0.81, 0.82, and 0.79, respectively (Figure 7D). The calibration curves for predicting 1-, 3- and 5-year OS were in good agreement with the observed values (Figure 7E).
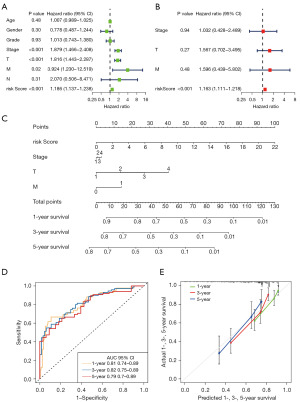
Function enrichment analysis
The result of GO analysis can be classified into three categories: biological processes, cellular components, and molecular functions. Where in biological processes, such as tubulin binding, microtubule binding, antigen binding, serine hydrolase activity; Cellular components, such as spindle, chromosomal region, microtubule, condensed chromosome; and molecular functions, such as organelle fission, nuclear division, chromosome segregation, mitotic nuclear division (Figure 8A,8B). KEGG pathways of high-risk group were enriched in chromosome organization, chromosome segregation, meiotic cell cycle process, microtubule cytoskeleton organization and organelle fission (Figure 8C). KEGG pathways of low-risk group were enriched in alpha amino acid catabolic process, cellular amino acid catabolic process, complement activation, monocarboxylic acid catabolic process and antigen binding (Figure 8D).
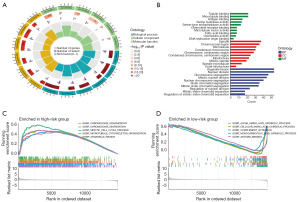
TMB and IC50 of different chemotherapeutic drugs in high-risk and low-risk group
The TCGA database provided valuable information on somatic mutations in high-risk and low-risk groups. The three most common gene mutations in the high-risk group were TP53 (43%), CTNNB1 (27%), and TTN (25%) (Figure 9A). In contrast, the three most common gene mutations in the low-risk group were CTNNB1 (24%), TTN (21%), and MUC16 (14%) (Figure 9B). TMB analysis indicated a significant difference in TMB levels between the high-risk and low-risk groups (P=0.02). The high-risk group’s TMB was significantly higher than the low-risk group’s TMB (Figure 9C). Based on the median TMB value obtained, the patients were divided into high TMB group and low TMB group, and KM analysis was performed. The results showed that the prognosis of the low TMB group was better (P<0.001), indicating that TMB may be an indicator of poor prognosis in HCC patients (Figure 9D). Based on the risk score and TMB, patients were divided into four subgroups for survival evaluation. The results showed that the subgroup with low TMB and low risk score had the best prognosis (P<0.001), indicating the effectiveness of the model in identifying the best prognostic subgroup (Figure 9E). We further examined whether the risk score could predict the sensitivity of eight Food and Drug Administration (FDA)-approved drugs, and found that patients in the low-risk group were more sensitive to axitinib (Figure 10A), erlotinib (Figure 10B), gefitinib (Figure 10C), and lapatinib (Figure 10D) (P<0.001). Patients in the high-risk group were more sensitive to PD.173074 (Figure 10E), tipifarnib (Figure 10F) and vinorelbine (Figure 10G) and sorafenib (Figure 10H) (P<0.001). These results suggest that the CD8+ T cells exhaustion signature is of great significance in drug therapy.
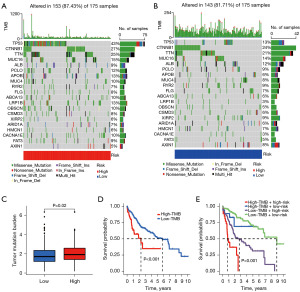
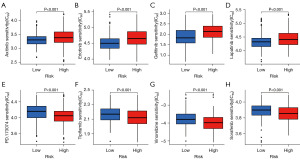
The relationship between risk score and infiltrating immune cells in HCC
To estimate the effect of the 13-gene model on the tumor immune microenvironment (TIME) of HCC, we analyzed the association between the risk score and the infiltration levels of various types of immune cells using the ESTIMATE method. The results showed that the stromal score (Figure 11A), immune score (Figure 11B), and ESTIMATE score (Figure 11C) were higher in the low-risk group than in the high-risk group, while tumor purity was higher in the high-risk group than in the low-risk group (Figure 11D). The correlation between the abundance of 22 immune cells and the risk score was calculated using Pearson’s correlation analysis. The risk score was negatively correlated with the abundance of CD8+ T cells (Figure 11E) and naive B cells (Figure 11F). The risk score was positively correlated with the abundance of regulatory T cells (Figure 11G), macrophages M0 (Figure 11H).
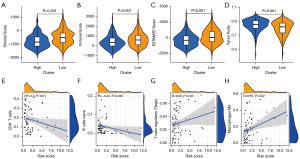
The relationship between risk score and the infiltration of CD8+ T cells
TIMER, CIBERSORT, CIBERSORT-ABS, QUANTISEQ, MCPCOUNTER, XCELL, and EPIC algorithms were used to analyze immune cell infiltration in tumor samples. First, to explore the relationship between the risk score and the infiltration of CD8+ T cells, Spearman correlation analysis was performed, with a resulting lollipop shape, as displayed in Figure 12A. The results indicated that CD8+ T cell infiltration was negatively correlated with the risk score. The heatmap demonstrated that CD8+ T cell infiltration was higher in the low-risk group than in the high-risk group (Figure 12B).
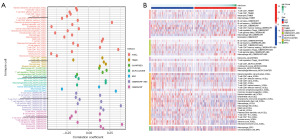
Discussion
Comprehensive anti-tumor therapy is an effective method for treating early-stage HCC, including surgical resection, liver transplantation, radiation therapy, chemotherapy, percutaneous ablation, and immunotherapy. However, the measures available for treating advanced HCC are limited and have poor efficacy (17). Due to the complex tumor microenvironment and high heterogeneity in patients with HCC, there are significant differences in treatment response among individuals (18). Traditional tumor staging only focuses on the tumor status at a given time point and cannot reflect the dynamic changes in the tumor microenvironment and immune characteristics (19). Therefore, it cannot accurately predict the treatment response of HCC patients. New approaches are needed to improve the efficacy of HCC treatment and better predict patient outcomes. High-resolution single-cell data, particularly spatial proteomics, has provided profound insights into the tumor microenvironment, cell-cell interactions, and tumor heterogeneity, thus facilitating the identification of novel diagnostic, prognostic, and therapeutic biomarkers (20,21). CD8+ TEX is a phenomenon that occurs in cancer patients and is characterized by a state of functional impairment of these immune cells. This exhaustion is a result of chronic antigen stimulation and leads to a decrease in the ability of CD8+ T cells to recognize and eliminate cancer cells. CD8+ T cells are a type of immune cells that can recognize and destroy cancer cells by recognizing specific antigens expressed on their surface. However, in many cases, cancer cells can evade immune surveillance and proliferate, leading to the development of tumors. One mechanism by which cancer cells evade the immune system is by inducing TEX (7). This occurs when CD8+ T cells are exposed to persistent antigen stimulation, which leads to the upregulation of inhibitory receptors on their surface, such as programmed cell death-1 (PD-1) (8), T cell immunoglobulin and mucin domain-containing protein 3 (TIM-3) (22), and lymphocyte-activation gene 3 (LAG-3) (8). These inhibitory receptors dampen T cell activation and function, leading to a state of functional exhaustion (8,23-26). Although TEX plays an important role in the immunotherapy of HCC, systematic studies have been lacking. Therefore, we have developed a CD8+ T cell signature that can help physicians evaluate the prognosis and tumor microenvironment of patients with HCC, and provide a theoretical basis for individualized and precise treatment.
scRNA-seq is a powerful tool for analyzing the infiltration of immune cells into tumors. Single-cell data of HCC and para-cancerous samples were obtained from the GEO database (GSE149614). Twenty-nine clusters were identified by using the UMAP algorithm. We found that cluster 0 had the greatest difference between cancerous and para-cancerous tissues. Cluster 0 cells were identified as CD8+ T cells by ‘SingleR’ package, marker genes and cell dot plots. Therapies with antibodies targeting PD-1 and programmed death-ligand 1 (PD-L1) have been shown to be associated with the objective remission rate (ORR) in various cancers and have revolutionized cancer treatments (27). According to the literature, the ORR of PD-1 or PD-L1 drugs is significantly correlated with CD8+ TEX in patients. Patients with CD8+ T cells severe exhaustion may not have a good response to immune checkpoint inhibitors (7,23,28). Therefore, the exhaustion of CD8+ T cells in patients with HCC is of great significance for guiding immune checkpoint blockade therapy. Therefore, it is necessary to construct a model for predicting CD8+ TEX to predict the response to immunotherapy.
Firstly, we identified 797 DEGs associated with CD8+ T cells exhaustion in cancerous and para-cancerous tissues. Secondly, 30 CD8+ T cells exhaustion gene associated with prognosis were identified using univariate and LASSO Cox regression analyses. Finally, 13 genes (HSPD1, UBB, DNAJB4, CALM1, LGALS3, BATF, COMMD3, IL7R, FDPS, DRAP1, RPS27L, PAPOLA, and GPR171) were used to construct a risk prediction model. Based on the median risk score, patients were equally divided into low- and high-risk groups. KM analysis showed that patients in the high-risk group had a higher death rate and a shorter survival time than those in the low-risk group in different (TCGA, GSE14520, and ICGC-LIRI-JP) cohorts and in different specific populations. The ROC curve showed that the model had a good predictive ability in the three cohorts. We also found that there is a significant positive correlation between the risk score and grade stage, stage, and T stage. To facilitate clinical application, we constructed a nomogram by combining risk scores with clinical characteristics (stage, T stage, and M stage). The model predicted 1-, 3-, and 5-year OS rates as high as 0.81, 082, and 0.79, respectively. The calibration curves for predicting 1-, 3- and 5-year OS were in good agreement with the observed values.
Moreover, function enrichment analysis was performed in different risk groups. KEGG pathways of high-risk group were enriched in cell cycle, DNA replication, extracellular matrix (ECM) receptor interaction, neuroactive ligand receptor interaction, pathways in cancer. KEGG pathways of low-risk group were enriched in complement and coagulation cascades, drug metabolism cytochrome p450, fatty acid metabolism, glycine serine and threonine metabolism, retinol metabolism. Additionally, TMB analysis was performed in different risk groups. The three most common gene mutations in the different risk group were different. The TMB was significantly higher in the high-risk group than in the low-risk group. The prognosis of high TMB group was worse than that of low TMB group. Furthermore, we found that patients in the high-risk group were more sensitive to sorafenib. This has important guiding significance for targeted drug therapy. We analyzed the infiltration of immune cells in the high- and low-risk groups. We found that the infiltration of immune cells was significantly reduced in the high-risk group by the ESTIMATE method, and the risk score was negatively correlated with CD8+ T cells and naive B cells, and positively correlated with regulatory T cells and macrophages M0 cells by Spearman correlation analysis. Finally, we further verified the negative correlation between CD8+ T cells infiltration and the risk score using the TIMER, CIBERSORT, CIBERSORT−ABS, QUANTISEQ, MCPCOUNTER, XCELL, and EPIC algorithms. This also means that high-risk patients may not respond well to immunotherapy, which has important guiding implications for immune checkpoint inhibitors.
By searching the literature, we found that there are similar studies whose conclusions are consistent with our study, proving that the prediction model based on CD8+ TEX related genes can predict the prognosis of HCC, providing a new perspective for pre-immunization efficacy assessment, and providing a useful basis for future research in precision immuno-oncology. Our study differs from these studies in the differences in the methods used to screen for CD8+ T cell-related genes and in the gene combinations used to construct the CD8+ T cell prediction model (29,30). However, there are still certain limitations in this study. Firstly, there is lack of immune checkpoint inhibitor cohorts as validation. In addition, there is a lack of experimental verification and mechanism exploration of CD8+ TEX-related genes.
Conclusions
In summary, we screened CD8+ T cell-related genes and constructed a 13-gene risk prediction model by integrated analysis of scRNA-seq and bulk RNA-seq data. This model has strong guiding significance for targeted therapy and immunotherapy in HCC.
Acknowledgments
The authors acknowledge the members of the Mengchao Hepatobiliary Hospital of Fujian Medical University, especially Jianyang Zeng, for excellent technical assistance.
Funding: This research project was supported by
Footnote
Reporting Checklist: The authors have completed the TRIPOD reporting checklist. Available at https://tcr.amegroups.com/article/view/10.21037/tcr-24-650/rc
Peer Review File: Available at https://tcr.amegroups.com/article/view/10.21037/tcr-24-650/prf
Conflicts of Interest: All authors have completed the ICMJE uniform disclosure form (available at https://tcr.amegroups.com/article/view/10.21037/tcr-24-650/coif). The authors have no conflicts of interest to declare.
Ethical Statement:
Open Access Statement: This is an Open Access article distributed in accordance with the Creative Commons Attribution-NonCommercial-NoDerivs 4.0 International License (CC BY-NC-ND 4.0), which permits the non-commercial replication and distribution of the article with the strict proviso that no changes or edits are made and the original work is properly cited (including links to both the formal publication through the relevant DOI and the license). See: https://creativecommons.org/licenses/by-nc-nd/4.0/.
References
- Toh MR, Wong EYT, Wong SH, et al. Global Epidemiology and Genetics of Hepatocellular Carcinoma. Gastroenterology 2023;164:766-82. [Crossref] [PubMed]
- Llovet JM, Kelley RK, Villanueva A, et al. Hepatocellular carcinoma. Nat Rev Dis Primers 2021;7:6. [Crossref] [PubMed]
- Hanahan D, Weinberg RA. Hallmarks of cancer: the next generation. Cell 2011;144:646-74. [Crossref] [PubMed]
- Mittrücker HW, Visekruna A, Huber M. Heterogeneity in the differentiation and function of CD8+ T cells. Arch Immunol Ther Exp (Warsz) 2014;62:449-58. [Crossref] [PubMed]
- Shibutani M, Maeda K, Nagahara H, et al. The Prognostic Significance of the Tumor-infiltrating Programmed Cell Death-1(+) to CD8(+) Lymphocyte Ratio in Patients with Colorectal Cancer. Anticancer Res 2017;37:4165-72. [PubMed]
- Okadome K, Baba Y, Yagi T, et al. Prognostic Nutritional Index, Tumor-infiltrating Lymphocytes, and Prognosis in Patients with Esophageal Cancer. Ann Surg 2020;271:693-700. [Crossref] [PubMed]
- Wherry EJ, Kurachi M. Molecular and cellular insights into T cell exhaustion. Nat Rev Immunol 2015;15:486-99. [Crossref] [PubMed]
- Nguyen LT, Ohashi PS. Clinical blockade of PD1 and LAG3--potential mechanisms of action. Nat Rev Immunol 2015;15:45-56. [Crossref] [PubMed]
- Stark R, Grzelak M, Hadfield J. RNA sequencing: the teenage years. Nat Rev Genet 2019;20:631-56. [Crossref] [PubMed]
- Wang Y, Navin NE. Advances and applications of single-cell sequencing technologies. Mol Cell 2015;58:598-609. [Crossref] [PubMed]
- Lovett M. The applications of single-cell genomics. Hum Mol Genet 2013;22:R22-6. [Crossref] [PubMed]
- Mcinnes L, Healy J, Melville J. UMAP: Uniform Manifold Approximation and Projection for Dimension Reduction. Journal of Open Source Software 2018;3:861. [Crossref]
- Mayakonda A, Lin DC, Assenov Y, et al. Maftools: efficient and comprehensive analysis of somatic variants in cancer. Genome Res 2018;28:1747-56. [Crossref] [PubMed]
- Robinson DR, Wu YM, Lonigro RJ, et al. Integrative clinical genomics of metastatic cancer. Nature 2017;548:297-303. [Crossref] [PubMed]
- Newman AM, Liu CL, Green MR, et al. Robust enumeration of cell subsets from tissue expression profiles. Nat Methods 2015;12:453-7. [Crossref] [PubMed]
- Chen B, Khodadoust MS, Liu CL, et al. Profiling Tumor Infiltrating Immune Cells with CIBERSORT. Methods Mol Biol 2018;1711:243-59. [Crossref] [PubMed]
- Llovet JM, Zucman-Rossi J, Pikarsky E, et al. Hepatocellular carcinoma. Nat Rev Dis Primers 2016;2:16018. [Crossref] [PubMed]
- Li L, Wang H. Heterogeneity of liver cancer and personalized therapy. Cancer Lett 2016;379:191-7. [Crossref] [PubMed]
- Zongyi Y, Xiaowu L. Immunotherapy for hepatocellular carcinoma. Cancer Lett 2020;470:8-17. [Crossref] [PubMed]
- Ho WJ, Zhu Q, Durham J, et al. Neoadjuvant Cabozantinib and Nivolumab Converts Locally Advanced HCC into Resectable Disease with Enhanced Antitumor Immunity. Nat Cancer 2021;2:891-903. [Crossref] [PubMed]
- Mi H, Ho WJ, Yarchoan M, et al. Multi-Scale Spatial Analysis of the Tumor Microenvironment Reveals Features of Cabozantinib and Nivolumab Efficacy in Hepatocellular Carcinoma. Front Immunol 2022;13:892250. [Crossref] [PubMed]
- Liu S, Xu C, Yang F, et al. Natural Killer Cells Induce CD8(+) T Cell Dysfunction via Galectin-9/TIM-3 in Chronic Hepatitis B Virus Infection. Front Immunol 2022;13:884290. [Crossref] [PubMed]
- Wherry EJ. T cell exhaustion. Nat Immunol 2011;12:492-9. [Crossref] [PubMed]
- Pauken KE, Wherry EJ. Overcoming T cell exhaustion in infection and cancer. Trends Immunol 2015;36:265-76. [Crossref] [PubMed]
- Barber DL, Wherry EJ, Masopust D, et al. Restoring function in exhausted CD8 T cells during chronic viral infection. Nature 2006;439:682-7. [Crossref] [PubMed]
- Schietinger A, Greenberg PD. Tolerance and exhaustion: defining mechanisms of T cell dysfunction. Trends Immunol 2014;35:51-60. [Crossref] [PubMed]
- Boussiotis VA. Molecular and Biochemical Aspects of the PD-1 Checkpoint Pathway. N Engl J Med 2016;375:1767-78. [Crossref] [PubMed]
- Speiser DE, Ho PC, Verdeil G. Regulatory circuits of T cell function in cancer. Nat Rev Immunol 2016;16:599-611. [Crossref] [PubMed]
- Liu K, Liu J, Zhang X, et al. Identification of a Novel CD8(+) T cell exhaustion-related gene signature for predicting survival in hepatocellular carcinoma. BMC Cancer 2023;23:1185. [Crossref] [PubMed]
- Chi H, Zhao S, Yang J, et al. T-cell exhaustion signatures characterize the immune landscape and predict HCC prognosis via integrating single-cell RNA-seq and bulk RNA-sequencing. Front Immunol 2023;14:1137025. [Crossref] [PubMed]