Copper metabolism-related lncRNAs predict prognosis and immune landscape in liver cancer patients
Highlight box
Key findings
• We created prognostic models with four copper metabolism-related long non-coding RNAs (CMRLs) (PRRT3-AS1, AC108752.1, AC092115.3, and AL031985.3), and uncovered the relationships between four CMRLs and immune infiltration, drug response, and tumor mutation.
• Our research analyzed The Cancer Genome Atlas-Liver Hepatocellular Carcinoma data (n=424) using the “limma” package in R for differential gene analysis and prognostic signature construction. Validation involved a 1:1 random division and assessment through Kaplan-Meier, C-index, and receiver operating characteristic curves. Multivariate Cox regression revealed independent prognostic indicators, and Gene Ontology/Kyoto Encyclopedia of Genes and Genomes analyses explored biological pathways. Drug sensitivity and mutation analyses were conducted using Genomics of Drug Sensitivity in Cancer and maftools.
• Our study suggested that the CMRLs play a vital role in the prognosis of hepatocellular carcinoma (HCC), which highlights their outstanding predictive performance and improves the precision of immuno-oncology.
What is known and what is new?
• CMRLs are closely associated with the development and prognosis of HCC.
• Our study aimed to develop a prognostic model of CMRLs and further explore the characteristic of immune infiltration, drug response, and tumor mutation.
What is the implication, and what should change now?
• The study’s findings suggest that the predictive model using CMRLs is highly effective in assessing HCC prognosis and could guide personalized treatment. The model should be integrated into clinical practice to better stratify patients, optimize therapeutic decisions, and potentially improve survival outcomes.
Introduction
Currently, hepatocellular carcinoma (HCC) poses a significant global health burden, primarily due to its grave prognosis and a low overall survival (OS) rate, ranking it as the third leading cause of cancer mortality (1-5). Recently, the combination of vascular endothelial growth factor (VEGF) inhibitors and immune checkpoint inhibitors (ICIs) has represented the first-line immune therapy, which has been shown to extend patient life (6). However, there are still many gaps in immunotherapy for liver cancer; new biological markers are urgently needed to close this gap and predict prognosis for HCC.
Copper, a critical nutrient, vital for mitochondrial function, cell death pathways, and antioxidant defense, has recently absorbed more attention for its substantial impact in the development and progression of cancer (7-9). When specific proteins misfold, gathering, or failure occur, copper can accumulate excessively or be transported improperly, leading to the dysfunction of copper homeostasis. This imbalance can result in oxidative stress and cellular toxicity, which have been linked to the development of HCC (10-13). The regulation of cell homeostasis by copper highlights its metabolic fragility, making it a promising target for anti-cancer therapy (14), increasing interest in the relationship between copper and cancer (8,15,16). Copper ion-mediated cell death mechanisms in liver-related tumors and their associated genes are currently a major focus in research, particularly as novel biological signals for exploring immunotherapy and prognosis prediction (17-19). Despite this, the study of copper metabolism in HCC remains scarce.
As an independent unit, long non-coding RNA (lncRNA) genes have been confirmed for their involvement in tumor progression and immune regulation through cell metabolism, promoting proliferation and invasion (20-22), indicating their potential as biomarkers for diagnosis and treatment. Yet, research on the mechanisms of copper metabolism-related lncRNAs (CMRLs) in the occurrence and development of HCC, their clinical applications, and the exploration of related biological signals is almost entirely lacking, severely limiting their clinical applicability in predicting the prognosis and immunotherapeutic guidance of HCC.
In this investigation, we focused on advancing the understanding of the prognostic impact and potential immune-related pathways of CMRLs in HCC. We aimed to develop and validate a novel prognostic tool to refine prognosis estimation of HCC and assist in guiding treatment choices. We present this article in accordance with the TRIPOD reporting checklist (available at https://tcr.amegroups.com/article/view/10.21037/tcr-24-611/rc).
Methods
Materials collection
The foundation of our research involved the expression profiles and clinical data for 424 HCC patients from The Cancer Genome Atlas (TCGA) dataset (https://portal.gdc.nih.gov; accessed on 7 September 2023); patients with missing clinical information were excluded. We validated the signature using training and validation groups divided at a ratio of 1:1. For the identification of copper metabolism-related genes (CMRGs), we utilized the gene dataset from the study of Chang et al. (published on 29 November 2022) (23). The study was conducted in accordance with the Declaration of Helsinki (as revised in 2013).
The differential expressed analysis and construction of the prediction signature of CMRLs
Using the “limma” package in R (The R Foundation for Statistical Computing, Vienna, Austria), we isolated differentially expressed genes related to copper metabolism, by a threshold of |log fold change (logFC)| >1 and P<0.05, and pinpointed the associated CMRLs with a P value of less than 0.001 in correlation analyses. To develop a risk signature of CMRLs, we conducted least absolute shrinkage and selection operator (LASSO) and univariate Cox regression analyses using the “glmnet” package. Overfitting was eliminated by tenfold cross-validation. Multivariate Cox regression analysis was used to identify key genes and obtain their correlation coefficients. The risk score was calculated by a formula (risk score = expressionlncRNA1 × coeflncRNA1 + expressionlncRNA2 × coeflncRNA2 + expressionlncRNA3 × coeflncRNA3) from the weighted expressions of CMRLs.
Assessment and validation of the prognostic efficacy of the CMRLs signature
The HCC patients were randomized into equal-sized training and validation groups. Employing the R package ’survminer’, the median value of the risk score enabled the classification of the TCGA cohort into totally distinct low- and high-risk categories. Survival comparison in both training and validation groups between low- and high-risk categories was conducted via Kaplan-Meier curves and log-rank tests (P<0.05) via the ’survival’ R package. To show the specificity and accuracy of the signature, time-dependent receiver operating characteristic (ROC) curves, assessing 1-, 3-, and 5-year survival rates in both training and validation groups, were further evaluated.
Independent prognostic evaluation and innovative nomogram design
Both univariate and multivariate Cox regression analyses were employed to determine whether the risk score and clinical features (including age, gender, grade, stage) were independent prognostic factors in HCC. A nomogram, developed using the ‘rms’ package in R, incorporated these independent prognostic factors to estimate the OS of HCC patients. Finally, the nomogram’s prognostic precision was assessed through calibration curves and C-index values by R package “pec”.
Comprehensive Gene Ontology (GO) and Kyoto Encyclopedia of Genes and Genomes (KEGG) analyses
To perform thorough analysis in the underlying biological mechanisms of the selected signals, we conducted GO and KEGG pathway enrichment analyses utilizing the ‘clusterProfiler’ package in R, which enabled an in-depth exploration of the gene functions and pathway involvements.
Assessment of immune landscape in the risk signature
Immune infiltration scores were measured by XCELL, TIMER, QUANTISEQ, MCPCOUNT, EPIC, CIBERSORT, and CIBERSORT-ABS. Spearman correlation analysis was employed to investigate the association between immune cells and risk. According to immune cell features of CIBERSORT, HCC cases were divided into two groups. A total of 20 therapeutic suppressive immune checkpoints summarized through browsing data from previous articles was used to compare their expression differences in high- and low-risk groups. The genes positively linked to anti-programmed cell death ligand 1 (PD-L1) drug response obtained from Xu et al.’s cancer and immunity website (http://biocc.hrbmu.edu.cn/TIP/) (24) and Mariathasan et al.’s research features (25) were enriched with biological signal features favorable for cancer immunotherapy in low- and high-risk categories according to gene set variation analysis (GSVA). Besides, the ‘ggcor’ R package was employed to illustrate the association between risk scores and features of biological signal transmission.
Drug sensitivity of the signature
The data of drug sensitivity were obtained from the Genomics of Drug Sensitivity in Cancer (GDSC). Through its treatment response, HCC patient groups were delineated into high- and low-risk categories, according to the half-maximum inhibitory concentration (IC50) gained from the GDSC database using the “oncoPredict” in R.
Analysis in somatic mutations
TCGA-Liver Hepatocellular Carcinoma (TCGA-LIHC) mutation database (https://portal.gdc.cancer.gov/) was the primary resource. We used mutation annotation format to record the mutation information of TCGA samples, and the maftools program to evaluate the information. To better understand the risk of HCC, a comparison was made between patients with and without a tumor mutational burden (TMB) score calculated by taking the ratio of mutations to covered bases and multiplying it by 106.
Statistical analysis
In this study, R software v4.3.1 and v4.1.3 were used for the statistical analysis of the experimental data. For comparison between the two samples, data with normal distribution and uniform variance were analyzed using Student’s t-test; data with uneven variances were analyzed using the Wilcoxon test. Statistical significance was set at P<0.05.
Results
Identification of candidate CMRLs
Totals of 53 differentially expressed CMRGs and 870 CMRLs were identified (Figure 1A,1B). Prognosis-associated CMRLs were identified and overfitting was eliminated by regression analysis. A total of four CMRLs were retained, containing PRRT3-AS1, AC108752.1, AC092115.3, and AL031985.3 (Figure 1C,1D). The four CMRLs were systematically weighted by applying a formula derived from their individual correlation coefficients: risk score = (0.264931734475329 × expression value of PRRT3-AS1) + (0.491168261485836 × expression value of AC108752.1) + (0.768284627469391 × expression value of AC092115.3) + (0.695952669533969 × expression value of AL031985.3). A significant association was identified between the four CMRLs and increased risk of HCC (Figure 1E). The relationship between the four CMRLs and the differentially expressed CMRGs was analyzed (Figure 1F,1G). The differential expression analysis revealed a notable upregulation in the expression of the 4 identified CMRLs within HCC tissue samples (P<0.05) (Figure 1H).
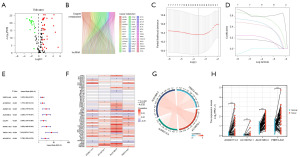
Assessment and validation of the prognostic implications of the signatures in HCC
Using the calculated median risk score derived from our previous formula as a basis, patients were stratified into high- and low-risk groups. Notably, the expression levels of the four genes, including PRRT3-AS1, AC108752.1, AC092115.3, and AL031985.3, were significantly elevated in the high-risk group (Figure 2A). A higher risk score was associated with shorter survival time, which was highlighted by the Kaplan-Meier survival curves (Figure 2B,2C). Meanwhile, The Kaplan-Meier survival curves also showed that OS of higher risk score was significantly reduced in all sample sets (P<0.001, Figure 2D-2F). Additionally, the time-dependent ROC curves demonstrated that all the area under the curve (AUC) values were above 0.65 (Figure 2G-2I), revealing a satisfactory predictive efficacy in HCC of our predictive signature.
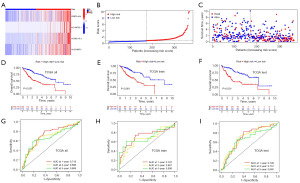
Comprehensive principal component analysis (PCA) of genes and lncRNAs in copper metabolism and the CMRL model for HCC
As shown in Figure 3A-3C, the PCA of CMRGs and lncRNAs, particularly those in the CMRL model, possessed the highest discriminative power in differentiating patients into high- and low-risk groups, highlighting the robust discriminative capabilities of our CMRL model in HCC.
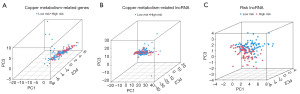
Evaluating the relationship between CMRLs and clinicopathological features
A heatmap was created displaying the various clinical pathological features of all TCGA liver cancer patients (Figure 4A), including gender, grading, stage, T stage, N stage, M stage, and the risk score. We also found the frequency of the clinical pathological features were different between the high- and low-risk groups (Figure 4B-4E), which could be the result of the influence of the four CMRLs.
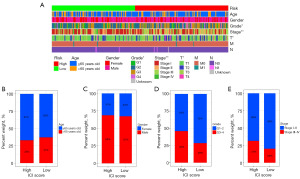
Subgroup analysis of risk models in clinical cohorts of HCC
To further investigate whether our model’s predictive efficacy extends to various clinical subgroups in HCC, all TCGA liver cancer samples were classified by age (>65 vs. ≤65 years old), gender (male vs. female), grade (G1–2 vs. G3–4), and T stage (T1–2 vs. T3–4) to evaluate the survival rate. The OS rate of high-risk patients in all categories was significantly lower than that of low-risk patients (Figure 5A-5H). These results underscore the efficacy of our CMRLs risk model in precisely forecasting the prognostic outcomes across various clinical subgroups within HCC.
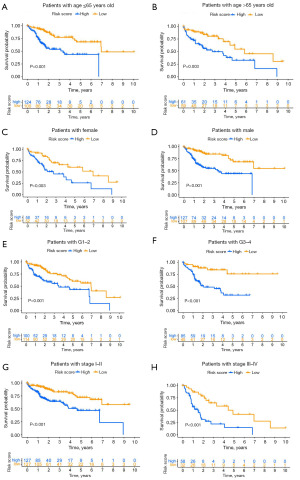
Construction of a nomogram and its prognostic ability
Both univariate regression and multivariate analyses indicated that stage (P<0.001) and risk score (P<0.001) were markedly associated with OS, which were shown in the forest plots (Figure 6A,6B), confirming a significant association between dismal prognosis and advanced disease stages. To enhance the prognostic accuracy of our risk model and broaden its applicability in clinical practice, we established a nomogram based on gender, age, stage, grade, and risk score to predict 1-, 3-, and 5-year prognostic survival of HCC patients (Figure 6C). The risk model basis of four CMRLs played a key determining role in forecasting OS and had the best predictive performance for the prognosis of liver cancer patients (Figure 6D). The predictive power of individual clinical pathological features was lower than that of the CMRLs risk scoring model (AUC =0.718) (Figure 6D,6E), indicating that our CMRLs risk model had marked advantages in predicting the prognosis of HCC. The calibration curve (Figure 6F) showed that the 1-, 3-, and 5-year observed survival results were closely aligned with those of the predicted survival results in our training set.
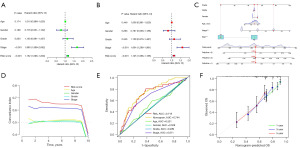
GO and KEGG analyses of HCC patients based on prognostic marker signatures
Functional analysis was conducted of the previously confirmed differential expressed CMRGs. GO analysis predominantly linked these factors to essential biological processes including “mitotic sister chromatid segregation”, “negative regulation of mitotic nuclear division”, “mitotic spindle assembly checkpoint signaling”, “spindle assembly checkpoint signaling”, and “mitotic spindle checkpoint signaling” (Figure 7A-7C), and the cellular component including “chromosomal region”, “chromosome, centromeric region”, “condensed chromosome, centromeric region”, “kinetochore”, and “outer kinetochore” (Figure 7A-7C). Meanwhile, the enrichment pathways of KEGG analysis were mostly associated with “cell cycle” and “oocyte meiosis” (Figure 7D-7F).
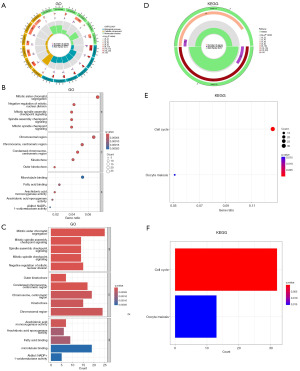
Predictive analysis of tumor microenvironment (TME) and immune cell infiltration
To start with, we examined the risk score and explore the relationship between risk scores and the immune cells (Figure 8A). It was found that the expression of monocytes and macrophages M0 were notably different between the high- and low-risk groups after comparing the immune infiltration in the two groups by using CIBERSORT (Figure 8B). CMRLs may affect the response of HCC patients to immunotherapy by regulating the expression and function of macrophages. However, further biological experiments are needed to support this assumption. Additionally, more noteworthy in immune checkpoint expression was that all of the 29 immune checkpoint genes were significantly unregulated in the high-risk group, including LGALS9, TNFSF4, IDO1, BTNL2, TIGIT, TNFRSF18, PDCD1, HAVCR2, TNFSF18, CD86, CD70, TNFRSF14, TNFRSF4, CD200R1, CD44, NRP1, VTCN1, HHLA2, ICOS, TNFRSF25, TNFRSF9, TNFRSF8, CD80, CTLA4, LAIR1, TNFSF15, CD276, CD28, and TNFSF9 (Figure 8C). Further research of the immune function showed that only APC_co_stimulation and MHC_class_I were significantly down-regulated in the low-risk group, whereas type_II_IFN_response and cytolytic_activity were significantly up-regulated (Figure 8D,8E). Besides, the tumor immune dysfunction and exclusion (TIDE) algorithm was used to calculate the risk scores of immunotherapy for high- and low-risk groups. It revealed that the TIDE scores of the low-risk group were significantly higher than those of the high-risk group (P<0.05) (Figure 8F), indicating that ICI drug therapy is not helpful for low-risk patients because immune evasion increases. Finally, we found that the StromalScore was higher in low-risk patients by testing the tumor immune microenvironment, showing the higher immune levels and immunogenicity of the TME (Figure 8G).
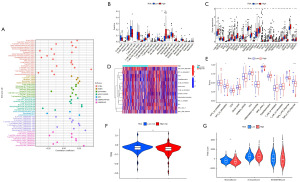
Assessment of CMRLs’ drug sensitivity in HCC
The GDSC database was applied for the analysis of drug sensitivity. Lower IC50 values indicated higher drug sensitivity. Our analysis revealed that the low-risk group exhibited higher IC50 values for eight chemotherapy drugs: fluorouracil, afatinib, alpelisib, cedranib, crizotinib, erlotinib, gefitinib, and ipatasertib (P<0.05, Figure 9A-9H), suggesting that patients in the high-risk category could potentially obtain therapeutic advantages from these drugs.
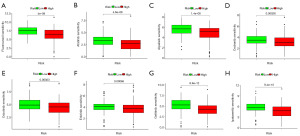
Relationship between TMN and CMRLs in HCC
The HCC patients were separated into high- and low-risk groups to facilitate further examination of the somatic mutation data. A waterfall chart showed that the most commonly mutated genes were TP53, CTNNB1, TTN, MUC16, PCLO, ALB, RYR2, APOB, LRP1B, CSMD3, XIRP2, ABCA13, OBSCN, FLG, HMCN1. In the high-risk groups, TP53 (39%) and CTNNB1 (30%) had higher mutational rates (Figure 10A), whereas TTN (23%) was more highly mutated in the low-risk groups (Figure 10B). According to somatic mutation data, we determined the TMB and found that the TMB of the low-risk group was significantly lower than that of the high-risk group (P=0.047) (Figure 10C). In the correlation analysis, there was a significant positive correlation between risk ratings and the TMB (R=0.18, P=0.00055) (Figure 10D). Finally, in the association assessment between TMB and the combined risk score of CMRLs on OS, high TMB was consistently associated with poor prognosis, indicating a significant synergistic effect between TMB and CMRLs (Figure 10E,10F).
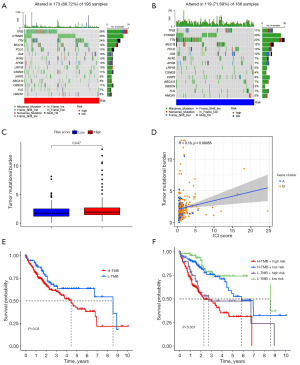
Discussion
Copper is a vital element that plays a crucial role in numerous biological processes within the human body, including the regulation of conditions such as anemia, metabolic disorders, and cancer (26). Substantial evidence has demonstrated that CMRGs are reliable predictors of survival outcomes in cancer patients. Additionally, treatment strategies targeting copper or proteins involved in copper metabolism have already been established (23,27-29). However, there is little information about its role in liver cancer. Liver cancer is one of the primary malignancies worldwide (30). Despite the continuous exploration and great progress made in medicine and treatment methods, HCC still remains a major concern for global health due to its persistently high incidence and mortality rates (30,31). In our study, we constructed a prognostic model after univariate Cox regression and LASSO analyses, to clarify the possible connection between copper metabolism and the prognosis of liver cancer and broaden the immunotherapy prospects of liver cancer.
In order to further illuminate the role of CMRLs in the prognosis and immune landscape of liver cancer, we ultimately chose to construct a prognostic risk score for liver cancer patients based on the expression levels of four independent prognostic CMRLs (PRRT3-AS1, AC108752.1, AC092115.3, and AL031985.3). According to the risk score, liver cancer patient samples were divided into different independent prognostic groups. Existing evidence suggests that PRRT3-AS1 and AL031985.3 can serve as the prognostic markers for liver cancer. Through autophagy-related, ferroptosis-related, or immune-related pathways, PRRT3-AS1 has been shown to be a predictive factor for the prognosis of HCC patients (32-34). In addition, it has been confirmed that AL031985.3 not only can participate in the progression of liver cancer through CD4+ conventional T cells-related and ferroptosis-related pathways, but serve as a prognostic marker (35,36). However, the potential mechanisms of AC108752.1 and AC092115.3 in the progression of liver cancer have not been reported yet. The accuracy of the prognostic risk score model constructed for liver cancer patients was demonstrated by the ROC curve and calibration curve. Ultimately, we created a nomogram integrating clinical information and the prognostic risk score model and uncovered the relationships between four CMRLs and immune infiltration, drug response, and tumor mutation, further expanding the predictive ability and clinical application of the prognostic risk score model and enabling personalized immunotherapy and precise targeted therapy for liver cancer patients.
The TME contains tumor stem cells and molecules that promote the occurrence and progression of tumors. Therefore, regulating certain specific molecules and cells in the TME can help control malignant tumors and achieve positive tumor outcomes (37). The immune microenvironment, composed of various immune cells, is not only an important component of the TME, but its predictive value as a biomarker for tumors and immune efficacy has been affirmed (38). It was found that monocytes and macrophages M0 were different between the high- and low-risk groups after comparing the immune infiltration in the two groups. Research has found that PKM2 can upregulate transcription factors by inducing metabolic reprogramming and STAT3 phosphorylation in monocytes, leading to monocyte differentiation into macrophages and affecting the TME of liver cancer (39). Meanwhile, macrophages M0 have also been evaluated to be an independent prognostic risk factor for liver cancer (40). Macrophages are vital for homeostasis and immunity (41). However, tumor-associated macrophages (TAMs) hijack proinflammatory pathways for immune depression, building an environment that supports cancer growth and metastasis at primary and secondary sites (42). Increasing of macrophage M0 in liver cancer patients in the high-risk HCC patients corroborates the confirmed conclusion above. At the same time, we further improved our research on immune checkpoint genes. The increased expression of the immune checkpoint genes mentioned may be closely related to the recurrence and poor prognosis of liver cancer. We hypothesize that using the immune risk score mentioned above allows physicians to better evaluate the prognosis of patients based on their immune checkpoint gene expression and adjust their immune treatment plans in real-time.
Somatic mutations are one of the common mechanisms of HCC pathway dysfunction and can serve as a biomarker to predict the response of liver cancer patients to specific treatments (43). In the high-risk group, the somatic mutation rate of TP53 and CTNNB1 were significantly higher than that of the low-risk group (TP53: 39% vs. 11%, CTNNB1: 30% vs. 20%). TP53 mutation is one of the most common mutations in malignant tumors. Wang et al. summarized previous research and confirmed that tumor suppressor gene TP53 mutations exist in approximately 50% of human cancers (44). The protein encoded by mutated TP53 is dysfunctional and continuously accumulates in the nucleus, which is considered a highly specific marker of malignant tumors (45). This could be one of the reasons for the poor prognosis of liver cancer, but more studies are still required to confirm the potential mechanism between the TP53 and HCC. CTNNB1 mutation within TME is primarily associated with a lower proportion of activated immune cells, a higher proportion of depleted immune cells, reduced expression of immune-stimulating and checkpoint molecules, decreased activation of immune-related pathways, and enhanced activation of pathways linked to tumor growth or drug resistance, leading to immunotherapy for liver cancer becoming ineffective (46). Thus, the higher frequency of TP53 and CTNNB1 mutations in high-risk populations for HCC may be related to imbalanced copper metabolism.
Recent studies have highlighted that copper metabolism can modulate key signaling pathways involved in tumor progression, such as regulating copper homeostasis, impacting cancer cell proliferation, apoptosis, and metastasis (7-9). In our research, the differential expression of CMRLs in tumors compared to normal tissues in HCC has been predominantly associated with crucial biological processes such as “mitotic sister chromatid segregation”, “negative regulation of mitotic nuclear division”, and “mitotic spindle checkpoint signaling”. These processes are crucial for maintaining genomic stability and proper cell division (47,48). Moreover, cellular components associated with these processes include “chromosomal region”, “chromosome, centromeric region”, and “kinetochore” KEGG pathway enrichment analysis highlighted pathways such as “cell cycle” and “oocyte meiosis”. These findings underscore that CMRLs play a vital role in chromosomal segregation and cell cycle regulation. Additionally, their involvement in the immune landscape presents new avenues for immunotherapy in HCC patients. Future research should focus on elucidating the precise mechanisms by which CMRLs influence tumor biology.
Although our study has clinical significance in evaluating the prognosis of HCC patients and guiding immunotherapy regimens, it also has some drawbacks. Firstly, the research was only evaluated on the TCGA dataset. We attempted to validate with data from other databases including the Gene Expression Omnibus and International Cancer Genome Consortium, but the limitations of existing technology and data biases prevented us from obtaining accurate lncRNA data. Secondly, due to limitations in research funding and laboratory conditions, we did not conduct further in vivo and in vitro studies on the discovered mechanisms through biological experiments. Finally, the model we have established still requires extensive clinical trials to confirm its predictive efficacy.
Conclusions
In summary, we constructed a highly specific and sensitive prediction model based on four CMRLs to predict the OS of HCC patients. To expand the application of the model, a nomogram was constructed by combining patient clinical characteristics and risk scores. Besides, we further explored its TME, tumor mutations, and so on. More importantly, our research can also predict the efficacy of individualized immunotherapy, which is of not only great significance for guiding immunotherapy in clinical practice but also paving the way for the development of precise immunotherapy for liver cancer.
Acknowledgments
Funding: This study was supported by
Footnote
Reporting Checklist: The authors have completed the TRIPOD reporting checklist. Available at https://tcr.amegroups.com/article/view/10.21037/tcr-24-611/rc
Peer Review File: Available at https://tcr.amegroups.com/article/view/10.21037/tcr-24-611/prf
Conflicts of Interest: All authors have completed the ICMJE uniform disclosure form (available at https://tcr.amegroups.com/article/view/10.21037/tcr-24-611/coif). The authors have no conflicts of interest to declare.
Ethical Statement: The authors are accountable for all aspects of the work in ensuring that questions related to the accuracy or integrity of any part of the work are appropriately investigated and resolved. The study was conducted in accordance with the Declaration of Helsinki (as revised in 2013).
Open Access Statement: This is an Open Access article distributed in accordance with the Creative Commons Attribution-NonCommercial-NoDerivs 4.0 International License (CC BY-NC-ND 4.0), which permits the non-commercial replication and distribution of the article with the strict proviso that no changes or edits are made and the original work is properly cited (including links to both the formal publication through the relevant DOI and the license). See: https://creativecommons.org/licenses/by-nc-nd/4.0/.
References
- Brown ZJ, Tsilimigras DI, Ruff SM, et al. Management of Hepatocellular Carcinoma: A Review. JAMA Surg 2023;158:410-20. [Crossref] [PubMed]
- Bray F, Laversanne M, Sung H, et al. Global cancer statistics 2022: GLOBOCAN estimates of incidence and mortality worldwide for 36 cancers in 185 countries. CA Cancer J Clin 2024;74:229-63. [Crossref] [PubMed]
- Xia C, Dong X, Li H, et al. Cancer statistics in China and United States, 2022: profiles, trends, and determinants. Chin Med J (Engl) 2022;135:584-90. [Crossref] [PubMed]
- Jean K, Tawheed A, Luong Nguyen LB, et al. Changes in Presentation, Treatment, and Survival of Patients with Hepatocellular Carcinoma in Damietta, Egypt, 2007-2019: A Retrospective Monocentric Cohort Study. J Hepatocell Carcinoma 2023;10:99-111. [Crossref] [PubMed]
- Devarbhavi H, Asrani SK, Arab JP, et al. Global burden of liver disease: 2023 update. J Hepatol 2023;79:516-37. [Crossref] [PubMed]
- Oura K, Morishita A, Tani J, et al. Tumor Immune Microenvironment and Immunosuppressive Therapy in Hepatocellular Carcinoma: A Review. Int J Mol Sci 2021;22:5801. [Crossref] [PubMed]
- Ge EJ, Bush AI, Casini A, et al. Connecting copper and cancer: from transition metal signalling to metalloplasia. Nat Rev Cancer 2022;22:102-13. [Crossref] [PubMed]
- Jiang Y, Huo Z, Qi X, et al. Copper-induced tumor cell death mechanisms and antitumor theragnostic applications of copper complexes. Nanomedicine (Lond) 2022;17:303-24. [Crossref] [PubMed]
- Lelièvre P, Sancey L, Coll JL, et al. The Multifaceted Roles of Copper in Cancer: A Trace Metal Element with Dysregulated Metabolism, but Also a Target or a Bullet for Therapy. Cancers (Basel) 2020;12:3594. [Crossref] [PubMed]
- Scheiber I, Dringen R, Mercer JF. Copper: effects of deficiency and overload. Met Ions Life Sci 2013;13:359-87. [Crossref] [PubMed]
- Saporito-Magriñá CM, Musacco-Sebio RN, Andrieux G, et al. Copper-induced cell death and the protective role of glutathione: the implication of impaired protein folding rather than oxidative stress. Metallomics 2018;10:1743-54. [Crossref] [PubMed]
- Davis CI, Gu X, Kiefer RM, et al. Altered copper homeostasis underlies sensitivity of hepatocellular carcinoma to copper chelation. Metallomics 2020;12:1995-2008. [Crossref] [PubMed]
- Capriotti G, Piccardo A, Giovannelli E, et al. Targeting Copper in Cancer Imaging and Therapy: A New Theragnostic Agent. J Clin Med 2022;12:223. [Crossref] [PubMed]
- Shanbhag VC, Gudekar N, Jasmer K, et al. Copper metabolism as a unique vulnerability in cancer. Biochim Biophys Acta Mol Cell Res 2021;1868:118893. [Crossref] [PubMed]
- Li Y. Copper homeostasis: Emerging target for cancer treatment. IUBMB Life 2020;72:1900-8. [Crossref] [PubMed]
- da Silva DA, De Luca A, Squitti R, et al. Copper in tumors and the use of copper-based compounds in cancer treatment. J Inorg Biochem 2022;226:111634. [Crossref] [PubMed]
- Zhang Y, Li X, Li X, et al. Comprehensive analysis of cuproptosis-related long noncoding RNA immune infiltration and prediction of prognosis in patients with bladder cancer. Front Genet 2022;13:990326. [Crossref] [PubMed]
- Wang H, Lv Z, Xia H, et al. Cuproptosis-related long noncoding RNAs predicts overall survival and reveal immune microenvironment of bladder cancer. Heliyon 2023;9:e21153. [Crossref] [PubMed]
- Zhang G, Sun J, Zhang X. A novel Cuproptosis-related LncRNA signature to predict prognosis in hepatocellular carcinoma. Sci Rep 2022;12:11325. [Crossref] [PubMed]
- Yan H, Bu P. Non-coding RNA in cancer. Essays Biochem 2021;65:625-39. [Crossref] [PubMed]
- Park EG, Pyo SJ, Cui Y, et al. Tumor immune microenvironment lncRNAs. Brief Bioinform 2022;23:bbab504. [Crossref] [PubMed]
- Agostini M, Mancini M, Candi E. Long non-coding RNAs affecting cell metabolism in cancer. Biol Direct 2022;17:26. [Crossref] [PubMed]
- Chang W, Li H, Zhong L, et al. Development of a copper metabolism-related gene signature in lung adenocarcinoma. Front Immunol 2022;13:1040668. [Crossref] [PubMed]
- Xu L, Deng C, Pang B, et al. TIP: A Web Server for Resolving Tumor Immunophenotype Profiling. Cancer Res 2018;78:6575-80. [Crossref] [PubMed]
- Mariathasan S, Turley SJ, Nickles D, et al. TGFβ attenuates tumour response to PD-L1 blockade by contributing to exclusion of T cells. Nature 2018;554:544-8. [Crossref] [PubMed]
- Ren F, Logeman BL, Zhang X, et al. X-ray structures of the high-affinity copper transporter Ctr1. Nat Commun 2019;10:1386. [Crossref] [PubMed]
- Zhang S, Zhang L, Lu H, et al. A cuproptosis and copper metabolism-related gene prognostic index for head and neck squamous cell carcinoma. Front Oncol 2022;12:955336. [Crossref] [PubMed]
- Liu H, Tang T. Pan-cancer genetic analysis of cuproptosis and copper metabolism-related gene set. Front Oncol 2022;12:952290. [Crossref] [PubMed]
- Akagawa M. Protein carbonylation: molecular mechanisms, biological implications, and analytical approaches. Free Radic Res 2021;55:307-20. [Crossref] [PubMed]
- Chidambaranathan-Reghupaty S, Fisher PB, Sarkar D. Hepatocellular carcinoma (HCC): Epidemiology, etiology and molecular classification. Adv Cancer Res 2021;149:1-61. [Crossref] [PubMed]
- Vogel A, Meyer T, Sapisochin G, et al. Hepatocellular carcinoma. Lancet 2022;400:1345-62. [Crossref] [PubMed]
- Kong W, Wang X, Zuo X, et al. Development and Validation of an Immune-Related lncRNA Signature for Predicting the Prognosis of Hepatocellular Carcinoma. Front Genet 2020;11:1037. [Crossref] [PubMed]
- Zhang Z, Zhang W, Wang Y, et al. Construction and Validation of a Ferroptosis-Related lncRNA Signature as a Novel Biomarker for Prognosis, Immunotherapy and Targeted Therapy in Hepatocellular Carcinoma. Front Cell Dev Biol 2022;10:792676. [Crossref] [PubMed]
- Yang S, Zhou Y, Zhang X, et al. The prognostic value of an autophagy-related lncRNA signature in hepatocellular carcinoma. BMC Bioinformatics 2021;22:217. [Crossref] [PubMed]
- Chen ZA, Tian H, Yao DM, et al. Identification of a Ferroptosis-Related Signature Model Including mRNAs and lncRNAs for Predicting Prognosis and Immune Activity in Hepatocellular Carcinoma. Front Oncol 2021;11:738477. [Crossref] [PubMed]
- Zhu L, Zhang XP, Xu S, et al. Identification of a CD4+ conventional T cells-related lncRNAs signature associated with hepatocellular carcinoma prognosis, therapy, and tumor microenvironment. Front Immunol 2023;13:1111246. [Crossref] [PubMed]
- Arneth B. Tumor Microenvironment. Medicina (Kaunas) 2019;56:15. [Crossref] [PubMed]
- Zhang Z, Qiu X, Yan Y, et al. Evaluation of Ferroptosis-related Gene AKR1C1 as a Novel Biomarker Associated with the Immune Microenvironment and Prognosis in Breast Cancer. Int J Gen Med 2021;14:6189-200. [Crossref] [PubMed]
- Hou PP, Luo LJ, Chen HZ, et al. Ectosomal PKM2 Promotes HCC by Inducing Macrophage Differentiation and Remodeling the Tumor Microenvironment. Mol Cell 2020;78:1192-1206.e10. [Crossref] [PubMed]
- You JA, Gong Y, Wu Y, et al. WGCNA, LASSO and SVM Algorithm Revealed RAC1 Correlated M0 Macrophage and the Risk Score to Predict the Survival of Hepatocellular Carcinoma Patients. Front Genet 2022;12:730920. [Crossref] [PubMed]
- Chen D, Zhang X, Li Z, et al. Metabolic regulatory crosstalk between tumor microenvironment and tumor-associated macrophages. Theranostics 2021;11:1016-30. [Crossref] [PubMed]
- Christofides A, Strauss L, Yeo A, et al. The complex role of tumor-infiltrating macrophages. Nat Immunol 2022;23:1148-56. [Crossref] [PubMed]
- Garcia-Lezana T, Lopez-Canovas JL, Villanueva A. Signaling pathways in hepatocellular carcinoma. Adv Cancer Res 2021;149:63-101. [Crossref] [PubMed]
- Wang Z, Strasser A, Kelly GL. Should mutant TP53 be targeted for cancer therapy? Cell Death Differ 2022;29:911-20. [Crossref] [PubMed]
- Dowell SP, Wilson PO, Derias NW, et al. Clinical utility of the immunocytochemical detection of p53 protein in cytological specimens. Cancer Res 1994;54:2914-8. [PubMed]
- Chen L, Zhou Q, Liu J, et al. CTNNB1 Alternation Is a Potential Biomarker for Immunotherapy Prognosis in Patients With Hepatocellular Carcinoma. Front Immunol 2021;12:759565. [Crossref] [PubMed]
- Wassmann K. Sister chromatid segregation in meiosis II: deprotection through phosphorylation. Cell Cycle 2013;12:1352-9. [Crossref] [PubMed]
- Villarroya-Beltri C, Malumbres M. Mitotic Checkpoint Imbalances in Familial Cancer. Cancer Res 2022;82:3432-4. [Crossref] [PubMed]