Potential role of TWIST1 and its methylation in bladder urothelial carcinoma
Highlight box
Key findings
• Hypermethylation of both the preoperative and postoperative TWIST1 promoters is useful as a biomarker for monitoring bladder urothelial carcinoma (BLCA) recurrence, particularly when considering the methylation status of specific CpG sites. Additionally, TWIST1 expression, promoter methylation, and immune infiltration immunoreactive markers correlated significantly in BLCA.
What is known and what is new?
• TWIST1 expression was down-regulated in BLCA, which was negatively correlated with DNA methylation. The association between TWIST1 promoter hypermethylation and the progression, staging, grading, and recurrence of BLCA is highly significant.
• This study is the first to investigate the methylation status of TWIST1 CpG sites in BLCA using bioinformatics methods, as well as the correlation between TWIST1 expression, promoter methylation, and immune infiltration markers.
What is the implication, and what should change now?
• TWIST1 has the potential to influence tumor progression and patient prognosis by regulating immune cell infiltration. More in-depth exploration of the relationship between TWIST1 hypermethylation and the immune system is needed in the future, hopefully providing new insights into the treatment of BLCA.
Introduction
The most frequent pathologic type of bladder cancer is bladder urothelial carcinoma (BLCA), tenth in the world in terms of incidence, with approximately 550,000 people suffering from the disease each year and nearly 200,000 people dying from BLCA in 2018 alone (1). Globally, 81,400 new cases and 17,980 fatalities were expected by 2020, for a 2.4% incidence rate (2). Although age-standardized incidence rates show considerable variation across geographic regions, they are expected to continue to rise over the next decade (3). As a highly sensitive method of diagnosing bladder cancer, cystoscopy is currently the gold standard, but it can be costly and uncomfortable for patients. Despite the fact that urine cytologic analysis is the most often used noninvasive approach for cancer detection, it is not very sensitive, particularly in low-grade migratory cell carcinomas, so this method lacks the sensitivity and specificity that are widely used (4).
There is a growing body of evidence suggesting that genetic and epigenetic changes play a crucial role in the development of tumors. DNA methylation causes abnormal expression of tumor-related genes, which is linked to tumor growth, recurrence, and a poor prognosis (5). Furthermore, it has been proposed that DNA methylation can be used as a biological biomarker to detect and predict cancer by stratifying patients based on their susceptibility to various clinical outcomes and treatment responses (6). Abnormal DNA methylation plays a critical role in the development of bladder cancer. Ruan et al. (7) demonstrated that urinary DNA methylation is closely associated with the early detection and recurrence monitoring of bladder cancer. Similarly, Chen et al. (8) concluded that urinary DNA methylation is a rapid, non-invasive, and promising method for detecting and monitoring this disease. Additionally, recent studies have shown that DNA methylation can regulate the tumor microenvironment, with PD-L1 methylation identified as an independent predictor of survival in bladder cancer patients (9,10). Thus, DNA methylation is a good predictive library. Epithelial-mesenchymal transition (EMT) is the most critical process in the initiation of cancer metastasis. The transcription factor TWIST1, known to promote EMT, has been discovered as a possible therapeutic target for cancer. It is widely acknowledged that TWIST1 undergoes hypermethylation in various tumor types, including colorectal (11) and breast cancers (12). To further investigate the role of the TWIST1 gene in BLCA, this study employed bioinformatics analysis and validated its findings through clinical cases. The aim was to establish a foundation for early diagnosis, prognostic assessment, and potential therapeutic interventions in BLCA. We present this article in accordance with the REMARK reporting checklist (available at https://tcr.amegroups.com/article/view/10.21037/tcr-24-1029/rc).
Methods
Downloading data from The Cancer Genome Atlas (TCGA) and Gene Expression Omnibus (GEO) database
The TCGA database website (https://portal.gdc.cancer.gov/) was utilized to collect RNA sequencing (RNA-seq) (level 3) data for BLCA as well as associated clinical information. Following that, we retrieved the GSE13507 and GSE37815 gene chip datasets from the GEO database (https://www.ncbi.nlm.nih.gov/geo/) and downloaded the data format for MINiML.
University of Alabama at Birmingham (UALCAN) database analysis
UALCAN (https://ualcan.path.uab.edu/), a comprehensive website that contains gene expression and survival analysis across 33 distinct tumor types in the TCGA (13). TWIST1 expression and promoter methylation profiles based on the patient’s individual cancer stage, gender, age, smoking status, and ethnicity were examined in this study using the UALCAN database.
Prognostic analysis
Kaplan-Meier survival curves were created by the R software package. BLCA patients were divided into high and low groups based on TWIST1 messenger RNA (mRNA) optimized grouping thresholds.
Gene Expression Profiling Interactive Analysis 2 (GEPIA2) database
The GEPIA2 website (http://gepia2.cancer-pku.cn/) contains RNA-seq expression data from 9,736 tumor samples and 8,587 normal samples from the TCGA and Genotype-Tissue Expression (GTEx) projects, providing an effective platform for biologists and clinicians to process comprehensive and complex data (14). In this study, GEPIA2 was used to analyze survival heat maps.
LinkedOmics database
The LinkedOmics database (http://www.linkedomics.org/login.php) is a database of protein sequences and functions. A significant cohort of 11,158 TCGA patients is included in this database, which comprises clinical and multi-omics data for 32 distinct cancer types (15). To assess the co-expression of TWIST1, a statistical analysis was conducted employing Pearson correlation coefficients, and the outcomes were visually depicted through volcano and heat maps. Additionally, gene set enrichment analysis (GSEA) (https://www.gsea-msigdb.org/gsea/index.jsp) was used to identify enriched functional modules within the Gene Ontology (GO) biological processes (BPs) and Kyoto Encyclopedia of Genes and Genomes (KEGG) databases.
cBio Cancer Genomics Portal (c-BioPortal) database analysis
The c-BioPortal (http://cbioportal.org) makes extensive cancer genomic datasets available (16). The c-BioPortal was employed in this research to investigate the relationship between TWIST1 mRNA expression and methylation in BLCA, which was visualized using scatter plots. Additionally, TWIST1 methylation and survival analyses were carried out utilizing the DNA Methylation Information Visualization Database (DNMIVD) database (http://119.3.41.228/dnmivd/index/).
DNA methylation of prognosis-associated TWIST1 genes
MethSurv (https://biit.cs.ut.ee/methsurv/) is a visual methylation analysis tool built on 450K data in the TCGA set. The tool incorporates a comprehensive dataset of 7,358 methylation data points pertaining to 25 distinct types of human cancers. Within the scope of this investigation, the MethSurv database was employed to examine the DNA methylation locations of TWIST1 within the TCGA dataset. Additionally, the predictive efficacy of TWIST1 CpG methylation sites was evaluated using overall survival (OS) as the indicator of survival outcome.
TWIST1 gene expression/methylation and tumor immune infiltration analysis
We were using the corr.test function of the R software package psych, and we calculated the Pearson’s correlation coefficient between TWIST1 and immune infiltration levels in BLCA. Using the Gene Set Cancer Analysis (GSCA; http://bioinfo.life.hust.edu.cn/GSCA) and Tumor-Immune System Interaction Database (TISIDB; http://cis.hku.hk/TISIDB) datasets, we investigated the connection between TWIST1 transcript expression/DNA methylation and cancer immune infiltration.
Clinical samples
All samples utilized in this study were sourced from the Harbin Medical University Cancer Hospital. The study enrolled a total of 718 cases, which included 44 homologous postoperative samples. Following the application of exclusion criteria, 95 cases and 11 postoperative samples were deemed ineligible, resulting in a total of 106 samples being excluded. Among these excluded samples, 56 cases (58.95%) were identified as recurrent bladder cancer cases. Ultimately, the study included a total of 579 cases that adhered to the protocol analysis set, along with 33 postoperative samples, resulting in a total of 612 samples. The clinicopathological data utilized in this study was collected from the hospital’s medical record system, and the follow-up duration spanned from the initial diagnosis until the patient’s ultimate visit or date of demise.
Here are the criteria for inclusion in this study: (I) participants must be at least 18 years old, regardless of gender. (II) Individuals provided their consent to take part in this clinical trial. (III) Participants who expressed willingness to undergo cystoscopy, urinary exfoliative cytology, and urinary ultrasonography due to hematuria or other clinical considerations. (IV) Urine exfoliative cytology, urologic CT plain enhancement scan, retrograde upper urinary tract urography, or diagnostic ureterocystoscopy with pathologically confirmed bladder cancer were used as diagnostic methods. (V) Patients with post-treatment recurrent bladder cancer diagnosed through clinical assessment. (VI) Patients diagnosed with various urologic tumors, including ureteral carcinoma, pyeloid carcinoma, bladder inverted papillary neoplasms, renal cell carcinoma, and prostate carcinoma. (VII) Patients presenting with benign conditions such as cystitis, prostatitis, and urethritis. (VIII) Individuals who have undergone cystoscopy and received a report indicating the absence of occupying lesions or individuals who have undergone urinary ultrasonography and received a report indicating the absence of bladder abnormalities, along with three consecutive urine exfoliative cytology diagnostic reports showing no presence of cancer cells. Exclusion criteria: one of the following is sufficient: (I) subjects who express a desire to withdraw from the clinical trial. (II) Subjects who, as determined by the investigator, are deemed unsuitable to continue participating in the ongoing clinical trial. (III) Subjects who fail to meet the inclusion criteria or are mistakenly enrolled despite meeting the exclusion criteria. (IV) In a comparative study involving the collection of samples from the same patient multiple times alongside a “gold standard”, only the initial sample is retained, while all subsequent samples are excluded. (V) Samples lack complete case information. (VI) Samples that do not meet quality control (QC) standards due to issues related to sample collection, preservation, transportation, and other factors result in the classification of failed samples in terms of sample QC. (VII) Samples that do not meet QC standards in terms of DNA quality. (VIII) Samples for which testing could not be concluded due to instrument or reagent quality issues or human factors such as sample contamination during experimental procedures.
Methylation-specific real-time fluorescence polymerase chain reaction (PCR)
Time of urine sample collection for comparative studies with the “gold standard”: (I) within 15 days before cystoscopy, after cystoscopy, or before chemotherapy or surgical treatment. (II) Three consecutive urine samples were collected at the time of the urine exfoliative cytopathologic examination and sent for testing. Urine samples are collected before cystoscopy or surgery, and postoperative urine samples are collected after surgery and within 2 weeks before discharge from the hospital. The initial morning urine, without restriction to midstream urine, was collected and preserved in a urine sample collection tube with a capacity ranging from 80 to 200 mL. Subsequently, the urine sample was promptly refrigerated at a temperature of 4 ℃ for a maximum duration of 24 hours. The nucleic acid extraction or purification reagent, a general-purpose product manufactured by Anhui Dajian Medical Technology Co., Ltd. (Wuhu, China), was employed as the first step, and nucleic acid extraction was conducted following the instructions provided in the kit. Then make 20 test rinses (add 9.6 mL of anhydrous ethanol to the rinse container, screw the cap on snugly, turn up and down 5 times, and thoroughly mix) and 50 test rinses (as above, but with 24 mL of anhydrous ethanol). Subsequently, the conversion solution was prepared by first subjecting it to centrifugation for 30 seconds. Next, added 750 µL of purified water and 210 µL of buffer. After that, the mixture was vortexed and agitated for ten minutes at room temperature to ensure complete dissolution. The bisulfite conversion process was completed according to the bisulfite conversion kit. Subsequently, the collected bisulfite-treated DNA (Bis-DNA) was promptly utilized for subsequent experiments. Ten µL of PCR mix, 5 µL of primer-probe mix, and 15 µL of total/test dose components were combined to make the PCR reaction solution. The resulting PCR reaction solution was thoroughly mixed and then dispensed at a volume of 15 µL per tube into PCR octuplex tubes, which were appropriately labeled. These tubes were then transferred to the sample processing area. Five µL of positive control, negative control, and bis-DNA from the samples under examination were added to the PCR octuplex tubes for PCR amplification (Fluorescence Quantitative PCR Instrument, ABI 7500, Thermo Fisher Scientific, Waltham, MA, USA).
Pyrophosphate sequencing
The urine samples were extracted using the nucleic acid extraction or purification reagent (general purpose) (Anhui Wuhu Measures No. 20190002) manufactured by Anhui Dajian Medical Technology Co., Ltd., following the provided instructions. Subsequently, the extracted DNA was converted into bisulfite using the “Kit I: Bisulfite Transformation Kit (Centrifugal Column)” from the “Human Twist1 Gene Methylation Detection Kit” also produced by Anhui Dajian Medical Technology Co., Ltd. Subsequently, the positive QC standard solution consisted of bladder cancer cell line DNA, which was measured and then diluted to 20 ng/µL in concentration using eluent to obtain the positive QC solution. The negative QC standard comprised normal human leukocyte DNA. The thermal cycler was programmed to undergo a series of temperature cycles: a 15-minute period at 95 ℃, 40 cycles of 30 seconds at 94 ℃, 30 seconds at 56 ℃, and 30 seconds at 72 ℃, and a 10-minute extension step at 72 ℃. It is essential to establish both negative and positive controls for each pyrophosphate sequencing experiment, and these controls were provided by Anhui Dajian Medical Technology Co., Ltd. Finally, sequencing was performed according to the instructions, and the Pyromark Q48 Autoprep program was run to export the results.
Statistical analysis
The non-parametric or Chi-square test was performed using the statistical software SPSS 24.0 to assess the relationship between the parameters. The diagnostic utility of TWIST1 methylation in predicting the mortality of BLCA was evaluated using the area under the receiver operating characteristic (ROC) curve (AUC). The selection of optimal cut-off points was based on the maximum sensitivity and specificity, as determined by the coordinates on the receiver ROC curve. The log-rank test was employed for survival analysis, while Spearman correlation analysis was used to investigate the connections between TWIST1 and immune infiltration as well as particular immune cell markers. A statistically significant outcome was defined as having a P value less than 0.05.
Ethics consideration
The study was conducted in accordance with the Declaration of Helsinki (as revised in 2013). The study was approved by Harbin Medical University Cancer Hospital (No. 2018-151-SJ) and informed consent was obtained from all individual participants.
Results
TWIST1 gene expression in BLCA
Tissue samples from 406 patients diagnosed with BLCA and 19 samples from normal tissues were obtained from the TCGA database. The ggplot2 package in R was employed for the analysis of these samples. The transcription level of TWIST1 was shown to be considerably reduced in BLCA tissues, according to the study (P<0.001) (Figure 1A); the UALCAN database also corroborated this finding (P<0.001) (Figure 1B). Similarly, based on the GEO database, TWIST1 mRNA expression was shown to be significantly reduced in BLCA patients in the GSE13507 (P<0.001) (9 normal samples, 82 recurrent samples, and 165 tissue samples from patients with primary BLCA) and GSE37815 (18 tissue samples from patients with BLCA and 5 normal tissue samples) datasets (P<0.05) (Figure 1C,1D). To further investigate the expression of TWIST1 mRNA levels in BLCA patients, TCGA-BLCA samples were subgrouped according to multiple clinicopathological characteristics [stage (Figure 1E), age (Figure 1F), gender (Figure 1G), race (Figure 1H), weight (Figure 1I), and smoking habits (Figure 1J)] using the UALCAN database. The final findings revealed a significant downregulation of TWIST1 transcript levels in BLCA patients compared to individuals without the condition. However, there were no differences in TWIST1 mRNA levels between the normal and female patients (Figure 1G). The aforementioned findings indicate that TWIST1 expression could potentially function as a diagnostic marker for BLCA.
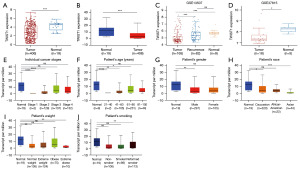
Prognostic impact of TWIST1 on BLCA patients
In the initial analysis, the interaction of clinicopathological variables and TWIST1 expression was examined using univariate Cox regression. The findings revealed that TWIST1 expression (P=0.006), age (P=2e−05), and tumor stage (P<0.001) were all found to be strongly related to prognosis (Figure 2A). However, in the subsequent multivariate Cox analysis, only age (P<0.001) and tumor stage (P<0.001) remained statistically significant (Figure 2B). To further determine the effect of TWIST1 on the prognosis of BLCA patients, the BLCA samples were divided into high and low-risk groups based on the median TWIST1 expression as the cut-off point; according to the Kaplan-Meier survival curves, there were no statistically significant variations in OS between the low- and high-risk groups (P>0.05) (Figure 2C). Furthermore, we generated ROC curves using the R package timeROC (Figure 2D), and the corresponding AUC consistently approximated 0.5, suggesting that the model’s predictive ability was limited. These results suggest that TWIST1 transcription levels may have limited prognostic value in patients with BLCA.
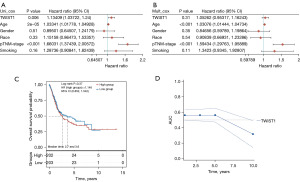
TWIST1 co-expression network in BLCA
To acquire a better understanding of the biological relevance of TWIST1 in BLCA, co-expression patterns for TWIST1 were discovered using the LinkedOmics database. The analysis revealed that 5,710 genes (represented by dark red dots) correlated favorably with TWIST1, whereas 3,712 genes (represented by dark green dots) displayed a negative correlation with it [false discovery rate (FDR) <0.01] (Figure 3A). Table available at https://cdn.amegroups.cn/static/public/tcr-24-1029-1.xlsx contains more information about the co-expressed genes. Figure 3B,3C showcase a heatmap illustrating the top 50 genes that are significantly positively and negatively linked with TWIST1. Among them, TWIST1 has a high positive association with AEBP1 expression (r=0.789, P=5.34e−88), COL1A1 (r=0.784, P=4.72e−86) and COL3A1 (r=0.782, P=1.96e−85), and was also associated with SSH3 expression (r=−0.466, P=2.26e−23), PLEKHH1 (r=−0.463, P=4.06e−23), and TRERF1 (r=−0.460, P=1.03e−22) were significantly negatively correlated. Notably, among the top 50 significantly positively associated genes, 23 had a high hazard ratio (HR) (P<0.05), while only 16 of the negatively linked genes showed a low HR (Figure S1). The GSEA found that TWIST1 co-expressed genes were mostly engaged in extracellular structural organization, leukocyte chemotaxis, and the regulation of skeletal and vascular system development. Furthermore, the KEGG pathway analysis demonstrated enrichment of hematopoietic cell profiles, complement and coagulation cascade reactions, cell adhesion molecules, and dilated cardiomyopathy (Figure 3D). These findings imply that TWIST1 influences cell migration.
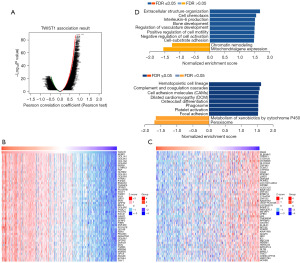
TWIST1 promoter hypermethylation correlates with clinicopathological parameters
DNA methylation patterns are established during early embryonic development and play a pivotal role in regulating gene expression through diverse mechanisms, thereby exerting influence on tumor progression and formation (17). To investigate this, we examined the alterations in TWIST1 promoter methylation levels in BLCA using the UALCAN database. It was found that TWIST1 promoter DNA hypermethylation in BLCA (Figure 4A). The TCGA-BLCA samples were further categorized based on various clinicopathological characteristics, including stage (Figure 4B), smoking habits (Figure 4C), age (Figure 4D), race (Figure 4E), gender (Figure 4F), and weight (Figure 4G). The analysis of these subgroups revealed that TWIST1 promoter methylation levels were considerably higher than those reported in normal participants.
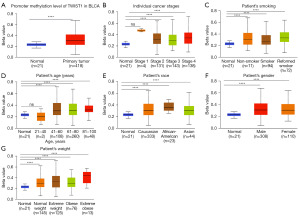
Diagnostic value of TWIST1 methylation in BLCA
The study contained 579 samples altogether, with 178 allocated to the case group, 304 to the control group, and 97 to the interference group (table available at https://cdn.amegroups.cn/static/public/tcr-24-1029-2.xlsx). Table 1 shows the clinicopathological features of the tumor specimens, indicating that the majority of cases were at stage 0a (56.74%) and non-invasive (56.74%). TWIST1 methylation frequencies in urine were 87.64% (156/178), and a total of 41 cases in the control and interference groups showed TWIST1 hypermethylation (86.03% specificity) (Table S1). In order to assess the reliability of TWIST1 as a predictor of BLCA, a sample of 76 patients diagnosed with BLCA was selected at random for pyrophosphate sequencing. Notably, pyrophosphate sequencing was found to have a 98.68% compliance rate with the methylation fluorescence PCR method, with a Kappa value of 0.957, indicating very high accuracy (Table S2).
Table 1
Parameters | Case | Control | Interference | Total |
---|---|---|---|---|
Total | 178 | 304 | 97 | 579 |
Methylated TWIST1 | 156 (87.64) | 34 (11.18) | 22 (22.68) | 212 (36.61) |
Gender | ||||
Male | 133 (74.72) | 128 (42.11) | 68 (70.10) | 329 (56.82) |
Female | 45 (25.28) | 176 (57.89) | 29 (29.90) | 250 (43.18) |
Age (years) | 64 [21–87] | 48 [20–77] | 61 [36–86] | – |
Tumor stage | – | – | – | |
Stage 0a | 101 (56.74) | |||
Stage I | 43 (24.16) | |||
Stage II | 14 (7.87) | |||
Stage III | 17 (9.55) | |||
Unclear | 3 (1.69) | |||
Tumor grade | – | – | – | |
Low grade | 62 (34.83) | |||
High grade | 60 (33.71) | |||
Unclear | 56 (31.46) | |||
Infiltration degree | – | – | – | |
No | 101 (56.74) | |||
Yes | 68 (38.20) | |||
Unclear | 9 (5.06) |
Data are presented as number, n (%), or median [min–max].
Prognostic value of the TWIST1 methylation in BLCA
All of the above results have illustrated that TWIST1 hypermethylation is strongly associated with the risk of BLCA. Additionally, our study revealed a substantial correlation between TWIST1 hypermethylation and tumor stage (P=0.02), pathological grade (P=0.001), and degree of infiltration (P=0.01) (Table 2). To examine the correlation between TWIST1 methylation and BLCA recurrence, a total of 33 cases from the case group were randomly selected to undergo repeat testing after their surgical procedure. Out of the 29 subjects who had TWIST1 hypermethylation before surgery, 15 still had hypermethylation after the operation, while 14 did not (Table S3). Additionally, four subjects who had TWIST1 hypomethylation before surgery maintained this status postoperatively. During the follow-up period, six patients experienced recurrence after surgery, and five of them had TWIST1 hypermethylation both before and after the operation. The study results indicate that postoperative TWIST1 hypermethylation can be a predictor of recurrence in patients with bladder cancer (P=0.03) (Table 2, Figure 5A). Additionally, our results demonstrate a strong link between OS in BLCA patients and TWIST1 hypermethylation (P<0.05) (Figure 5B). To further validate TWIST1 methylation in relation to BLCA patient prognosis, the prognostic value of methylation at each CpG locus was assessed individually by utilizing the MethSurv database. Remarkably, it was observed that the cg27013696 site of the TWIST1 promoter showed the highest level of DNA methylation (Figure 5C). Furthermore, out of the 34 CpGs situated within the promoter region of TWIST1, 11 were determined to possess substantial prognostic value for patients with BLCA through Kaplan-Meier survival analysis (Figure S2); the data pertaining to the remaining 23 CpGs can be found in Figure S3.
Table 2
Parameters | Total, n | TWIST1/postoperative-TWIST1, n (%) | P value | |
---|---|---|---|---|
+ | − | |||
Tumor stage | 0.02* | |||
Stage 0a | 101 | 83 (82.18) | 18 (17.82) | |
Stage I | 43 | 41 (95.35) | 2 (4.65) | |
Stage II | 14 | 14 (100.00) | 0 | |
Stage III | 17 | 17 (100.00) | 0 | |
Unclear | 3 | 1 (33.33) | 2 (66.67) | |
Tumor grade | 0.001* | |||
Low grade | 62 | 45 (72.58) | 17 (27.42) | |
High grade | 60 | 57 (95.00) | 3 (5.00) | |
Unclear | 56 | 54 (96.43) | 2 (3.57) | |
Infiltration degree | 0.01* | |||
No | 101 | 84 (83.17) | 17 (16.83) | |
Yes | 68 | 65 (95.59) | 3 (4.41) | |
Unclear | 9 | 7 (77.78) | 2 (22.22) | |
Tumor recurrence | 0.03* | |||
Yes | 7 | 6 (85.71) | 1 (14.29) | |
No | 26 | 9 (34.62) | 17 (65.38) |
*, P<0.05.
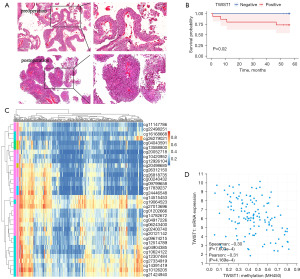
TWIST1 methylation is negatively correlated with its transcriptional expression and both are associated with immune infiltration
TWIST1 promoter methylation exhibited a negative correlation with its transcription level (r=−0.3, P=7.603e−4) (Figure 5D). The presence of tumor-infiltrating immune cells in the intricate microenvironment has been linked to the survival and biological parameters of BLCA patients (18). Notably, the degree of CD8+ T cell infiltration was shown to be substantially linked with prognosis (P=0.006) (Figure S4). TWIST1 expression and promoter methylation were further investigated in relation to B cells, CD4+ T cells, CD8+ T cells, central_memory, cytotoxic, dendritic cells, and effector_memory in BLCA. We conducted an analysis utilizing data obtained from the GSCA database for BLCA. The results revealed significant negative correlations with B cells (Cor =−0.18, P=1.35e−4) and effector memory (Cor =−0.18, P=1.89e−4). But with CD4+ T cells (Cor =0.15, P=2.40e−3), CD8+ T cells (Cor =0.05, P=0.27), central_memory (Cor =0.13, P=7.38e−3), cytotoxic (Cor =0.40, P=2.04e−17), and dendritic (Cor =0.26, P=4.58e−8) were positively correlated (Figure 6A). Interestingly, except for B cells, the link between TWIST1 methylation and immunological infiltration was exactly opposite to the one described above (Figure 6B).
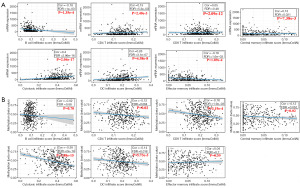
Correlation of TWIST1 transcript expression/DNA methylation with immune markers
To facilitate a thorough investigation into the correlation between immunological markers and TWIST1 transcript expression/methylation, we first employed the GEPIA2 database to scrutinize the top 20 immune-related genes that demonstrated positive or negative associations with TWIST1. The final findings revealed that FAP, PDGFRB, TGFB3, ANXA6, and EDNRA were the top five immune markers that positively correlated with TWIST1. Conversely, LPA, SRC, FAM3D, THRB, and RXRA were identified as the top five immune markers that exhibited a negative correlation with TWIST1 (Figure 7A). Upon further analysis utilizing the TISIDB online database, TWIST1 expression was found to significantly and favorably correlate with immune-related indicators associated with tumor-infiltrating lymphocytes (TILs) such as T helper 1 (Th1), regulatory T (Treg), immune stimulators [tumor necrosis factor ligand superfamily member 4 (TNFSF4)], and immunosuppressants [interleukin 10 (IL10)]. Furthermore, there is a weak to strong correlation with lymphocytes, immune stimulators, and other markers of immunosuppressants, as well as with immunological markers such as major histocompatibility complex (MHC) molecules, chemokines, and receptors (Figure 7B). Interestingly, the correlation between the immune markers and TWIST1 methylation and their correlation with the transcript was completely opposite (Figure 7C, Figure S5).
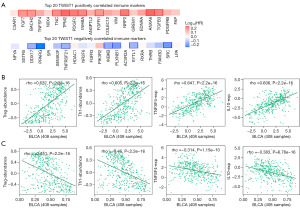
Discussion
Epigenetics pertains to the regulatory mechanisms governing gene expression, which impact transcription and translation processes without modifying the DNA sequence (19). While genetic factors contribute to the development of certain malignancies, it is epigenetic abnormalities that induce more profound and extensive disruptions in cellular signaling pathways, consequently exerting a greater influence on tumorigenesis and disease progression (20). Promoter methylation of DNA is a prevalent epigenetic modification, and the aberrant methylation of genes specific to tumors can be discovered early on, prior to clinical diagnosis (21). Promoter hypermethylation have been reported to play a key role in BLCA development and progression (22). The process of DNA methylation can have a direct influence on the engagement of DNA-binding proteins with their respective binding sites, exerting regulatory control on the expression of linked genes (23). Through urine detection, DNA methylation has the potential to be used as a non-invasive means of detecting and monitoring bladder cancer. Given its demonstrated high accuracy, reproducibility, and cost-effectiveness, it is anticipated that this technique will be incorporated into routine clinical protocols (24). Tumor-infiltrating immune cells contribute significantly to tumor progression and the body’s immune checkpoint blockage response (25). In addition to genetic and epigenetic changes, immunotherapies are a highly promising cancer treatment strategy currently available. The occurrence of aberrant TWIST1 expression is observed in multiple cancer types. Significantly, a positive correlation was observed between the expression of TWIST1 and the regulation of tumor immunity, as well as immune checkpoint mechanisms (26).
Using the TCGA database, we examined the role of TWIST1 and its promoter methylation in BLCA. TWIST1 expression is related to prognosis according to univariate Cox regression analysis. However, the multivariate Cox regression analysis did not yield consistent outcomes. These data show that more research is needed to establish TWIST1 as an independent risk factor for BLCA prognosis. Cancer emerges due to abnormal signaling within cellular systems responsible for controlling cell survival and apoptosis. TWIST1 is a basic helix-loop-helix transcription factor that demonstrates prominent expression in tissues derived from the mesoderm. In addition to regulating oncogene-induced cellular senescence and apoptosis (27), anomalous changes in TWIST1 can impart treatment resistance in cancer cells (28), self-renewal of tumor stem cells (29), and facilitate cancer cell invasion and metastasis (30). TWIST1’s biological role in BLCA is incomplete at the moment. Long non-coding RNA (lncRNA) urothelial carcinoma-associated 1 (UCA1) has been identified as a potential biomarker for the diagnosis and prognosis of bladder cancer. Liu et al. (31) discovered that UCA1 regulates guanine nucleotide production via TWIST1, which is mediated by the rate-limiting enzyme inosine monophosphate dehydrogenase 1/2. This regulation leads to guanine nucleotide metabolism reprogramming, resulting in increased bladder cancer cell proliferation, migration, and invasion. In recent years, it has also been found that ubiquitin-specific protease 5 (USP5) is a binding chaperone for TWIST1 and that the USP5-TWIST1 axis is a novel regulatory mechanism driving bladder cancer progression (32). In order to explore other potential biological functions of TWIST1, we utilized the LinkedOmics database. Our research has identified numerous genes that exhibit positive associations with TWIST1, including AEBP1, COL1A1, and COL3A1. AEBP1 functions as a pro-inflammatory mediator that activates NF-κB signaling, ultimately facilitating tumor cell invasion and metastasis (33). COL1A1 and COL3A1, members of the collagen family, play roles in EMT, have strong connections with immune cells such as CD8+ T cells, CD4+ T cells, and dendritic cells, and exhibit potential as molecular markers for prognosticating in pan-cancerous (34,35). Hu et al. (36) found a significant negative correlation between TWIST1 and SSH3, PLEKHH, and TRERF1. SSH3 belongs to the phosphatase family and enhances invasion and metastasis of colorectal cancer cells. Additionally, PLEKHH1 functions as an effector of EphB2, EphB2-ephrinB signaling pathways crucial for embryonic development (37). TRERF1 plays a key role in steroid hormone production, and this hormone has a potent anti-inflammatory and immunosuppressive effect (38). The roles of all of these genes in BLCA are currently unknown, and the interaction of TWIST1 with them has the potential to be a potential target for BLCA therapy. In addition, through enrichment analysis of co-expressed genes, we observed a significant association between TWIST1 and cell adhesion, indicating a potential impact on cell migration. TWIST1 plays a pivotal role in orchestrating cell migration and tissue remodeling during embryonic development. In the context of carcinogenesis, its function is not to facilitate cellular transformation but rather to instigate aberrant EMT within primary tumors, mirroring its developmental role (39). Moreover, it has been observed that tumor cells overexpressing TWIST1 have the capability to undergo transdifferentiation into endothelial cells, thereby actively contributing to the angiogenic process (40). To summarize, TWIST1 serves as a crucial regulator of gene expression, exerting significant biological impacts. Nevertheless, further mechanisms need to be verified experimentally.
An increasing amount of work examines the function of DNA methylation in BLCA, with many studies highlighting its correlation with tumor grade and aggressiveness (41,42). When selecting biomarkers for BLCA, it is crucial to consider both specificity and sensitivity. Friedrich et al. (43) have identified certain methylation markers, such as RASSF1A, EDNRB, and TNFRSF25, which should not be used for bladder cancer detection due to their methylation in normal controls. TWIST1 was shown to have a sensitivity of 87.64% and a specificity of 86.03% in this investigation. Notably, the sensitivity and specificity were both greater than with urine cytology. Our findings demonstrate that TWIST1 promoter DNA hypermethylation in BLCA tissues is inversely associated with transcript levels and significantly correlates with tumor progression, patient demographics, and various clinical parameters. Similarly, our clinical sample analysis reveals a substantial relationship between TWIST1 methylation and the development, stage, and grade of BLCA. TWIST1 promoter hypermethylation appears to be a possible biomarker for the diagnosis and progression of BLCA. Furthermore, our study indicates a substantial correlation between hypermethylation of the TWIST1 promoter and recurrence in patients with BLCA, which aligns with the findings reported by El Azzouzi et al. (22). Additionally, this study identified a link between hypermethylation of the TWIST1 promoter and OS in BLCA patients. The methylation status of TWIST1 promoter CpG sites in TCGA was investigated using the MethSurv database, revealing that 11 CpG sites exhibited a significant correlation with the OS of BLCA. These findings suggest that these specific CpG sites may serve as prognostic factors for BLCA and potentially function as binding sites for various transcription factors.
The relationship between cancer and the immune response is inherently interconnected. The immune system suppresses tumors by acting as an external agent, but it can also encourage cancer development (44). The identification of infiltrating immune cells as pivotal regulators of tumor development and progression has been established, with TWIST1 exhibiting immunomodulatory properties, including the regulation of macrophage activity and the promotion of dendritic cell function (45). The present study conducted immune cell differential and correlation analyses, revealing a significant association between CD8+ T immune cells within the BLCA tumor microenvironment and prognosis. Furthermore, the study found a link between TWIST1 mRNA expression, its promoter methylation, and a variety of immune cell infiltrates (such as macrophages, B cells, CD4+ T cells, and CD8+ T cells). In addition, the presence of TWIST1 mRNA and DNA methylation exhibited significant associations with diverse lymphocytes, immunomodulators, immunosuppressants, as well as the MHC molecule, chemokines and receptors documented in TISIDB. This finding suggests a close link between TWIST1 and immune cells, specifically T cells. Consequently, our investigation offers a novel avenue for exploring the involvement of TWIST1 in the context of BLCA. This study, in fact, possesses certain limitations, as we have merely conducted a preliminary investigation into the association between TWIST1 and immunity through bioinformatics techniques. There was an overall lack of relevant experimental evidence (PCR, western blot, or immunohistochemistry) to confirm the link between TWIST1 and indicators of immune inflammation. Consequently, further research and exploration are imperative to address the deficiencies above.
Conclusions
To conclude, our findings show that TWIST1 methylation may play a role in BLCA. TWIST1 transcript levels are down-regulated in BLCA and negatively correlate with TWIST1 promoter methylation. TWIST1 promoter hypermethylation exhibits a strong correlation with the progression, staging, grading, and recurrence of BLCA. At the same time, the methylation status of this specific locus holds promise as a potential prognostic marker for BLCA patients. Furthermore, the levels of the TWIST1 transcript and its promoter methylation are strongly linked to immune cell infiltration in BLCA. Mechanistically, it is plausible that TWIST1 promoter hypermethylation modulates tumor progression or patient prognosis through the regulation of immune cell infiltration.
Acknowledgments
Funding: This work was supported by grants from
Footnote
Reporting Checklist: The authors have completed the REMARK reporting checklist. Available at https://tcr.amegroups.com/article/view/10.21037/tcr-24-1029/rc
Data Sharing Statement: Available at https://tcr.amegroups.com/article/view/10.21037/tcr-24-1029/dss
Peer Review File: Available at https://tcr.amegroups.com/article/view/10.21037/tcr-24-1029/prf
Conflicts of Interest: All authors have completed the ICMJE uniform disclosure form (available at https://tcr.amegroups.com/article/view/10.21037/tcr-24-1029/coif). The authors have no conflicts of interest to declare.
Ethical Statement: The authors are accountable for all aspects of the work in ensuring that questions related to the accuracy or integrity of any part of the work are appropriately investigated and resolved. The study was conducted in accordance with the Declaration of Helsinki (as revised in 2013). The study was approved by Harbin Medical University Cancer Hospital (No. 2018-151-SJ) and informed consent was obtained from all individual participants.
Open Access Statement: This is an Open Access article distributed in accordance with the Creative Commons Attribution-NonCommercial-NoDerivs 4.0 International License (CC BY-NC-ND 4.0), which permits the non-commercial replication and distribution of the article with the strict proviso that no changes or edits are made and the original work is properly cited (including links to both the formal publication through the relevant DOI and the license). See: https://creativecommons.org/licenses/by-nc-nd/4.0/.
References
- Richters A, Aben KKH, Kiemeney LALM. The global burden of urinary bladder cancer: an update. World J Urol 2020;38:1895-904. [Crossref] [PubMed]
- Lenis AT, Lec PM, Chamie K, et al. Bladder Cancer: A Review. JAMA 2020;324:1980-91. [Crossref] [PubMed]
- Wong MCS, Fung FDH, Leung C, et al. The global epidemiology of bladder cancer: a joinpoint regression analysis of its incidence and mortality trends and projection. Sci Rep 2018;8:1129. [Crossref] [PubMed]
- Lopez-Beltran A, Cheng L, Gevaert T, et al. Current and emerging bladder cancer biomarkers with an emphasis on urine biomarkers. Expert Rev Mol Diagn 2020;20:231-43. [Crossref] [PubMed]
- Locke WJ, Guanzon D, Ma C, et al. DNA Methylation Cancer Biomarkers: Translation to the Clinic. Front Genet 2019;10:1150. [Crossref] [PubMed]
- Miozzo M, Vaira V, Sirchia SM. Epigenetic alterations in cancer and personalized cancer treatment. Future Oncol 2015;11:333-48. [Crossref] [PubMed]
- Ruan W, Chen X, Huang M, et al. A urine-based DNA methylation assay to facilitate early detection and risk stratification of bladder cancer. Clin Epigenetics 2021;13:91. [Crossref] [PubMed]
- Chen X, Zhang J, Ruan W, et al. Urine DNA methylation assay enables early detection and recurrence monitoring for bladder cancer. J Clin Invest 2020;130:6278-89. [Crossref] [PubMed]
- Zhang MW, Fujiwara K, Che X, et al. DNA methylation in the tumor microenvironment. J Zhejiang Univ Sci B 2017;18:365-72. [Crossref] [PubMed]
- Xu J, Wei L, Liu H, et al. CD274 (PD-L1) Methylation is an Independent Predictor for Bladder Cancer Patients' Survival. Cancer Invest 2022;40:228-33. [Crossref] [PubMed]
- Lin PC, Lin JK, Lin CH, et al. Clinical Relevance of Plasma DNA Methylation in Colorectal Cancer Patients Identified by Using a Genome-Wide High-Resolution Array. Ann Surg Oncol 2015;22:S1419-27. [Crossref] [PubMed]
- Yang X, Hu J, Shi C, et al. Activation of TGF-β1 Pathway by SCUBE3 Regulates TWIST1 Expression and Promotes Breast Cancer Progression. Cancer Biother Radiopharm 2020;35:120-8. [Crossref] [PubMed]
- Chandrashekar DS, Karthikeyan SK, Korla PK, et al. UALCAN: An update to the integrated cancer data analysis platform. Neoplasia 2022;25:18-27. [Crossref] [PubMed]
- Li C, Tang Z, Zhang W, et al. GEPIA2021: integrating multiple deconvolution-based analysis into GEPIA. Nucleic Acids Res 2021;49:W242-6. [Crossref] [PubMed]
- Vasaikar SV, Straub P, Wang J, et al. LinkedOmics: analyzing multi-omics data within and across 32 cancer types. Nucleic Acids Res 2018;46:D956-63. [Crossref] [PubMed]
- Xu M, Xu J, Zhu D, et al. Expression and prognostic roles of PRDXs gene family in hepatocellular carcinoma. J Transl Med 2021;19:126. [Crossref] [PubMed]
- Moarii M, Boeva V, Vert JP, et al. Changes in correlation between promoter methylation and gene expression in cancer. BMC Genomics 2015;16:873. [Crossref] [PubMed]
- Kardoust Parizi M, Shariat SF, Margulis V, et al. Value of tumour-infiltrating immune cells in predicting response to intravesical BCG in patients with non-muscle-invasive bladder cancer: a systematic review and meta-analysis. BJU Int 2021;127:617-25. [Crossref] [PubMed]
- Harvey ZH, Chen Y, Jarosz DF. Protein-Based Inheritance: Epigenetics beyond the Chromosome. Mol Cell 2018;69:195-202. [Crossref] [PubMed]
- Davalos V, Esteller M. Cancer epigenetics in clinical practice. CA Cancer J Clin 2023;73:376-424. [Crossref] [PubMed]
- Yang J, Niu H, Huang Y, et al. A Systematic Analysis of the Relationship of CDH13 Promoter Methylation and Breast Cancer Risk and Prognosis. PLoS One 2016;11:e0149185. [Crossref] [PubMed]
- El Azzouzi M, El Ahanidi H, Hafidi Alaoui C, et al. Evaluation of DNA methylation in promoter regions of hTERT, TWIST1, VIM and NID2 genes in Moroccan bladder cancer patients. Cancer Genet 2022;260-261:41-5. [Crossref] [PubMed]
- Zhu H, Wang G, Qian J. Transcription factors as readers and effectors of DNA methylation. Nat Rev Genet 2016;17:551-65. [Crossref] [PubMed]
- Satyal U, Srivastava A, Abbosh PH. Urine Biopsy-Liquid Gold for Molecular Detection and Surveillance of Bladder Cancer. Front Oncol 2019;9:1266. [Crossref] [PubMed]
- Petitprez F, Meylan M, de Reyniès A, et al. The Tumor Microenvironment in the Response to Immune Checkpoint Blockade Therapies. Front Immunol 2020;11:784. [Crossref] [PubMed]
- Wang Y, Li C, Jiang T, et al. A comprehensive exploration of twist1 to identify a biomarker for tumor immunity and prognosis in pan-cancer. Medicine (Baltimore) 2024;103:e37790. [Crossref] [PubMed]
- Wang Y, Dai G, Lin Z, et al. TWIST1 rescue calcium overload and apoptosis induced by inflammatory microenvironment in S. aureus-induced osteomyelitis. Int Immunopharmacol 2023;119:110153. [Crossref] [PubMed]
- Li R, Wu C, Liang H, et al. Knockdown of TWIST enhances the cytotoxicity of chemotherapeutic drugs in doxorubicin-resistant HepG2 cells by suppressing MDR1 and EMT. Int J Oncol 2018;53:1763-73. [Crossref] [PubMed]
- Li C, Li Z, Zhang M, et al. An overview of Twist1 in glioma progression and recurrence. Int Rev Neurobiol 2023;172:285-301. [Crossref] [PubMed]
- Khatiwada P, Kannan A, Malla M, et al. Androgen up-regulation of Twist1 gene expression is mediated by ETV1. PeerJ 2020;8:e8921. [Crossref] [PubMed]
- Liu SS, Li JS, Xue M, et al. LncRNA UCA1 Participates in De Novo Synthesis of Guanine Nucleotides in Bladder Cancer by Recruiting TWIST1 to Increase IMPDH1/2. Int J Biol Sci 2023;19:2599-612. [Crossref] [PubMed]
- Cai H, Ke ZB, Chen JY, et al. Ubiquitin-specific protease 5 promotes bladder cancer progression through stabilizing Twist1. Oncogene 2024;43:703-13. [Crossref] [PubMed]
- Cheng L, Shao X, Wang Q, et al. Adipocyte enhancer binding protein 1 (AEBP1) knockdown suppresses human glioma cell proliferation, invasion and induces early apoptosis. Pathol Res Pract 2020;216:152790. [Crossref] [PubMed]
- Liu Y, Xue J, Zhong M, et al. Prognostic Prediction, Immune Microenvironment, and Drug Resistance Value of Collagen Type I Alpha 1 Chain: From Gastrointestinal Cancers to Pan-Cancer Analysis. Front Mol Biosci 2021;8:692120. [Crossref] [PubMed]
- Zhang H, Ding C, Li Y, et al. Data mining-based study of collagen type III alpha 1 (COL3A1) prognostic value and immune exploration in pan-cancer. Bioengineered 2021;12:3634-46. [Crossref] [PubMed]
- Hu YH, Lu YX, Zhang ZY, et al. SSH3 facilitates colorectal cancer cell invasion and metastasis by affecting signaling cascades involving LIMK1/Rac1. Am J Cancer Res 2019;9:1061-73. [PubMed]
- Prospéri MT, Pernier J, Lachuer H, et al. Plekhh1, a partner of myosin 1 and an effector of EphB2, controls the cortical actin network during cell repulsion. J Cell Sci 2021;134:jcs258802. [Crossref] [PubMed]
- Cao B, Zeng Y, Wu F, et al. Novel TRERF1 mutations in Chinese patients with ovarian endometriosis. Mol Med Rep 2018;17:5435-9. [Crossref] [PubMed]
- Chakraborty S, Wirrig EE, Hinton RB, et al. Twist1 promotes heart valve cell proliferation and extracellular matrix gene expression during development in vivo and is expressed in human diseased aortic valves. Dev Biol 2010;347:167-79. [Crossref] [PubMed]
- Nurnberg ST, Guerraty MA, Wirka RC, et al. Genomic profiling of human vascular cells identifies TWIST1 as a causal gene for common vascular diseases. PLoS Genet 2020;16:e1008538. [Crossref] [PubMed]
- Yu Y, Cao H, Zhang M, et al. Prognostic value of DNA methylation for bladder cancer. Clin Chim Acta 2018;484:207-12. [Crossref] [PubMed]
- Chen JQ, Salas LA, Wiencke JK, et al. Immune profiles and DNA methylation alterations related with non-muscle-invasive bladder cancer outcomes. Clin Epigenetics 2022;14:14. [Crossref] [PubMed]
- Friedrich MG, Weisenberger DJ, Cheng JC, et al. Detection of methylated apoptosis-associated genes in urine sediments of bladder cancer patients. Clin Cancer Res 2004;10:7457-65. [Crossref] [PubMed]
- Zand H, Pourvali K. The Function of the Immune System, Beyond Strategies Based on Cell-Autonomous Mechanisms, Determines Cancer Development: Immune Response and Cancer Development. Adv Biol (Weinh) 2024;8:e2300528. [Crossref] [PubMed]
- Luo Y, Chen J, Liu M, et al. Twist1 promotes dendritic cell-mediated antitumor immunity. Exp Cell Res 2020;392:112003. [Crossref] [PubMed]