Multi-omics decipher the immune microenvironment and unveil therapeutic strategies for postoperative ovarian cancer patients
Highlight box
Key findings
• Our study focused on postoperative ovarian cancer (OC) patients and we aimed to elucidate the intricate association between programmed cell death (PCD) and the prognosis of patients undergoing surgery for OC, while also providing tailored therapeutic strategies for different patient subgroups.
What is known and what is new?
• It is known that OC represents a formidable global gynecological malignancy. It is also known that it is important and necessary to establish a pioneering classification system and nomogram for prognostic prediction in this patient population.
• In this study, we employed a new PCD classification system to phenotype postoperative OC patients. Furthermore, leveraging machine learning algorithms, we established a new programmed cell death index (PCDI) and developed a nomogram that effectively predicts the clinical prognosis of OC patients after surgery. Importantly, the validity of the new PCDI was confirmed across four independent datasets comprising hundreds of patients, consistently demonstrating that patients with high PCDI values experienced worse postoperative prognoses in OC.
What is the implication, and what should change now?
• We provided tailored therapeutic strategies. In essence, our investigation has elucidated the genomic alterations and immune infiltration patterns correlated with PCD genes, leading to the development of a robust PCDI that holds great promise for accurately assessing prognosis and determining the therapeutic benefits of drug interventions. This signature represents a potentially invaluable tool for optimizing decision-making processes and surveillance protocols tailored to individual OC patients undergoing surgery.
Introduction
Ovarian cancer (OC) is a significant global gynecological malignancy with the highest mortality rate compared to other gynecological malignancies (1). In 2020, there were 313,959 new cases of OC and 207,252 deaths worldwide (2). Unfortunately, due to the difficulty in detecting the early stages of OC, more than half of the patients miss the opportunity for early diagnosis, which significantly impacts their prognosis (3). Surgery combined with chemotherapy is a commonly used treatment for OC. However, despite the effectiveness of surgery, relapse is still a common occurrence. Despite advancements in diagnostics and treatment, the ability to quickly diagnose and predict the prognosis of OC patients who have undergone surgery is still inadequate. Therefore, molecular methods are considered crucial for early detection of this disease.
Programmed cell death (PCD) not only acts as a natural defense against tumorigenesis and progression, but also plays a significant role in the tumor microenvironment (TME) (4-6). Pyroptosis is an inflammatory type of regulated cell death characterized by cell swelling and the release of abundant inflammatory cytokines (7). Recently, cupronoptosis has been identified as a novel PCD in the mitochondrial tricarboxylic acid (TCA) cycle induced by copper-dependent proteotoxic stress (8). Ferroptosis is an iron-dependent process that results in the accumulation of lipid hydroperoxides at lethal concentrations (9). Interestingly, a recent study showed that inhibition of ferroptosis induced drug resistance in anti-programmed cell death protein 1 (PD-1)/programmed cell death ligand 1 (PD-L1) cells (10). Furthermore, apoptotic vesicles are occupied by macrophages, which do not trigger an inflammatory response or interfere with neighboring cells (11). The autophagy-dependent cell death process involves multiple lysosomal degradation steps that contribute to metabolic adaptation and nutrient cycling. PCD, known to affect the TME and antitumor immunity, affects OC prognosis, TME, and chemotherapy outcomes.
In this study, we aimed to characterize the prognosis of PCD-related genes, the TME, and the underlying immune landscapes across distinct OC patient subgroups. Additionally, we investigated treatment strategies for different groups of OC patients. Besides, we created a programmed cell death index (PCDI) using a machine learning algorithm and conducted multi-omics analysis to investigate its prognostic value and associated molecular alterations. We present the following article in accordance with the TRIPOD reporting checklist (available at https://tcr.amegroups.com/article/view/10.21037/tcr-24-656/rc).
Methods
Transcriptome and single cell datasets collection
Uterine Corpus Endometrial Carcinoma (UCEC) transcriptome datasets combined with clinical data (e.g., stage T stage, overall survival) of OC patients were downloaded from The Cancer Genome Atlas (TCGA) Program data portal (https://portal.gdc.cancer.gov/) (12). In addition, Gene-Expression Omnibus (GEO) datasets including GSE9891 (13), GSE26712 (14), GSE49997 (15) and GSE63885 (16) were obtained from GEO (https://www.ncbi.nlm.nih.gov/geo/) to verify the precision of the model. Raw data of single cell from 10 OC patients were retrieved from GEO under accession numbers GSE210347 (17) (39,316 single cells isolated from 3 adjacent samples and 7 tumors samples). The TCGA data were normalized to transcripts per million (TPM), while the microarray datasets were normalized using z-scores. Additionally, all datasets were analyzed using R (version 4.1.1) along with R Bioconductor packages. The study was conducted in accordance with the Declaration of Helsinki (as revised in 2013).
Immunotherapy results calculation
Tumor Immune Dysfunction and Exclusion (TIDE) score was calculated by TIDE website (http://tide.dfci.harvard.edu/) (18).
The immune signatures calculation
To explore the immune landscapes of OC patients, we acquired 25 classical immune signatures, e.g., Antigen-presenting cell (APC) co inhibition, APC co stimulation, cytokine-cytokine receptor (CCR) and human leukocyte antigen (HLA) from the study by He et al. (19).
Pathway enrichment analysis
In order to study the functional differences among OC patients, we used gene set variation analysis (GSVA) R package (20) to conduct GSVA enrichment analysis. HALLLMARK gene set was retrieved from the MSigDB database (http://software.broadinstitute.org/gsea/msigdb/index.jsp). Pathway enrichment analysis was performed using the “fgsea” package, an algorithm for fast preranked gene set enrichment analysis using cumulative statistic calculation. In addition, “clusterProfiler” R package was used to conduct Gene Ontology (GO) enrichment analysis to comprehend the biological processes and molecular functions of the differential genes and Kyoto Encyclopedia of Genes and Genomes (KEGG) enrichment analysis to identify potential related biological pathways.
The construction of machine learning model based on PCD genes
The PCD related genes, including the key regulatory genes of 12 PCD patterns, were collected by Zou et al. (21). The “Limma” (22) package was subsequently used to screen out differentially expressed genes (DEGs) with the criterion of the adjusted P<0.05 and |log2fold change (FC)| >1. We also performed univariate Cox proportional hazard regression to explore masked prognosis related PCD genes among OC patients and finally we selected 28 PCD genes for future analysis, which related with the progress and prognosis of OC patients. To predict the prognosis of OC patients using these PCD-related genes, we applied a machine learning algorithm to establish the model.
Single cell data processing and cell type annotation
The data normalization, dimensionality reduction, and clustering for the OC (GSE210347) datasets were performed using Seurat v.3.0.0 (23) with default parameters. Cells were filtered based on the criterion of expressing at least 200 genes and having unique molecular indentifiers <6,000 during the preprocessing stage. Cells with more than 20% mitochondrial gene expression contribution were also removed. The cell clusters were annotated using classical cell signatures with SingleR (24).
Prediction of drug sensitivity in high- and low-PCDI groups
The calc Phenotype function in the R package ‘pRRophetic’ was utilized to predict the half maximal inhibitory concentration (IC50) of drugs based on gene expression profiles in cell lines. A P value of less than 0.05 was used as the threshold for selecting drugs deemed favorable in the context of the study (25).
Statistical analysis
In our study, we conducted a Wilcoxon test to compare the various features between the two groups of OC patients. To determine the correlation between the PCD index and immune-associated features, we combined Drug IC50 data and calculated the Spearman’s correlation coefficient. InferCNV (26) was applied to infer malignant epithelial cells from epithelial cell group. To examine the association between different groups of OC patients and survival, Kaplan-Meier survival analysis was applied using the ‘survminer’ R package. Additionally, univariate Cox proportional hazard regression was used to assess the association between PCDI and overall survival. Multivariate Cox regression was used to assess the independent prognostic value of PCDI in comparison to other clinical factors. A P value of less than 0.05 was considered statistically significant.
Results
Data preprocessing
For training and validation cohorts, we collected 379 patients from the TCGA, 285 patients from GSE9891, 185 patients from GSE26712, 101 patients from GSE63885, and 266 patients from GSE49997, among which, 76 patients from the TCGA, 285 patients from GSE9891, 185 patients from GSE26712, 101 patients from GSE63885, and 266 patients from GSE49997 who underwent surgery and have survival features met the inclusion criteria. As there was a lack of normal ovarian patient data in the TCGA database, we obtained transcriptome data from 88 ovarian normal tissues in the Genotype-Tissue Expression (GTEx) database. After integration, we identified 680 PCD genes that were expressed in both the training and validation cohorts, which were then used for further analysis. Additionally, we obtained single-cell RNA transcriptome data from 10 patients, which included 3 adjacent samples and 7 tumor samples from GSE210347.
Genotyping and clinical features of OC patients after surgery
Given the adequacy of sample size and the comprehensiveness of corresponding clinical information, we employed GSE9891 for phenotyping OC patients (Figure 1). By performing nonnegative matrix factorization (NMF) analysis using the expression matrix of 285 prognosis associated cell death-related genes defined by FSbyCox algorithm, we obtained two different subtypes of OC (Figure 1A). These OC clusters exhibited significant differences in overall survival (OS, P=2×10−5). Among which, cluster 2 showed favorable survival, while cluster 1 showed the worst survival (Figure 1B). Additionally, we assessed the clinical characteristics of patients in different clusters. We found that 64% of patients in cluster 1 were diagnosed with grade 3. Meanwhile, the 97% of patients in cluster 1 were diagnosed with stage III/IV, while stage I/II predominated in patients in clusters 2. Besides, all patients in cluster 1 were diagnosed with Malignant and all low malignant potential (LMP) patients were in cluster 2, indicating that cluster 1 was characterized by advanced pathological stages, which is consistent with a faster decline in its survival curve (Figure 1C). We further investigated Kyoto Encyclopedia of Genes and Genomes (KEGG) enrichment pathways between these clusters. We found that cluster 1 showed significantly enriched in pathways associated with tumor invasion and metastasis containing phosphatidylinositol-3 kinase-RAC-α serine/threonine-protein kinase (PI3K-Akt) signaling pathway, Janus kinase-signal transducer and activator of transcription (JAK-STAT) signaling pathway, Wnt signaling pathway, transforming growth factor (TGF)-beta signaling pathway, tumor necrosis factor (TNF) signaling pathway and NF-κB signaling pathway (Figure 1D). Additionally, we performed a GSEA analysis based on DEGs between C1 and C2 groups. Pathways associated with treatment resistance and tumor invasion [e.g., hypoxia, mitotic spindle, epithelial-mesenchymal transition (EMT) and myogenesis] were significantly upregulated in the cluster 1 group (Figure 1E, https://cdn.amegroups.cn/static/public/tcr-24-656-1.xlsx). Taken together, our results suggested that this classification demonstrates excellent prognostic potential for predicting postoperative outcomes in OC patients undergo surgery. Enrichment of pathways associated with treatment resistance and tumor invasion in the cluster 1 group are potential causes of its poor prognosis.
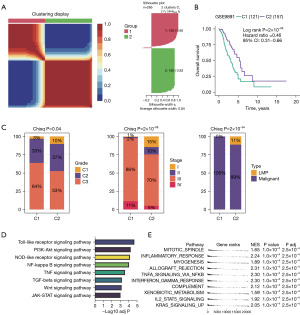
TME and chemotherapy response in different clusters
Given the prominent role of immunotherapy as a highly promising therapeutic strategy for OC, and recognizing the crucial involvement of cell death in activating antitumor immune responses, we conducted an analysis of the TME within these two identified clusters. Tertiary lymphoid structures (TLS) are acknowledged as germinal centers for immune cells in the TME, and thus we assessed the expression levels of a range of chemokines implicated in TLS formation. Intriguingly, we observed significantly higher expression of most chemokines responsible for regulating immune infiltration in cluster 1 (Figure 2A). Furthermore, several interferons (INFs), including their receptors (e.g., IFNAR2, IFNGR2), and the majority of interleukins (ILs) and their receptors were associated with transcripts linked to immune activation. Consistent with the higher expression of chemokines and increased immune cell infiltration within the TME of cluster 1, we noted elevated levels of these INFs, ILs, and their receptors in this particular cluster (Figure 2B,2C).
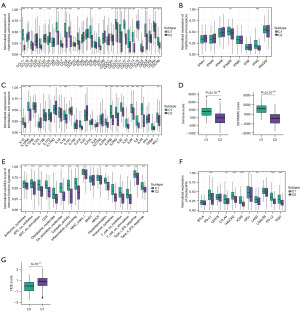
Subsequently, we proceeded to calculate the immune score and ESTIMATE score using the ESTIMATE algorithm within the different clusters. A higher immune score signifies greater infiltration of immune cells, while a higher ESTIMATE score indicates lower tumor purity. Notably, we observed significantly elevated immune scores and ESTIMATE scores in cluster 1 compared to cluster 2, indicative of increased immune cell infiltration within cluster 1 (Figure 2D). Additionally, we analyzed the immune-related function scores for each sample, revealing that most immune-related function scores were lower in cluster 2, suggesting suppression of these functions within this cluster (Figure 2E).
Given that the expression of immune checkpoints serves as a crucial foundation for immune checkpoint inhibitors (ICIs) therapy, we proceeded to evaluate the expression levels of immune checkpoints within the two identified clusters. Remarkably, cluster 1 exhibited higher expression of most immune checkpoints, including CD274/PD-L1, CTLA4, HAVCR2/TIM-3, ICOS, IDO1, PD-L2, LAG3, and TIGIT, in comparison to cluster 2. This observation suggests that cluster 1 may potentially derive greater benefit from immunotherapy interventions (Figure 2F). Furthermore, we conducted an additional assessment of the response to ICIs across the different clusters. Notably, patients in cluster 1 displayed higher TIDE scores, indicating a more favorable response to immunotherapy, whereas cluster 2 exhibited relative resistance. This finding aligns with the expression patterns of immune checkpoints observed (Figure 2G). Overall, the TME in cluster 1 appeared to support anti-tumor immunity and exhibited higher expression of immune checkpoints, thereby suggesting potential benefits from ICIs therapy.
Variant landscape of PCD genes in OC patients
Then, we used the TCGA-OV (ovarian cancer) and GTEx cohort and applied the Limma algorithm to identify 239 DEGs associated with 12 PCD patterns. The DEGs were selected based on an adjusted P value less than 0.05 and |log2FC| >1. A comprehensive list of these DEGs can be found in https://cdn.amegroups.cn/static/public/tcr-24-656-2.csv, while their corresponding volcano plot is depicted in Figure S1A. The KEGG was used to perform an enrichment analysis of the DEGs, revealing their important role in regulating pathways associated with cancer processes. Specifically, the DEGs were found to be involved in the NF-κB signaling pathway, PI3K-Akt signaling pathway, and mammalian target of rapamycin (mTOR) signaling pathway. Furthermore, the Gene Ontology (GO) enrichment analysis revealed that the DEGs are involved in crucial biological pathways such as regulating apoptotic signaling, autophagy, cellular response to hydrogen peroxide, and cell death in response to oxidative stress (Figure S1B). Subsequent analysis using the UniCox method revealed that 28 of the identified genes were significantly correlated with the prognosis of OC patients Supplementary (Figure S1C). The Circos plot of the expression of these 28 PCD genes demonstrated that CTSB was expressed at higher levels in OC prognostic effect patients who underwent surgery (Figure S1D). Additionally, we screened the copy number variation (CNV) level of these features (Figure S1E).
Establishment and evaluation of a PCDI in OC
To examine the prognosis of PCD-related mRNAs, we created a PCDI using least absolute shrinkage and selection operator (LASSO) and multiple Cox regression analysis. In contrast to conventional approaches, we employed multiple iterations (1,000 times) to mitigate the impact of random errors in LASSO regression and reduce contingency issues. The process involved five steps: (I) screening of 28 PCD-related genes associated with prognosis, and removal of those with low variance (Var <5); (II) dimensional reduction of selected PCD-related genes using LASSO regression, repeated for 1,000 times, and counting the total number of occurrences of each gene; (III) retention of genes with a total number of occurrences of more than 999 times among the PCD-genes; (IV) the model with the maximum area under the curve (AUC) value was selected as final prognostic model through multiple repetitions; (V) calculate the PCDI for each sample.
Through this process, we finally selected 8 PCD-related genes to establish model corresponding to the maximum AUC (5-year AUC =0.81) (Figure 3A-3C), including FBXO7, NFATC4, PIK3R1, PPP1R15A, SIAH1, STXBP1, TIMP3 and UNC5B. Among them, FBXO7 and SIAH1 were positively correlated with survival rate while NFATC4, PIK3R1, PPP1R15A, SIAH1, STXBP1, TIMP3 and UNC5B were negatively correlated with survival rate (Figure S2). The PCDI of each patient was exported using the following formula: PCDI = (−0.120880 × FBXO7 exp.) + (0.020864 × NFATC4 exp.) + (0.069234 × PIK3R1 exp.) + (0.008061 × PPP1R15A exp.) + (−0.179442 × SIAH1 exp.) + (0.032142 × STXBP1 exp.) + (0.066505 × TIMP3 exp.) + (0.013387 × UNC5B exp.). The PCDI was calculated using the formula above and patients were categorized into a high or low-PCDI group based on the best cut-off defined by the ’survminer’ R package. In the TCGA cohort, 51 OC patients were in the high-PCDI group and 25 OC patients were in the low-PCDI group. Results from Kaplan-Meier analysis indicated that patients in the high-PCDI group had a lower overall survival rate compared to those in the low-PCDI group (P=1×10−6) (Figure 3B). Additionally, a low PCDI was associated with a prolonged survival time (Figure 3D). Time-dependent receiver operating characteristic (ROC) curves were drawn using R software, and the AUC was calculated at various time points to estimate the predictive performance of the prognostic model in the TCGA-OV cohorts. The ROC curve indicated that the PCDI possessed notable credibility and predictive value, with an AUC of 0.810 at 3 years and 0.774 at 5 years in the TCGA cohorts (Figure 3C). Univariate Cox analysis revealed that only PCDI was associated with the survival rate of ovarian patients who underwent surgery (Figure 3E). Furthermore, the multivariate Cox model identified PCDI as the only independent predictive factor with a P value less than 0.001 (Figure 3F).
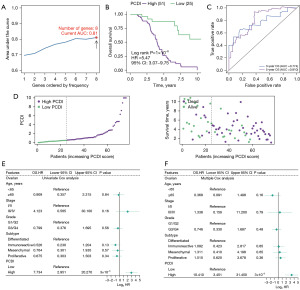
External validation of the accuracy of PCDI
To assess the effectiveness and precision of the prognostic model in practical clinical use, we proceeded with external validation analysis. Our validation cohorts included GSE9891, GSE26712, GSE63885, and GSE49997. We determined the best cut-off using the ‘survminer’ R package and divided the patients into two groups. Specifically, 285 patients from GSE9891, 185 patients from GSE26712, 101 patients from GSE63885, and 266 patients from GSE49997 were included in the analysis. The results of the Kaplan-Meier analysis indicated that patients in the high-PCDI group had a significantly shorter overall survival and higher death rates (GSE9891: P=3×10−4; GSE26712: P=0.009; GSE49997: P=0.005; GSE63885: P=3×10−4), as shown in Figure 4A. At the same time, in order to avoid the optimal cutoff affecting the reliability of the results, we also used the median value for further verification of the survival analysis, and the results showed that patients with high PCDI had higher mortality (Figure S4). Finally, multivariable Cox and logistic regression analyses were involved to establish a nomogram model in the OC cohort to estimate the overall survival. Sample type, stage, grade, and PCDI were included in the model (Figure 4B).
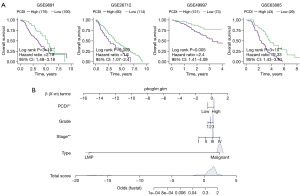
Immune and functional landscapes of low- and high-PCDI groups
The immune system plays a crucial role in fighting tumors, and its effectiveness is influenced by various components within the TME. These components include the major histocompatibility complex (MHC) molecules that are responsible for presenting antigens to the immune system and IFNs that help eradicate cancer cells. Furthermore, immune checkpoints, ILs, cytokines, and their receptors represent essential TME components, with chemokines responsible for immune cell migration into the TME, and IFNs regulating the anti-tumor activity of effector T cells (27-29). To explore the reasons behind the lower survival rate of patients with high-PCDI, a comprehensive analysis of the TCGA cohort was carried out. The study involved comparing immune functions of both groups to identify any discrepancies.
GSVA analysis was performed to examine the correlation between HALLMARK pathways and PCDI. Our results revealed that patients with high-PCDI exhibited an enrichment of pathways that support tumor progression and metastasis, including Epithelial mesenchymal transition, Hypoxia, myogenesis and TGF-β signaling (Figure 5A). On the other hand, immune associated pathways such as IFN-α response, IFN-γ response, and inflammatory response were found to be enriched in the low-PCDI group (Figure 5B).
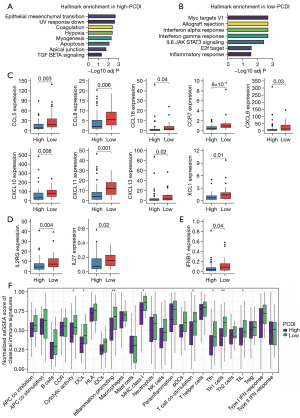
Additionally, we found a strong negative correlation between PCDI and the expression of various chemokines and receptors, such as CCL5, CCL8, CCL18, CCR7, CXCL9, CXCL10, CXCL11, CXCL13, and XCL1 (Figure 5C). Additionally, we observed a negative correlation between PCDI and the expression of chemokines and their receptors, including IFNs and their receptors (Figure 5D,5E). Moreover, our findings indicate that low-PCDI patients exhibited a significant improvement in classical immune function-associated signatures, such as cytolytic activity, inflammation-promoting, T cell co-stimulation, and TIL. This suggests that these patients may have an increased response to immunotherapy, as shown in Figure 5F.
Dissection of TME-based on single cell transcriptome
To investigate the differences in the TME between low- and high-PCDI groups, we analyzed single-cell mRNA profiles of OC tissues from the GSE210347 dataset. We applied a filter to only include cells that expressed at least 200 genes and removed cells with over 20% expression of mitochondrial genes. Following quality control, the filtered cells were classified into seven distinct cell types based on established biomarkers. These types include epithelial, identified by KRT8, KRT18, and EPCAM; T & NK, identified by CD3D, IL7R, NKG7 and GZMK cells; cancer-associated fibroblast (CAF), identified by COL1A1, DCN and COL1A2; Plasma, identified by JCHAIN, MZB1, and IGKC; perivascular-like (PVL) cell, identified by TAGLN, MYH11, and MCAM; macrophage, identified by SPP1, C1QC, S100A9 and CD14; Monocytes, identified by HLA-DRA and FCGR3A; cycling cell, identified by MKI67 and CDK1; Cycling cell, identified by MKI67 and CDK1; endothelial cell, identified by VWF and PECAM1; and B cell, identified by CD79A and MS4A1 (Figure 6A,6B and Figure S3). We utilized the LASSO algorithm to evaluate the score of individual cells, using 8 PCD genes (Figure 6C). Our findings indicate that the PCDI was significantly higher in the adjacent tissues as compared to the tumor tissues (Figure 6D).
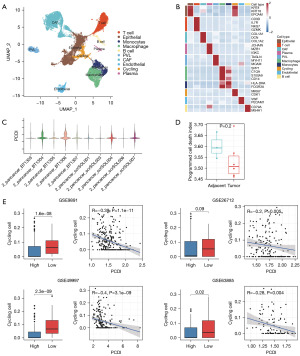
To overcome the limitations of our scRNA-seq dataset’s sample size, we employed the use of BisqueRNA (30), a deconvolution algorithm, to simulate cell-type-specific gene expression profiles. This allowed us to predict the abundance of each cell type, as quantified by scRNA-seq, in larger scale datasets from four independent OC cohorts sourced from the GEO. The BisqueRNA tool’s ability to accurately predict gene expression profiles specific to certain cell types was developed through training with our own single-cell RNA sequencing data. In order to better understand the relationships between various cell populations in the ovarian microenvironment, we conducted an analysis of pairwise Spearman correlations within infiltration patterns of ten major cell types across four independent OC cohorts. We observed a strong negative correlation between cycling cells and PCDI across all groups studied and considered a Spearman correlation coefficient (|Rs|) >0.2 and P value <0.05 to indicate significant correlation. The correlation ranged from −0.2 in the GSE26712 dataset with 185 OC samples to −0.4 in the GSE49997 dataset with 266 OC samples (Figure 6E). In addition, we found that the PCDI was higher in CAF, PVL, and endothelial cells, whereas it was almost zero in epithelial, T cell, plasma, and monocytes (Figure S3A). Then, we utilized the Wilcoxon test to compare the proportion of Endothelial and CAF in low-PCDI samples to that in high-PCDI samples (Figure S3B,S3C). To further analyze the changes in the immune microenvironment between the two groups, T&NK, macrophage, and monocytes were reaggregated (Figure S3D,S3E). Additionally, infer-CNV was utilized to distinguish between malignant and normal epithelial cells (Figure S3F,S3G). However, we did not find significant differences in the proportion of cell subclasses between the high and low PCDI groups (Figure S3H).
Efficacy of PCDI in predicting drug sensitivity
To investigate potential treatment strategies for high- and low-PCDI groups, we first explored the expression of immune checkpoints in the high- and low- PCDI groups, and revealed that OV patients with low-PCDI were more sensitive to immunotherapy drug, supported by the higher expression of immunotherapy targets such as BTLA, PD-L1 (CD274), CTLA4, ICOS and TIGIT in low-PCDI group (Figure 7A). What’s more, by using TIDE webserver, we found that patients with high-PCDI responded poorly to checkpoint inhibitors (CPIs) therapy treatment, whereas the proportion of patients with low-PCDI responded to CPIs treatment was higher than patients with high-PCDI (Figure 7B). In line with this, the TIDE score in OC patients were analyzed, revealing a higher TIDE score in high-PCDI patients and suggesting a significant positive correlation between the TIDE score and PCDI (Figure 7B,7C). Our results imply that OV patients with low-PCDI may benefit from immunotherapy.
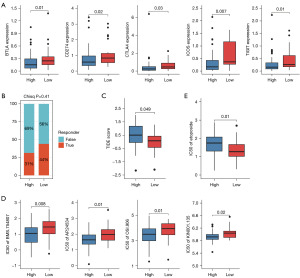
To further investigate the correlation between the PCDI and drug sensitivity, we analyzed the IC50 values of each drug in OC samples to identify any significant variations. Our study revealed that patients in the high-PCDI group exhibited greater sensitivity to tyrosine kinase inhibitors, such as BMS.754807, AP.24534, OSI.906 and KIN001.135, as illustrated in Figure 7D. Additionally, our findings indicate that OC patients with low-PCDI were more sensitive to etoposide, a cell cycle specific antitumor agent, supported by the lower IC50 values of these drugs in the high-PCDI group (Figure 7E).
Discussion
In our study, we performed phenotyping of postoperative OC patients based on PCD-associated genes that are prognostically relevant. We found that patients in cluster 1, despite having poorer prognosis, exhibited heightened sensitivity to immune-based therapies. Then, we also conducted a comprehensive analysis of twelve distinct PCD patterns in OC by constructing a PCD associated cluster and establishing a PCD signature using machine learning algorithm within the TCGA cohort and further validate its excellent performance in four other external cohorts (TCGA-OV, GSE9891, GSE26712, GSE49997 and GSE63885), demonstrating satisfactory results. We then explored the immune and functional landscapes of different patient groups based on both bulk and single-cell transcriptome data. Lastly, we examined the association between different patient subgroups, immunomodulators, TME, and drug sensitivity and provided different treatment strategies for high- and low-PCDI patients.
Surgery plus chemotherapy is a classic treatment for OC. Nevertheless, despite the efficacy of surgery, relapse remains a frequent occurrence. Recent studies have connected PCD to the release of cancer antigens, IFN activity, and immune cell damage, involving various mechanisms (31-33). PCD has been increasingly recognized as playing a critical role in biological processes, particularly in the development and metastasis of malignant tumors (31). To investigate this further, we constructed an 8 genes PCD signature (FBXO7, NFATC4, PIK3R1, PPP1R15A, SIAH1, STXBP1, TIMP3 and UNC5B) using a LASSO algorithm and categorized OC patients in the TCGA database into low- and high-PCDI groups. The model demonstrated satisfactory AUC values in the TCGA-cohort and four validation cohorts (34,35). In the Kaplan-Meier analysis, OC patients with low-PCDI displayed significantly better survival rates compared to those with high-PCDI. Additionally, we confirmed the constructed PCDI as an exclusive independent predictive factor for OC patients after surgery in both univariate and multivariate Cox regression analyses.
PCD has been associated with the release of cancer antigens, IFN activity, and immune cell damage (4). Our findings reveal a robust negative correlation between PCDI and the expression of chemokines and their receptors, IFNs and their receptors and classical immune functional signatures. Cytokines and their receptors are essential components of TME, with chemokines responsible for the migration of immune cells into TME, ILs enriched in the TME as immunomodulatory cytokines, and IFNs mediating antitumor activity of effector T cells (27,28). We found that the expression of chemokines, including CCL5, CCL8, CCL18, CCR7, CXCL9, CXCL10, CXCL11, CXCL13 and XCL1 were higher in low-PCDI group than in high-PCDI group. It has been established that CCL5 plays an important role in attenuating T cell inflammation (36). CXCL9 was found to be able to inhibit tumor growth and drive anti-PD-L1 therapy in OC (37) as well as CXCL11 (38). In addition, CXCL13 has also been proved to function as a biomarker for predicting the effect of immunotherapy (39). In our study, IL2RG, IL27 and IFNB1 were also expressed higher in low-PCDI group. Specifically, it is involved in anti-inflammatory effects against spondylarthritis through the suppression of Th17 responses (40).
Previous studies have focused on the relationship between cell death and prognosis; however, they have not addressed the heterogeneity of the immune microenvironment in patients with varying prognoses, thereby overlooking the connection between the immune microenvironment and patient outcomes using single cell RNA sequence (38654239; 38654239). The role of immune cells in tumor development has been extensively documented, with varying subpopulations present in different types of tumors and even among patients with the same pathological type. The PCDI was higher in adjacent tissues than in tumor tissues, which was consistent with the result that PCDI was associated with lower survival rate in OC patients. Additionally, we also found that E2f target and Myc targets V1 pathways were significantly enriched in low-PCDI OC patients, which may indicate that cell cycle processes were disrupted in patients with low-PCDI.
Hitherto, the continuous advances in the immuno-oncology and the development of immunotherapeutic agents, such as CPIs and chimeric antigen receptor (CAR) T cell therapy, provide promising strategies to overcome tumors by activating endogenous immune system (41,42). In our study, we observed a significant increase in the expression of BTLA, PD-L1 (CD274), CTLA4, ICOS and TIGIT in OC patients with high-PCDI. Consistent with these results, we found that the TIDE score of low-PCDI patients was lower than that of high-PCDI patients. In line with this, the patients with low-PCDI may have an anti-TME and be more sensitive to immunotherapy. Moreover, our findings revealed that OC patients with low-PCDI were more sensitive to etoposide, a cell cycle specific antitumor agent.
Despite the excellent performance of our model in both the training and validation cohorts, some limitations still need to be addressed. The absence of multi-omics data, such as tumor burden and methylation levels, hinders our ability to investigate the potential landscape of different PCDI groups. Besides, the biological function of some drug therapy strategies has not been investigated in OC cells, which is essential for further experimental research (43,44). Additionally, this cohorts ignored the quality of treatment, which was optimal or suboptimal debulking of the patients. Therefore, further validation of the results obtained requires more multi-center randomized controlled trials with high quality, large sample sizes, and adequate follow-up.
Conclusions
In summary, our research has developed a reliable PCDI that can be used to assess prognosis and determine drug therapy benefits. This signature has the potential to be a valuable tool for optimizing decision-making and surveillance protocols for individual OC patients who undergo surgery.
Acknowledgments
Funding: This work was supported by
Footnote
Reporting Checklist: The authors have completed the TRIPOD reporting checklist. Available at https://tcr.amegroups.com/article/view/10.21037/tcr-24-656/rc
Peer Review File: Available at https://tcr.amegroups.com/article/view/10.21037/tcr-24-656/prf
Conflicts of Interest: All authors have completed the ICMJE uniform disclosure form (available at https://tcr.amegroups.com/article/view/10.21037/tcr-24-656/coif). The authors have no conflicts of interest to declare.
Ethical Statement: The authors are accountable for all aspects of the work in ensuring that questions related to the accuracy or integrity of any part of the work are appropriately investigated and resolved. The study was conducted in accordance with the Declaration of Helsinki (as revised in 2013).
Open Access Statement: This is an Open Access article distributed in accordance with the Creative Commons Attribution-NonCommercial-NoDerivs 4.0 International License (CC BY-NC-ND 4.0), which permits the non-commercial replication and distribution of the article with the strict proviso that no changes or edits are made and the original work is properly cited (including links to both the formal publication through the relevant DOI and the license). See: https://creativecommons.org/licenses/by-nc-nd/4.0/.
References
- Kuroki L, Guntupalli SR. Treatment of epithelial ovarian cancer. BMJ 2020;371:m3773. [Crossref] [PubMed]
- Sung H, Ferlay J, Siegel RL, et al. Global Cancer Statistics 2020: GLOBOCAN Estimates of Incidence and Mortality Worldwide for 36 Cancers in 185 Countries. CA Cancer J Clin 2021;71:209-49. [Crossref] [PubMed]
- Miller EA, Pinsky PF, Schoen RE, et al. Effect of flexible sigmoidoscopy screening on colorectal cancer incidence and mortality: long-term follow-up of the randomised US PLCO cancer screening trial. Lancet Gastroenterol Hepatol 2019;4:101-10. [Crossref] [PubMed]
- Galluzzi L, Vitale I, Warren S, et al. Consensus guidelines for the definition, detection and interpretation of immunogenic cell death. J Immunother Cancer 2020;8:e000337. [Crossref] [PubMed]
- Peng F, Liao M, Qin R, et al. Regulated cell death (RCD) in cancer: key pathways and targeted therapies. Signal Transduct Target Ther 2022;7:286. [Crossref] [PubMed]
- Tang D, Kang R, Berghe TV, et al. The molecular machinery of regulated cell death. Cell Res 2019;29:347-64. [Crossref] [PubMed]
- Tang R, Xu J, Zhang B, et al. Ferroptosis, necroptosis, and pyroptosis in anticancer immunity. J Hematol Oncol 2020;13:110. [Crossref] [PubMed]
- Tsvetkov P, Coy S, Petrova B, et al. Copper induces cell death by targeting lipoylated TCA cycle proteins. Science 2022;375:1254-61. [Crossref] [PubMed]
- Stockwell BR, Friedmann Angeli JP, Bayir H, et al. Ferroptosis: A Regulated Cell Death Nexus Linking Metabolism, Redox Biology, and Disease. Cell 2017;171:273-85. [Crossref] [PubMed]
- Jiang Z, Lim SO, Yan M, et al. TYRO3 induces anti-PD-1/PD-L1 therapy resistance by limiting innate immunity and tumoral ferroptosis. J Clin Invest 2021;131:e139434. [Crossref] [PubMed]
- Zhao R, Kaakati R, Lee AK, et al. Novel roles of apoptotic caspases in tumor repopulation, epigenetic reprogramming, carcinogenesis, and beyond. Cancer Metastasis Rev 2018;37:227-36. [Crossref] [PubMed]
- Kent WJ, Sugnet CW, Furey TS, et al. The human genome browser at UCSC. Genome Res 2002;12:996-1006. [Crossref] [PubMed]
- Tothill RW, Tinker AV, George J, et al. Novel molecular subtypes of serous and endometrioid ovarian cancer linked to clinical outcome. Clin Cancer Res 2008;14:5198-208. [Crossref] [PubMed]
- Vathipadiekal V, Wang V, Wei W, et al. Creation of a Human Secretome: A Novel Composite Library of Human Secreted Proteins: Validation Using Ovarian Cancer Gene Expression Data and a Virtual Secretome Array. Clin Cancer Res 2015;21:4960-9. [Crossref] [PubMed]
- Pils D, Hager G, Tong D, et al. Validating the impact of a molecular subtype in ovarian cancer on outcomes: a study of the OVCAD Consortium. Cancer Sci 2012;103:1334-41. [Crossref] [PubMed]
- Lisowska KM, Olbryt M, Student S, et al. Unsupervised analysis reveals two molecular subgroups of serous ovarian cancer with distinct gene expression profiles and survival. J Cancer Res Clin Oncol 2016;142:1239-52. [Crossref] [PubMed]
- Luo H, Xia X, Huang LB, et al. Pan-cancer single-cell analysis reveals the heterogeneity and plasticity of cancer-associated fibroblasts in the tumor microenvironment. Nat Commun 2022;13:6619. [Crossref] [PubMed]
- Jiang P, Gu S, Pan D, et al. Signatures of T cell dysfunction and exclusion predict cancer immunotherapy response. Nat Med 2018;24:1550-8. [Crossref] [PubMed]
- He Y, Jiang Z, Chen C, et al. Classification of triple-negative breast cancers based on Immunogenomic profiling. J Exp Clin Cancer Res 2018;37:327. [Crossref] [PubMed]
- Subramanian A, Tamayo P, Mootha VK, et al. Gene set enrichment analysis: a knowledge-based approach for interpreting genome-wide expression profiles. Proc Natl Acad Sci U S A 2005;102:15545-50. [Crossref] [PubMed]
- Zou Y, Xie J, Zheng S, et al. Leveraging diverse cell-death patterns to predict the prognosis and drug sensitivity of triple-negative breast cancer patients after surgery. Int J Surg 2022;107:106936. [Crossref] [PubMed]
- Ritchie ME, Phipson B, Wu D, et al. limma powers differential expression analyses for RNA-sequencing and microarray studies. Nucleic Acids Res 2015;43:e47. [Crossref] [PubMed]
- Stuart T, Butler A, Hoffman P, et al. Comprehensive Integration of Single-Cell Data. Cell 2019;177:1888-1902.e21. [Crossref] [PubMed]
- Aran D, Looney AP, Liu L, et al. Reference-based analysis of lung single-cell sequencing reveals a transitional profibrotic macrophage. Nat Immunol 2019;20:163-72. [Crossref] [PubMed]
- Lu X, Jiang L, Zhang L, et al. Immune Signature-Based Subtypes of Cervical Squamous Cell Carcinoma Tightly Associated with Human Papillomavirus Type 16 Expression, Molecular Features, and Clinical Outcome. Neoplasia 2019;21:591-601. [Crossref] [PubMed]
- Fan J, Lee HO, Lee S, et al. Linking transcriptional and genetic tumor heterogeneity through allele analysis of single-cell RNA-seq data. Genome Res 2018;28:1217-27. [Crossref] [PubMed]
- Bonati L, Tang L. Cytokine engineering for targeted cancer immunotherapy. Curr Opin Chem Biol 2021;62:43-52. [Crossref] [PubMed]
- Kondoh N, Mizuno-Kamiya M. The Role of Immune Modulatory Cytokines in the Tumor Microenvironments of Head and Neck Squamous Cell Carcinomas. Cancers (Basel) 2022;14:2884. [Crossref] [PubMed]
- Wei X, Zhang Y, Yang Z, et al. Analysis of the role of the interleukins in colon cancer. Biol Res 2020;53:20. [Crossref] [PubMed]
- Li B, Zhang W, Guo C, et al. Benchmarking spatial and single-cell transcriptomics integration methods for transcript distribution prediction and cell type deconvolution. Nat Methods 2022;19:662-70. [Crossref] [PubMed]
- Su Z, Yang Z, Xu Y, et al. Apoptosis, autophagy, necroptosis, and cancer metastasis. Mol Cancer 2015;14:48. [Crossref] [PubMed]
- Oehadian A, Koide N, Mu MM, et al. Interferon (IFN)-β induces apoptotic cell death in DHL-4 diffuse large B cell lymphoma cells through tumor necrosis factor-related apoptosis-inducing ligand (TRAIL). Cancer letters 2005;225:85-92. [Crossref] [PubMed]
- Liang M, Yang H, Fu J. Nimesulide inhibits IFN-γ-induced programmed death-1-ligand 1 surface expression in breast cancer cells by COX-2 and PGE2 independent mechanisms. Cancer letters 2009;276:47-52. [Crossref] [PubMed]
- Ma G, Zeng S, Zhao Y, et al. Development and validation of a nomogram to predict cancer-specific survival of mucinous epithelial ovarian cancer after cytoreductive surgery. J Ovarian Res 2023;16:120. [Crossref] [PubMed]
- Cai X, Lin J, Liu L, et al. A novel TCGA-validated programmed cell-death-related signature of ovarian cancer. BMC Cancer 2024;24:515. [Crossref] [PubMed]
- Bruand M, Barras D, Mina M, et al. Cell-autonomous inflammation of BRCA1-deficient ovarian cancers drives both tumor-intrinsic immunoreactivity and immune resistance via STING. Cell Rep 2021;36:109412. [Crossref] [PubMed]
- Seitz S, Dreyer TF, Stange C, et al. CXCL9 inhibits tumour growth and drives anti-PD-L1 therapy in ovarian cancer. Br J Cancer 2022;126:1470-80. [Crossref] [PubMed]
- Shi Z, Zhao Q, Lv B, et al. Identification of biomarkers complementary to homologous recombination deficiency for improving the clinical outcome of ovarian serous cystadenocarcinoma. Clin Transl Med 2021;11:e399. [Crossref] [PubMed]
- Liu B, Zhang Y, Wang D, et al. Single-cell meta-analyses reveal responses of tumor-reactive CXCL13(+) T cells to immune-checkpoint blockade. Nat Cancer 2022;3:1123-36. [Crossref] [PubMed]
- Jouhault Q, Cherqaoui B, Jobart-Malfait A, et al. Interleukin 27 is a novel cytokine with anti-inflammatory effects against spondyloarthritis through the suppression of Th17 responses. Front Immunol 2023;13:1072420. [Crossref] [PubMed]
- Zhang J, He S, Ying H. Refining molecular subtypes and risk stratification of ovarian cancer through multi-omics consensus portfolio and machine learning. Environ Toxicol 2024; Epub ahead of print. [Crossref] [PubMed]
- Jia W, Li N, Wang J, et al. Immune-related gene methylation prognostic instrument for stratification and targeted treatment of ovarian cancer patients toward advanced 3PM approach. EPMA J 2024;15:375-404. [Crossref] [PubMed]
- Li Z, Gu H, Xu X, et al. Unveiling the novel immune and molecular signatures of ovarian cancer: insights and innovations from single-cell sequencing. Front Immunol 2023;14:1288027. [Crossref] [PubMed]
- Zhou Q, Ding DD, Lu M, et al. Multi-Omics Analysis of the Prognostic and Immunological Role of Runt-Related Transcription Factor 3 in Pan-Cancer. Crit Rev Eukaryot Gene Expr 2023;33:63-83. [Crossref] [PubMed]