Determining new disulfidptosis-associated lncRNA signatures pertinent to breast cancer prognosis and immunological microenvironment
Highlight box
Key findings
• Providing novel indicators associated with disulfidptosis-associated long non-coding RNAs (lncRNAs) for forecasting the prognosis of patients with breast cancer (BC).
What is known and what is new?
• Disulfidptosis is a novel form of cell death triggered by disulfide stress.
• We investigated lncRNAs markers associated with disulfidptosis that may either enhance or suppress BC, and we elucidated their connection with immune responses.
What is the implication, and what should change now?
• These markers of disulfidptosis-related lncRNAs could offer novel therapeutic targets for BC treatment, pending validation through additional experimental research.
Introduction
Breast cancer (BC) is the most common malignancy worldwide, threatening women’s health and longevity (1). Contrary to other solid tumors, the prognosis of many BC patients can be improved with multiple treatments, such as radical surgery, chemotherapy, radiotherapy, and targeted therapy, but some BC patients still experience unfavorable outcomes due to the variable heterogeneity of BC (2,3). At the same time, several clinical trials have shown that the development of tumor resistance often leads to a poor prognosis. The etiology of BC is greatly influenced by the tumor microenvironment (TME). The tumor immunological microenvironment and related immune escape mechanisms have a substantial influence on the course of BC (4). TME, essential for the proliferation and development of neoplastic cells, is made of different cellular constituents such as endothelial cells, immune cells, and lymphocytes, in addition to non-cellular components (5). M2 macrophages, which make up the majority of tumor-associated macrophages (TAMs), secrete cytokines such as matrix metalloproteinase (MMP), vascular endothelial growth factor-A (VEGF-A), CCL18, and IL-10 to advance tumors (6). In addition, regulatory T cells (Tregs) promote tumor progression, angiogenesis and metastasis by suppressing anti-tumor responses (7). In addition to suppressing immune cell function by producing various cytokines or metabolites that promote tumor progression, invasion and metastasis, cancer-associated fibroblasts (CAFs) also have the ability to shape the extra-tumoral stroma, creating a blockage to the penetration of drugs or therapeutic immune cells. This may inhibit immune cells and medications from penetrating tumor tissue deeply, decreasing the effectiveness of tumor therapy (8,9).
Disulfidptosis represents a novel mode of cell death that operates independently from recognized pathways such as apoptosis, ferroptosis, and cuproptosis. In sugar-free SLC7A11-high UMRC6 cells, the accumulation of a large number of disulfide bonds led to abnormal disulfide bond cross-linking between actin cytoskeletal proteins, contraction of the cytoskeleton, disruption of its organisation, and ultimately destruction of actin networks and cell death, according to the study. Although the study of disulfidptosis in their tumors is still ongoing at this time, it remains a prospective novel method for the treatment of tumors (10).
LncRNAs are molecules of non-coding RNA that are longer than 200 nucleotides. They are capable of modulating gene expression at various stages, including epigenetic, transcriptional, and post-transcriptional regulation. There is mounting evidence that lncRNAs play key roles in regulating tumorigenesis and metastasis. For example, OCC1 lncRNA promotes HuR protein ubiquitinated degradation, thereby inhibiting the proliferation of colonic carcinoma cells (11). In addition, some lncRNA that can be detected in the plasma and urine of tumor patients due to their high stability, such as PCA3, PCGEM1 and PCAT-1, are expected to be potential tumor biomarkers (12,13). Therefore, it is of utmost importance to investigate the relationship between lncRNAs and the mechanism of BC formation, to develop new treatment options for BC, and to provide benefits to patients.
The purpose of this research was to create a prediction signature using lncRNAs linked to disulfidptosis and then test its reliability using internal validation. This signature was then analysed to see if it could be used to predict prognosis, tumour immune cell infiltration, and tumour immune function in patients with BC. Potential anti-cancer drugs associated with this signature have also been predicted. We present this article in accordance with the TRIPOD reporting checklist (available at https://tcr.amegroups.com/article/view/10.21037/tcr-24-513/rc).
Methods
Data collection and processing
A total of 1,098 BC patients’ clinical information and RNA-sequencing data were extracted from The Cancer Genome Atlas (TCGA, https://portal.gdc.cancer.gov/) database. After data cleaning, data from 1,059 BC patients were used for subsequent analysis. Fifteen disulfidptosis-related genes were extracted from previously published literature (10). Figure 1 illustrates the flowchart of the data analysis process. The study was conducted in accordance with the Declaration of Helsinki (as revised in 2013).
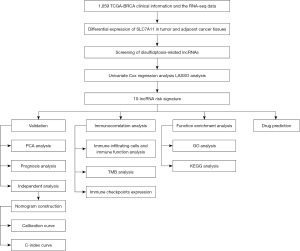
Construction of the risk signature
We used the “limma” package in R to calculate the correlation between disulfidptosis-associated genes and lncRNAs. A total of 172 disulfide-related lncRNAs were identified using correlation coefficient (|R2|>0.3) with a P value <0.05 as a screening condition. Using the R package “survival”, a univariate Cox regression analysis was conducted to identify lncRNAs associated with disulfidptosis prognosis in BC patients. The data set was subsequently divided into training and testing cohorts using a 7:3 random assignment ratio. The training cohort was subjected to LASSO analysis in order to generate a risk signature. The risk score was calculated as: risk score = (Expi × βi), where Exp represents the expression level of the model gene and β denotes the risk coefficient of the signature lncRNAs.
Independent prognostic analysis and establishment of a predictive nomogram
Using R package “survival”, univariate and multivariate Cox regression analyses identified independent prognostic factors. Subsequently, we constructed a nomogram utilizing the “rms” and “survminer” R packages, based on the multivariate regression analysis results. The concordance index (C-index) and calibration curves were plotted to assess the one, three, and five-year overall survival probabilities.
Tumor mutation burden (TMB) analyses
TMB files, which contained information about somatic mutations, were retrieved from the TCGA database. Using the “maftoolsv, “ggpubr”, and “ggplot2” R packages, we were able to estimate and visualise the differences in TMB levels between the two risk groupings. R packages “limma”, “ggpubr”, “ggplot2”, and “ggExtra” were used to analyse and visualize the relationship between risk scores and TMB scores. Kaplan-Meier analysis, performed using the “survival” and “survminer” R packages, was used to compare the survival rates of patients with varied TMB levels and risk statuses.
Analysis of tumor immune microenvironment
ssGSEA was used to assess immune infiltrating cell activity and immune-related functions. This method made use of the “GSVA” R packages. Five immunological checkpoints were evaluated for expression levels across the two groups.
Functional enrichment analysis
Differentially expressed genes (DEGs) were identified between the high-risk and low-risk groups using the screening criteria of false discovery rate (FDR) 0.05 and log2 fold change >1 or <−1. Then we performed Gene Ontology (GO) and Kyoto Encyclopedia of Genes and Genomes (KEGG) enrichment analysis for DEGs and mapping using “clusterProfiler”, “ggplot2”, and “circlize” software packages.
Immunotherapy and drug sensitivity prediction methods
The Tumor Immune Dysfunction and Exclusion (TIDE) (http://tide.dfci.harvard.edu/) online tool was used to evaluate the ability of immune escape in high- and low-risk groups within the risk signature. Using the gene expression profile of tumor samples, the framework assesses the potential for immunological escape from tumors (14). The “oncoPredict” package was used to predict and screen potential drugs associated with the model, with a P value less than 0.001 as a screening condition (15).
Statistical analysis
Differences in percentages were analysed using either a Chi-squares test (parametric) or a Wilcoxon test (non-parametric). The R statistical programming language (version 4.1.2) was used for all studies. Statistical significance was assumed at a P value of less than 0.05.
Results
Construction of the risk signature on disulfidptosis-related lncRNA
Differential analysis revealed a substantial upregulation of SLC7A11 expression in BC tissues compared to adjacent normal tissues (Figure 2A). We identified 172 lncRNA associated with disulfidptosis. In the training cohort, univariate Cox analysis was performed and eleven lncRNAs were identified that were significantly associated with BC prognosis (Table 1). In addition, a risk signature is constructed by LASSO analysis (Figure 2B,2C). The risk signature consists of ten lncRNA and the risk score per each patient was calculated as: risk score = (0.207 × expression level of LINC01589) − (0.020 × expression level of LINC01087) − (0.085 × expression level of AL137847.1) − (0.066 × expression level of ELOVL2-AS1) − (2.626 × expression level of AL137856.1) − (0.072 × expression level of LINC01948) + (0.104 × expression level of AP003031.1) + (0.443 × expression level of AP001922.3) − (0.231 × expression level of MAPT-IT1) − (0.208 × expression level of AC004967.2) (Table 2). LINC01589, AP003031.1, and AP001922.3 were risk factors. LINC01087, AL137847.1, ELOVL2-AS1, AL137856.1, LINC01948, MAPT-IT1, and AC004967.2 were protective factors. BC patients were separated into a high-risk group and a low-risk group based on the median value. Principal component analysis (PCA) analysis revealed that the risk signature’s 10 lncRNAs involved with disulfidptosis were very effective at telling the two groups apart (Figure 2D-2H).
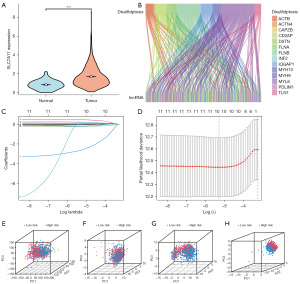
Table 1
LncRNA | HR | Lower 95% CI | Upper 95% CI | P value |
---|---|---|---|---|
LINC01087 | 0.884 | 0.795 | 0.983 | 0.02 |
AL137847.1 | 0.418 | 0.199 | 0.876 | 0.02 |
ELOVL2-AS1 | 0.809 | 0.677 | 0.967 | 0.02 |
AL137856.1 | 0.044 | 0.006 | 0.344 | 0.003 |
LINC01589 | 1.319 | 1.026 | 1.695 | 0.03 |
LINC01948 | 0.791 | 0.636 | 0.983 | 0.03 |
AP003031.1 | 1.472 | 1.12 | 1.934 | 0.006 |
AP001922.3 | 1.816 | 1.178 | 2.798 | 0.007 |
MAPT-IT1 | 0.655 | 0.498 | 0.861 | 0.002 |
AC004967.2 | 0.608 | 0.372 | 0.993 | 0.047 |
AC018398.2 | 0 | 0 | 0.847 | 0.046 |
LncRNA, long non-coding RNA; HR, hazard ratio; CI, confidence interval.
Table 2
LncRNA | Coef |
---|---|
LINC01087 | −0.020078 |
AL137847.1 | −0.0849331 |
ELOVL2-AS1 | −0.0660437 |
AL137856.1 | −2.62639 |
LINC01589 | 0.20721027 |
LINC01948 | −0.0719397 |
AP003031.1 | 0.10402237 |
AP001922.3 | 0.44334307 |
MAPT-IT1 | −0.2313379 |
AC004967.2 | −0.2081916 |
LncRNA, long non-coding RNA.
Survival analysis and risk signature verification
Using the established risk signature, we classified the samples into two distinct subgroups: high-risk and low-risk (Figure 3A-3F). Heatmaps showed downregulation of LINC01087, AL137847.1, ELOVL2-AS1, AL137856.1, LINC01948, and AC004967.2 in high-risk groups. LINC01589, AP003031.1, and AP001922.3 were upregulated (Figure 3G-3I). In the high-risk subgroup, Kaplan-Meier (KM) analysis showed an unfavorable prognosis (Figure 3J). Consistently, similar prognostic patterns were observed in both the testing and entire sets (Figures 3K,3L). The reliability of the risk signature was assessed by evaluating the area under the curve (AUC) of the training set (Figure 3M), testing set (Figure 3N), and entire sets (Figure 3O) for 1, 3, and 5 years, with respective AUCs of 0.761/0.725/0.677, 0.733/0.718/0.700 and 0.748/0.723/0.681. Figure 3P-3R depict receiver operating characteristic (ROC) curves, illustrating the performance of clinical and risk signature. These findings provide further evidence to support the robustness and validity of our risk profile in accurately forecasting overall survival outcomes in BC patients.
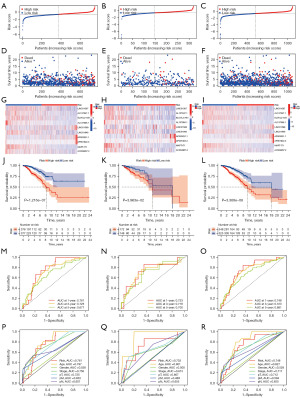
Independent prognostic analysis and establishment of a predictive nomogram
We analyzed the clinical correlation of risk signature and showed that the risk signature created were significantly correlated with pathological T-stage (pT), pathological M-stage (pM), and pathological stage (Figure 4 and Figure S1). The results of the univariate Cox analysis, integrating clinical factors, revealed that age, gender, pT, pN, pM, stage, and the risk score exhibited independent predictive predictors (Figure 5A). Moreover, the multivariate Cox analysis demonstrated that age, stage, and the risk score remained as independent predictors of the outcome (Figure 5B). A nomogram was developed for the prediction of survival of BC patients at 1, 3, and 5 years on the basis of the results of multivariate Cox analysis (Figure 5C) and calibration graphs were drawn for 1-, 3-, and 5-year OS probabilities (Figure 5D). The C-index of the nomogram was 0.783. The C-index plot learns that the predictive power of this risk profile is superior (Figure 5E). The calibration curves demonstrated a close alignment between the predicted values of 1-, 3-, and 5-year survival times and the corresponding true survival times. This observation underscores the high predictive accuracy and reliability of the developed nomogram.
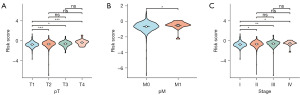
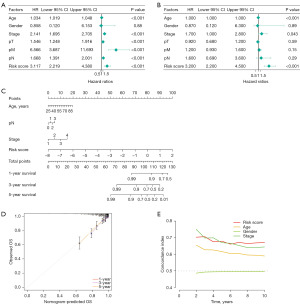
TMB analyses
The TMB was statistically greater in the high-risk group compared to the low-risk group (Figure 6A), and the TMB is positively and substantially correlated with the risk score (Figure 6B). The fifteen genes with the highest mutation rates also differed between the two groups (Figure 6C,6D). PI3KCA and CDH1, which are considered BC oncogenes, and GATA3, which is considered a potential BC biomarker, were mutated with increased frequency in the low-risk group (16-18). The high-risk group demonstrated an elevated frequency of mutations in TP53 and TTN genes, both of which are known to be associated with BC development (19,20). To compare the predictive abilities of the risk signature and TMB on survival outcomes, the sample population was stratified into high-TMB and low-TMB subgroups using the median TMB value. Patients in the high-TMB group and those in the low-TMB group did not have significantly different survival rates (Figure 6E). Compared to the high-TMB-low-risk group, the high-TMB-high-risk group had poorer survival rates (Figure 6F), indicating that the prediction model is quite accurate in predicting BC survival.
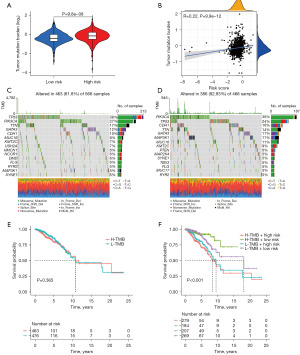
TME analysis
The correlation between immune cell infiltration and immune function in the TME of two groups was assessed using the ssGSEA method. Activated CD4 T cell, activated dendritic cell, CD56bright natural killer cell, central memory CD4 T cell, gamma delta T cell, natural killer T cell, T follicular helper cell, Tregs, type 1 T helper cell, type 17 T helper cell, type 2 T helper cell, among others, obtained higher ssGSEA scores in the higher group (Figure 7A). Meanwhile, the high-risk group exhibited elevated ssGSEA scores for immune function-related genes in the C-C chemokine receptor (CCR) and antigen-presenting cells (APC) co-stimulation pathways. But type II interferon (IFN) response in the low-risk group obtained higher ssGSEA scores (Figure 7B).
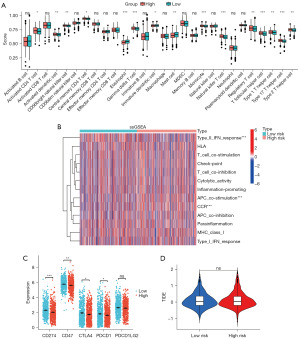
In addition, we analyzed the expression of five classical immune checkpoint genes in the high-risk and low-risk groups. The expression levels of classical immune checkpoint genes, including CD274, CD47, CTLA-4, and PDCD1, were found to be significantly higher in the low-risk group compared to the high-risk group (Figure 7C). Unfortunately, we did not find a significant difference in TIDE scores between the high- and low-risk groups (Figure 7D).
Functional enrichment analysis
To explore potential distinctions between the high-risk and low-risk groups, enrichment analysis of DEGs was conducted. The enriched GO terms for DEGs primarily included epidermis development, skin development, synaptic membrane, intermediate filament cytoskeleton, intermediate filament, signaling receptor activator activity, receptor ligand activity, and serine hydrolase activity (Figure 8A). KEGG pathway enrichment analysis revealed that the DEGs were primarily involved in neuroactive ligand-receptor interaction, salivary secretion and IL-17 signaling pathway (Figure 8B).
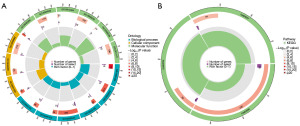
Screening of sensitive anticancer drugs
We identified 55 anticancer drugs whose sensitivities showed a significant correlation with our signature. Among these drugs, 31 drugs showed high sensitivity in the low-risk group, while 21 drugs showed high sensitivity in the high-risk group (Figure 9 and Figure S2).
Discussion
Although the mortality rate of BC patients has declined due to early detection and improved treatment methods, the incidence rate is still increasing year by year, becoming the cancer with the highest incidence rate in the world. Finding new treatments that benefit patients is one direction scientists are pursuing. Disulfidptosis is a newly discovered way of cell death. In 2020, researchers found that the process of reducing cysteine intake into cysteine mediated by SLC7A11 is highly dependent on reduced nicotinamide adenine dinucleotide phosphate (NADPH) generated by the glucose-pentose phosphate pathway (21). Therefore, under glucose deficiency, NADPH in highly expressed cells of SLC7A11 is rapidly depleted and cysteine and other disulfides accumulate abnormally, thus inducing disulfide stress and rapid cell death. However, what kind of cell death type this is and the mechanism of disulfide stress triggering cell death are still unknown. Researchers found in subsequent studies that under glucose deficiency, NADPH in SLC7A11 high-expression cells will be rapidly exhausted, and cysteine and other disulfides will accumulate abnormally, thereby inducing disulfide stress and rapid cell death (Figure 10). Unlike other cell death mechanisms, disulfidptosis is related to the actin cytoskeleton (10,22).
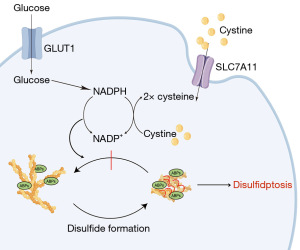
Disulfide metabolism is closely associated with cancer development, as it encompasses redox reactions involving the breakdown and formation of disulfide bonds (23). The impact of oxidative stress on disulfide metabolism has been observed in numerous cancer cells, leading to disturbances in cellular survival and proliferation (24,25). Additionally, disulfide metabolism in cancer cells has been implicated in various biological behaviors, including drug resistance, metastasis, and immune evasion. However, disulfidptosis-associated lncRNAs have been less studied in BC. A study constructed a BC subtype prediction model containing four key lncRNAs (GATA3-AS1, LINC00511, LINC02188, LINC01488) using the random forest model, and found that the modeled key lncRNAs had a strong correlation with immune environment, RNA methylation, and angiogenesis, revealing the potential role of disulfidptosis-associated lncRNAs in BC subtypes (26).
This study established a prognostic model of disulfidptosis-related lncRNA to evaluate the survival rate and death risk of BC patients. We identified disulfidptosis-related lncRNAs from the TCGA database and employed LASSO analysis to develop a prognostic signature comprising 10 lncRNAs. We verified the accuracy and stability of the signature on the inner testing set, and found that the signature has high discriminative and predictive power. The prognostic signature we constructed is not only significantly associated with the survival rate and death risk of BC patients, but also closely related to the immune cell infiltration and TMB of BC patients. Higher risk scores are associated with higher TMB and immune cell infiltration, and study has shown an association between higher TMB and higher survival after immune checkpoint therapy in a variety of tumors (27). Thus, higher risk scores may imply greater sensitivity to immunotherapy. In addition, we also performed potential drug prediction for high and low risk groups and found 55 potential anti-cancer drugs related to the model. Risk scores are used to infer a patient’s sensitivity to immunotherapy and which antitumor agents they may be more sensitive to, thus helping to develop precise clinical treatment strategies.
Multiple studies have demonstrated that ELOVL2-AS1 acts as a novel tumor suppressor factor (28-30). The expression levels of ELOVL2-AS1 vary across different subtypes of BC, with significantly lower expression observed in the highly invasive triple-negative BC (TNBC) compared to the less invasive luminal A subtype. Furthermore, ELOVL2-AS1 expression is notably reduced in BC cells resistant to tamoxifen compared to wild-type cells. Prognostic analysis reveals that BC patients with high ELOVL2-AS1 expression have better outcomes, which is consistent with the findings of this study.
Studies have shown that knocking down the expression of ELOVL2-AS1 can promote the proliferation of BC cells and exacerbate the malignant phenotype of MCF-7 cells resistant to tamoxifen (28,30). Conversely, overexpressing ELOVL2-AS1 significantly inhibits the migration of BC cells and restores the sensitivity of resistant cells to tamoxifen. However, the specific mechanism by which ELOVL2-AS1 inhibits BC is not fully understood. Research suggests that the tumor-suppressing effects of ELOVL2-AS1 may be related to cilium function, and the weakening of cilium function may promote BC growth and metastasis. ELOVL2 catalyzes the synthesis of polyunsaturated very long-chain fatty acids (C20 and C22-PUFA). Reduced expression of ELOVL2, leading to decreased PUFA production, may disrupt tumor ferroptosis balance and favor tumor development and progression. In BC, ELOVL2-AS1 regulates the expression of ELOVL2, and a decrease in ELOVL2-AS1 levels at least partially contributes to the downregulation of ELOVL2, thereby promoting BC progression.
Nevertheless, it is important to acknowledge the limitations of our study. Firstly, our internal validation solely relied on data obtained from the TCGA database, thus necessitating external validation using additional databases to thoroughly assess the generalizability and robustness of our prediction model. Secondly, this study lacks in vivo and in vitro experiments to validate the function of disulfidptosis-related lncRNAs, and lacks the exploration of specific biological mechanisms.
Conclusions
In summary, our study has identified a novel lncRNA risk signature associated with disulfidptosis in BC. The disulfidptosis-associated lncRNA signature holds the potential to provide new insights into the mechanisms underlying the occurrence and progression of BC. The identification of potential anticancer drugs associated with these features may offer benefits to BC patients.
Acknowledgments
We sincerely acknowledge TCGA database for providing their platforms and contributors for uploading their meaningful datasets.
Funding: This work was supported by
Footnote
Reporting Checklist: The authors have completed the TRIPOD reporting checklist. Available at https://tcr.amegroups.com/article/view/10.21037/tcr-24-513/rc
Peer Review File: Available at https://tcr.amegroups.com/article/view/10.21037/tcr-24-513/prf
Conflicts of Interest: All authors have completed the ICMJE uniform disclosure form (available at https://tcr.amegroups.com/article/view/10.21037/tcr-24-513/coif). The authors have no conflicts of interest to declare.
Ethical Statement: The authors are accountable for all aspects of the work in ensuring that questions related to the accuracy or integrity of any part of the work are appropriately investigated and resolved. The study was conducted in accordance with the Declaration of Helsinki (as revised in 2013).
Open Access Statement: This is an Open Access article distributed in accordance with the Creative Commons Attribution-NonCommercial-NoDerivs 4.0 International License (CC BY-NC-ND 4.0), which permits the non-commercial replication and distribution of the article with the strict proviso that no changes or edits are made and the original work is properly cited (including links to both the formal publication through the relevant DOI and the license). See: https://creativecommons.org/licenses/by-nc-nd/4.0/.
References
- Sung H, Ferlay J, Siegel RL, et al. Global Cancer Statistics 2020: GLOBOCAN Estimates of Incidence and Mortality Worldwide for 36 Cancers in 185 Countries. CA Cancer J Clin 2021;71:209-49. [Crossref] [PubMed]
- Promny T, Kutz CS, Jost T, et al. An In Vitro Approach for Investigating the Safety of Lipotransfer after Breast-Conserving Therapy. J Pers Med 2022;12:1284. [Crossref] [PubMed]
- Wang L, Yu X, Wang C, et al. The anti-ErbB2 antibody H2-18 and the pan-PI3K inhibitor GDC-0941 effectively inhibit trastuzumab-resistant ErbB2-overexpressing breast cancer. Oncotarget 2017;8:52877-88. [Crossref] [PubMed]
- Yang H, Xue M, Su P, et al. RNF31 represses cell progression and immune evasion via YAP/PD-L1 suppression in triple negative breast Cancer. J Exp Clin Cancer Res 2022;41:364. [Crossref] [PubMed]
- Arneth B. Tumor Microenvironment. Medicina (Kaunas) 2019;56:15. [Crossref] [PubMed]
- Qiu SQ, Waaijer SJH, Zwager MC, et al. Tumor-associated macrophages in breast cancer: Innocent bystander or important player? Cancer Treat Rev 2018;70:178-89. [Crossref] [PubMed]
- Lan HR, Du WL, Liu Y, et al. Role of immune regulatory cells in breast cancer: Foe or friend? Int Immunopharmacol 2021;96:107627. [Crossref] [PubMed]
- Hu D, Li Z, Zheng B, et al. Cancer-associated fibroblasts in breast cancer: Challenges and opportunities. Cancer Commun (Lond) 2022;42:401-34. [Crossref] [PubMed]
- Fernández-Nogueira P, Mancino M, Fuster G, et al. Tumor-Associated Fibroblasts Promote HER2-Targeted Therapy Resistance through FGFR2 Activation. Clin Cancer Res 2020;26:1432-48. [Crossref] [PubMed]
- Liu X, Nie L, Zhang Y, et al. Actin cytoskeleton vulnerability to disulfide stress mediates disulfidptosis. Nat Cell Biol 2023;25:404-14. [Crossref] [PubMed]
- Lan Y, Xiao X, He Z, et al. Long noncoding RNA OCC-1 suppresses cell growth through destabilizing HuR protein in colorectal cancer. Nucleic Acids Res 2018;46:5809-21. [Crossref] [PubMed]
- Bhan A, Soleimani M, Mandal SS. Long Noncoding RNA and Cancer: A New Paradigm. Cancer Res 2017;77:3965-81. [Crossref] [PubMed]
- Boehm BE, York ME, Petrovics G, et al. Biomarkers of Aggressive Prostate Cancer at Diagnosis. Int J Mol Sci 2023;24:2185. [Crossref] [PubMed]
- Chi H, Peng G, Yang J, et al. Machine learning to construct sphingolipid metabolism genes signature to characterize the immune landscape and prognosis of patients with uveal melanoma. Front Endocrinol (Lausanne) 2022;13:1056310. [Crossref] [PubMed]
- Maeser D, Gruener RF, Huang RS. oncoPredict: an R package for predicting in vivo or cancer patient drug response and biomarkers from cell line screening data. Brief Bioinform 2021;22:bbab260. [Crossref] [PubMed]
- Ge S, Wang D, Kong Q, et al. Function of miR-152 as a Tumor Suppressor in Human Breast Cancer by Targeting PIK3CA. Oncol Res 2017;25:1363-71. [Crossref] [PubMed]
- Xu QT, Wang ZW, Cai MY, et al. A novel cuproptosis-related prognostic 2-lncRNAs signature in breast cancer. Front Pharmacol 2023;13:1115608. [Crossref] [PubMed]
- Guo Y, Yu P, Liu Z, et al. Prognostic and clinicopathological value of GATA binding protein 3 in breast cancer: A systematic review and meta-analysis. PLoS One 2017;12:e0174843. [Crossref] [PubMed]
- Zheng QX, Wang J, Gu XY, et al. TTN-AS1 as a potential diagnostic and prognostic biomarker for multiple cancers. Biomed Pharmacother 2021;135:111169. [Crossref] [PubMed]
- Badve SS, Gökmen-Polar Y. TP53 Status and Estrogen Receptor-Beta in Triple-Negative Breast Cancer: Company Matters. J Natl Cancer Inst 2019;111:1118-9. [Crossref] [PubMed]
- Liu X, Olszewski K, Zhang Y, et al. Cystine transporter regulation of pentose phosphate pathway dependency and disulfide stress exposes a targetable metabolic vulnerability in cancer. Nat Cell Biol 2020;22:476-86. [Crossref] [PubMed]
- Zheng P, Zhou C, Ding Y, et al. Disulfidptosis: a new target for metabolic cancer therapy. J Exp Clin Cancer Res 2023;42:103. [Crossref] [PubMed]
- Zhao S, Wang L, Ding W, et al. Crosstalk of disulfidptosis-related subtypes, establishment of a prognostic signature and immune infiltration characteristics in bladder cancer based on a machine learning survival framework. Front Endocrinol (Lausanne) 2023;14:1180404. [Crossref] [PubMed]
- Hogg PJ. Biological regulation through protein disulfide bond cleavage. Redox Rep 2002;7:71-7. [Crossref] [PubMed]
- Daly EB, Wind T, Jiang XM, et al. Secretion of phosphoglycerate kinase from tumour cells is controlled by oxygen-sensing hydroxylases. Biochim Biophys Acta 2004;1691:17-22. [Crossref] [PubMed]
- Xia Q, Yan Q, Wang Z, et al. Disulfidptosis-associated lncRNAs predict breast cancer subtypes. Sci Rep 2023;13:16268. [Crossref] [PubMed]
- Valero C, Lee M, Hoen D, et al. The association between tumor mutational burden and prognosis is dependent on treatment context. Nat Genet 2021;53:11-5. [Crossref] [PubMed]
- Zhang X, Gao S, Li Z, et al. Identification and Analysis of Estrogen Receptor α Promoting Tamoxifen Resistance-Related lncRNAs. Biomed Res Int 2020;2020:9031723. [Crossref] [PubMed]
- Zhu M, Zhang J, Li G, et al. ELOVL2-AS1 inhibits migration of triple negative breast cancer. PeerJ 2022;10:e13264. [Crossref] [PubMed]
- Kim HW, Baek M, Jung S, et al. ELOVL2-AS1 suppresses tamoxifen resistance by sponging miR-1233-3p in breast cancer. Epigenetics 2023;18:2276384. [Crossref] [PubMed]