Construction and validation of a novel prognostic model with palmitoylation-related genes for glioblastoma
Highlight box
Key findings
• A novel prognostic risk model consisting of 7 palmitoylation-related signature genes was established to predict the prognosis of glioblastoma multiforme (GBM) patients with superior efficacy.
What is known and what is new?
• Palmitoylation is known to play a critical role in the development and progression of various cancers, including glioma. But the molecular mechanisms involved in palmitoylation and its prognostic significance in GBM are still not fully understood.
• This study constructed and validated a prognostic risk model based on palmitoylation-related genes in GBM, and found that the risk model correlated with prognosis, immune infiltration, drug sensitivity and immunotherapy responses in GBM patients.
What is the implication, and what should change now?
• This model provides valuable guidance for predicting overall survival and tailoring personalized treatment strategies for GBM patients.
Introduction
Glioma is the most common primary malignant tumor of the central nervous system. According to the World Health Organization (WHO) classification, brain gliomas are divided into grades 1–4 based on their molecular biomarkers and histological features (1). The most frequent and malignant form of glioma is glioblastoma multiforme (GBM, WHO grade 4 glioma) (1,2). The standard therapy for glioma patients is the Stupp protocol, which consists of maximal safe surgical resection or a diagnostic biopsy, followed by concurrent chemoradiotherapy and then maintenance chemotherapy, where chemotherapy is comprised of temozolomide (3). GBM has special biological characteristics presenting high heterogeneity, diffusing invasiveness, and capacity to resist conventional therapies. In addition, the existence of biological barriers such as the blood-brain barrier makes this tumor difficult to treat (4). The average survival time for patients with GBM is less than two years (2,5), with a 5-year survival rate of only 7.2% post-diagnosis in the United States (2). Therefore, there is a critical need to comprehensively understand the mechanisms driving GBM development, identify new prognostic markers, and develop more effective treatment approaches.
Post-translational modifications of proteins involve chemical alterations that occur after the translation process. These modifications impact protein structure, function, cellular location, and interactions with other proteins by attaching chemical groups to specific amino acid residues, thereby controlling various cellular processes (6,7). There are numerous types of post-translational modifications, including phosphorylation, acetylation, ubiquitination, glycosylation, methylation, and lipidation, with farnesylation, N-myristoylation, and palmitoylation being common lipid modifications (8). S-palmitoylation, a type of lipid modification, involves the attachment of long-chain fatty acids, such as palmitic acid, to protein cysteine residues through a thioester bond. This dynamic and reversible modification plays a crucial role in regulating protein transport, cellular localization, and stability in organisms (9). In mammalian cells, S-palmitoylation is controlled by a group of enzymes called palmitoyl acyltransferases, which have a zinc finger Asp-His-His-Cys-type (ZDHHC) family that contains 23 distinct genes (10). Several members of the ZDHHC family in humans have been found to be linked to the regulation of GBM tumorigenesis (11-14). Therefore, investigating the role of palmitoylation in GBM presents a valuable research avenue, offering potential new targets and treatment strategies for glioma. A comprehensive understanding of these mechanisms is essential for the development of more effective therapies and the improvement of patient outcomes.
In this study, clusters related to palmitoylation metabolism with distinct prognostic features were established, leading to the categorization of GBM tumor patients into two clusters based on the expression of palmitoylation prognostic genes. Through analysis of differentially expressed genes (DEGs) in these subtypes, characteristic genes specific to GBM were identified. Subsequently, a novel prognostic model for predicting cancer prognosis and treatment response in GBM was developed using palmitoylation signature genes through bioinformatics analysis. The risk score derived from signature genes was proved effective in identifying GBM patients with poor prognosis. Additionally, the study delved into the relationship between palmitoylation signature genes in GBM and the immune microenvironment. These findings are expected to enhance our comprehension of the molecular mechanisms underlying GBM and facilitate the implementation of more personalized therapies for this prevalent disease. We present this article in accordance with the TRIPOD reporting checklist (available at https://tcr.amegroups.com/article/view/10.21037/tcr-24-787/rc).
Methods
Data download
The Cancer Genome Atlas (TCGA) database is currently the largest cancer genetic information database, containing gene expression data, copy number variations, single nucleotide polymorphisms (SNPs), and other data. We obtained the original messenger ribonucleic acid (mRNA) expression data of GBM, collecting a total of 175 specimens, including 5 normal samples and 170 tumor samples. Palmitoylation-related genes from the literature ‘Identification and validation of palmitoylation metabolism-related signature for liver hepatocellular carcinoma’ were used for prognostic analysis. The GEO database, also known as Gene Expression Omnibus, is a gene expression database maintained by the National Center for Biotechnology Information (NCBI). We downloaded the Series Matrix File data file of GSE74187 from the NCBI GEO public database, with annotation file GPL6480 containing expression profile data of 60 patients. Additionally, we downloaded the Series Matrix File data file of GSE83300 from the NCBI GEO public database, with annotation file GPL6480 including expression profile data of 50 patients. Lastly, we extracted data from the GSE138794 data file in the NCBI GEO public database, specifically focusing on 9 GBM patient data for single cell analysis. The study was conducted in accordance with the Declaration of Helsinki (as revised in 2013).
Consensus clustering of palmitoylation genes
The study utilized the consensus clustering method to group GBM based on the expression of palmitoylation genes. This involved 50 iterations of clustering, each iteration including 90% of the samples. The optimal number of clusters was identified by analyzing the characteristics of the cumulative distribution function curve of the consistency score.
Differential expression analysis
The Limma package is a software tool in R designed for conducting differential expression analysis on gene expression profiles to pinpoint notably DEGs across group comparisons. In this study, we utilized the Limma package to investigate variations in the molecular mechanisms of GBM data, focusing on distinguishing DEGs between subtype C1 and C2 samples. The criteria for screening differential genes were set as P value 0.585. Additionally, we generated volcano plots and heat maps to visually represent the differential gene analysis results.
Model construction and prognosis
Genes associated with inter-individual differences were identified and utilized in a Least absolute shrinkage and selection operator (LASSO) regression analysis to develop a prognostic model. By integrating the expression levels of specific genes, a personalized risk score formula was created for each patient, taking into account the estimated regression coefficient from the LASSO analysis. Patients were then categorized into low-risk and high-risk groups based on their individual risk scores, using the median score as the threshold. The divergence in survival outcomes between these groups was evaluated through Kaplan-Meier analysis and compared using the log-rank test. LASSO regression and stratified analysis were employed to investigate the predictive value of risk scores in patient prognosis. Additionally, the accuracy of the model predictions was assessed using receiver operating characteristic (ROC) curve analysis.
Differential gene functional enrichment analysis
This study investigated the biological functions and signaling pathways associated with differential genes. The R package ‘ClusterProfiler’ was utilized to annotate the functional aspects of these genes, providing a comprehensive analysis of their functional relationships. Gene Ontology (GO) and Kyoto Encyclopedia of Genes and Genomes (KEGG) were employed to assess relevant functional categories. Pathways enriched in both GO and KEGG with P values and q-values less than 0.05 were deemed significant.
Immune cell infiltration analysis
The CIBERSORT method is a widely utilized approach for assessing immune cell types within the microenvironment. This method is grounded in support vector regression principles and conducts deconvolution analysis on the expression matrix of various immune cell subtypes. With a repertoire of 547 biomarkers, it can effectively differentiate 22 human immune cell phenotypes, encompassing T cells, B cells, plasma cells, and various myeloid cell subsets. In this study, the CIBERSORT algorithm is employed to analyze patient data, enabling the inference of relative proportions of 22 immune infiltrating cell types. Additionally, correlation analysis is conducted between gene expression and immune cell content.
Drug sensitivity analysis
Utilizing data from the GDSC (Genomics of Drug Sensitivity in Cancer) database, we employed the R package ‘pRRophetic’ to predict the sensitivity of tumor samples to chemotherapy. Through regression analysis, we estimated the half-maximal inhibitory concentration (IC50) values for each chemotherapy drug treatment. The accuracy of these predictions was evaluated through 10-fold cross-validation using the GDSC training set. Default parameters, such as ‘combat’ for batch effect removal and averaging duplicate gene expression, were utilized in this analysis.
Gene Set Variation Analysis (GSVA) analysis
GSVA is a non-parametric and unsupervised approach used to assess transcriptome gene set enrichment. By scoring the gene set of interest, GSVA translates gene-level changes into pathway-level changes, enabling the determination of the biological function of the sample. In this study, gene sets will be downloaded from the Molecular Signatures Database (version 7.0) and the GSVA algorithm will be applied to comprehensively score each gene set, allowing for the evaluation of potential biological function changes in various samples.
Gene Set Enrichment Analysis (GSEA) analysis
Patients were categorized into high and low risk groups based on the model’s risk assessment. GSEA was then conducted to examine the variations in signaling pathways between these two groups. The background gene set utilized for this analysis was obtained from the MsigDB database, specifically focusing on annotated gene sets related to subtype pathways. Pathway differential expression analysis was carried out, and significantly enriched gene sets were identified using a consistency score (adjusted P value <0.05). GSEA analysis is frequently employed to link tumor classification with biological relevance.
Nomogram model construction
Nomogram construction is rooted in regression analysis, where gene expression and clinical symptoms are utilized to draw line segments with corresponding scales on a plane. These segments represent the interplay between different variables in the predictive model. Through the development of a multifactor regression model, a score is assigned to each level of an influencing factor based on its contribution to the outcome variable (determined by the regression coefficient). The individual scores are then summed to derive a total score, which is used to calculate the predicted value.
Regulatory network analysis of important genes
This study utilized the R package ‘RcisTarget’ to predict transcription factors (TFs). The calculations conducted by RcisTarget are reliant on motifs. The normalized enrichment score (NES) of a motif is influenced by the overall number of motifs in the database. Apart from the motifs documented in the source data, this study also generated additional annotation files through motif similarity and gene sequence analysis. The initial step in assessing the overexpression impact of each motif on a gene set involves computing the area under the curve (AUC) for each motif-motif set pair. This calculation was based on recovery curve analyses of the gene set in relation to the motif order. The NES for each motif is determined by the AUC distribution of all motifs within the gene set.
Single cell analysis
The data were initially processed using the Seurat package, followed by analysis with the t-distributed stochastic neighbor embedding (tSNE) algorithm to determine the positional relationships between each cluster. Subsequently, the clusters were annotated using the celldex package, focusing on cells that play a significant role in tumor development. Marker genes for each cell subtype were then extracted from the single-cell expression profile by setting the logfc.threshold parameter of FindAllMarkers to 1.
Statistical analysis
Survival curves were generated using the Kaplan-Meier method and compared using the log-rank test. Multivariate analysis was conducted using the Cox proportional hazards model. All statistical analyses were carried out using R language (version 4.3.0), with a significance level set at P<0.05.
Results
Identification of prognosis-related genes
Clinical information of GBM patients from the TCGA database was collected and palmitoylation-related genes were used to identify prognostic genes in GBM through Cox single-factor regression analysis. The findings revealed 5 prognosis-related genes (P value <0.05) identified through Cox single-factor regression analysis (Figure 1A).
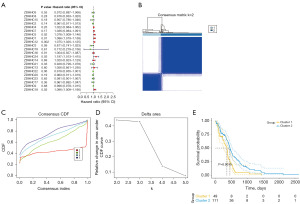
Consensus clustering of palmitoylation genes
The tumor group was molecularly classified using a consistent clustering method based on the expression of palmitoylation prognostic genes (Figure 1B-1D). Our results indicated that with k=2, the boundaries of the two subtypes of samples were closely defined, effectively dividing GBM patients into two clusters, named cluster 1 and cluster 2 (Table S1). Subsequently, survival analysis was conducted on the identified subtypes (Figure 1E), revealing a significant difference in survival rates between the subtypes (P<0.05).
Differential expression analysis and functional enrichment analysis
This study utilized the limma package to conduct differential analysis on clusters C1 and C2 in the expression profile. The criteria for differential gene screening were set as P<0.05 and |log[fold change (FC)]| >0.585. A total of 570 differential genes were identified as palmitoylation-related signature genes (table available at https://cdn.amegroups.cn/static/public/tcr-24-787-1.pdf), with 284 up-regulated genes and 286 down-regulated genes (Figure 2A,2B). Subsequently, pathway analysis was carried out on these differential genes. The GO results indicated enrichment in pathways such as extracellular matrix organization, glial cell differentiation, and cell-substrate adhesion (Figure 2C). In the meanwhile, KEGG analysis revealed enrichment in pathways like PI3K-Akt signaling, tumor necrosis factor (TNF) signaling, and p53 signaling pathways (Figure 2D).
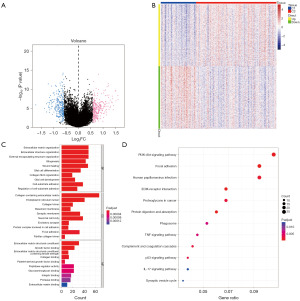
Model construction and verification
Based on the differential genes identified in the previous step, we used the LASSO regression feature selection algorithm to screen for characteristic genes in GBM. The processed GBM dataset from the TCGA database, including survival data, was randomly divided into a training set and a validation set at a 4:1 ratio. LASSO regression analysis was performed to obtain data for each sample (refer to Figure 3A-3C). The optimal risk score was calculated as risk score = FERMT1 × (−0.115012368817603) + TIMP4 × (−0.0579900792698844) + CA14 × (−0.0370831299137056) + UPP1 × 0.0388599956862593 + SOD3 × 0.0481153351393476 + IGFBP6 × 0.0733554222478931 + COL22A1 × 0.089235983107718. Patients were stratified into high-risk and low-risk groups based on these scores and analyzed using Kaplan-Meier curves. The overall survival of the high-risk group in both the training and test sets was notably lower than that of the low-risk group (refer to Figure 3D,3E). Furthermore, the ROC curve analysis of both the training and test sets demonstrated good model validation performance (refer to Figure 3F,3G).
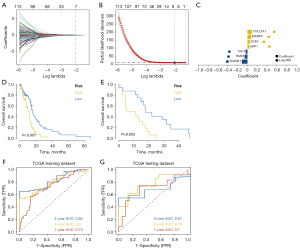
Validation of model in external datasets
Downloaded data from GSE74187 and GSE83300 in the GEO database were used to predict the clinical classification of GBM patients. Kaplan-Meier analysis was employed to evaluate the survival difference between high-risk and low-risk groups, demonstrating significantly lower overall survival in the high-risk group (Figure 4A,4B). ROC curve analysis on external datasets confirmed the model’s strong predictive performance in prognosis prediction (Figure 4C,4D).
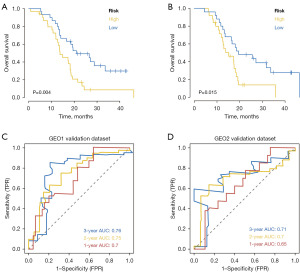
Validation of model in clinical samples
The risk score corresponding to the sample was divided into different groups based on the size of the clinical indicator value. The results of each clinical indicator group were presented in the form of a box plot (Figure 5A-5C), and the risk score was determined using the rank sum test. A significant difference in the distribution of values was observed between groups in the clinical indicator Fustat (P value <0.05), indicating that the risk score derived from modeling analysis is applicable for typing GBM samples. Furthermore, the risk score was identified as an independent prognostic factor in patients with GBM through single-factor and multi-factor analysis (Figure 5D,5E).
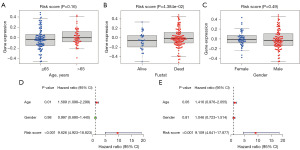
Immune cell infiltration analysis
The tumor microenvironment is a complex network of tumor-associated fibroblasts, immune cells, extracellular matrix components, growth factors, and inflammatory factors, all of which interact with cancer cells. This microenvironment plays a crucial role in tumor diagnosis, survival rates, and treatment responses. Through an analysis of the relationship between risk scores and immune cell infiltration in gliomas, this study aims to uncover the molecular mechanisms underlying tumor progression. The comparison of immune cell proportions between high and low-risk groups is illustrated in Figure 6A. Additionally, the study examines variations in immune cell content between these groups, revealing significant differences in mast cells resting, monocytes, neutrophils, natural killer (NK) cells activated, T cells CD4 memory activated, T cells follicular helper, and T cells regulatory (Tregs) as depicted in Figure 6B. Furthermore, the investigation highlights the positive correlation between risk scores and Tregs, T cells CD4 memory activated, NK cells resting, and neutrophils, while showing a negative correlation with NK cells activated and Mast cells resting as shown in Figure 6C. By exploring immune regulatory genes from the TISIDB database, including immunosuppressive factors, immune stimulating factors, chemokines, major histocompatibility complex and chemokine receptors, the study evaluates the differences in these factors between high- and low-risk groups as presented in Figure 7.
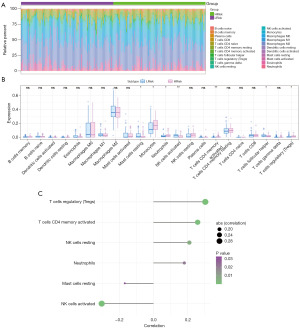
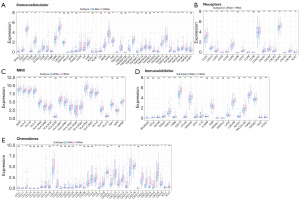
Drug sensitivity analysis, GSVA analysis and GSEA analysis
The study investigates the treatment effect of early-stage glioma with surgery and chemotherapy, utilizing drug sensitivity data from the GDSC database. The R package ‘pRRophetic’ is used to predict chemotherapy sensitivity in tumor samples, examining risk scores and sensitivity to common chemotherapy drugs. Results indicate a significant correlation between risk score levels and sensitivity to AZD8055, BAY.61.3606, BIRB.0796, BI.D1870, CCT007093, and CEP.701 drugs (Figure 8A). Further analysis delves into the signaling pathways associated with high and low-risk models, uncovering potential molecular mechanisms influencing tumor progression. GSVA results highlight differential pathways like IL2_STAT5_SIGNALING, P53_PATHWAY, and IL6_JAK_STAT3_SIGNALING between patient groups (Figure 8B). GSEA results reveal involvement in pathways such as galactose metabolism, TNF signaling pathway, and IL-17 signaling pathway (Figure 8C). The molecular interaction network for each pathway is depicted in the figure (Figure 8D).
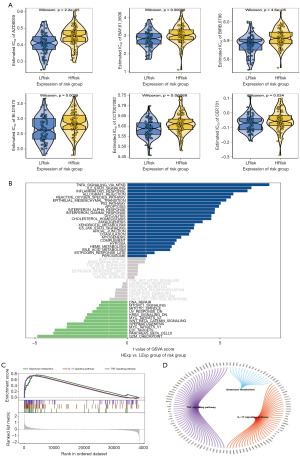
The construction of nomogram
The study categorizes the samples into high-risk and low-risk groups based on the median risk score value and presents the results of their regression analysis through a nomogram. The logistic regression analysis findings indicate a substantial impact of the risk score value on the nomogram prediction model scoring process across all samples (Figure 9A). Additionally, prediction analyses were conducted for both one-year and three-year periods of GBM (Figure 9B), accompanied by the visualization of ROC and decision curve analysis (DCA) (Figure 9C,9D).
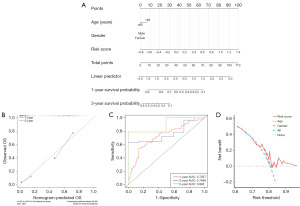
Regulatory network analysis of important genes
This study utilized a gene set consisting of 7 model genes for analysis, revealing that they are regulated by common mechanisms involving multiple TFs. The Motif-TF annotation and selection analysis identified cisbp__M1669 as the motif with the highest NES (NES: 6.23). Visualization of all enriched motifs and corresponding TFs of key genes was performed using Cytoscape (Figure 10A,10B). Subsequently, the study predicted the sensitivity of anti-tumor immunotherapy in high- and low-risk groups, with results indicating a poorer response to immunotherapy in the high-risk group (Figure 10C).
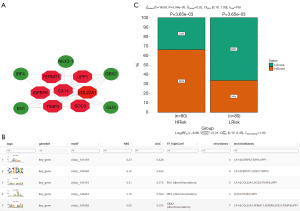
Single cell analysis
The tSNE algorithm was utilized in this study to conduct cell clustering, resulting in the identification of 17 subtypes (Figure 11A). Subsequently, the R package SingleR was employed to annotate each subtype, with 17 clusters being categorized into astrocyte, macrophage, monocyte, and endothelial cells (Figure 11B). As Figure 11C,11D illustrated, FERMT1 was predominantly expressed in astrocytes; TIMP4 and SOD3 were mostly observed in astrocytes and endothelial cells; UPP1 was mainly seen in astrocytes, macrophages, and endothelial cells; whereas IGFBP6 was mainly found in endothelial cells.
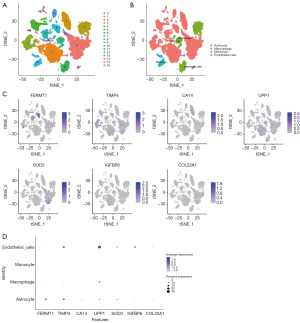
Discussion
GBM is the most common primary brain tumor, presenting unique challenges to therapy due to its aggressive behavior and infiltrative growth, leading to high morbidity and mortality rates (15). It is essential to investigate new prognostic markers and tailor therapeutic strategies accordingly (16). The complexity and heterogeneity of GBM make accurate prognosis prediction challenging with single gene analysis alone, leading to a focus on combined molecular markers. Genome sequencing has provided vast biological data that enhance prognostic information, complementing traditional WHO classification criteria (17). Numerous researchers have developed gene prognostic models for GBM using various bioinformatics analysis methods, showing promising predictive performance (17-20). However, the palmitoylation of GBM prognostic models remains unexplored. This study aims to investigate the molecular subtypes associated with GBM palmitoylation and key factors influencing prognosis assessment.
According to the relevant literature, GBM cells have high levels of free fatty acids to enhance post-translational modifications, such as palmitoylation, which play important roles in tumor cell function (21). Meanwhile, palmitoylation affects various proteins and is involved in metabolic and cancer-promoting pathways (22). The research by Zhang et al. has showed that ZDHHC9 contributes to the malignant progression of GBM cell lines by facilitating glucose transporter GLUT1 palmitoylation both in vivo and in vitro (12). Additionally, research by Chen et al. has revealed that Oct4A palmitoylation, mediated by ZDHHC17, modulates tumorigenicity and stemness in human GBM cells (13). Targeting protein palmitoylation appears to be a promising strategy for GBM therapy.
In this study, GBM patients were categorized into two clusters based on palmitoylation metabolism, each with distinct prognoses. By identifying differential genes in these clusters, we were able to pinpoint characteristic genes in GBM using the LASSO regression feature selection algorithm. Subsequently, a prognostic risk score model for GBM patients was successfully developed, incorporating 7 palmitoylation signature genes. Unfavorable genes associated with poor overall survival were identified as COL22A1, IGFBP6, SOD3, and UPP1, while favorable genes linked to better overall survival included CA14, TIMP4, and FERMT1. ROC curves, survival curves and nomograms results indicated that this model had a good ability to predict the prognosis of GBM patients. Furthermore, single-factor and multi-factor analysis found that the risk score was an independent prognostic indicator in patients with GBM.
COL22A1, a small quantifiable collagen from the fibril associated collagen family with interrupted triple helices, has been linked to maintaining vascular stability and potential association with intracranial aneurysms (23). Overexpression of COL22A1 has been shown to promote the progression of grade 4 diffuse gliomas in glioma U87 and LN18 cells (24). SOD3, also known as superoxide dismutase 3, has been investigated for its involvement in GBM. Research suggests that elevated SOD3 levels are associated with a poor prognosis in GBM patients. Knockdown of SOD3 has been shown to reduce M2-like macrophage polarization, potentially hindering tumor progression. Targeting SOD3 could be a promising strategy for GBM treatment by modifying the tumor microenvironment (25). Additionally, UPP1, or uridine phosphorylase 1, is among the upregulated genes in GBM. Its overexpression, along with other c-Jun targets like FOSL2 and GPR3, is linked to worse prognosis in GBM patients, suggesting UPP1’s contribution to tumor aggressiveness and its potential as a prognostic marker for GBM (26).
CA14, or carbonic anhydrase 14, demonstrates higher expression levels in normal tissue compared to tumor tissue. It plays a role in nitrogen metabolism and is included in a gene signature utilized for predicting disease-free survival (27). Various researches support its potential as a biomarker for disease prognosis (28-30). TIMP-4, or tissue inhibitor of metalloproteinases 4, is found in gliomas where its expression negatively correlates with tumor aggressiveness. It mainly resides in tumor cells and inhibits invasion. A previous study has shown that TIMP-4 can also hinders angiogenesis (31). FERMT1, also referred to as fermitin family member 1, has been investigated in the cancer context. Research suggests that in oral squamous cell cancer, silencing FERMT1 suppresses epithelial-mesenchymal transition and invasion by deactivating the PI3K/Akt signaling pathway, a pathway of significance in GBM (32). However, the specific role of FERMT1 in glioma pathogenesis or prognosis is yet to be fully explored, as there is currently no published evidence on this topic (27).
Research on immune infiltration in gliomas is rapidly advancing, shedding light on the complex relationship between the immune system and tumor development (33). The particular interest is the connection between immune infiltration and GBM, the most aggressive form of glioma. GBMs are known for their profound immune suppression, which is influenced by factors like immune checkpoint molecules, cytokines, and regulatory T cells. This immunosuppressive environment enables tumor growth and allows evasion of immune surveillance. On the other hand, there is evidence to suggest that certain immune cell subsets, such as cytotoxic T cells and M1-polarized macrophages, exhibit anti-tumor properties and can contribute to tumor regression (33,34). Recognizing the prognostic significance of immune infiltration in GBM is essential for the development of effective treatments and the enhancement of patient outcomes. Our study reveals a positive correlation between risk score and immunosuppressive Tregs, activated CD4 memory T cells, resting NK cells, and neutrophils in the complex immune landscape of GBM. These results support previous research on the role of Tregs in immune evasion and the potential of activated CD4 memory T cells in tumor growth (35-37). The presence of resting NK cells and neutrophils indicates an immunosuppressive tumor microenvironment, while the negative correlation with activated NK cells and resting mast cells suggests possible anti-tumor functions for these cells (38,39). Activated NK cells exhibit cytotoxic effects against tumor cells, and resting mast cells may inhibit tumor proliferation and migration (37,40). Our findings underscore the intricate interactions among immune cell subsets in GBM progression, providing valuable insights for potential therapeutic interventions to modulate the immune response and enhance patient outcomes. Our study on prognostic model genes in GBM has highlighted their importance in predicting patient outcomes. Identified through rigorous bioinformatics analyses, these genes play key roles in various biological pathways involved in GBM progression. By using the expression profiles of these prognostic genes, researchers can generate risk scores to categorize patients into different prognostic groups. These risk scores reflect the activation or suppression of specific signaling pathways that contribute to glioma development, such as the PI3K-Akt, TNF, and p53 pathways. Understanding the molecular complexities of these pathways not only improves our knowledge of glioma pathogenesis but also reveals potential targets for therapeutic interventions.
However, there are certain limitations in this study. Firstly, it relied on bioinformatics methods and retrospective data, potentially introducing biases. Secondly, functional experiments are needed to confirm the roles of immune cell subsets identified. Future research should address these limitations to better understand the immune dysregulation in GBM.
Conclusions
Our study has successfully constructed a palmitoylation-related risk prediction signature for the prognosis of GBM using bioinformatics methods. Furthermore, relationships between risk scores, immune infiltration, drug sensitivity and immunotherapy responses were revealed. These findings offer valuable insights for GBM classification, prognosis, and the development of personalized treatment strategies.
Acknowledgments
Funding: This research was funded by grants from
Footnote
Reporting Checklist: The authors have completed the TRIPOD reporting checklist. Available at https://tcr.amegroups.com/article/view/10.21037/tcr-24-787/rc
Peer Review File: Available at https://tcr.amegroups.com/article/view/10.21037/tcr-24-787/prf
Conflicts of Interest: All authors have completed the ICMJE uniform disclosure form (available at https://tcr.amegroups.com/article/view/10.21037/tcr-24-787/coif). The authors have no conflicts of interest to declare.
Ethical Statement: The authors are accountable for all aspects of the work in ensuring that questions related to the accuracy or integrity of any part of the work are appropriately investigated and resolved. The study was conducted in accordance with the Declaration of Helsinki (as revised in 2013).
Open Access Statement: This is an Open Access article distributed in accordance with the Creative Commons Attribution-NonCommercial-NoDerivs 4.0 International License (CC BY-NC-ND 4.0), which permits the non-commercial replication and distribution of the article with the strict proviso that no changes or edits are made and the original work is properly cited (including links to both the formal publication through the relevant DOI and the license). See: https://creativecommons.org/licenses/by-nc-nd/4.0/.
References
- Horbinski C, Berger T, Packer RJ, et al. Clinical implications of the 2021 edition of the WHO classification of central nervous system tumours. Nat Rev Neurol 2022;18:515-29. [Crossref] [PubMed]
- Ostrom QT, Patil N, Cioffi G, et al. CBTRUS Statistical Report: Primary Brain and Other Central Nervous System Tumors Diagnosed in the United States in 2013-2017. Neuro Oncol 2020;22:iv1-iv96. [Crossref] [PubMed]
- Jin L, Guo S, Zhang X, et al. Optimal treatment strategy for adult patients with newly diagnosed glioblastoma: a systematic review and network meta-analysis. Neurosurg Rev 2021;44:1943-55. [Crossref] [PubMed]
- Azimi P, Yazdanian T, Ahmadiani A. mRNA markers for survival prediction in glioblastoma multiforme patients: a systematic review with bioinformatic analyses. BMC Cancer 2024;24:612. [Crossref] [PubMed]
- Davis ME. Epidemiology and Overview of Gliomas. Semin Oncol Nurs 2018;34:420-9. [Crossref] [PubMed]
- Zamaraev AV, Kopeina GS, Prokhorova EA, et al. Post-translational Modification of Caspases: The Other Side of Apoptosis Regulation. Trends Cell Biol 2017;27:322-39. [Crossref] [PubMed]
- Patwardhan A, Cheng N, Trejo J. Post-Translational Modifications of G Protein-Coupled Receptors Control Cellular Signaling Dynamics in Space and Time. Pharmacol Rev 2021;73:120-51. [Crossref] [PubMed]
- Williams CAC, Soufi A, Pollard SM. Post-translational modification of SOX family proteins: Key biochemical targets in cancer? Semin Cancer Biol 2020;67:30-8. [Crossref] [PubMed]
- Elliot Murphy R, Banerjee A. In vitro reconstitution of substrate S-acylation by the zDHHC family of protein acyltransferases. Open Biol 2022;12:210390. [Crossref] [PubMed]
- De I, Sadhukhan S. Emerging Roles of DHHC-mediated Protein S-palmitoylation in Physiological and Pathophysiological Context. Eur J Cell Biol 2018;97:319-38. [Crossref] [PubMed]
- Lu F, Shen SH, Wu S, et al. Hypomethylation-induced prognostic marker zinc finger DHHC-type palmitoyltransferase 12 contributes to glioblastoma progression. Ann Transl Med 2022;10:334. [Crossref] [PubMed]
- Zhang Z, Li X, Yang F, et al. DHHC9-mediated GLUT1 S-palmitoylation promotes glioblastoma glycolysis and tumorigenesis. Nat Commun 2021;12:5872. [Crossref] [PubMed]
- Chen X, Niu W, Fan X, et al. Oct4A palmitoylation modulates tumorigenicity and stemness in human glioblastoma cells. Neuro Oncol 2023;25:82-96. [Crossref] [PubMed]
- Wang Z, Wang Y, Shen N, et al. AMPKα1-mediated ZDHHC8 phosphorylation promotes the palmitoylation of SLC7A11 to facilitate ferroptosis resistance in glioblastoma. Cancer Lett 2024;584:216619. [Crossref] [PubMed]
- Abbruzzese C, Persico M, Matteoni S, et al. Molecular Biology in Glioblastoma Multiforme Treatment. Cells 2022;11:1850. [Crossref] [PubMed]
- Ostrom QT, Gittleman H, Liao P, et al. CBTRUS Statistical Report: Primary brain and other central nervous system tumors diagnosed in the United States in 2010-2014. Neuro Oncol 2017;19:v1-v88. [Crossref] [PubMed]
- Zhang B, Xie L, Liu J, et al. Construction and validation of a cuproptosis-related prognostic model for glioblastoma. Front Immunol 2023;14:1082974. [Crossref] [PubMed]
- Zhou M, Deng Y, Fu Y, et al. A new prognostic model for glioblastoma multiforme based on coagulation-related genes. Transl Cancer Res 2023;12:2898-910. [Crossref] [PubMed]
- Lei C, Chen W, Wang Y, et al. Prognostic Prediction Model for Glioblastoma: A Metabolic Gene Signature and Independent External Validation. J Cancer 2021;12:3796-808. [Crossref] [PubMed]
- Wang J, Qiu X, Huang J, et al. Development and validation of a novel mitophagy-related gene prognostic signature for glioblastoma multiforme. BMC Cancer 2022;22:644. [Crossref] [PubMed]
- Tang F, Liu Z, Chen X, et al. Current knowledge of protein palmitoylation in gliomas. Mol Biol Rep 2022;49:10949-59. [Crossref] [PubMed]
- Feng R, Cheng D, Chen X, et al. Identification and validation of palmitoylation metabolism-related signature for liver hepatocellular carcinoma. Biochem Biophys Res Commun 2024;692:149325. [Crossref] [PubMed]
- Ton QV, Leino D, Mowery SA, et al. Collagen COL22A1 maintains vascular stability and mutations in COL22A1 are potentially associated with intracranial aneurysms. Dis Model Mech 2018;11:dmm033654. [Crossref] [PubMed]
- Liu H, Zeng Z, Sun P. Prognosis and immunoinfiltration analysis of angiogene-related genes in grade 4 diffuse gliomas. Aging (Albany NY) 2023;15:9842-57. [Crossref] [PubMed]
- Liang X, Wang Z, Dai Z, et al. Oxidative stress is involved in immunosuppression and macrophage regulation in glioblastoma. Clin Immunol 2024;258:109802. [Crossref] [PubMed]
- Roura AJ, Szadkowska P, Poleszak K, et al. Regulatory networks driving expression of genes critical for glioblastoma are controlled by the transcription factor c-Jun and the pre-existing epigenetic modifications. Clin Epigenetics 2023;15:29. [Crossref] [PubMed]
- Lu CH, Wei ST, Liu JJ, et al. Recognition of a Novel Gene Signature for Human Glioblastoma. Int J Mol Sci 2022;23:4157. [Crossref] [PubMed]
- Zhao Y, Tao Z, Li L, et al. Predicting biochemical-recurrence-free survival using a three-metabolic-gene risk score model in prostate cancer patients. BMC Cancer 2022;22:239. [Crossref] [PubMed]
- Lee S, Toft NJ, Axelsen TV, et al. Carbonic anhydrases reduce the acidity of the tumor microenvironment, promote immune infiltration, decelerate tumor growth, and improve survival in ErbB2/HER2-enriched breast cancer. Breast Cancer Res 2023;25:46. [Crossref] [PubMed]
- Wang G, Bie F, Li G, et al. Study of the co-expression gene modules of non-small cell lung cancer metastases. Cancer Biomark 2021;30:321-9. [Crossref] [PubMed]
- Groft LL, Muzik H, Rewcastle NB, et al. Differential expression and localization of TIMP-1 and TIMP-4 in human gliomas. Br J Cancer 2001;85:55-63. [Crossref] [PubMed]
- Wang X, Chen Q. FERMT1 knockdown inhibits oral squamous cell carcinoma cell epithelial-mesenchymal transition by inactivating the PI3K/AKT signaling pathway. BMC Oral Health 2021;21:598. [Crossref] [PubMed]
- Attarha S, Roy A, Westermark B, et al. Mast cells modulate proliferation, migration and stemness of glioma cells through downregulation of GSK3β expression and inhibition of STAT3 activation. Cell Signal 2017;37:81-92. [Crossref] [PubMed]
- Wang G, Zhang Z, Zhong K, et al. CXCL11-armed oncolytic adenoviruses enhance CAR-T cell therapeutic efficacy and reprogram tumor microenvironment in glioblastoma. Mol Ther 2023;31:134-53. [Crossref] [PubMed]
- Kuang Z, Liu X, Zhang N, et al. USP2 promotes tumor immune evasion via deubiquitination and stabilization of PD-L1. Cell Death Differ 2023;30:2249-64. [Crossref] [PubMed]
- Duurland CL, Santegoets SJ, Abdulrahman Z, et al. CD161 expression and regulation defines rapidly responding effector CD4+ T cells associated with improved survival in HPV16-associated tumors. J Immunother Cancer 2022;10:e003995. [Crossref] [PubMed]
- Cui Y, Shen T, Xu F, et al. KCNN4 may weaken anti-tumor immune response via raising Tregs and diminishing resting mast cells in clear cell renal cell carcinoma. Cancer Cell Int 2022;22:211. [Crossref] [PubMed]
- De Lerma Barbaro A, Palano MT, Cucchiara M, et al. Metabolic Rewiring in the Tumor Microenvironment to Support Immunotherapy: A Focus on Neutrophils, Polymorphonuclear Myeloid-Derived Suppressor Cells and Natural Killer Cells. Vaccines (Basel) 2021;9:1178. [Crossref] [PubMed]
- Chen X, Yan L, Lu Y, et al. A Hypoxia Signature for Predicting Prognosis and Tumor Immune Microenvironment in Adrenocortical Carcinoma. J Oncol 2021;2021:2298973. [Crossref] [PubMed]
- Sivori S, Pende D, Quatrini L, et al. NK cells and ILCs in tumor immunotherapy. Mol Aspects Med 2021;80:100870. [Crossref] [PubMed]