Construction of a disulfidptosis-related lncRNAs signature of the subtype, prognostic, and immunotherapy in neuroblastoma
Highlight box
Key findings
• Our study identifies a novel disulfidptosis-related lncRNAs (DRLs) signature that effectively predicts survival, immune response, and drug sensitivity in neuroblastoma (NB).
What is known and what is new?
• The involvement of lncRNAs and disulfidptosis in cancer is significant, with these elements playing crucial roles in tumor progression and patient outcomes.
• This is the first study to establish a DRL signature in NB, providing a novel prognostic model that predicts patient survival and informs personalized treatment strategies, including immunotherapy and drug sensitivity.
What is the implication, and what should change now?
• The DRL signature could be a promising prognostic biomarker for NB, aiding in survival prediction and therapeutic response evaluation. To validate its clinical utility, further research is needed to test this signature in independent datasets and experimental settings.
Introduction
Neuroblastoma (NB), an embryonal tumor originating from neural crest cells, represents a prevalent pediatric malignancy (1). It constitutes 15% of mortality attributed to childhood cancer (2). Ranging from spontaneous regression to aggressive metastasis, NB poses a significant challenge in diagnosis and treatment (3). Current management strategies encompass a multidisciplinary approach, involving surgery, chemotherapy, radiation therapy, and immunotherapy (4,5). However, the intricate heterogeneity of NB introduces distinctions in therapeutic response, resulting in varied prognoses between high and low-risk cases (6). This complexity underscores the pressing need for novel biomarkers and therapeutic targets to refine treatment strategies and enhance outcomes.
In the dynamic landscape of cancer biology, diverse cell death modalities contribute to tumor progression and therapeutic responses (7). Classical mechanisms like apoptosis have been extensively studied, while emerging paradigms such as ferroptosis and cuproptosis have broadened our understanding (8,9). Disulfidptosis is a recently characterized form of regulated cell death resulting from the anomalous accumulation of intracellular disulfides in cells that exhibit overexpression of SLC7A11, particularly in the presence of glucose deprivation (10). Heightened SLC7A11-facilitated cystine uptake, coupled with glucose deprivation, induces pronounced disulfide stress, fostering abnormal disulfide bonding in actin cytoskeleton proteins. This, in turn, results in actin filament contraction and detachment from the plasma membrane (10). Recent studies have highlighted the utility of disulfidptosis in clinical diagnostics for hepatocellular carcinoma, offering predictive insights into prognosis and potential therapeutic targets (11-13). However, the utilization of disulfidptosis in understanding the progression, assessing prognosis, and identifying therapeutic targets in NB still lacks clarity. Comprehending these intricate mechanisms is paramount for elucidating the complexities of the disease and discerning potential therapeutic interventions, particularly within the realm of distinct high and low-risk NB cases.
Long noncoding RNA (lncRNA) is a form of noncoding RNA with a length exceeding 200 nucleotides. LncRNAs form a significant category of transcripts encoded by the genome, yet they are predominantly not translated into proteins (14). LncRNAs have emerged as essential regulators of gene expression, influencing various facets of tumorigenesis (15). In the context of NB, the dysregulation of lncRNAs has been implicated in disease progression (e.g., EZH2), providing valuable insights into its molecular underpinnings (16). An increasing body of research demonstrates that lncRNA can emerge as prognostic biomarkers and potential drug targets for NB patients. Previous studies have found that prognostic models based on disulfidptosis-related lncRNAs (DRLs) have significant prognostic value for survival prediction, immune cell infiltration, immune evasion, and immunotherapy in malignant tumors such as lung adenocarcinoma, human papillomavirus (HPV)-negative oral squamous cell carcinoma, and ovarian cancer (17-19). The exploration of DRLs offers a unique avenue for uncovering potential biomarkers and therapeutic targets, with the potential to address the distinct treatment responses observed in high and low-risk NB cases.
In this study, our objective was to identify DRLs influencing the overall survival (OS) of NB patients. Molecular subtypes of NB were discerned based on the identification of these DRLs. Subsequently, we constructed and validated a prognostic model based on DRLs, demonstrating high accuracy in predicting survival area under the curve (AUC) for 5-year survival: 0.830. In comparison to International Neuroblastoma Staging System (INSS) stage and MYCN proto-oncogene, bHLH transcription factor (MYCN) status, our prognostic model emerged as an independent risk factor. Furthermore, the risk score from this model could be employed to assess the tumor immune microenvironment and sensitivity to immunotherapy and chemotherapy. Our research findings underscore the critical role of DRLs in NB progression, providing a foundation for prognostic assessment and immunotherapeutic efficacy in NB patients. By introducing this innovative lncRNA signature model derived from NB datasets, we are paving a new path for precision medicine to optimize the treatment of NB patients. We present this article in accordance with the TRIPOD reporting checklist (available at https://tcr.amegroups.com/article/view/10.21037/tcr-24-510/rc).
Methods
Data acquisition
The RNA-Seq gene expression data of pediatric NB patients, along with corresponding clinical data, were retrieved from the TARGET (Therapeutically Applicable Research to Generate Effective Treatments, https://ocg.cancer.gov/programs/target) and GEO (Gene Expression Omnibus, https://www.ncbi.nlm.nih.gov/geo/) public databases. The TARGET-NB cohort, consisting of 150 pediatric NB samples with complete clinical data, was selected for inclusion as the training set in this study. The GSE62564 cohort, comprising 498 samples with comprehensive clinical data, served as the validation set. After downloading gene expression data from both datasets, the Combat function from the “SVA” R package was employed for batch correction, aiming to eliminate data biases resulting from diverse experimental setups (20). The latest clinical information for TARGET-NB samples was obtained from the TARGET database, and clinical information for the GSE62564 cohort was gathered from the GEO database. Samples with missing clinical data were excluded from this study. Somatic mutation data for the TARGET-NB cohort were obtained from (https://portal.gdc.cancer.gov/) for subsequent analysis. The study was conducted in accordance with the Declaration of Helsinki (as revised in 2013).
Collection of the disulfidptosis-related genes (DRGs) and lncRNAs
The list of DRGs was collected from the previous study conducted by Liu and their colleagues (10). To identify DRLs, we performed a detailed correlation analysis, setting stringent thresholds (|cor| >0.3, P<0.001) to ensure statistical significance and biological relevance. For visual representation, we employed the “ggplot2” and “ggalluvial” R packages to create an informative Sankey diagram, effectively illustrating the relationships between DRGs and DRLs (21). Univariate Cox regression analysis was employed to identify DRLs with predictive value for patient OS (P<0.01). This rigorous selection process enabled us to identify key DRLs for subsequent in-depth analyses, ensuring the robustness and reliability of our prognostic model.
Unsupervised clustering of NB based on DRLs
The R package “ConsensusClusterPlus” was employed to perform unsupervised clustering based on the expression data of DRLs with significant prognostic value (22). This clustering aimed to recognize distinct DRLs subtypes among NB patients. Unsupervised clustering involved 50 iterations, with an optimal classification range of K=2–9. The optimal number of clusters was determined through cumulative distribution function (CDF) curves and silhouette shadows of consensus clustering. Subsequently, Kaplan-Meier curves were generated using the “survival” R package to explore survival differences among the identified subtypes (23). A significance threshold of P<0.05 was considered statistically significant.
Estimation of stromal and immune scores
The “ESTIMATE” R package is utilized to estimate tumor purity in malignant tumors by evaluating the presence of stromal tissue and immune cells. This methodology involves estimating the proportions of stromal and immune cells within tumor samples by incorporating the expression levels of specific genes. Utilizing gene features associated with immune cells and stromal tissues, the single-sample gene set enrichment analysis (ssGSEA) algorithm is applied to compute immune and stromal scores. Subsequently, the ESTIMATE score is derived based on the stromal and immune scores. The tumor purity is calculated using the formula: Tumor Purity = cos(0.6049872018 + 0.0001467884 × ESTIMATE score) (24).
Tumor infiltrating immune cells analysis
To quantify the proportion of immune cells in each NB sample, we employed six immune cell infiltration estimation methods, including TIMER (25), CIBERSORT (26), quanTIseq (27), xCell (28), EPIC (29), and MCP-counter (30). Specifically, the TIMER algorithm (https://cistrome.shinyapps.io/timer/) provides robust estimation algorithms for the infiltration of six immune cell types, namely B cells, CD4+ T cells, CD8+ T cells, neutrophils, macrophages, and dendritic cells. CIBERSORT (http://cibersort.stanford.edu/) is a gene expression-based method for detecting 22 immune cell types, utilizing linear support vector regression. We set permutations =1,000 for more stable results. The quanTIseq method (https://icbi.i-med.ac.at/software/quantiseq/doc/) quantifies absolute scores for ten immune cell types based on extensive RNA-seq data. xCell (https://xcell.ucsf.edu/) provides abundance scores for 28 Bindea Signatures for each sample using a gene signature-based approach. EPIC (https://gfellerlab.shinyapps.io/EPIC_1-1/) detects components of seven immune cells and cancer cells based on transcriptomic data. The microenvironment cell populations (MCP)-counter algorithm assesses eight immune cells and two stromal cells. Visualization of the data was implemented using the “pheatmap” R package.
ssGSEA
We utilized the ssGSEA algorithm to quantify the enrichment status of samples for 29 immune-related gene sets. These 29 gene sets encompass a broad spectrum of immune cells or immune molecules, providing a comprehensive reflection of innate immunity, antigen presentation, immune co-stimulatory molecules, immune co-inhibitory molecules, and immune checkpoint profiles. The genes within these 29 immune-related gene sets were obtained from previous publications (31-33). Visualization was conducted using the “ggpubr” R package.
Establishment and validation of the DRLs prognostic model
Building the prognostic model involves constructing the model on the training set and validating it using both the test set and the complete set. Drawing on previously identified prognostically relevant DRLs, least absolute shrinkage and selection operator (LASSO) regression analysis was applied to pinpoint lncRNAs with minimal bias (34). Subsequently, a multivariate Cox regression analysis was conducted to establish the prognostic model based on eight DRLs. The computational formula is as follows:
The “Coef” represents the LASSO regression coefficient of the lncRNA, and “Expr” represents the expression level of the gene. Utilizing the median value from the training set, samples from the training set, validation set, and complete set were stratified into high-risk and low-risk groups. Subsequently, within these three sets, Kaplan-Meier survival analysis was employed to differentiate survival differences between the high-risk and low-risk groups. Furthermore, diverse methods were employed to evaluate the model’s accuracy, including receiver operating characteristic (ROC) curves, C-index, nomograms, and calibration curves. The implementation of these analyses utilized R packages such as “glmnet,” “survival,” “survminer,” “timeROC,” and “rms” (35).
Functional enrichment analysis of differentially expressed genes (DEGs)
We employed the “limma” R package to discern DEGs between high and low-risk score groups (|log fold change (FC)|>0.5, P<0.05) (36). To unveil the potential functions of DEGs between these groups, we conducted Gene Ontology (GO) and Kyoto Encyclopedia of Genes and Genomes (KEGG) enrichment analyses. The GO enrichment analysis encompassed three main categories: biological process (BP), cellular component (CC), and molecular function (MF). Furthermore, gene set enrichment analysis (GSEA) was performed based on the gene expression profiles of high and low-risk score groups (37). A gene set was deemed enriched if the P value was below 0.05. These processes were executed using the R packages “org.Hs.e.g.db” and “clusterProfiler” (38).
Tumor mutational burden (TMB) analysis
Based on the total number of somatic base substitutions, we computed the TMB and mutation frequency for each sample. TMB is defined as the number of non-synonymous alterations per million bases in the genome sequence. We analyzed the gene mutation differences between the high-risk and low-risk groups, utilizing the “Maftools” R package for visualizing the mutation frequency of genes in NB patients (39). NB patients were stratified into two groups based on the optimal cut-off derived from the TMB score, and survival analysis was conducted to explore the impact of TMB on OS. Simultaneously, joint analysis of TMB scores and risk score was performed to assess their combined effect on OS.
Immunotherapy response and drug sensitivity prediction
We employed the tumor immune dysfunction and exclusion (TIDE) platform to compute TIDE scores for the samples, with this score negatively correlated with the efficacy of immunotherapy (40). The Tumor Inflammation Signature (TIS), based on an 18-gene signature, was utilized to calculate the suppressed adaptive immune response within the tumor, and this score is positively correlated with the efficacy of immunotherapy (41). By comparing TIDE scores and TIS scores between the high-risk and low-risk groups, we assessed the differences in immunotherapeutic efficacy between the two groups.
The “oncoPredict” R package, based on gene expression data, was employed to predict drug sensitivity for each sample (42). Drug-related data were downloaded from the Genomics of Drug Sensitivity in Cancer database (GDSC) (https://osf.io/c6tfx/). After calculating the sensitivity of each sample to drugs, we compared the differences in sensitivity to commonly used chemotherapy drugs in NB patients between the high-risk and low-risk groups. Drug sensitivity data were downloaded from the CellMiner website (https://discover.nci.nih.gov/cellminer/home.do) (43), and Pearson correlation analysis was utilized for analyzing the cell sensitivity data and target gene data for FDA-approved drugs.
Statistical analysis
All data analyses were conducted using R (4.3.1). The relationship between two continuous variables was explored through Spearman correlation analysis. Survival analysis employed Kaplan-Meier estimation and log-rank tests. For the comparison of paired independent samples, Wilcoxon tests were utilized, while Kruskal-Wallis tests were applied for comparisons involving three or more independent samples. Unless specified otherwise, statistical significance was considered at P<0.05.
Results
Identification of DRLs in NB
The flowchart of our study is shown in (Figure 1). Gene expression data were collected from TARGET-NB (n=150) and GSE62564 (n=498). The datasets were batch-corrected and merged, resulting in a complete set (n=648) for subsequent analyses. Initially, protein-coding mRNAs and lncRNAs were distinguished based on gene type annotations. To identify DRLs, a stringent screening approach was applied to assess the correlation between these lncRNAs and 10 DRGs using Pearson correlation analysis (|Pearson R|>0.3 and P<0.001). A total of 175 DRLs were identified for further analysis, demonstrating significant correlations with 9 out of the 10 DRGs. Notably, LRPPRC, NDUFA11, and SLC3A2 exhibited the most associations with DRLs (Figure 2A; Table S1). Subsequently, univariate cox regression analysis (P<0.01) was conducted on the identified 175 DRLs to assess their relationship with prognosis, leading to the identification of 151 DRLs with significant prognostic value (Table S2).
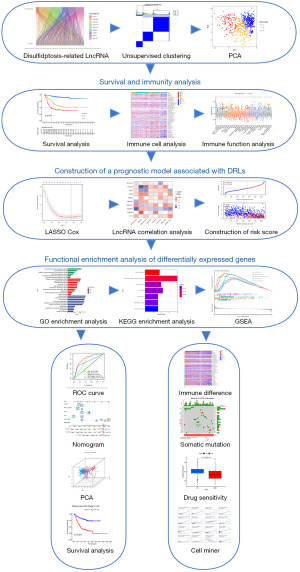
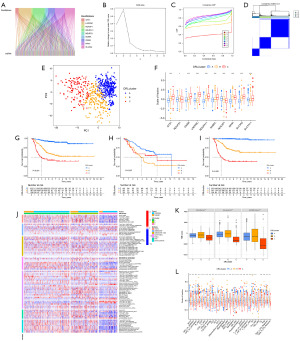
Unsupervised clustering of NB samples based on DRLs and analysis of tumor immune microenvironment
In order to explore molecular subtypes of NB based on prognostic-related DRLs, unsupervised consensus clustering analysis was performed on 648 NB patients, dividing all samples into k (k=2–9) distinct molecular subtypes. Based on the CDF curve of consensus clustering, k=3 showed the best clustering effect (Figure 2B,2C). We named the three subtypes as DRLclusterA (n=322), DRLclusterB (n=197), and DRLclusterC (n=129), respectively. The heatmap results and principal component analysis (PCA) confirmed the better clustering effect at k=3 (Figure 2D,2E). Except for NCKAP1, the expression of the other 9 DRGs was higher in DRLclusterC and lower in DRLclusterA (Figure 2F). Survival curves revealed that DRLclusterA had the best survival, DRLclusterB had intermediate survival, and DRLclusterC had the worst survival, with significant differences among these three subtypes (P<0.001) (Figure 2G). The same results were validated in TARGET-NB and GSE62564 datasets (Figure 2H,2I).
To discern the differences in immune infiltration among distinct DRLs subtypes, we employed various algorithms to analyze the tumor immune cell infiltration across all patients, including TIMER, CIBERSORT, CIBERSORT-ABS, QUANTISEQ, xCell, EPIC, and MCP-counter. The heatmap displays immune cells with significant differences between DRL clusters (P<0.05) (Figure 2J). DRLclusterA exhibited the highest levels of anti-tumor immune cell infiltration, including B cells, T cells CD4+, T cells CD8+, NK cells, neutrophils, macrophages, and myeloid dendritic cells. In contrast, DRLclusterC demonstrated lower infiltration levels in these cells. Figure 2K illustrates that DRLclusterC has lower StromalScore, ImmuneScore, and ESTIMATEScore compared to DRLclusterA and DRLclusterB, with significant differences (P<0.001), indicating weaker immune infiltration in DRLclusterC. Figure 2L depicts the enrichment of 29 immune-related gene sets among the subtypes, revealing that DRLclusterC expresses lower levels in immune-related gene sets, indicating a poorer prognosis.
Establishment of the DRLs prognostic model
To establish a prognostic model for DRLs, we constructed the model using training set and validated it using both validation set and complete set. Only 151 prognosis-related lncRNAs were included in model construction. Utilizing LASSO cox regression analysis based on these 151 prognosis-related DRLs, we developed a prognostic model composed of 8 lncRNAs (Figure 3A,3B) (Table S3). Subsequently, we calculated the risk score for each NB patient using the formula: risk score = [0.162916552 × URB1-AS1 expression value (EV)] − (0.051288179 × CASC15 EV) + (0.013206852 × MIR7-3HG EV) − (0.165981247 × FAM13A-AS1 EV) + (0.159384989 × ATP2A1-AS1 EV) + (0.12844152 × VPS9D1-AS1 EV) + (0.11457447 × SNHG16 EV) + (0.24818803 × TOB1-AS1 EV). Among the eight lncRNAs, CASC15 and FAM13A-AS1 were found to be favorable for prognosis, while the others were associated with unfavorable prognosis (Figure 3C). Figure 3D depicts the correlation between the eight lncRNAs used in constructing the prognostic model and the ten DRGs.
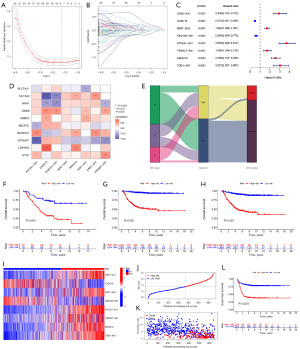
According to the median value of the risk score in the training set, all NB samples were categorized into high-risk and low-risk groups. Figure 3E illustrates that the majority of DRLclusterA members belong to the low-risk group, and most have MYCN not amplification, whereas the majority of DRLclusterC members belong to the high-risk group. Survival analysis results reveal a poorer prognosis for the high-risk group, characterized by lower OS time and a significant difference between the high-risk and low-risk groups (P<0.001) (Figure 3F). Similar survival analysis results were obtained for the validation set and the complete set, both demonstrating significant differences (P<0.001) (Figure 3G,3H). Figure 3I presents the expression heatmap of the 8 DRLs used in model construction between the high-risk and low-risk groups, indicating lower expression of CASC15 and FAM13A-AS1 in the high-risk group, supporting their favorable prognostic role. Individual patient risk scores and survival status are depicted in (Figure 3J,3K), highlighting that higher risk scores are associated with lower survival time. Additionally, in the validation set, higher risk scores predict poorer event-free survival (Figure 3L).
The DRLs prognostic model is an independent prognostic indicator
To validate whether our prognostic model is an independent prognostic factor unaffected by other clinical factors, we included age, gender, INSS stage, and MYCN status in the Cox regression analysis. Univariate Cox regression analysis revealed that the risk score is a significant prognostic factor [P<0.001, hazard ratio (HR): 8.758, 95% confidence interval (CI): 6.245–12.281] (Figure 4A). Multivariate Cox regression analysis confirmed that the risk score is an independent prognostic factor (P<0.001, HR: 4.268, 95% CI: 2.914–6.251) (Figure 4B). To assess the accuracy of the prognostic model in predicting patient outcomes, ROC curve analysis was performed. The AUC values for patient 1-, 3-, and 5-year survival were 0.797, 0.805, and 0.830, respectively (Figure 4C), indicating high accuracy of the prognostic model. Furthermore, a comparison of AUC values between the risk score (AUC =0.805) and other clinical factors, such as age (AUC =0.713), gender (AUC =0.491), INSS stage (AUC =0.710), and MYCN status (AUC =0.700), revealed superior accuracy of the risk score (Figure 4D). C-index analysis demonstrated that the prognostic model has higher sensitivity in predicting NB patient outcomes compared to other clinical parameters (age, gender, INSS stage, MYCN status) (Figure 4E). Additionally, based on the results of univariate and multivariate analyses, we created a nomogram by assigning age, gender, MYCN status, INSS stage, and risk score, revealing the reliable prediction of 1-, 3-, and 5-year survival rates for NB patients (Figure 4F,4G).
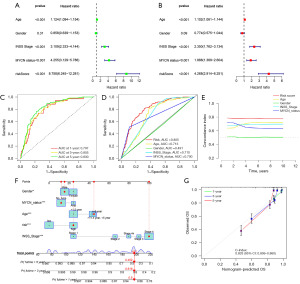
Predicting the prognosis of high-risk and low-risk patients with the clinical characteristics
Based on the DRLs prognostic model, we grouped NB patients according to clinical information such as age, gender, INSS stage, and MYCN status, comparing the survival rates between high-risk and low-risk groups. Kaplan-Meier survival curves demonstrated significant differences between high and low-risk groups in the following cohorts: age <1.5 years (P<0.001, Figure 5A), age ≥1.5 and <5 years (P<0.001, Figure 5B), age ≥5 years (P=0.006, Figure 5C), female (P<0.001, Figure 5D), male (P<0.001, Figure 5E), INSS stage I–II (P<0.001, Figure 5F), INSS stage III–IV (P<0.001, Figure 5G), MYCN: amplification (P=0.008, Figure 5H), MYCN: no amplification (P<0.001, Figure 5I). Therefore, this prognostic model can predict the survival outcomes of NB patients with any clinical features, demonstrating considerable clinical utility irrespective of age, gender, INSS stage, and MYCN status constraints.
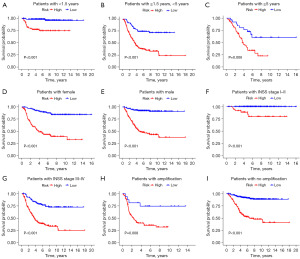
PCA and biological functional analysis
Dimension variables based on the whole genome expression profile, 10 DRGs, 175 DRLs, and 8 model-building lncRNAs are depicted in four independent PCA plots shown in Figure 6A-6D. The results indicate a clear separation in the distribution between the high-risk and low-risk groups in the PCA based on the eight model-building lncRNAs (Figure 6D). Each group exhibits a certain clustering effect, underscoring the efficacy of the risk score in classifying NB patients.
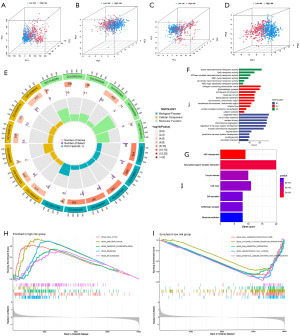
To explore the biological differences between the high-risk and low-risk groups, we conducted differential gene expression analysis, identifying a total of 447 DEGs [|logFC| >0.5, false discovery rate (FDR) <0.05]. Subsequent GO and KEGG enrichment analyses of these DEGs revealed associations with processes such as organelle fission, cell junction assembly, nuclear division, chromosome segregation, regulation of nervous system development, and nuclear chromosome segregation (Figure 6E,6F). KEGG enrichment analysis further indicated that these DEGs are associated with neuroactive ligand-receptor interaction, cell cycle, and Oocyte meiosis (Figure 6G). Additionally, GSEA enrichment analysis was performed separately for the high-risk and low-risk groups (Figure 6H,6I). The top five enriched pathways for the high-risk group were cell cycle, DNA replication, oxidative phosphorylation, ribosome, and spliceosome. Conversely, the top five enriched pathways for the low-risk group included cell adhesion molecules (CAMs), cytokine receptor interaction, extracellular matrix (ECM) receptor interaction, hematopoietic cell lineage, and intestinal immune network for IgA production.
Immunocyte infiltration and somatic cell mutations
The tumor immune microenvironment plays a crucial role in the progression of cancer. Given that the GSEA results above indicate enrichment of immune-related processes in the low-risk group, we hypothesized differences in the tumor immune microenvironment between the high-risk and low-risk groups. Initially, we compared the differences in immune cells between the high-risk and low-risk groups, with the heatmap displaying only immune cells that exhibited significant differences between the groups (P<0.05) (Figure 7A). Notably, the low-risk group demonstrated a higher abundance of anti-tumor immune cell infiltration compared to the high-risk group, including B cells, T cells CD4+, T cells CD8+, NK cells, neutrophils, macrophages, and myeloid dendritic cells. Furthermore, the low-risk group exhibited higher Stromal score, Immune score, and ESTIMATE score, and these scores differed significantly between the high-risk and low-risk groups (P<0.001), indicating a lower immune cell infiltration in the high-risk group (Figure 7B). Figure 7C illustrates the relative percentages of infiltrating immune cells between the high-risk and low-risk groups. The enrichment analysis of 29 immune-related gene sets among the subtypes revealed lower expression in the high-risk group, suggesting a poorer prognosis (Figure 7D). The differences in immune checkpoints between the high-risk and low-risk groups showed elevated expression of the TNF family in the low-risk group, which may be one of the reasons inhibiting tumor progression (Figure 7E). In summary, these results indicate that, based on our DRLs model classification, high-risk NB groups may have defects in immune cell or functional components in their tumor microenvironment, leading to tumor progression and a poorer prognosis.
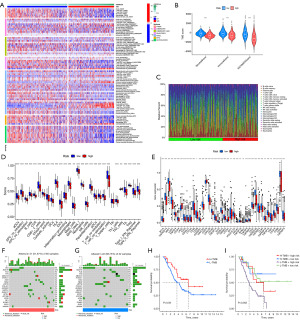
TMB is a crucial factor influencing cancer prognosis. No significant differences in TMB were observed between the high-risk and low-risk groups. Mutations between the high-risk and low-risk groups are depicted in Figure 7F,7G. Notably, ALK gene mutations were significantly higher in the low-risk group, while MUC16 gene mutations were significantly lower than those in the high-risk group. Further analysis of TMB’s predictive role in OS revealed that when the samples were stratified into H-TMB (high TMB) and L-TMB (low TMB) groups based on the optimal cutoff of TMB values, no significant differences were observed in OS prediction using only the TMB grouping (P=0.08, Figure 7H). However, when combining TMB and risk score for joint grouping, we observed that the H-TMB combined with the low-risk group had a better prognosis, while the L-TMB combined with the high-risk group had the worst prognosis, with statistically significant differences (P=0.002, Figure 7I).
Predicting sensitivity to immunotherapy and other antitumor drugs
To explore the role of our DRLs model in predicting immunotherapy response, we initially analyzed PD-L1 differences between high-risk and low-risk groups (Figure 8A). PD-L1 exhibited higher expression in the low-risk group, suggesting a potentially favorable immunotherapy response. Subsequent comparisons of TIDE and TIS differences between DRLclusters revealed that DRLclusterC had higher TIDE and lower TIS scores, indicating a poorer response to immunotherapy (Figure 8B,8C). These differences between high-risk and low-risk groups further supported these observations, with the high-risk group showing higher TIDE scores and lower TIS scores, signifying a poorer immunotherapy response and prognosis, consistent with prior research (Figure 8D,8E).
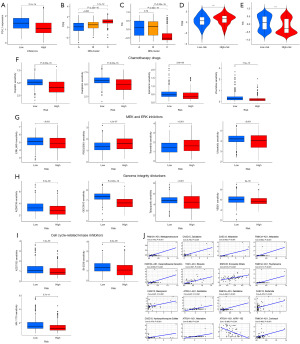
Furthermore, we explored the relationship between risk score and sensitivity to other antitumor drugs. Using gene expression data from NB patients, we calculated drug sensitivity scores with the oncoPredict package. Compared to the low-risk group, the high-risk group demonstrated reduced sensitivity to various antitumor drugs, including common chemotherapy drugs (cisplatin, oxaliplatin, epirubicin, and vincristine) (Figure 8F), MEK and ERK inhibitors (ERK_6604, PD0325901, trametinib, and ulixertinib) (Figure 8G), drugs disturbing genome integrity (AZD6738, GDC0810, talazoparib, and VE821) (Figure 8H), and inhibitors of cell cycle-related kinases (AZD7762, BI-2536, and MK-1775) (Figure 8I). These findings suggest that our DRLs prognostic model is a potential tool for predicting immunotherapy and chemotherapy effectiveness in NB patients. Additionally, we analyzed the potential correlation between the expression of the 8 DRLs in different cancer cell lines and drug sensitivity using the CellMiner database. Figure 8J highlights the top 16 drugs with the highest correlation. Notably, FAM13A-AS1 correlated positively with six drugs [methylprednisolone, nelarabine, dexamethasone (Decadron), fluphenazine, zalcitabine, cortivazol], CASC15 correlated positively with five drugs (zalcitabine, nelarabine, masoprocol, ifosfamide, hydroxychloroquine sulfate), TOB1-AS1 correlated positively with one drug (ribavirin), SNHG16 correlated positively with one drug (econazole nitrate), and ATP2A1-AS1 correlated positively with two drugs (zalcitabine, nelarabine), while ATP2A1-AS1 correlated negatively with one drug (ARRY-162).
Discussion
Disulfidptosis is a newly identified cell death modality characterized by the induction of disulfide stress in tumor cells under conditions of glucose deficiency due to NADPH depletion (10). The identification and characterization of novel biomarkers are crucial for understanding the biological behavior, disease progression, and guiding prognosis-based treatment decisions in NB. Recent studies have reported the value of DRGs in liver cancer, bladder cancer, and lung cancer (11,44,45). However, their application in NB remains limited. LncRNAs play a significant role in regulating the malignant behavior of tumor cells and have been demonstrated as potential biomarkers and targets for cancer diagnosis and treatment (46). Currently, treatment modalities for NB mainly include surgical resection, chemotherapy, radiotherapy, and immunotherapy. However, for high-risk or advanced-stage patients, the treatment outcomes are still suboptimal, necessitating more precise treatment strategies and prognostic models. To date, the application of DRGs in NB has been reported only in Zhu’s study (47), and the prognostic value of DRLs in NB remains unclear. In this study, we identified DRLs and established an NB prognostic model based on eight DRLs.
Utilizing gene expression data, we identified 175 DRLs and filtered out lncRNAs associated with OS for unsupervised clustering and model construction. DRLs offer a novel avenue for subtyping NB, revealing distinct differences between subtypes. Using LASSO regression, we built a prognostically relevant signature comprising eight DRLs. Validation in the training set, test set, and complete set demonstrated a molecular feature with predictive significance. Subsequent analyses revealed a negative correlation trend between risk score and OS, identifying risk score as independent prognostic factors for predicting patient 1-, 3-, and 5-year survival rates. Compared to INSS stage and MYCN status, risk score exhibited superior performance in predicting the clinical trajectory of NB patients. The intricate interplay between these lncRNAs and DRGs delineates a complex regulatory network at play in NB, providing valuable insights for prognostic assessment.
The immune microenvironment stands out as a critical component within the tumor microenvironment, playing a pivotal role in orchestrating disease progression and responses to anti-cancer treatments (48,49). Research indicates that these lncRNAs can modulate the infiltration and functionality of immune cells, influencing their proportions within the tumor microenvironment. For example, some DRLs may enhance tumor progression by inhibiting the activity of immune cells, which results in immune evasion by tumor cells. In our investigation, distinct immune scores and levels of immune cell infiltration were evident across molecular subtypes. The prognosis-poor DRLclusterC exhibited lower ImmuneScores and reduced immune cell infiltration. Similarly, NB patients in high-risk groups displayed varying immune scores and levels of immune cell infiltration. Notably, the high-risk group manifested lower immune cell infiltration, encompassing B cells, T cells CD4+, T cells CD8+, NK cells, neutrophils, macrophages, and myeloid dendritic cells. Given the documented anti-tumor effects of T cells and B cells (50,51), this suggests compromised inhibitory effects on tumor cell growth in the high-risk group. These findings point to the existence of an immune-suppressive environment characterized by elevated DRLs in NB, potentially contributing to the diminished immunotherapeutic efficacy observed in the high-risk group. This enhances our comprehension of the immune system’s role in combating NB. Somatic mutations play a pivotal role in tumor initiation and progression. Our observations revealed a higher mutation frequency in the cancer-related gene MUC16 (52) and a lower mutation frequency in the tumor suppressor gene ALK (53) within the high-risk group. This dissonance may represent another significant factor contributing to reduced immune cell infiltration and activity, thus foreshadowing an unfavorable prognosis in the high-risk group. The integration of TMB and risk scores not only hones predictions but also furnishes a nuanced understanding of the genetic mutation landscape, providing supplementary tools for personalized treatment decisions.
NB presents formidable challenges in the domain of immunotherapy (54). Despite recent strides in immunotherapeutic strategies, such as immune checkpoint inhibitors and chimeric antigen receptor (CAR) T-cell therapy, their efficacy in NB remains constrained (55,56). Consequently, we aimed to ascertain whether our prognostic model based on DRLs could predict the immunotherapeutic response in NB patients. The results revealed that the low-risk group exhibited lower TIDE scores and higher TIS scores, indicative of a more favorable response to immune therapy. The observed enrichment of anti-tumor immune cells and increased PD-L1 expression in the low-risk group implies a potential synergistic interaction between our prognostic model and immune therapeutic strategies. Expanding our focus to drug sensitivity, the low-risk group demonstrated enhanced responses to various anti-tumor drugs. This underscores the transformative potential of our prognostic model, offering guidance in the intricate landscape of NB treatment decisions.
In conclusion, our exploration of the DRLs signature not only deepens our understanding of NB biology but also provides a multifaceted approach to subtype classification, prognosis, and personalized therapeutic interventions. A significant advantage of our study is the innovative use of DRLs to construct a prognostic model, which contributes to the growing body of knowledge in this area. Additionally, our DRLs prognostic model accurately stratifies NB patients into distinct risk groups, potentially leading to tailored treatment strategies and improved outcomes. However, our study has certain limitations as it primarily relies on computational methods using publicly available datasets, introducing inherent biases and limitations in data interpretation. Additionally, further research is needed to elucidate the precise roles and molecular mechanisms of the eight lncRNAs used to construct the model in regulating disulfidptosis-related cell death. Continued research in this field holds the potential to advance our understanding and treatment of NB, ultimately benefiting patient prognosis.
Conclusions
In summary, we established a prognostic model based on eight DRLs to predict the prognosis of NB patients, assess the immune cell infiltration landscape, analyze TMB, evaluate the effectiveness of immunotherapy, and gauge sensitivity to anti-tumor drug treatments. Integrating these lncRNAs into clinical practice allows for a refined risk stratification, guiding personalized treatment decisions for NB patients.
Acknowledgments
The authors sincerely thank all the participants in this study.
Funding: This project was funded by
Footnote
Reporting Checklist: The authors have completed the TRIPOD reporting checklist. Available at https://tcr.amegroups.com/article/view/10.21037/tcr-24-510/rc
Peer Review File: Available at https://tcr.amegroups.com/article/view/10.21037/tcr-24-510/prf
Conflicts of Interest: All authors have completed the ICMJE uniform disclosure form (available at https://tcr.amegroups.com/article/view/10.21037/tcr-24-510/coif). The authors have no conflicts of interest to declare.
Ethical Statement: The authors are accountable for all aspects of the work in ensuring that questions related to the accuracy or integrity of any part of the work are appropriately investigated and resolved. The study was conducted in accordance with the Declaration of Helsinki (as revised in 2013).
Open Access Statement: This is an Open Access article distributed in accordance with the Creative Commons Attribution-NonCommercial-NoDerivs 4.0 International License (CC BY-NC-ND 4.0), which permits the non-commercial replication and distribution of the article with the strict proviso that no changes or edits are made and the original work is properly cited (including links to both the formal publication through the relevant DOI and the license). See: https://creativecommons.org/licenses/by-nc-nd/4.0/.
References
- Maris JM. Recent advances in neuroblastoma. N Engl J Med 2010;362:2202-11. [Crossref] [PubMed]
- Lundberg KI, Treis D, Johnsen JI. Neuroblastoma Heterogeneity, Plasticity, and Emerging Therapies. Curr Oncol Rep 2022;24:1053-62. [Crossref] [PubMed]
- Matthay KK, Maris JM, Schleiermacher G, et al. Neuroblastoma. Nat Rev Dis Primers 2016;2:16078. [Crossref] [PubMed]
- Monclair T, Brodeur GM, Ambros PF, et al. The International Neuroblastoma Risk Group (INRG) staging system: an INRG Task Force report. J Clin Oncol 2009;27:298-303. [Crossref] [PubMed]
- Park JR, Bagatell R, London WB, et al. Children's Oncology Group's 2013 blueprint for research: neuroblastoma. Pediatr Blood Cancer 2013;60:985-93. Erratum in: Pediatr Blood Cancer 2014;61:958. [Crossref] [PubMed]
- DuBois SG, Kalika Y, Lukens JN, et al. Metastatic sites in stage IV and IVS neuroblastoma correlate with age, tumor biology, and survival. J Pediatr Hematol Oncol 1999;21:181-9. [Crossref] [PubMed]
- Galluzzi L, Vitale I, Aaronson SA, et al. Molecular mechanisms of cell death: recommendations of the Nomenclature Committee on Cell Death 2018. Cell Death Differ 2018;25:486-541. [Crossref] [PubMed]
- Jiang L, Kon N, Li T, et al. Ferroptosis as a p53-mediated activity during tumour suppression. Nature 2015;520:57-62. [Crossref] [PubMed]
- Ge EJ, Bush AI, Casini A, et al. Connecting copper and cancer: from transition metal signalling to metalloplasia. Nat Rev Cancer 2022;22:102-13. [Crossref] [PubMed]
- Liu X, Nie L, Zhang Y, et al. Actin cytoskeleton vulnerability to disulfide stress mediates disulfidptosis. Nat Cell Biol 2023;25:404-14. [Crossref] [PubMed]
- Wang T, Guo K, Zhang D, et al. Disulfidptosis classification of hepatocellular carcinoma reveals correlation with clinical prognosis and immune profile. Int Immunopharmacol 2023;120:110368. [Crossref] [PubMed]
- Xu K, Dai C, Yang J, et al. Disulfidptosis-related lncRNA signatures assess immune microenvironment and drug sensitivity in hepatocellular carcinoma. Comput Biol Med 2024;169:107930. [Crossref] [PubMed]
- Chen G, Zhang G, Zhu Y, et al. Identifying disulfidptosis subtypes in hepatocellular carcinoma through machine learning and preliminary exploration of its connection with immunotherapy. Cancer Cell Int 2024;24:194. [Crossref] [PubMed]
- Uszczynska-Ratajczak B, Lagarde J, Frankish A, et al. Towards a complete map of the human long non-coding RNA transcriptome. Nat Rev Genet 2018;19:535-48. [Crossref] [PubMed]
- Rinn JL, Chang HY. Genome regulation by long noncoding RNAs. Annu Rev Biochem 2012;81:145-66. [Crossref] [PubMed]
- Ye M, Gao R, Chen S, et al. Downregulation of MEG3 and upregulation of EZH2 cooperatively promote neuroblastoma progression. J Cell Mol Med 2022;26:2377-91. [Crossref] [PubMed]
- Pan Y, Jin X, Xu H, et al. Developing a prognostic model using machine learning for disulfidptosis related lncRNA in lung adenocarcinoma. Sci Rep 2024;14:13113. [Crossref] [PubMed]
- Yang F, Niu X, Zhou M, et al. Development and validation of a novel disulfidptosis-related lncRNAs signature in patients with HPV-negative oral squamous cell carcinoma. Sci Rep 2024;14:14436. [Crossref] [PubMed]
- Wei J, Wang M, Wu Y. A disulfidptosis-related lncRNAs cluster to forecast the prognosis and immune landscapes of ovarian cancer. Front Genet 2024;15:1397011. [Crossref] [PubMed]
- Leek JT, Johnson WE, Parker HS, et al. The sva package for removing batch effects and other unwanted variation in high-throughput experiments. Bioinformatics 2012;28:882-3. [Crossref] [PubMed]
- Gustavsson EK, Zhang D, Reynolds RH, et al. ggtranscript: an R package for the visualization and interpretation of transcript isoforms using ggplot2. Bioinformatics 2022;38:3844-6. [Crossref] [PubMed]
- Wilkerson MD, Hayes DN. ConsensusClusterPlus: a class discovery tool with confidence assessments and item tracking. Bioinformatics 2010;26:1572-3. [Crossref] [PubMed]
- Holleczek B, Brenner H. Model based period analysis of absolute and relative survival with R: data preparation, model fitting and derivation of survival estimates. Comput Methods Programs Biomed 2013;110:192-202. [Crossref] [PubMed]
- Yoshihara K, Shahmoradgoli M, Martínez E, et al. Inferring tumour purity and stromal and immune cell admixture from expression data. Nat Commun 2013;4:2612. [Crossref] [PubMed]
- Li T, Fu J, Zeng Z, et al. TIMER2.0 for analysis of tumor-infiltrating immune cells. Nucleic Acids Res 2020;48:W509-14. [Crossref] [PubMed]
- Newman AM, Steen CB, Liu CL, et al. Determining cell type abundance and expression from bulk tissues with digital cytometry. Nat Biotechnol 2019;37:773-82. [Crossref] [PubMed]
- Finotello F, Mayer C, Plattner C, et al. Molecular and pharmacological modulators of the tumor immune contexture revealed by deconvolution of RNA-seq data. Genome Med 2019;11:34. [Crossref] [PubMed]
- Aran D, Hu Z, Butte AJ. xCell: digitally portraying the tissue cellular heterogeneity landscape. Genome Biol 2017;18:220. [Crossref] [PubMed]
- Racle J, Gfeller D. EPIC: A Tool to Estimate the Proportions of Different Cell Types from Bulk Gene Expression Data. Methods Mol Biol 2020;2120:233-48. [Crossref] [PubMed]
- Becht E, Giraldo NA, Lacroix L, et al. Estimating the population abundance of tissue-infiltrating immune and stromal cell populations using gene expression. Genome Biol 2016;17:218. [Crossref] [PubMed]
- Bindea G, Mlecnik B, Tosolini M, et al. Spatiotemporal dynamics of intratumoral immune cells reveal the immune landscape in human cancer. Immunity 2013;39:782-95. [Crossref] [PubMed]
- Liu Z, Li M, Jiang Z, et al. A Comprehensive Immunologic Portrait of Triple-Negative Breast Cancer. Transl Oncol 2018;11:311-29. [Crossref] [PubMed]
- He Y, Jiang Z, Chen C, et al. Classification of triple-negative breast cancers based on Immunogenomic profiling. J Exp Clin Cancer Res 2018;37:327. [Crossref] [PubMed]
- Tibshirani R. The lasso method for variable selection in the Cox model. Stat Med 1997;16:385-95. [Crossref] [PubMed]
- Chen S, Liu Y, Yang J, et al. Development and Validation of a Nomogram for Predicting Survival in Male Patients With Breast Cancer. Front Oncol 2019;9:361. [Crossref] [PubMed]
- Ritchie ME, Phipson B, Wu D, et al. limma powers differential expression analyses for RNA-sequencing and microarray studies. Nucleic Acids Res 2015;43:e47. [Crossref] [PubMed]
- Subramanian A, Tamayo P, Mootha VK, et al. Gene set enrichment analysis: a knowledge-based approach for interpreting genome-wide expression profiles. Proc Natl Acad Sci U S A 2005;102:15545-50. [Crossref] [PubMed]
- Yu G, Wang LG, Han Y, et al. clusterProfiler: an R package for comparing biological themes among gene clusters. OMICS 2012;16:284-7. [Crossref] [PubMed]
- Mayakonda A, Lin DC, Assenov Y, et al. Maftools: efficient and comprehensive analysis of somatic variants in cancer. Genome Res 2018;28:1747-56. [Crossref] [PubMed]
- Jiang P, Gu S, Pan D, et al. Signatures of T cell dysfunction and exclusion predict cancer immunotherapy response. Nat Med 2018;24:1550-8. [Crossref] [PubMed]
- Danaher P, Warren S, Lu R, et al. Pan-cancer adaptive immune resistance as defined by the Tumor Inflammation Signature (TIS): results from The Cancer Genome Atlas (TCGA). J Immunother Cancer 2018;6:63. [Crossref] [PubMed]
- Maeser D, Gruener RF, Huang RS. oncoPredict: an R package for predicting in vivo or cancer patient drug response and biomarkers from cell line screening data. Brief Bioinform 2021;22:bbab260. [Crossref] [PubMed]
- Reinhold WC, Sunshine M, Liu H, et al. CellMiner: a web-based suite of genomic and pharmacologic tools to explore transcript and drug patterns in the NCI-60 cell line set. Cancer Res 2012;72:3499-511. [Crossref] [PubMed]
- Chen H, Yang W, Li Y, et al. Leveraging a disulfidptosis-based signature to improve the survival and drug sensitivity of bladder cancer patients. Front Immunol 2023;14:1198878. [Crossref] [PubMed]
- Qi C, Ma J, Sun J, et al. The role of molecular subtypes and immune infiltration characteristics based on disulfidptosis-associated genes in lung adenocarcinoma. Aging (Albany NY) 2023;15:5075-95. [Crossref] [PubMed]
- Chi Y, Wang D, Wang J, et al. Long Non-Coding RNA in the Pathogenesis of Cancers. Cells 2019;8:1015. [Crossref] [PubMed]
- Zhu A, Li X, Wang J. Integrating bulk-seq and single-cell-seq reveals disulfidptosis potential index associating with neuroblastoma prognosis and immune infiltration. J Cancer Res Clin Oncol 2023;149:16647-58. [Crossref] [PubMed]
- Fridman WH, Galon J, Pagès F, et al. Prognostic and predictive impact of intra- and peritumoral immune infiltrates. Cancer Res 2011;71:5601-5. [Crossref] [PubMed]
- Galon J, Angell HK, Bedognetti D, et al. The continuum of cancer immunosurveillance: prognostic, predictive, and mechanistic signatures. Immunity 2013;39:11-26. [Crossref] [PubMed]
- Zhong S, Malecek K, Johnson LA, et al. T-cell receptor affinity and avidity defines antitumor response and autoimmunity in T-cell immunotherapy. Proc Natl Acad Sci U S A 2013;110:6973-8. [Crossref] [PubMed]
- Zhu T, Zheng J, Hu S, et al. Construction and validation of an immunity-related prognostic signature for breast cancer. Aging (Albany NY) 2020;12:21597-612. [Crossref] [PubMed]
- Kanwal M, Ding XJ, Song X, et al. MUC16 overexpression induced by gene mutations promotes lung cancer cell growth and invasion. Oncotarget 2018;9:12226-39. [Crossref] [PubMed]
- Hallberg B, Palmer RH. The role of the ALK receptor in cancer biology. Ann Oncol 2016;27:iii4-iii15. [Crossref] [PubMed]
- Anderson J, Majzner RG, Sondel PM. Immunotherapy of Neuroblastoma: Facts and Hopes. Clin Cancer Res 2022;28:3196-206. [Crossref] [PubMed]
- Yeku OO, Longo DL. CAR T Cells for Neuroblastoma. N Engl J Med 2023;388:1328-31. [Crossref] [PubMed]
- Crunkhorn S. Understanding PI3K inhibitor mechanism of action. Nat Rev Drug Discov 2021;20:816. [PubMed]