N-acetyltransferase 10 as a novel prognostic biomarker in papillary renal cell carcinoma: a machine learning and experimental validation study
Highlight box
Key findings
• N-acetyltransferase 10 (NAT10) is aberrantly expressed in papillary renal cell carcinoma (pRCC) and is closely linked to multiple malignancy-related pathways, contributing to a disrupted tumor microenvironment in pRCC progression. A NAT10-based signature can serve as an effective predictor of prognosis among patients with pRCC.
What is known and what is new?
• NAT10 functions as an acetyltransferase for RNA modifications and has been associated with cancer progression.
• We identified NAT10’s specific prognostic value in pRCC, developed a related survival model, and confirmed its relationship with several malignancy-related pathways and immunotherapy response.
What is the implication, and what should change now?
• Our findings suggest that NAT10 could be a valuable biomarker and therapeutic target in pRCC, and further research to confirm its role in personalized cancer treatment is warranted.
Introduction
Papillary renal cell carcinoma (pRCC), the most common type of non-clear cell renal cell carcinoma (nccRCC), constitutes about 15% of all renal cell carcinomas (1-3). Although patients with pRCC generally have a more favorable prognosis compared to those with clear cell renal cell carcinoma (ccRCC), the prognosis for patients with metastatic pRCC is significantly worse (2). This could be due to its resistance to immunotherapy and chemotherapy (4,5). Furthermore, most studies and guidelines are centered on patients with ccRCC, and given the high heterogeneity of pRCC, there is limited understanding of the specific mechanisms underlying pRCC development (2,6). Additionally, there is a lack of biomarkers to guide the clinical treatment of pRCC. Existing biomarkers and prognostic models for pRCC are very limited, and most of those available are unsatisfactory. Traditional biomarkers, such as mutations in MET, NF2, SETD2, and Nrf2 pathway genes, have limitations due to the low frequency of mutation carriers, restricting their applicability (7-9). Prognostic models for pRCC are also hindered by limited samples and public datasets, resulting in a lack of external validation with independent datasets and insufficient experimental validation (10,11). Furthermore, these models are often constructed from similar perspectives, with limited exploration of potential biomarkers or model frameworks that might play crucial roles in pRCC (11-13). Therefore, it is essential to develop a new, reliable prognostic model for pRCC. Considering these factors, it is crucial to identify new biomarkers that can predict the prognosis of pRCC and the response to immunotherapy, targeted therapy, chemotherapy, and other treatments to advance the precision treatment of pRCC.
N-acetyltransferase 10 (NAT10), a lysine acetyltransferase of the Gcn5-related N-acetyltransferase family, is known for its role in catalyzing N4-acetylcytidine (ac4C) modifications on ribosomal RNAs, transfer RNAs, and messenger RNAs (mRNAs). These modifications are critical as they enhance the stability and translation efficiency of these RNAs, which are fundamental for cellular function and protein synthesis (14). Recent studies have increasingly linked NAT10 and ac4C modifications to the pathogenesis of several diseases, including inflammatory conditions, metabolic disorders, autoimmune diseases, and a variety of cancers (14-16). In the context of cancers, NAT10 has been implicated in the progression of several malignancies, such as liver cancer (17), gastric cancer (18), colorectal cancer (19), cervical cancer (20), bladder urothelial carcinoma (21), and ccRCC (22). As a primary “writer” of ac4C on RNAs, NAT10 exhibits diverse mechanisms of action in tumors. It can promote tumor progression by enhancing cell proliferation, regulating apoptosis, facilitating epithelial-mesenchymal transition, altering tumor cell metabolism, and promoting immunosuppression (14). However, the specific role and prognostic significance of NAT10 in pRCC remain poorly understood.
In this study, we conducted an in-depth bioinformatics analysis on the function of NAT10 in pRCC. Our findings revealed a strong association between NAT10 expression and patients’ prognosis and with several malignancy-related pathways. To maximize its clinical value, we also developed a NAT10-related prognostic model using machine learning, which showed robust predictive value for patients’ prognosis and response to immunotherapy. Additionally, experimental validation was performed to enhance the reliability of our findings. We present this article in accordance with the TRIPOD and MDAR reporting checklists (available at https://tcr.amegroups.com/article/view/10.21037/tcr-2024-2195/rc).
Methods
Data collection
Transcriptional expression data from tumor and adjacent normal tissues were obtained from The Cancer Genome Atlas (TCGA), including 285 samples of kidney renal papillary cell carcinoma (KIRP) and 32 normal samples, along with corresponding clinical information, via the Genomic Data Commons (GDC) Data Portal (https://portal.gdc.cancer.gov/) (23,24). An independent pRCC cohort, GSE2748, comprising 28 KIRP samples (25), was obtained from the Gene Expression Omnibus (GEO; https://www.ncbi.nlm.nih.gov/geo/) (26) and was used as an external validation set. The TCGA training set (n=285) included 42 events (deaths) and 243 censored cases. The GSE2748 validation set included 13 events and 15 censored cases. In these two datasets, sample eligibility was primarily determined according to the availability of tumor specimens from patients diagnosed with pRCC. These specimens were typically collected from patients who had tumors surgically removed as part of their clinical care. The primary difference between the TCGA and GSE2748 datasets is the data type: TCGA data consists of RNA-sequencing (RNA-seq) data, whereas GSE2748 is based on microarray data. The baseline characteristics of these two datasets are shown in Table S1. Additionally, a dataset containing single-cell RNA-seq data for multiple renal cancer pathologic types was downloaded from a previous study, in which pRCC samples were used to study NAT10 expression at the single-cell level (27). The RNA-seq data of normal kidney tissue was downloaded from GTEx database (28) Data from an anti–PD-L1 clinical study cohort, IMvigor210 (29), were downloaded using the “IMvigor210CoreBiologies” R package (30) (The R Foundation for Statistical Computing) and used to clarify the relationship between NAT10-related risk scores and response to immunotherapy. Moreover, the protein expression levels of NAT10 and immunohistochemistry data were obtained from the Human Protein Atlas (HPA) database (https://www.proteinatlas.org/) (31). The study was conducted in accordance with the Declaration of Helsinki (as revised in 2013).
Single-cell RNA-seq data processing
The single-cell (sc) RNA-seq data were processed with the scanpy module (32) in Python (Python Software Foundation, Wilmington, DE, USA). The quality control criteria included genes expressed in at least three cells and cells with at least 500 expressed genes, with cells with more than 5,000 expressed genes being excluded. Additionally, cells with mitochondrial gene expression exceeding 20% were excluded. Doublets were removed using the Scrublet algorithm (33), and batch effects were corrected using the BBKNN algorithm (34). The infercnvpy module was used to identify potential malignant cells (https://github.com/icbi-lab/infercnvpy). Marker genes for cell annotation were downloaded from the CellMarker 2.0 database (http://bio-bigdata.hrbmu.edu.cn/CellMarker/index.html) (35).
Pathway enrichment analysis and immune cell infiltration analysis
Gene set variance analysis (GSVA) was performed using the R package “GSVA”, with gene sets downloaded from the Molecular Signatures Database (MSigDB; https://www.gsea-msigdb.org/gsea/index.jsp) (36,37). Pathways with a P value <0.05 in the Wilcoxon test, with the medians being used as the grouping factor, were considered significantly different. Overrepresentation analysis was conducted using the “enrichKEGG” and “enrichGO” functions in the R package “clusterProfiler” (38). Two immune cell infiltration analysis algorithms, ESTIMATE and CIBERSORT, were implemented using the R package “IOBR” (39-41).
A NAT10-related prognostic model based on machine learning
A list of genes positively correlated with NAT10 in pRCC was downloaded from the UALCAN database (42) and intersected with another gene set identified through ac4C RNA immunoprecipitation sequencing (ac4C-RIP-seq) and subjected to NAT10-mediated ac4C modification, which was downloaded from a supplementary file in a previous study (43). This intersection produced the candidate NAT10-related genes. Clinical data, including 285 pRCC samples from the TCGA database and 28 samples from the GSE2748 external validation, were used to develop and validate the prognostic model.
To further refine the gene set, univariate Cox regression analysis was performed, identifying genes significantly associated with overall survival in pRCC patients. Genes meeting the significance threshold (P<0.001) were subsequently included in least absolute shrinkage and selection operator (LASSO) regression to select the final genes for the NAT10-related prognostic model. The lambda parameter in the LASSO regression was optimized using 10-fold cross-validation to minimize deviance, and genes with nonzero coefficients at the minimum lambda value (lambda =0.0268604) were retained. These genes were then incorporated into a multivariate Cox regression to construct the final model for predicting overall survival. These analyses were conducted using the R packages “glmnet” and “survival” (44,45).
Then, the model’s performance was evaluated through both internal validation within the TCGA training set and external validation using the GSE2748 dataset, to assess the model’s robustness and generalizability. The predictive accuracy of the model was assessed by calculating the area under the curve (AUC) values for 1-, 3-, and 5-year survival in both datasets using the “timeROC” R package (46), providing a comprehensive measure of the model’s prognostic performance.
Cell lines
The RCC cell lines 786-O and ACHN, as well as the normal proximal tubular cell line HK2, were obtained from the Institute of Biochemistry and Cell Biology of the Chinese Academy of Sciences (Shanghai, China). All cells were cultured in RPMI-1640 medium with 10% fetal bovine serum (Invitrogen, Thermo Fisher Scientific, Waltham, MA, USA) in a humidified incubator at 37 ℃ in a 5% CO2 atmosphere.
Quantitative real-time polymerase chain reaction (qRT-PCR)
Total RNA was isolated from two RCC cell lines, 786-O and ACHN, and a normal proximal tubular cell line, HK2, using a TRIzol reagent (Invitrogen). First-strand complement DNA (cDNA) was synthesized using the HiFiScript cDNA Synthesis Kit (CoWin Biosciences, Cambridge, MA, USA). qRT-PCR was conducted on a LightCycler 480 System (Roche Diagnostics, Basel, Switzerland) with SYBR Green chemistry in accordance with the manufacturer’s standard protocols. Relative mRNA expression levels were determined using the 2−ΔΔCt method, with β-actin used as the internal reference for normalization. The primer sequences used for NAT10 were as follows: forward primer, 5'-GGATTGCCTCAACATCACTCGG-3'; and reverse primer, 5'-CGTTGGAGGAAAACTTCAGAGGC-3'. For β-actin, the primer sequences were as follows: forward primer, 5'-CACCATTGGCAATGAGCGGTTC-3'; and reverse primer, 5'-AGGTCTTTGCGGATGTCCACGT-3'. Three biological replicates were performed to ensure the reliability of the experimental results.
Western blot assay
Cells were lysed using RIPA buffer (Beyotime, Shanghai, China), and the resulting proteins were combined with loading buffer (FDbio, Hangzhou, China). The protein mixtures were resolved on 8–12% via sodium dodecyl-sulfate polyacrylamide gel electrophoresis and then transferred onto polyvinylidene fluoride (PVDF) membranes (Bio-Rad, Hercules, CA, USA). The membranes were blocked with 5% nonfat milk and incubated overnight at 4 ℃ with primary antibodies. This was followed by incubation with horseradish peroxidase (HRP)-conjugated secondary antibodies. The immunoreactive bands were detected using an enhanced chemiluminescence (ECL) system (Bio-Rad). The primary antibodies used were anti-NAT10 (1:1,000; cat. no. 13365-1-AP; Proteintech, Rosemon, IL, USA) and anti-GAPDH (1:5,000; cat. no. 60004-1-Ig; Proteintech). Three biological replicates were performed to ensure the reliability of the experimental results.
Statistical analysis
All statistical analyses were conducted with R software. For continuous variables, t-tests or Wilcoxon tests were used based on the type of data distribution to compare differences between groups. For categorical variables, Chi-squared tests were employed to assess associations. Prognostic analysis was conducted with the Kaplan-Meier method for survival curves, and Cox regression was used for assessing the impact of variables on survival via the R packages “survminer” and “survival”. A P value less than 0.05 was considered significant.
Results
NAT10 was aberrantly expressed in most cancers and associated with poor prognosis in pRCC
To clarify the biological function of NAT10, we first analyzed its expression across various cancers. As shown in Figure 1A, based on RNA expression data from TCGA, NAT10 is upregulated in multiple cancers, including bladder urothelial carcinoma (BLCA), breast cancer (BRCA), cholangiocarcinoma (CHOL), colorectal adenocarcinoma (COAD), esophageal carcinoma (ESCA), head-and-neck squamous cell carcinoma (HNSC), kidney renal clear cell carcinoma (KIRC), liver hepatocellular carcinoma (LIHC), lung adenocarcinoma (LUAD), lung squamous cell carcinoma (LUSC), rectal adenocarcinoma (READ), stomach adenocarcinoma (STAD), thymoma (THYM), and KIRP. This finding aligns with the results of the paired sample analysis (Figure 1B) and with previous reports, indicating the involvement of NAT10 in cancer malignancy (17-20). In KIRP, the primary focus of this study, NAT10 is significantly elevated in tumor tissues, both in the standalone TCGA database (with limited normal samples) and with the addition of normal tissue samples from GTEx database (Figure 1C,1D).
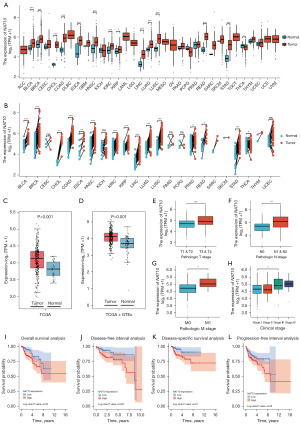
We further analyzed the association between NAT10 expression and clinical characteristics and prognosis in pRCC. As illustrated in Figure 1E-1H, patients with higher TNM stages exhibited increased NAT10 expression. Additionally, elevated NAT10 expression was significantly associated with shorter overall survival, disease-free interval, and disease-specific survival (Figures 1I-1K). There was also a potential association with shorter progression-free survival (P=0.06, Figure 1L). These results suggested a link between elevated NAT10 expression and higher tumor malignancy.
Given the limitation of bulk RNA-seq techniques, which actually reflect a mix of different cell types, we also examined the expression of NAT10 in pRCC at the single-cell level using data from Young et al. (27). As shown in Figure 2A-2C, the expression of NAT10 was significantly higher in malignant pRCC cells compared with adjacent normal epithelial cells. This finding further supports the role of NAT10 in pRCC.
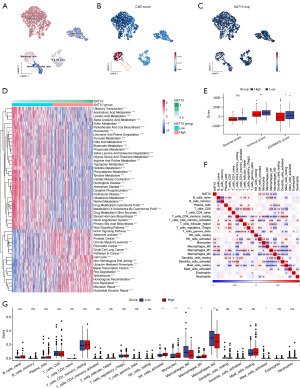
NAT10 expression was associated with several malignancy-related pathways and the tumor microenvironment (TME)
Pathway analyses were conducted to elucidate the specific mechanisms through which NAT10 promotes pRCC progression. NAT10 expression was found to be negatively associated with several metabolism-related pathways, including fatty-acid metabolism, tryptophan metabolism, the citrate cycle (tricarboxylic acid cycle), and oxidative phosphorylation. Additionally, it was negatively correlated with immune-related pathways such as antigen processing and presentation, natural killer (NK) cell-mediated cytotoxicity, and the chemokine signaling pathway (Figure 2D, Figure S1). Conversely, NAT10 expression showed positive correlations with malignancy-related pathways, including RCC, pathways in cancer, DNA replication, cell cycle, Wnt signaling pathway, ErbB signaling pathway, mTOR signaling pathway, and Notch signaling pathway (Figure 2D). These findings suggest that pRCC with high NAT10 expression may exhibit more abnormal metabolism, increased malignancy, and a more disordered immune microenvironment.
To investigate the potential association between NAT10 expression and the immune microenvironment, we performed immune cell infiltration analyses. As illustrated in Figure 2E, ESTIMATE analysis indicated a negative correlation between NAT10 expression and both immune score and ESTIMATE score, suggesting a “colder” TME and higher tumor purity. Regarding specific cell types, CIBERSORT analysis revealed that patients with high NAT10 expression had increased percentages of activated NK cells and M1 macrophages, while the abundance of M0 macrophages and M2 macrophages was decreased (Figure 2F,2G).
Machine learning-based construction of a NAT10-related prognostic model
To further clarify the clinical value of NAT10, we sought to construct a NAT10-related prognostic model in pRCC using machine learning. First, we identified genes significantly positively correlated with NAT10 expression in TCGA-KIRP, as NAT10-mediated ac4C modification is believed to enhance RNA stability (Figure 3A). To ensure this correlation is due to NAT10-mediated ac4C modification, we intersected these identified genes with those obtained from a previous study that used ac4C-RIP-seq to screen for NAT10-modified genes in both wild-type and NAT10-knockout HeLa cells (Figure 3A) (43). This process yielded 897 candidate genes for further analysis.
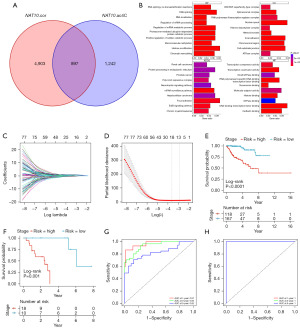
We then performed enrichment analysis to elucidate the functions of these genes (Figure 3B). Gene Ontology (GO) biological process (BP) analysis indicated that NAT10-related genes are primarily involved in RNA metabolism and protein turnover, including processes such as RNA splicing, RNA localization, and regulation of mRNA processing. GO cellular component (CC) analysis revealed that these genes are associated with the structural organization and functional regulation of the cell nucleus and chromatin, including components such as the SWI/SNF superfamily-type complex and spliceosomal complex. GO molecular function (MF) analysis showed that NAT10-related genes are involved in transcriptional regulation and signal transduction, with significant enrichment in functions such as transcription coactivator activity and RNA polymerase II-specific DNA-binding transcription factor binding. Kyoto Encyclopedia of Genes and Genomes (KEGG) pathway analysis highlighted NAT10’s role in oncogenesis and cellular signaling, indicating enrichment in cancer-related pathways such as renal cell carcinoma, hepatocellular carcinoma, and chronic myeloid leukemia. These enrichment results were consistent with previous reports suggesting that NAT10 promotes RNA stability and is associated with tumor progression, providing valuable insights into its biological and oncological roles (17,18,43).
To further refine our selection in the machine learning model, we performed univariate Cox regression analysis on these candidate genes. This initial screening yielded 87 genes significantly associated with patient outcomes (P<0.001; Table S2). As illustrated in Figure 3C,3D, LASSO regression was subsequently applied to these genes, effectively reducing the number of variables by penalizing less informative ones. Through this process, we ultimately identified 18 core genes that were robustly associated with patient prognosis. These core genes were then incorporated into the multivariate Cox regression model to calculate the NAT10-related risk scores for each patient. The final formula used to calculate the risk score is as follows: 0.479 × ZNHIT6 + 0.468 × HNRNPC –0.228 × TFE3 + 0.633 × HSP90B1 + 0.125 × AHSA1 + 0.735 × TFAP4 + 0.884 × TAP2 − 0.255 × PABPC4 + 0.726 × NUMBL + 0.109 × RRS1 – 0.827 × WWC1 + 1.138 × SERPINH1 – 0.069 × SLC12A4 + 0.029 × CERCAM + 0.925 × PLK1 + 0.388 × GCLC + 0.382 × SNX3 – 1.014 × CAPN1. Patients with positive z-score risk scores were placed into the high-risk group, while those with negative scores were placed into the low-risk group.
Assessment of the efficacy and reliability of the NAT10-related prognostic model
Finally, we assessed the accuracy and reliability of the model using the TCGA-KIRP training set and an external test set, GSE2748. As illustrated in Figure 3E,3F, patients with higher NAT10-related risk scores (NRS) exhibited significantly poorer prognosis in both the training and test sets. Receiver operating characteristic (ROC) curve analysis demonstrated that the model achieved AUC values of 0.97 (95% CI: 0.93–1.00), 0.93 (95% CI: 0.88–0.96), and 0.82 (95% CI: 0.71–0.94) for 1-, 3-, and 5-year survival predictions in the training set, respectively, and 1.00 (95% CI: 1.00) in the test set (Figure 3G,3H). These results indicate that the model could accurately predict the prognosis of patients with pRCC.
The association between NRS and clinical characteristics of patients with pRCC was also assessed. As shown in Figure 4A-4D, patients with higher T stage, clinical stage, N stage, and M stage showed significantly higher NRS, while patients’ age showed no significant association with NRS (Figure 4E). Univariate and multivariate Cox regression analyses indicated that NRS could accurately predict patient outcomes, and this remained significant even when factors such as age, gender, and disease stage were accounted for (Figure 4F,4G). This demonstrates the clinical value of NRS as an independent prognostic factor for patients with pRCC.
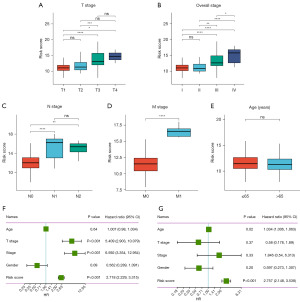
The NRS could predict the immunotherapy response
We then examined the relationship between the NRS and immunotherapy response in the IMvigor210 cohort. As illustrated in Figure 5A, patients with the higher NRS had significantly lower complete response (CR) and partial response (PR) rates, along with higher stable disease (SD) and progressive disease (PD) rates, indicating a poorer response to immunotherapy. Analysis of clinical characteristics revealed that the high-NRS group had a higher proportion of stage I and a lower proportion of stage II disease as compared to the low-NRS group, while there was no significant difference in the proportions of stage III or IV disease (Figure 5B). Additionally, patients exhibiting SD or PD response had a higher NRS, and there was no significant difference in the NRS between patients with different disease stages (Figure 5C,5D). Finally, survival analysis demonstrated that among patients receiving immunotherapy, those with high NRS had significantly worse prognosis (Figure 5E).
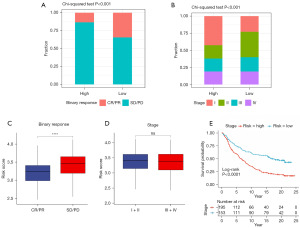
Prognostic value of NAT10 across multiple cancers and experimental validation of its aberrant expression in RCC cells
Considering the significant prognostic impact of NAT10 on patient outcomes in pRCC, we further examined its role across various cancers. As shown in Figure 6A, high NAT10 expression was found to be a significant risk factor for overall survival in glioblastoma multiforme, HNSC, KIRC, liver hepatocellular carcinoma, pancreatic adenocarcinoma, and pheochromocytoma and paraganglioma (PCPG) and a protective factor only in READ. Similar results were observed for disease-specific survival (DSS), progression-free interval (PFI), and disease-free interval (DFI). These findings suggest that the oncogenic role of NAT10 may be widespread across various types of tumors.
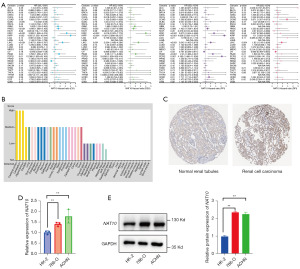
Given that genes not expressed at extremely low levels in normal tissues are more likely to play important biological roles in cancers and are less prone to false positives in analyses, we further characterized the expression levels of NAT10 in various normal tissues. We found that NAT10 was expressed at relatively high levels in multiple tissues, including kidney tissue, indicating its prominent role in essential cellular functions and physiological processes (Figure 6B). Additionally, we examined the protein expression of NAT10 using immunohistochemistry results from the HPA database, which showed that NAT10 protein levels are significantly higher in RCC compared to normal tissues (Figure 6C). Finally, considering the limitations of bioinformatics analysis, we conducted further experimental validation in RCC cell lines. The results demonstrated that NAT10 was significantly overexpressed at both the mRNA and protein levels in RCC cells, further supporting the reliability of our analysis results (Figure 6D,6E).
Discussion
NAT10, a lysine acetyltransferase responsible for catalyzing ac4C modifications on RNAs, has been associated with tumor progression in several cancers (5,9-11). However, its detailed mechanisms of action and clinical value in pRCC remain unclear. In this study, we conducted an in-depth bioinformatics analysis to investigate the expression, prognostic value, and potential mechanisms of action of NAT10 in pRCC. Furthermore, using machine learning, we developed a robust NAT10-related prognostic model that not only predicts the prognosis of patients with pRCC but also has the potential to predict their response to immunotherapy. This model may offer clinical utility by serving as a tool for patient stratification and personalized treatment planning, potentially aiding in the improvement of patient outcomes and the optimization of therapeutic strategies.
Pancancer expression analysis based on TCGA database revealed aberrant expression of NAT10 in various cancers, which is consistent with previous reports (17-20), including pRCC, the main focus of our study. Subsequent survival analysis demonstrated significantly poorer survival in patients with higher NAT10 expression, further confirming the association between NAT10 expression and tumor malignancy in pRCC. Given the limitations of bulk RNA-seq, we also examined the expression of NAT10 at single-cell resolution, which showed significantly higher expression in malignant cells compared to normal epithelial cells. Based on comprehensive expression and survival analysis, we propose a potential significant protumor role for NAT10 in pRCC.
GVSA indicated that several malignancy-related pathways were significantly enriched in patients with higher NAT10 expression, including RCC, pathways in cancer, DNA replication, cell cycle, Wnt signaling pathway, ErbB signaling pathway, mTOR signaling pathway, and Notch signaling pathway. Similar findings have been reported in previous studies. For example, Ding et al. found that NAT10 could promote diffuse large B-cell lymphoma growth by regulating AMPK/mTOR signaling (47). Zheng et al. reported that NAT10 regulates mitosis by acetylating Eg5 (48). Jin et al. revealed that NAT10 promotes colorectal cancer progression by regulating the Wnt/β-catenin signaling pathway (49). Liu et al. reported that NAT10 acetylates MORC2 to regulate cell-cycle checkpoint control (50). Furthermore, GSVA also revealed that NAT10 was associated with several metabolism-related pathways, including fatty-acid metabolism, tryptophan metabolism, the citrate cycle (tricarboxylic acid cycle), and oxidative phosphorylation. This is consistent with previous reports that NAT10 is involved in the regulation of fatty-acid metabolism, glucose metabolism, and the HIF-1 pathway in cancers (15,51) and aligns with the significant metabolic reprogramming characteristic of RCC (52). Collectively, these findings suggest that NAT10 may promote the progression of pRCC through various pathways, including tumor cell proliferation and metabolism.
NAT10 was also found to be correlated with the tumor-immune microenvironment. GSVA indicated that high NAT10 expression was negatively correlated with numerous immune-related pathways, including antigen processing and presentation, NK cell-mediated cytotoxicity, and the chemokine signaling pathway. In the immune cell infiltration analysis, ESTIMATE analysis indicated a “colder” TME and higher tumor purity in patients with higher NAT10 expression. In contrast, CIBERSORT analysis showed a higher proportion of NK cells and M1 macrophages and a lower proportion of M0 macrophages and M2 macrophages in these patients. This result appears somewhat contradictory, as M1 macrophages and NK cells are typically considered to be antitumor factors (53,54), while M2 macrophages are protumor ones (53). In other words, the CIBERSORT results suggest immune response activation, which seems inconsistent with the ESTIMATE findings. Considering that CIBERSORT outputs the proportion of different immune cells relative to the total immune cells rather than their absolute numbers (41) and that pathway enrichment analysis also showed that several immune-related pathways were negatively correlated with NAT10 expression, including cytotoxicity mediated by NK cells (which was predicted to be downregulated by CIBERSORT), we speculate that overall immune cell infiltration, including the absolute levels or activities of NK cells and M1 macrophages, may still be decreased in patients with high NAT10 expression. However, given the inherent complexity of the immune microenvironment and the limitations of bioinformatics analyses, further experimental validation in the future is needed to confirm these findings.
To fully clarify the clinical significance of NAT10, we constructed a NAT10-related prognostic model using machine learning to provide references for clinical practice. Considering that solely using mRNA expression levels to screen for NAT10-related genes could have included genes not directly affected by NAT10, potentially leading to a model that does not accurately reflect NAT10’s impact, we intersected these genes with a list confirmed by a previous study (43). We employed ac4C-RIP-seq in cells with and without NAT10 knockout to ensure that these genes are indeed subject to NAT10-mediated ac4C modification. Through this, we aimed to include genes in the final model that are genuinely regulated by NAT10, thereby reducing the likelihood of false positives. The results of the enrichment analysis supported this approach. GO analysis indicated that these genes are primarily enriched in BPs related to RNA metabolism and biomolecular modification. KEGG pathway analysis revealed enrichment in several malignancy-related pathways, including the RCC pathway. These findings are consistent with previous studies reporting that NAT10 can alter RNA stability through ac4C modification and is involved in the development and progression of various cancers (14,17-19). Additionally, they align with our earlier analysis linking NAT10 to the progression of pRCC.
The model established using LASSO Cox regression demonstrated high predictive accuracy for the prognosis of patients with pRCC in both the training set and external validation set. Furthermore, its significance remained evident in multivariate regression analysis that included factors such as TNM staging, indicating that it is an independent prognostic factor for patients with pRCC. This reflects the potential important biological role of NAT10-related genes in the progression of pRCC. The immunotherapy response analysis based on the IMvigor210 cohort indicated that patients with a higher NRS tend to be less sensitive to anti-PD-L1 treatment. This result aligns with a previous study that found NAT10 knockdown can enhance the efficacy of PD-L1 blockade treatment in vivo (20).
Finally, we validated our findings through multiple approaches, including the determination of the prognostic value of NAT10 at the pancancer level, examining its expression through immunohistochemistry results, qRT-PCR, and Western blot experiments. These results were consistent with our previous analyses, reinforcing the reliability of our conclusions from multiple dimensions. These findings indicate that the aberrant overexpression of NAT10 likely plays a significant role in the progression of pRCC.
However, it must be acknowledged that despite our study’s promising findings, there are still some limitations. First, this study primarily relied on publicly available datasets. Although we aimed to enhance the reliability of our findings through external dataset validation, the sample size of the validation set was limited due to the scarcity of available pRCC datasets. Consequently, further validation in larger, prospective clinical cohorts is necessary. Second, while this study examined the various potential mechanisms by which NAT10 exerts its effects in pRCC through multiple approaches and conducted essential experimental validation, direct evidence remains relatively lacking due to limitations in experimental conditions and the difficulties in obtaining samples. Therefore, more in-depth molecular mechanism studies are essential in the future. Finally, this study mainly focused on the role and prognostic value of NAT10 in pRCC, but its role in pancancer contexts has not been investigated in depth, which is another area that future research needs to address.
Conclusions
This research undertook a comprehensive bioinformatics analysis of the role of NAT10 in pRCC. The results demonstrated a significant link between NAT10 expression and patient prognosis, highlighting its involvement in various malignancy-associated pathways and its impact on the immune microenvironment. To further investigate its clinical relevance, we employed machine learning to create a NAT10-centric prognostic model, which was proved to be highly effective in predicting outcomes in patients and their response to immunotherapy. Experimental validation was also conducted to bolster the credibility of our findings. Overall, NAT10 may serve as a promising target for the diagnosis and treatment of pRCC.
Acknowledgments
Funding: This work was supported by
Footnote
Reporting Checklist: The authors have completed the TRIPOD and MDAR reporting checklists. Available at https://tcr.amegroups.com/article/view/10.21037/tcr-2024-2195/rc
Data Sharing Statement: Available at https://tcr.amegroups.com/article/view/10.21037/tcr-2024-2195/dss
Peer Review File: Available at https://tcr.amegroups.com/article/view/10.21037/tcr-2024-2195/prf
Conflicts of Interest: All authors have completed the ICMJE uniform disclosure form (available at https://tcr.amegroups.com/article/view/10.21037/tcr-2024-2195/coif). All authors report that this work was supported by the Natural Science Foundation of Zhejiang Province (No. LY22H160009). The authors have no other conflicts of interest to declare.
Ethical Statement: The authors are accountable for all aspects of the work in ensuring that questions related to the accuracy or integrity of any part of the work are appropriately investigated and resolved. The study was conducted in accordance with the Declaration of Helsinki (as revised in 2013).
Open Access Statement: This is an Open Access article distributed in accordance with the Creative Commons Attribution-NonCommercial-NoDerivs 4.0 International License (CC BY-NC-ND 4.0), which permits the non-commercial replication and distribution of the article with the strict proviso that no changes or edits are made and the original work is properly cited (including links to both the formal publication through the relevant DOI and the license). See: https://creativecommons.org/licenses/by-nc-nd/4.0/.
References
- Flippot R, Compérat E, Tannir NM, et al. Papillary Renal Cell Carcinoma: A Family Portrait. Eur Urol 2018;73:79-80. [Crossref] [PubMed]
- Steffens S, Janssen M, Roos FC, et al. Incidence and long-term prognosis of papillary compared to clear cell renal cell carcinoma--a multicentre study. Eur J Cancer 2012;48:2347-52. [Crossref] [PubMed]
- Aksionau A, Silva RA, Hartman B, et al. NHERF1/EBP50 immunoexpression in renal cell carcinomas and oncocytomas with ultrastructural analysis of clear cell renal cell carcinoma. Transl Androl Urol 2023;12:1283-95. [Crossref] [PubMed]
- Herrmann E, Brinkmann OA, Bode ME, et al. Histologic subtype of metastatic renal cell carcinoma predicts response to combined immunochemotherapy with interleukin 2, interferon alpha and 5-fluorouracil. Eur Urol 2007;51:1625-31; discussion 1631-2. [Crossref] [PubMed]
- Motzer RJ, Bacik J, Mariani T, et al. Treatment outcome and survival associated with metastatic renal cell carcinoma of non-clear-cell histology. J Clin Oncol 2002;20:2376-81. [Crossref] [PubMed]
- Zhang Y, Xu Y, Yu T, et al. Biphasic squamoid alveolar renal cell carcinoma: description of a rare case and a literature analysis. Quant Imaging Med Surg 2022;12:3987-94. [Crossref] [PubMed]
- Sourbier C, Liao PJ, Ricketts CJ, et al. Targeting loss of the Hippo signaling pathway in NF2-deficient papillary kidney cancers. Oncotarget 2018;9:10723-33. [Crossref] [PubMed]
- Kovac M, Navas C, Horswell S, et al. Recurrent chromosomal gains and heterogeneous driver mutations characterise papillary renal cancer evolution. Nat Commun 2015;6:6336. [Crossref] [PubMed]
- Schmidt LS, Nickerson ML, Angeloni D, et al. Early onset hereditary papillary renal carcinoma: germline missense mutations in the tyrosine kinase domain of the met proto-oncogene. J Urol 2004;172:1256-61. [Crossref] [PubMed]
- Zhang Y, Liu Y, Hu X, et al. Stemness-associated senescence genes as potential novel risk factors for papillary renal cell carcinoma. Transl Androl Urol 2021;10:4241-52. [Crossref] [PubMed]
- Wang L, Gu W, Ni H. Construction of a prognostic value model in papillary renal cell carcinoma by immune-related genes. Medicine (Baltimore) 2021;100:e24903. [Crossref] [PubMed]
- Zhou X, Qiu S, Jin D, et al. Development and Validation of an Individualized Immune-Related Gene Pairs Prognostic Signature in Papillary Renal Cell Carcinoma. Front Genet 2020;11:569884. [Crossref] [PubMed]
- Luo L, Zhou H, Su H. Identification of 4-genes model in papillary renal cell tumor microenvironment based on comprehensive analysis. BMC Cancer 2021;21:553. [Crossref] [PubMed]
- Xie L, Zhong X, Cao W, et al. Mechanisms of NAT10 as ac4C writer in diseases. Mol Ther Nucleic Acids 2023;32:359-68. [Crossref] [PubMed]
- Dalhat MH, Mohammed MRS, Alkhatabi HA, et al. NAT10: An RNA cytidine transferase regulates fatty acid metabolism in cancer cells. Clin Transl Med 2022;12:e1045. [Crossref] [PubMed]
- Guo G, Shi X, Wang H, et al. Epitranscriptomic N4-Acetylcytidine Profiling in CD4(+) T Cells of Systemic Lupus Erythematosus. Front Cell Dev Biol 2020;8:842. [Crossref] [PubMed]
- Tan Y, Zheng J, Liu X, et al. Loss of nucleolar localization of NAT10 promotes cell migration and invasion in hepatocellular carcinoma. Biochem Biophys Res Commun 2018;499:1032-8. [Crossref] [PubMed]
- Zhang Y, Jing Y, Wang Y, et al. NAT10 promotes gastric cancer metastasis via N4-acetylated COL5A1. Signal Transduct Target Ther 2021;6:173. [Crossref] [PubMed]
- Zhang H, Hou W, Wang HL, et al. GSK-3β-regulated N-acetyltransferase 10 is involved in colorectal cancer invasion. Clin Cancer Res 2014;20:4717-29. [Crossref] [PubMed]
- Chen X, Hao Y, Liu Y, et al. NAT10/ac4C/FOXP1 Promotes Malignant Progression and Facilitates Immunosuppression by Reprogramming Glycolytic Metabolism in Cervical Cancer. Adv Sci (Weinh) 2023;10:e2302705. [Crossref] [PubMed]
- Wang G, Zhang M, Zhang Y, et al. NAT10-mediated mRNA N4-acetylcytidine modification promotes bladder cancer progression. Clin Transl Med 2022;12:e738. [Crossref] [PubMed]
- Miao D, Shi J, Lv Q, et al. NAT10-mediated ac(4)C-modified ANKZF1 promotes tumor progression and lymphangiogenesis in clear-cell renal cell carcinoma by attenuating YWHAE-driven cytoplasmic retention of YAP1. Cancer Commun (Lond) 2024;44:361-83. [Crossref] [PubMed]
- Cancer Genome Atlas Research Network. The Cancer Genome Atlas Pan-Cancer analysis project. Nat Genet 2013;45:1113-20. [Crossref] [PubMed]
- Grossman RL, Heath AP, Ferretti V, et al. Toward a Shared Vision for Cancer Genomic Data. N Engl J Med 2016;375:1109-12. [Crossref] [PubMed]
- Yang XJ, Tan MH, Kim HL, et al. A molecular classification of papillary renal cell carcinoma. Cancer Res 2005;65:5628-37. [Crossref] [PubMed]
- Barrett T, Wilhite SE, Ledoux P, et al. NCBI GEO: archive for functional genomics data sets--update. Nucleic Acids Res 2013;41:D991-5. [Crossref] [PubMed]
- Young MD, Mitchell TJ, Vieira Braga FA, et al. Single-cell transcriptomes from human kidneys reveal the cellular identity of renal tumors. Science 2018;361:594-9. [Crossref] [PubMed]
- The Genotype-Tissue Expression (GTEx) project. Nat Genet 2013;45:580-5. [Crossref] [PubMed]
- Balar AV, Galsky MD, Rosenberg JE, et al. Atezolizumab as first-line treatment in cisplatin-ineligible patients with locally advanced and metastatic urothelial carcinoma: a single-arm, multicentre, phase 2 trial. Lancet 2017;389:67-76. Erratum in: Lancet 2017;390:848. [Crossref] [PubMed]
- Mariathasan S, Turley SJ, Nickles D, et al. TGFβ attenuates tumour response to PD-L1 blockade by contributing to exclusion of T cells. Nature 2018;554:544-8. [Crossref] [PubMed]
- Uhlén M, Fagerberg L, Hallström BM, et al. Proteomics. Tissue-based map of the human proteome. Science 2015;347:1260419. [Crossref] [PubMed]
- Wolf FA, Angerer P, Theis FJ. SCANPY: large-scale single-cell gene expression data analysis. Genome Biol 2018;19:15. [Crossref] [PubMed]
- Wolock SL, Lopez R, Klein AM. Scrublet: Computational Identification of Cell Doublets in Single-Cell Transcriptomic Data. Cell Syst 2019;8:281-291.e9. [Crossref] [PubMed]
- Polański K, Young MD, Miao Z, et al. BBKNN: fast batch alignment of single cell transcriptomes. Bioinformatics 2020;36:964-5. [Crossref] [PubMed]
- Hu C, Li T, Xu Y, et al. CellMarker 2.0: an updated database of manually curated cell markers in human/mouse and web tools based on scRNA-seq data. Nucleic Acids Res 2023;51:D870-6. [Crossref] [PubMed]
- Subramanian A, Tamayo P, Mootha VK, et al. Gene set enrichment analysis: a knowledge-based approach for interpreting genome-wide expression profiles. Proc Natl Acad Sci U S A 2005;102:15545-50. [Crossref] [PubMed]
- Hänzelmann S, Castelo R, Guinney J. GSVA: gene set variation analysis for microarray and RNA-seq data. BMC Bioinformatics 2013;14:7. [Crossref] [PubMed]
- Yu G, Wang LG, Han Y, et al. clusterProfiler: an R package for comparing biological themes among gene clusters. OMICS 2012;16:284-7. [Crossref] [PubMed]
- Zeng D, Ye Z, Shen R, et al. IOBR: Multi-Omics Immuno-Oncology Biological Research to Decode Tumor Microenvironment and Signatures. Front Immunol 2021;12:687975. [Crossref] [PubMed]
- Yoshihara K, Shahmoradgoli M, Martínez E, et al. Inferring tumour purity and stromal and immune cell admixture from expression data. Nat Commun 2013;4:2612. [Crossref] [PubMed]
- Newman AM, Liu CL, Green MR, et al. Robust enumeration of cell subsets from tissue expression profiles. Nat Methods 2015;12:453-7. [Crossref] [PubMed]
- Chandrashekar DS, Karthikeyan SK, Korla PK, et al. UALCAN: An update to the integrated cancer data analysis platform. Neoplasia 2022;25:18-27. [Crossref] [PubMed]
- Arango D, Sturgill D, Alhusaini N, et al. Acetylation of Cytidine in mRNA Promotes Translation Efficiency. Cell 2018;175:1872-1886.e24. [Crossref] [PubMed]
- Friedman J, Hastie T, Tibshirani R. Regularization Paths for Generalized Linear Models via Coordinate Descent. J Stat Softw 2010;33:1-22. [Crossref] [PubMed]
- Modeling Survival Data: Extending the Cox Model | SpringerLink [Internet]. [cited 2024 Jul 20]. Available from: https://link.springer.com/book/10.1007/978-1-4757-3294-8
- Blanche P, Dartigues JF, Jacqmin-Gadda H. Estimating and comparing time-dependent areas under receiver operating characteristic curves for censored event times with competing risks. Stat Med 2013;32:5381-97. [Crossref] [PubMed]
- Ding M, Yu Z, Lu T, et al. N-acetyltransferase 10 facilitates tumorigenesis of diffuse large B-cell lymphoma by regulating AMPK/mTOR signalling through N4-acetylcytidine modification of SLC30A9. Clin Transl Med 2024;14:e1747. [Crossref] [PubMed]
- Zheng J, Tan Y, Liu X, et al. NAT10 regulates mitotic cell fate by acetylating Eg5 to control bipolar spindle assembly and chromosome segregation. Cell Death Differ 2022;29:846-60. [Crossref] [PubMed]
- Jin C, Wang T, Zhang D, et al. Acetyltransferase NAT10 regulates the Wnt/β-catenin signaling pathway to promote colorectal cancer progression via ac(4)C acetylation of KIF23 mRNA. J Exp Clin Cancer Res 2022;41:345. [Crossref] [PubMed]
- Liu HY, Liu YY, Yang F, et al. Acetylation of MORC2 by NAT10 regulates cell-cycle checkpoint control and resistance to DNA-damaging chemotherapy and radiotherapy in breast cancer. Nucleic Acids Res 2020;48:3638-56. [Crossref] [PubMed]
- Yang Q, Lei X, He J, et al. N4-Acetylcytidine Drives Glycolysis Addiction in Gastric Cancer via NAT10/SEPT9/HIF-1α Positive Feedback Loop. Adv Sci (Weinh) 2023;10:e2300898. [Crossref] [PubMed]
- Linehan WM, Schmidt LS, Crooks DR, et al. The Metabolic Basis of Kidney Cancer. Cancer Discov 2019;9:1006-21. [Crossref] [PubMed]
- Li M, Yang Y, Xiong L, et al. Metabolism, metabolites, and macrophages in cancer. J Hematol Oncol 2023;16:80. [Crossref] [PubMed]
- Laskowski TJ, Biederstädt A, Rezvani K. Natural killer cells in antitumour adoptive cell immunotherapy. Nat Rev Cancer 2022;22:557-75. [Crossref] [PubMed]