Immunogenic cell death-related signature predicts prognosis and immunotherapy efficacy in bladder cancer
Highlight box
Key findings
• Immunogenic cell death (ICD)-related subtype is a crucial factor that impacts on clinical survival status.
• A total of seven ICD-related genes (CALR, CASP8, CD8A, IFNB1, IFNG, IFNGR1, and MYD88) were identified and elucidated an ICD-related risk signature.
• Signaling pathways from the ICD-low subgroup were closely associated with metabolism.
What is known and what is new?
• ICD has been verified as a modality of regulated cell death. Immunotherapy is one of the promising treatments for bladder cancer (BC).
• The ICD-high subgroup and -low subgroup corresponded to immune-active phenotype and immune-resting phenotype, respectively.
What is the implication, and what should change now?
• BC patients in the ICD-high risk subgroup may benefit from immunotherapy.
IntroductionOther Section
Immunogenic cell death (ICD) is considered a modality of regulated cell death (RCD), which can trigger or enhance the immune response against tumors (1). Damage-associated molecular patterns (DAMPs) make a huge contribution on the immunogenic characteristics of ICD, including Annexin A1 (ANXA1), adenosine triphosphate (ATP), calreticulin (CRT), deoxyribonucleic acid (DNA), high mobility group box 1protien (HMGB1), heat-shock protein 70&90 (HSP 70&90), type I interferon (IFN-I), ribonucleic acid (RNA), and uric acid (UA) (2-11). Increasing evidence has shown that ICD plays an important role in tumor therapy and initiates an antitumor response (12). Based on chemotherapeutics, various drugs have been found to trigger ICD (6), such as mitoxantrone, bortezomib, doxorubicin, idarubicin, cyclophosphamide, and oxaliplatin, which can be combined with immunotherapeutics (7). Promising results of a phase II study have shown that ICD-inducers optimize clinical outcomes in glioblastoma significantly (13). Previous clinical practice showed that radiotherapy and chemoradiotherapy manifested splendid power to induce the exposure of ICD-related DAMPs, including HSP70 and HMGB1 (14). Recent research has highlighted the powerful value of ICD-related classification to predict prognosis and immunotherapeutic responses (15). Therefore, discovery of an ICD-related risk signature that can be used to guide clinical practice will benefit the treatment of tumor patients.
Bladder cancer (BC) is a common malignant tumor that ranks tenth in incidence in global cancer epidemiology (16). The clinical pathology of BC can be divided into 3 types: urothelial carcinoma (90–95%), squamous cell carcinoma, and adenocarcinoma (17). Regarding tumor stage, tumor invading the muscle or beyond is called muscle-invasive bladder cancer (MIBC); tumor cells that invade only the urothelium or lamina propria is called non-muscle-invasive bladder cancer (NMIBC) (18). Although transurethral resection of bladder tumor (TURBT) is one of the first-line treatments for NMIBC patients’ first diagnosis, the 5-year recurrence rate is 50–70% and the progression rate is 10–30% (19). Due to the development of immunotherapy and clinical medicine, we are currently in a promising era of immunotherapy in the field of malignant tumors. Hence, now is the time to take full advantage of immunotherapy for BC patients. Generally, immunotherapy contains “targeted” type and “non-targeted” types. “Targeted” immunotherapy refers to a specific antigen that can be directly bonded to activate antigen T cells or antibodies, such as programmed death-1 (PD-1), cytotoxic T cell lymphocyte-associated protein 4 (CTLA-4), and so on. “Non-targeted” immunotherapy refers to the launching of generalized immune responses, including Bacille Calmette-Guérin (BCG), interferons, interleukins, and so on (20). With the development of immunotherapy and deeper knowledge of immunity, more precise clinical prognostic signatures and novel immunotherapy are expected to enhance the combination treatment in BC.
Our study aimed to construct a prognostic signature made of several ICD-related genes and offer a practical tool, which can predict the prognosis, components of microenvironment, and immunotherapy response. The novel signature may help clinical physicians to figure out the association between ICD and immunotherapy response and make a therapeutic strategy on BC treatment. We present this article in accordance with the TRIPOD reporting checklist (available at https://tcr.amegroups.com/article/view/10.21037/tcr-24-533/rc).
MethodsOther Section
Data preprocessing and identification of differentially expressed genes (DEGs)
Transcriptional expression information and matched clinical profiles (412 samples of BC patients) were downloaded from The Cancer Genome Atlas (TCGA, http://portal.gdc.cancer.gov/), which comprised the training set. Meanwhile, data of RNA sequencing (RNA-seq) transcriptome and clinical information were acquired from the Gene Expression Omnibus (GEO, accession number: GSE32894; https://www.ncbi.nlm.nih.gov/geo/query/acc.cgi?acc=GSE32894) (21), which were deemed to be the validation set. All raw expression data were normalized via Robust Multiarray Average (22). The “limma” R package was utilized to access DEGs. The criterion of a false discovery rate (FDR) <0.05 and |fold change| >2 was formulated to screen out false-positive data.
Consensus clustering
Consensus clustering was performed through the “limma”, “survival”, and “consensusclusterplus” R packages (23,24). Then, different molecular subtypes associated with ICD were identified via consensus clustering. Subsequently, ideal cluster numbers among K=1–9 were estimated, which was repeated 1,000 times to ensure stability of the results. A cluster map was exhibited by a pheatmap tool in R software (R Foundation for Statistical Computing, Vienna, Austria).
Functional enrichment and gene set enrichment analysis (GSEA)
To specify the signaling pathways and biological impacts among ICD, functional enrichment analyses, including Gene Ontology (GO) and Kyoto Encyclopedia of Genes and Genomes (KEGG), were carried out. The “clusterprofiler” R package was used to estimate GO and KEGG pathways between the ICD high- and low-risk cohorts. The positive premises of GO and KEGG pathways were q- and P value thresholds of <0.05. In order to determine whether considerable variations were present in the set of genes between the ICD high- and low-risk cohorts; GSEA was carried out and all results were visualized. The above processions were completed via the “clusterprofiler”, “limma”, “org.hs.eg.db”, and “enrichment” R packages.
Immune landscape features between ICD subgroups
For the sake of verifying immune features of BC samples, BC expression data was input into Cell-type Identification by Estimating Relative Subsets of RNA Transcripts (CIBERSORT, https://cibersort.starford.edu/) and we repeated the procession 1,000 times to ascertain the relative proportion of 22 immune cells (25). Furthermore, the results of 22 immune cells were compared between ICD high- and low-subgroup and visualized in a landscape map. The relative fraction of immune cells infiltration was investigated via The Cancer Immunome Atlas (TCIA, https://www.tcia.at/home) (26).
Prediction of immunotherapeutic response
Immunotherapeutic response in different ICD subgroups was predicted and compared via tumor immune dysfunction and exclusion (TIDE). TIDE offers a special analytic tool to predict immunotherapeutic response through immune mechanism of tumor invasion: T cell infiltration and dysfunction suppressed in low cytotoxic lymphocyte (CTL) level.
Somatic mutation and survival analysis
Somatic mutation profile of the BC samples was downloaded and preprocessed from TCGA database. Then, differences were analyzed between two ICD subgroups. The “maftools” R package was utilized to summarize and visualize mutated genes in waterfall plots (27).
Establishment and validation of the ICD-related risk signature
To avert overfitting and establish a new ICD-related risk signature, least absolute shrinkage and selection operator (LASSO) regression analyses were conducted through the “glmnet” R package (28). The algorithm of LASSO regression analyses is to select and shrink variables via eliminating variables with a regression coefficient equal to 0. Finally, an ICD-related risk signature was identified via specific coefficients. The risk score of each sample was established through multiplying the level of each gene’s corresponding coefficient. All BC samples were divided into high- and low-risk subgroups based on the median risk score.
Multiplexed immunofluorescence staining for tumor microenvironment (TME)
Detection of CD68, CD4, Foxp3, CD11c, and CD8 was conducted using 4'6-diamidino-2-phenylindol (DAPI) on the same slide in situ. Firstly, slides were retrieved via pH 8 ethylenediaminetetraacetic acid (EDTA) after deparaffinization and rehydration. Endogenous peroxidase was inactivated by dual endogenous peroxidase block. Meanwhile, nonspecific antigens were interdicted with 0.3% bovine serum albumin (BSA) in Tris-buffered saline/Tween. Primary monoclonal antibodies against CD68 (1:3,000, 30 minutes; Abcam, ab955), Foxp3 (1:200, 12 hours; Abcam, ab20034), CD11c (1:400, 1 hour; Abcam, ab52632), CD4 (1:200, 1 hour; Abcam, ab133616), and CD8 (1:500, 1 hour; Abcam, ab237709) (Abcam, Cambridge, UK) were incubated for different times at various temperatures, respectively. Slides were incubated via 3 horseradish peroxidase (HRP)-conjugated secondary antibodies for 10 minutes at room temperature in sequence. Then, slides were microwaved for 10 minutes. Diverse opal fluorophores from Akoya Multiplexed immunofluorescence kit (Akoya Biosciences, Marlborough, MA, USA) were utilized to match corresponding cell markers (CD8, 480 mm; CD4, 570 mm; Foxp3, 690 mm; CD11c, 620 mm); Slides were and incubated by Tyramide Signal Amplification/digoxigenin (TSA/DIG) solution for CD68 staining (opal 780 mm fluorophore). Finally, DAPI working solution was required for nuclei staining and all slides were scanned. Various immune cells were counted via Image J software (National Institutes of Health, Bethesda, MD, USA) and the results were presented in a violin map.
Statistical analysis
Student’s t-test was used to compare different groups for statistical significance. All functional experiments were carried out in triplicate, and the results were shown as mean value ± standard deviation (SD). GraphPad Prism 8.0 software (GraphPad Software, San Diego, CA, USA) was utilized to complete statistical analysis. The level of statistical significance is presented as *, P<0.05; **, P<0.01; ***, P<0.001.
Ethical consideration
The study was conducted in accordance with the Declaration of Helsinki (as revised in 2013). This study was approved by the research ethics committee of The Second Affiliated Hospital of Nanchang University (No. 202402KRDPJX73088), and informed consent was provided by each patient before data analyses.
ResultsOther Section
Two ICD-related subtypes were identified via consensus clustering
ICD-related genes have been discussed in many studies and summarized (29). Differential expression was analyzed in TCGA BC cohorts and 18 ICD-related genes (FOXP3, P2RX7, LY96, CD4, NLRP3, ENTPD1, IL6, NT5E, TLR4, HMGB1, HSP90AA1, IL17RA, CASP8, MYD88, IFNB1, BAX, CALR, PDIA3) were found to have significant difference on expression (Figure 1A). The Search Tool for the Identification of Interacting Genes/Proteins (STRING, https://cn.string-db.org/), a public database for protein analysis, was utilized to create a protein-protein interaction (PPI) network. The association among those ICD-related genes is presented in Figure 1B. As shown in Figure 1B, LY96, MYD88, TLR4, CASP8, HMGB1, HSP90AA1, and NLRP3 were identified as potential driver genes. Then, ICD-related consensus clustering was performed in TCGA BC cohort, which is visualized in Figure 1C. We identified two ICD-related subtypes with significant expression patterns of ICD genes. A heatmap demonstrated the best optimal K-means (K=2). A tracking plot revealed that the best consistency in clustering analysis of K value was 2, which is consistent with above results. Cluster c1 meant high ICD-related genes expression, which is an ICD-high subtype. Conversely, cluster c2 meant low ICD-related genes expression indicating an ICD-low subtype. Hence, cluster c1 as the ICD-high subtype and cluster c2 as the ICD-low subtype were defined (Figure 1D). Furthermore, overall survival (OS) analysis indicated that patients defined as ICD-high subtype have a promising prognosis. On the contrary, the ICD-low subtype exhibited an undesirable clinical outcome (Figure 1E). Therefore, ICD-related subtype is a crucial factor that has an impact on clinical survival status.
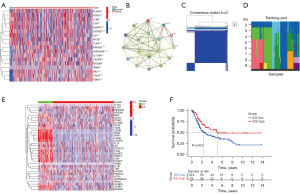
Verification of DEGs and functional enrichment in ICD subtypes
Due to opposite clinical results in different ICD-related subtype, DEGs were identified and visualized in a volcano plot (Figure 2A). A total of 100 dysregulated genes were found and enriched in different ICD-related subtypes (Figure 2B). Subsequently, to determine potential function of DEGs, GO and KEGG analyses were conducted (Figure 2C,2D). Lymphocyte-mediated immunity, external side of plasma membrane, and antigen binding were the biological process, cellular component, and molecular function with the largest number of enriched genes, respectively. Cytokine-cytokine receptor interaction is the signaling pathway with the largest number of enriched genes. In order to further understand related signaling pathways in ICD-high and -low subtype, GSEA was utilized to compare in subgroups and top five signaling pathways were shown (Figure 2E,2F). “KEGG_ALLOGRAFT_REJECTION”, “KEGG_ANTIGEN_PROCESSING_AND_PRESENTATION”, “KEGG_AUTOIMMUNE_THYROID_DISEASE”, “KEGG_CELL_ADHESION_MOLECULES_CAMS”, and “KEGG_CHEMOKINE_SIGNALING_PATHWAY” were enriched in ICD-high subgroup, which were related to immune signaling pathways. On the other hand, “KEGG_DRUG_METABOLISM_CYTOCHROME_P450”, “KEGG_METABOLISM_OF_XENOBIOTICS_BY_CYTOCHROME_P450”, “KEGG_PORPHYRIN_AND_CHLOROPHYLL_METABOLISM”, “KEGG_RETINOL_METABOLISM”, and “KEGG_STEROID_HORMONE_BIOSYNTHESIS” were enriched in ICD-low subgroup. Hence, it suggested that signaling pathways from the ICD-low subgroup were closely associated with metabolism.
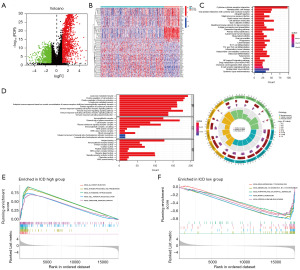
Somatic mutations analysis and TME landscape in high- and low-subtypes of ICD
To determine the difference of somatic mutation between the ICD-high and -low subtypes, we compared changes among top 20 somatic mutation profiles (Figure 3A,3B). There were eight genes (TP53, TTN, KDM6A, PIK3CA, RYR2, RB1, FAT4, FLG) for which somatic mutation in the two subgroups had significant differences (mutation rate ≥10%). The mutation rate of TTN in the two subgroups differed the most, up to 15%. Meanwhile, the mutation rate of only TP53 (58%) and TTN (55%) in the ICD-high subgroup was higher than 50%.
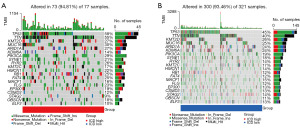
Immune reaction and immunotherapeutic response are conventionally influenced by ICD. Herein, we compared the immunescore and component of tumors in two subgroups via arithmetic scoring (Figure 4A). The tumor purity of the ICD-low subgroup was significantly higher than that in ICD-high subgroup (P<0.001). The immunescore of the ICD-high subgroup was significantly higher than that in ICD-low subgroup (P<0.001). The above results suggested that a better response might be detected in patients in the ICD-high subgroup. Relative distribution and correlation of immune infiltration on immune cells were analyzed and summarized (Figure 4B-4D). Almost consistent with the results from the public database, macrophages accounted for the largest proportion. Then, multiplexed immunofluorescence staining was performed to verify the immune infiltration in tumor tissue and results were analyzed and visualized (Figure 4E). CD4, CD8, Foxp3, CD11c, and CD68 corresponded to CD4 T cells, CD8 T cells, Treg cells, dendritic cells, and macrophages, respectively. The association among the five immune cells was visualized in a violin map. Dendritic cell was the immune cell with the fewest number of the five cells and macrophage was the immune cell with the largest number of five cells. The comparison of proportion of immune cells between ICD-high and -low subgroups is summarized in Figure 4F. For numeric detail, plasma cells (P=0.04), T cells CD4 memory resting (P=0.008), macrophage M0 (P=0.001), dendritic cells activated (P=0.02), and mast cells resting (P=0.04) in the ICD-low subgroup were higher than those in ICD-high subgroup. Meanwhile, CD8 T cells, T cells CD4 memory activated, T cells follicular helper, NK cells resting, and macrophage M1 in the ICD-low subgroup were lower than that in ICD-high subgroup. Moreover, the expression profiles of human leukocyte antigen (HLA) and immune checkpoints genes in ICD-related subgroups were accessed (Figure 4G,4H). The results indicated that all of the genes of HLA were upregulated in ICD-high subgroup. Most of the immune checkpoint genes were also upregulated, except for SIGLEC15, which was downregulated, in the ICD-high subgroup. Therefore, all evidence suggested that the ICD-high subgroup and -low subgroup corresponded to immune-active phenotype and immune-resting phenotype, respectively.
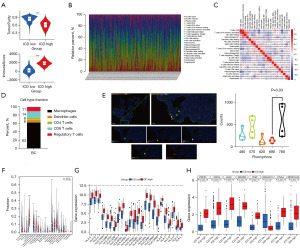
Establishment and validation of the ICD-related risk signature
Subsequently, we analyzed the prognostic power of ICD genes via univariate Cox regression in term of OS. A total of seven genes (CALR, CASP8, CD8A, IFNB1, IFNG, IFNGR1, and MYD88) were found to be significantly meaningful (Figure 5A). An ICD-related risk signature was established by LASSO regression, which was made of the seven genes (Figure 5B). The risk-score of the signature was calculated with risk coefficient of the seven genes: Risk-score = (0.4988)*CALR + (−0.0341)*CASP8 + (−0.0244)*CD8A + (−1.0072)*IFNB1 + (−0.2393)*IFNG + (−0.2089)*IFNGR1 + (−0.2300)*MYD88. Furthermore, the association between risk score and survival status was explored. The results indicated that the distribution of alive survival status in the ICD low risk subgroup was much better than that in the ICD high risk subgroup (Figure 5C). In addition, the expression profiles of seven ICD risk genes were visualized in two ICD subgroups through a heatmap (Figure 5D). CASP8, CD8A, IFNB1, IFNG, IFNGR1, and MYD88 had a high expression in ICD low risk subgroup, whereas CALR was highly expressed in the ICD high risk subgroup.
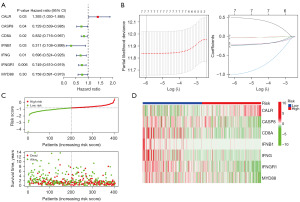
The correlation of TME and ICD-related risk signature
In light of the correlation between ICD and immune reaction and immunotherapeutic response, we investigated the prognostic value of the risk signature in TCGA BC cohort and utilized different BC cohorts to verify the results. The conclusion from TCGA cohort suggested that the OS in the ICD high-risk subgroup was significantly lower than that in the ICD low-risk subgroup (P=0.001) (Figure 6A). The conclusion from GEO cohort GSE32894 indicated that the OS in the ICD low-risk subgroup was significantly higher than that in the ICD high-risk subgroup (P=0.01) (Figure 6B). The conclusions from two different cohorts had a great consistency, which supported the crucial impact on the prognosis in BC patients. Next, we investigated the independent prognostic power of the ICD risk signature via univariate and multivariate Cox analysis. The analysis showed that patients in the ICD high-risk subgroup had a close relationship with poor clinical outcome (Figure 6C). The conclusion from multivariate Cox analysis indicated that the risk signature was an independent risk factor (Figure 6D). Furthermore, the association between immune cells in the TME and risk score was investigated. Macrophage M0, mast cells resting, NK cells activated, and macrophage M2 showed a positive correlation of risk score. Meanwhile, macrophage M1, NK cells resting, T cells CD4 memory activated, CD8 T cells, and T cells follicular helper exhibited a negative correlation with risk score (Figure 7A). Finally, the analysis of immunotherapy response from TIDE was used to evaluate the potential efficacy (Figure 7B). Patients in the immunotherapy-non responder group had a lower risk score and patients in the immunotherapy responder group had a higher risk score. The results implied that patients in the ICD high risk subgroup may benefit from immunotherapy.
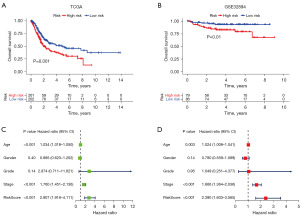
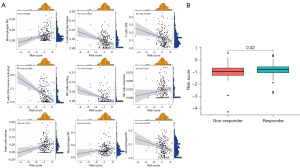
DiscussionOther Section
Depending on the development of immunity and the rising awareness of precision medicine, high-throughput sequencing and immunotherapy have been widely utilized to treat cancer. Due to their neoantigens, tumor cells have an important feature that they can escape from the attack of immune cells (30,31). In some situations, DAMPs, releasing from tumor cells with immune-activating patterns, enhance adaptive immune response, which has been observed in ICD (1). The immunogenic therapeutic value of this unique form of regulating cell death has been discussed in numerous studies (32-35). Although a minority of BC patients respond to immunotherapy, immunotherapy is considered one of the most promising treatments in BC. Hence, it is necessary to determine the ICD-related risk signature and subtype, which contribute to distinguishing BC patients based on immunotherapy.
Herein, two ICD-related subtypes were stratified via consensus clusters. Our results showed that the ICD-related genes were associated with clinical outcomes and had a crucial impact on the TME. A total of seven ICD-related genes (CALR, CASP8, CD8A, IFNB1, IFNG, IFNGR1, and MYD88) were identified and constructed an ICD-related risk signature. The risk signature successfully distinguished different risk subgroups in BC. In addition, the signature indicated an outstanding predictive power in the light of OS, which highlighted its potential as an independent prognostic predictor. Most of ICD-related genes were found to be closely related to tumor progression and ICD. Calreticulin (CALR) is an endoplasmic reticulum-associated chaperone, which is considered to participate in integrin-dependent cell adhesion and transmit “eat me” signals to antigen-presenting cells (APCs) (36-38). A previous study suggested that the sine qua condition of tumor-targeting immune response and immunogenicity for dying or dead diverse cancer cells is the surface exposure of calreticulin (39). Caspase-8 (CASP8) has long been considered the promotor of apoptosis and part of the procession that cytotoxic drugs that kill tumor cells (40). Lan et al. found that CASP8 was blocked via nanoformulation in a second near-infrared nanosystem, which transformed into more immunogenic death patterns (41). T-cell surface glycoprotein CD8 alpha chain (CD8A) was found to interact with the T cell receptor and the MHC class I protein presented by APCs, simultaneously (42). Interferon beta (IFNB1) belongs to the type I IFNs, which has been widely recognized to exert anticancer activities (43). Interferon gamma (IFNG) interacts with interferon gamma receptor 1 (IFNGR1) via its homodimers to regulate adaptive immunity and gene expression (44,45). Myeloid differentiation primary response protein 88 (MYD88) is the adaptor molecular of Toll-like receptor (TLR), which is involved in TLR/MYD88 and IL-1 receptor signaling pathways in innate immunity (46-48). A previous study indicated that the interaction of TLR/MYD88 and COX-2/PGE2 signaling played a vital role on the generation of an inflammatory microenvironment and tumorigenesis (49). Mechanistically, the exposure of diverse DAMPs facilitated the occurrence of ICD. Diversified pattern recognition receptors were exhibited via innate immune cells, including macrophages, dendritic cells, and monocytes. Due to limited condition, we elucidated immune infiltration of five immune cells (macrophages, dendritic cells, CD4 T cells, CD8 T cells, and regulatory T cells) in BC tissue. The subtype of macrophages was not listed. Nevertheless, an Asian omics study of the TME in BC showed more detailed content of immune cells. Our results were in general agreement with the results from the Asian omics study and TCIA. Previous research summarized the potential factors that might affect survival benefit in immunotherapy, including the expression of immune checkpoints and genes underlying innate and adaptive immune activity, tumor mutation burden (TMB), apolipoprotein B messenger RNA (mRNA) editing catalytic polypeptide-like (APOBEC) mutation signatures, and the number of alleles encoding high-affinity variants of activating Fcγ receptors. Individual biomarkers did not comprehensively identify patients who could benefit from therapy; however, multi-parameter models incorporating genomic alteration, immune responses, and tumor growth showed promising predictive utility, which emphasized the critical role of constructing a comprehensive signature of ICD in BC (50). Meanwhile, we showed that high-risk subgroups have a poorer OS but are better responders to immunotherapy. The poor prognosis in the high-risk group in our study was analyzed without receiving any immunotherapy, whereas ICD can be induced in a variety of ways, including chemotherapy, radiotherapy, photodynamic therapy, and certain biological agents. These therapies can cause tumor cells to release DAMPs, which activate the immune system (51). Hence, the immune system of the ICD high-risk subgroup is more likely to be activated by drugs to exert an anti-tumor effect. Therefore, specific mechanisms and associations should be further explored with increased resources.
ConclusionsOther Section
In brief, our research is the first to focus on the association of the ICD risk subtype with prognosis and changes of TME in BC. An ICD-related risk signature was established and validated successfully, which offered a promising strategy to guide clinical practice based on immunotherapy. Our observations in this study may help clinical urologists to develop and unveil a new mechanism of BC for diagnosis and treatment in future.
AcknowledgmentsOther Section
Funding: The study was supported by
FootnoteOther Section
Reporting Checklist: The authors have completed the TRIPOD reporting checklist. Available at https://tcr.amegroups.com/article/view/10.21037/tcr-24-533/rc
Data Sharing Statement: Available at https://tcr.amegroups.com/article/view/10.21037/tcr-24-533/dss
Peer Review File: Available at https://tcr.amegroups.com/article/view/10.21037/tcr-24-533/prf
Conflicts of Interest: All authors have completed the ICMJE uniform disclosure form (available at https://tcr.amegroups.com/article/view/10.21037/tcr-24-533/coif). The authors have no conflicts of interest to declare.
Ethical Statement: The authors are accountable for all aspects of the work in ensuring that questions related to the accuracy or integrity of any part of the work are appropriately investigated and resolved. The study was conducted in accordance with the Declaration of Helsinki (as revised in 2013). This study was approved by the research ethics committee of The Second Affiliated Hospital of Nanchang University (No. 202402KRDPJX73088), and informed consent was provided by each patient before data analyses.
Open Access Statement: This is an Open Access article distributed in accordance with the Creative Commons Attribution-NonCommercial-NoDerivs 4.0 International License (CC BY-NC-ND 4.0), which permits the non-commercial replication and distribution of the article with the strict proviso that no changes or edits are made and the original work is properly cited (including links to both the formal publication through the relevant DOI and the license). See: https://creativecommons.org/licenses/by-nc-nd/4.0/.
ReferencesOther Section
- Rodrigues MC, Morais JAV, Ganassin R, et al. An Overview on Immunogenic Cell Death in Cancer Biology and Therapy. Pharmaceutics 2022;14:1564. [Crossref] [PubMed]
- Kroemer G, Galassi C, Zitvogel L, et al. Immunogenic cell stress and death. Nat Immunol 2022;23:487-500. [Crossref] [PubMed]
- Galluzzi L, Vitale I, Warren S, et al. Consensus guidelines for the definition, detection and interpretation of immunogenic cell death. J Immunother Cancer 2020;8:e000337. [Crossref] [PubMed]
- Bruschi M, Petretto A, Vaglio A, et al. Annexin A1 and Autoimmunity: From Basic Science to Clinical Applications. Int J Mol Sci 2018;19:1348. [Crossref] [PubMed]
- Gavins FN, Hickey MJ. Annexin A1 and the regulation of innate and adaptive immunity. Front Immunol 2012;3:354. [Crossref] [PubMed]
- Obeid M, Tesniere A, Ghiringhelli F, et al. Calreticulin exposure dictates the immunogenicity of cancer cell death. Nat Med 2007;13:54-61. [Crossref] [PubMed]
- Vanmeerbeek I, Sprooten J, De Ruysscher D, et al. Trial watch: chemotherapy-induced immunogenic cell death in immuno-oncology. Oncoimmunology 2020;9:1703449. [Crossref] [PubMed]
- Sistigu A, Yamazaki T, Vacchelli E, et al. Cancer cell-autonomous contribution of type I interferon signaling to the efficacy of chemotherapy. Nat Med 2014;20:1301-9. [Crossref] [PubMed]
- Nastasi C, Mannarino L, D'Incalci M. DNA Damage Response and Immune Defense. Int J Mol Sci 2020;21:7504. [Crossref] [PubMed]
- Preissner KT, Fischer S, Deindl E. Extracellular RNA as a Versatile DAMP and Alarm Signal That Influences Leukocyte Recruitment in Inflammation and Infection. Front Cell Dev Biol 2020;8:619221. [Crossref] [PubMed]
- Wang Y, Ma X, Su C, et al. Uric acid enhances the antitumor immunity of dendritic cell-based vaccine. Sci Rep 2015;5:16427. [Crossref] [PubMed]
- Ahmed A, Tait SWG. Targeting immunogenic cell death in cancer. Mol Oncol 2020;14:2994-3006. [Crossref] [PubMed]
- Bota DA, Chung J, Dandekar M, et al. Phase II study of ERC1671 plus bevacizumab versus bevacizumab plus placebo in recurrent glioblastoma: interim results and correlations with CD4+ T-lymphocyte counts. CNS Oncol 2018;7:CNS22. [Crossref] [PubMed]
- Vaes RDW, Hendriks LEL, Vooijs M, et al. Biomarkers of Radiotherapy-Induced Immunogenic Cell Death. Cells 2021;10:930. [Crossref] [PubMed]
- Wang X, Wu S, Liu F, et al. An Immunogenic Cell Death-Related Classification Predicts Prognosis and Response to Immunotherapy in Head and Neck Squamous Cell Carcinoma. Front Immunol 2021;12:781466. [Crossref] [PubMed]
- Sung H, Ferlay J, Siegel RL, et al. Global Cancer Statistics 2020: GLOBOCAN Estimates of Incidence and Mortality Worldwide for 36 Cancers in 185 Countries. CA Cancer J Clin 2021;71:209-49. [Crossref] [PubMed]
- Lenis AT, Lec PM, Chamie K, et al. Bladder Cancer: A Review. JAMA 2020;324:1980-91. [Crossref] [PubMed]
- Hurst C, Rosenberg J, Knowles M. SnapShot: Bladder Cancer. Cancer Cell 2018;34:350-350.e1. [Crossref] [PubMed]
- Martinez Rodriguez RH, Buisan Rueda O, Ibarz L. Bladder cancer: Present and future. Med Clin (Barc) 2017;149:449-55. [Crossref] [PubMed]
- Vasekar M, Degraff D, Joshi M. Immunotherapy in Bladder Cancer. Curr Mol Pharmacol 2016;9:242-51. [Crossref] [PubMed]
- Sjödahl G, Lauss M, Lövgren K, et al. A molecular taxonomy for urothelial carcinoma. Clin Cancer Res 2012;18:3377-86. [Crossref] [PubMed]
- Irizarry RA, Hobbs B, Collin F, et al. Exploration, normalization, and summaries of high density oligonucleotide array probe level data. Biostatistics 2003;4:249-64. [Crossref] [PubMed]
- Yu G, Wang LG, Han Y, et al. clusterProfiler: an R package for comparing biological themes among gene clusters. OMICS 2012;16:284-7. [Crossref] [PubMed]
- Ritchie ME, Phipson B, Wu D, et al. limma powers differential expression analyses for RNA-sequencing and microarray studies. Nucleic Acids Res 2015;43:e47. [Crossref] [PubMed]
- Newman AM, Steen CB, Liu CL, et al. Determining cell type abundance and expression from bulk tissues with digital cytometry. Nat Biotechnol 2019;37:773-82. [Crossref] [PubMed]
- Charoentong P, Finotello F, Angelova M, et al. Pan-cancer Immunogenomic Analyses Reveal Genotype-Immunophenotype Relationships and Predictors of Response to Checkpoint Blockade. Cell Rep 2017;18:248-62. [Crossref] [PubMed]
- Mayakonda A, Lin DC, Assenov Y, et al. Maftools: efficient and comprehensive analysis of somatic variants in cancer. Genome Res 2018;28:1747-56. [Crossref] [PubMed]
- Engebretsen S, Bohlin J. Statistical predictions with glmnet. Clin Epigenetics 2019;11:123. [Crossref] [PubMed]
- Garg AD, De Ruysscher D, Agostinis P. Immunological metagene signatures derived from immunogenic cancer cell death associate with improved survival of patients with lung, breast or ovarian malignancies: A large-scale meta-analysis. Oncoimmunology 2015;5:e1069938. [Crossref] [PubMed]
- Jiang T, Shi T, Zhang H, et al. Tumor neoantigens: from basic research to clinical applications. J Hematol Oncol 2019;12:93. [Crossref] [PubMed]
- Yamamoto TN, Kishton RJ, Restifo NP. Developing neoantigen-targeted T cell-based treatments for solid tumors. Nat Med 2019;25:1488-99. [Crossref] [PubMed]
- Yang W, Zhang F, Deng H, et al. Smart Nanovesicle-Mediated Immunogenic Cell Death through Tumor Microenvironment Modulation for Effective Photodynamic Immunotherapy. ACS Nano 2020;14:620-31. [Crossref] [PubMed]
- Li Y, Zhang H, Li Q, et al. CDK12/13 inhibition induces immunogenic cell death and enhances anti-PD-1 anticancer activity in breast cancer. Cancer Lett 2020;495:12-21. [Crossref] [PubMed]
- Duan X, Chan C, Lin W. Nanoparticle-Mediated Immunogenic Cell Death Enables and Potentiates Cancer Immunotherapy. Angew Chem Int Ed Engl 2019;58:670-80. [Crossref] [PubMed]
- Kroemer G, Galluzzi L, Kepp O, et al. Immunogenic cell death in cancer therapy. Annu Rev Immunol 2013;31:51-72. [Crossref] [PubMed]
- Nakamura K, Zuppini A, Arnaudeau S, et al. Functional specialization of calreticulin domains. J Cell Biol 2001;154:961-72. [Crossref] [PubMed]
- Michalak M, Groenendyk J, Szabo E, et al. Calreticulin, a multi-process calcium-buffering chaperone of the endoplasmic reticulum. Biochem J 2009;417:651-66. [Crossref] [PubMed]
- Kotsias F, Cebrian I, Alloatti A. Antigen processing and presentation. Int Rev Cell Mol Biol 2019;348:69-121. [Crossref] [PubMed]
- Poon IK, Lucas CD, Rossi AG, et al. Apoptotic cell clearance: basic biology and therapeutic potential. Nat Rev Immunol 2014;14:166-80. [Crossref] [PubMed]
- Jiang M, Qi L, Li L, et al. Caspase-8: A key protein of cross-talk signal way in "PANoptosis" in cancer. Int J Cancer 2021;149:1408-20. [Crossref] [PubMed]
- Lan P, Chen H, Guo Y, et al. NIR-II Responsive Molybdenum Dioxide Nanosystem Manipulating Cellular Immunogenicity for Enhanced Tumor Photoimmunotherapy. Nano Lett 2022;22:4741-9. [Crossref] [PubMed]
- Zhu J, Peng T, Johnston C, et al. Immune surveillance by CD8αα+ skin-resident T cells in human herpes virus infection. Nature 2013;497:494-7. [Crossref] [PubMed]
- Ambjørn M, Ejlerskov P, Liu Y, et al. IFNB1/interferon-β-induced autophagy in MCF-7 breast cancer cells counteracts its proapoptotic function. Autophagy 2013;9:287-302. [Crossref] [PubMed]
- Wu S, Liu X, Wang Y, et al. Genetic Polymorphisms of IFNG and IFNGR1 with Latent Tuberculosis Infection. Dis Markers 2019;2019:8410290. [Crossref] [PubMed]
- Huang J, Chen P, Liu K, et al. CDK1/2/5 inhibition overcomes IFNG-mediated adaptive immune resistance in pancreatic cancer. Gut 2021;70:890-9. [Crossref] [PubMed]
- Kawai T, Sato S, Ishii KJ, et al. Interferon-alpha induction through Toll-like receptors involves a direct interaction of IRF7 with MyD88 and TRAF6. Nat Immunol 2004;5:1061-8. [Crossref] [PubMed]
- Semaan N, Alsaleh G, Gottenberg JE, et al. Etk/BMX, a Btk family tyrosine kinase, and Mal contribute to the cross-talk between MyD88 and FAK pathways. J Immunol 2008;180:3485-91. [Crossref] [PubMed]
- Campbell GR, To RK, Hanna J, et al. SARS-CoV-2, SARS-CoV-1, and HIV-1 derived ssRNA sequences activate the NLRP3 inflammasome in human macrophages through a non-classical pathway. iScience 2021;24:102295. [Crossref] [PubMed]
- Echizen K, Hirose O, Maeda Y, et al. Inflammation in gastric cancer: Interplay of the COX-2/prostaglandin E2 and Toll-like receptor/MyD88 pathways. Cancer Sci 2016;107:391-7. [Crossref] [PubMed]
- Komura K, Hirosuna K, Tokushige S, et al. The Impact of FGFR3 Alterations on the Tumor Microenvironment and the Efficacy of Immune Checkpoint Inhibitors in Bladder Cancer. Mol Cancer 2023;22:185. [Crossref] [PubMed]
- Powles T, Sridhar SS, Loriot Y, et al. Avelumab maintenance in advanced urothelial carcinoma: biomarker analysis of the phase 3 JAVELIN Bladder 100 trial. Nat Med 2021;27:2200-11. [Crossref] [PubMed]