Nomogram for predicting the early death of patients with stage IV ovarian cancer: a retrospective analysis of the SEER database
Highlight box
Key findings
• We have successfully developed a nomogram and risk classification system to accurately predict the probability of early death in patients with stage IV ovarian cancer.
What is known and what is new?
• Ovarian cancer is a major health problem for women all over the world and tends to progress to advanced stages. Therefore, it is important to predict the early survival of patients with advanced ovarian cancer.
• The study successfully analyzed the risk factors for their early mortality and constructed a prediction model based on them.
What is the implication, and what should change now?
• This nomogram can effectively help clinicians to choose better treatment strategies for these patients.
Introduction
Ovarian cancer is a malignant tumor that occurs in the tissue of a woman’s ovary. It is one of the tumors with the highest mortality rate in the female reproductive system and the most common type of gynecological malignancy (1). Ovarian cancer usually occurs in middle-aged or older women and is especially common in women over the age of 50 years old (2,3).
Ovarian cancer in the early stages comes usually with some of the common symptoms including abdominal distension, indigestion, bloating, frequent urination, loss of appetite, and weight loss. These symptoms may also be associated with other gynecological problems, making early diagnosis of ovarian cancer a challenge (3). Due to the insidious nature of early symptoms of ovarian cancer, it is difficult for patients who have not received regular medical checkups to achieve early detection (4). Therefore ovarian cancer often develops to stage IV, which will bring great psychological and physical burden to patients. Survival time is often one of the most important concerns for patients with advanced ovarian cancer. Accurate prediction of short-term survival status is also a major challenge for clinicians.
Once ovarian cancer is diagnosed, further staging and evaluation will help determine the best course of treatment. The treatment for ovarian cancer is usually surgery in conjunction with chemotherapy. Surgery is usually the treatment of choice with the aim to remove as much of the tumor as possible or to reduce tumor cells (5,6). Chemotherapy is also often used to treat ovarian cancer (7). The choice of treatment regimen depends on the type of cancer, its stage and individual circumstances (1). After reasonable clinical treatment, most of the patients can get complete remission. However, for patients with advanced stage, especially stage IV ovarian cancer, the recurrence rate is high and the prognosis is still poor (8). This is mainly because it is usually detected at a late stage, when the cancer has already spread to other organs. Early diagnosis and treatment are therefore crucial to improving the survival rate of patients.
Nomogram is a novel predictive tool that can be applied to various disease prognostic studies, and is now widely used in the prognostic prediction of many cancers. The data for this study came from the Surveillance, Epidemiology, and End Results (SEER) database, which contains clinical data on 28% of U.S. cancer patients (9-11). Early death is considered to be death due to all factors within 6 months after the patient is diagnosed. This is of great research value for the early prognosis of patients.
The aim of this study was to establish and internally validate a nomogram capable of predicting the early prognosis of patients with stage IV ovarian cancer for assisting doctors provide better medical strategies and helping patients choose treatment options. We present this article in accordance with the TRIPOD reporting checklist (available at https://tcr.amegroups.com/article/view/10.21037/tcr-24-625/rc).
Methods
Patients and data filtering
Patient data for this retrospective study were all obtained from, and contained clinical information on, 28% of U.S. cancer patients. Due to the publicly available database (Surveillance, Epidemiology, and End Results Program), ethical approval and informed patient consent were not required for our retrospective study. This study was conducted in accordance with the Declaration of Helsinki (as revised in 2013). We extracted patients’ clinical information including age, race, tumor grade, tumor stage, tumor histological type, marital status, chemotherapy, radiotherapy, surgical status, and survival status and survival time from the SEER database. After screening, 3,077 patients diagnosed from 2010–2015 were finally included. The specific screening process is shown in Figure 1.
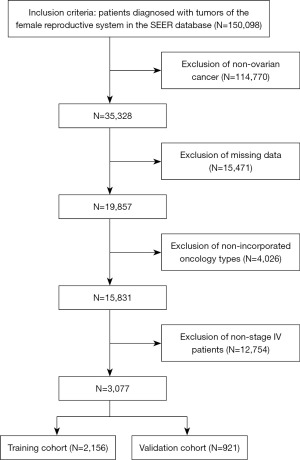
Statistical analysis
For internal validation purposes, the R software was used to randomly allocate cohorts into a training cohort and a validation cohort, maintaining a 7:3 ratio. In the training cohort, all variables were incorporated into the univariate logistic regression analysis. Subsequently, variables exhibiting a significant impact on total mortality were included in the multivariate logistic regression analyses to control for confounding effects. Through the multivariate logistic regression analyses, independent risk factors that significantly influenced survival were identified. A P value of 0.05 was deemed to possess statistical significance. These independent risk factors were employed to construct nomograms utilizing R software. The nomogram successfully predicted premature mortality in patients diagnosed with stage IV ovarian cancer. Receiver operating characteristic (ROC) curves were employed to evaluate the predictive capacity of this nomogram. Calibration curves were generated to evaluate the degree of concordance between the predicted outcomes and the actual outcomes. The horizontal coordinate is the actual value and the vertical coordinate is the predicted value, the closer the calibration curve is to 45° the more accurate the prediction is. X-tile software was used to select the optimal stage point to stratify patients for early mortality risk. In addition, Kaplan-Meier survival curves were used to predict the predictive value of the nomogram across risk strata. And finally, decision curve analysis (DCA) was used to assess how much benefit the nomogram would provide to patients. All analyses were performed by R software (version 4.2.1) and SPSS 25.0.
Results
Demographic characteristics
According to the screening process (Figure 1), a total of 3,077 patients were finally included in this study. Data on age, race, tumor grade, tumor stage, tumor histological type, marital status, radiotherapy status, and chemotherapy status of these patients were collected and counted (Table 1). All the included patients were then grouped in a ratio of 7:3 using R software, and the detailed data can be seen in Table 1.
Table 1
Variables | Total cohort, N=3,077 | Training cohort, N=2,156 | Validation cohort, N=921 |
---|---|---|---|
Age (years) | |||
≤60 | 1,265 (41.1) | 902 (41.8) | 363 (39.4) |
>60 | 1,812 (58.9) | 1,254 (58.2) | 558 (50.6) |
Race | |||
Black | 263 (8.5) | 173 (8) | 90 (9.8) |
White | 2,526 (82.1) | 1,763 (81.8) | 763 (82.8) |
Other | 288 (9.4) | 220 (10.2) | 68 (7.4) |
Grade* | |||
Grade I | 68 (2.2) | 47 (2.2) | 21 (2.3) |
Grade II | 255 (8.3) | 178 (8.3) | 77 (8.4) |
Grade III | 1,532 (49.8) | 1,063 (49.3) | 469 (50.9) |
Grade IV | 1,222 (39.7) | 868 (40.3) | 354 (38.4) |
Histological type | |||
Clear cell adenocarcinoma | 84 (2.7) | 61 (2.8) | 23 (2.5) |
Endometrioid carcinoma | 135 (4.4) | 102 (4.7) | 33 (3.6) |
Serous carcinoma | 2,781 (90.4) | 1,937 (89.8) | 844 (91.6) |
Mucinous carcinoma | 77 (2.5) | 56 (2.6) | 21 (2.3) |
Radiotherapy | |||
No | 3,034 (98.6) | 2,121 (98.4) | 913 (99.1) |
Yes | 43 (1.4) | 35 (1.6) | 8 (0.9) |
Chemotherapy | |||
No | 459 (14.9) | 311 (14.4) | 148 (16.1) |
Yes | 2,618 (85.1) | 1,845 (85.6) | 773 (83.9) |
Surgery | |||
No | 308 (10.0) | 211 (9.8) | 97 (10.5) |
Yes | 2,769 (90.0) | 1,945 (90.2) | 824 (89.5) |
Marital status | |||
No | 597 (19.4) | 408 (18.9) | 189 (20.5) |
Yes | 2,480 (80.6) | 1,748 (81.1) | 732 (79.5) |
Data are presented as n (%). *, grade: well differentiated (grade Ⅰ), moderately differentiated (grade II), poorly differentiated (grade III), undifferentiated (grade IV).
Logistic analysis
SPSS was used to perform one-way logistic regression analysis of various clinical oncology data of patients including age, race, tumor grade, tumor histological type, marital status, radiation therapy, and chemotherapy. Among them age, grade, histological type, surgery, and chemotherapy were considered as potential risk factors. In order to exclude confounding effects among variables, the variables screened by the above univariate logistic analysis were again included in the multivariate logistic analysis. Ultimately, grade, histological type, surgery, and chemotherapy were shown to be independent risk factors. The results of statistical analysis and data can be seen in Table 2.
Table 2
Variables | Univariate analysis | Multivariate analysis | |||
---|---|---|---|---|---|
HR (95% CI) | P value | HR (95% CI) | P value | ||
Age (years) | |||||
<60 | Reference | Reference | |||
≥60 | 0.544 (0.423–0.699) | <0.01 | 0.791 (0.585–1.070) | 0.13 | |
Race | |||||
Black | Reference | ||||
White | 1.129 (0.674–1.891) | 0.65 | |||
Other | 0.840 (0.578–1.220) | 0.36 | |||
Grade* | |||||
Grade I | Reference | Reference | |||
Grade II | 2.057 (1.039–4.071) | 0.04 | 0.511 (0.208–1.257) | 0.14 | |
Grade III | 0.890 (0.552–1.435) | 0.63 | 0.545 (0.297–1.000) | 0.050 | |
Grade IV | 1.182 (0.921–1.518) | 0.19 | 0.965 (0.715–1.302) | 0.82 | |
Histological type | |||||
Clear cell adenocarcinoma | Reference | Reference | |||
Endometrioid carcinoma | 0.875 (0.411–1.864) | 0.73 | 1.205 (0.477–3.049) | 0.69 | |
Serous carcinoma | 0.432 (0.210–0.891) | 0.02 | 0.401 (0.168–0.959) | 0.04 | |
Mucinous carcinoma | 0.271 (0.155–0.473) | <0.01 | 0.220 (0.107–0.454) | <0.01 | |
Radiotherapy | |||||
No | Reference | ||||
Yes | 0.884 (0.364–2.146) | 0.21 | |||
Chemotherapy | |||||
No | Reference | Reference | |||
Yes | 13.645 (10.345–17.996) | <0.01 | 12.883 (9.516–17.442) | <0.01 | |
Surgery | |||||
No | Reference | Reference | |||
Yes | 6.746 (4.984–9.132) | <0.01 | 6.714 (4.672–9.650) | <0.01 | |
Marital status | |||||
No | Reference | ||||
Yes | 1.242 (0.933–1.651) | 0.14 |
*, grade: well differentiated (grade I), moderately differentiated (grade II), poorly differentiated (grade III), undifferentiated (grade IV). HR, hazard ratio; CI, confidence interval.
Nomogram construction and validation
Variables screened by multifactorial logistic regression analysis were included for the construction of the nomogram, including grade, histological type, surgery, and chemotherapy (Figure 2). Each risk factor of the patient had a corresponding score, which can be obtained by making a plumb line from the corresponding marker to the coordinate axis in Figure 2. Summing the patient’s scores for each risk factor yielded a nomogram score, by virtue of which the patient’s probability of early death within 6 months can be inferred. ROC curves were used to assess this nomogram. The area under the curve for the training cohort and validation cohort were 0.816 and 0.827, respectively, demonstrating that this predictive model has excellent fidelity (Figure 3). Furthermore, the calibration curves for both the training and validation cohorts closely resemble a 45° angle, pointing out that this nomogram has high accuracy (Figure 4). After this, we performed DCA on both the training and validation cohorts, which proved that patients can get high economic benefits from this predictive model. So this nomogram has the ability to be applied to clinical decision making (Figure 5). Finally, we performed risk stratification and Kaplan-Meier test for these two cohorts by X-tile software. The results showed significant differences between the different risk stratifications, pointing out that the risk scores obtained from this nomogram have significant predictive value (Figure 6).
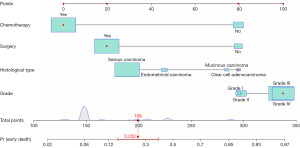
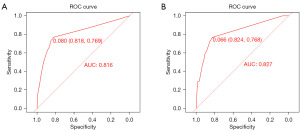
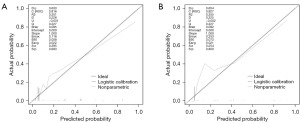
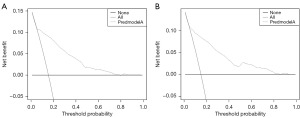
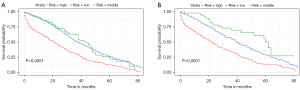
Interactive online nomogram
In order for clinicians to better apply the nomogram we developed, a network-based nomogram capable of predicting the early death of patients with stage IV ovarian cancer was built (https://abczz.shinyapps.io/dynnomapp/). For example, a stage IV, grade IV patient with clear cell adenocarcinoma who has undergone surgery and chemotherapy has an early probability of death of 0.2521 (Figure 7).
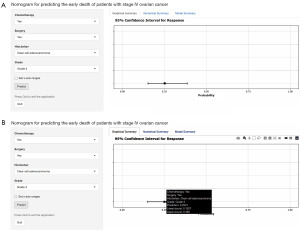
Discussion
Ovarian cancer, the fourth most common cause of female cancer deaths in the developed world, is undergoing a surge in therapeutic strategies in recent years (2). But many patients are diagnosed as well as reach stage IV and are often treated predominantly with palliative care in the absence of a complete treatment strategy. We have developed a nomogram to fill a gap in this field. It aims to provide clinicians with a basis for determining the risk of early death in patients with advanced ovarian cancer, with a view to improving patient prognosis.
Our findings conclude that tumor grade and histologic type correlate with early death in patients with advanced disease, which is consistent with other study. There is consensus that high-grade serous ovarian cancer has a very high probability of intra-abdominal spread. Although it has some initial sensitivity to chemotherapy, this sensitivity decreases with tumor recurrence (12-15). In contrast, low-grade serous ovarian cancers exhibit more inert behavior, which poses many difficulties for biologically targeted therapies (16). In addition, clear cell carcinoma has shown resistance to response to chemotherapy (3,17). However, one study noted that gemcitabine is 33% effective in platinum-resistant patients and may be an option for palliative care (18). Since the early stages often present with unilateral adnexal enlargement, mucinous ovarian cancer is usually easiest to diagnose at an early stage, and therefore mucinous ovarian cancer rarely progresses to advanced stages (19-21). In addition, although endometrioid carcinoma often occurs in younger women and has a better prognosis, patients with poorly differentiated carcinomas usually have a poorer prognosis and shorter survival (3,22). This is confirmed in our nomogram. Patients with different grades and histologic types of ovarian cancer often require different clinical treatment and care strategies, and more research is needed in this area.
In addition to this, our study has demonstrated that chemotherapy has a tremendous positive impact on the prognosis of advanced ovarian cancer. Previously, numerous studies have pointed out that ovarian cancer (especially plasma histology) is very sensitive to platinum-based chemotherapy (23,24). Especially for advanced patients, chemotherapy is widely used as a conventional first-line therapy to improve patient OS (23). The conventional chemotherapeutic agents for ovarian cancer are paclitaxel and carboplatin, and there are also studies that point to the potentially favorable efficacy of cisplatin (25-27). Research on the use of different combinations of chemotherapeutic agents and the methods of their use is still hotly pursued. Some studies have demonstrated that intraperitoneal injection of cisplatin together with intravenous paclitaxel has a more significant clinical effect on the clearance of tumor tissue infiltration, but it has not yet been widely used in the clinic due to the increased toxicity (27,28).
Of greater interest, our study found that the performance of surgery had a significant impact on reducing early mortality in patients with advanced ovarian cancer. This may be related to the use of adjuvant chemotherapy in conjunction. Cytoreduction is recommended for almost all patients with advanced ovarian cancer prior to chemotherapy to improve the efficiency of chemotherapy as well as to reduce the rate of tumor recurrence (5,6). Some advanced patients who are too ill to undergo cytoreduction or whose tumor cells have spread distantly are often recommended to receive neoadjuvant chemotherapy before surgery (7,29). This shows that surgery is extremely positive for the patient’s prognosis.
Interestingly, our study found that age after removal of confounding factors was not considered as an independent factor independently influencing early mortality in patients with stage IV ovarian cancer. Another study came to the same conclusion (30). Age is usually an important risk factor in the prognostic prediction of many cancers, which is related to the decreased tolerance of the body in the elderly. However, a systematic review has pointed out that elevated estrogen levels are strongly associated with ovarian carcinogenesis (31). Therefore, we consider the conclusions of this study to be highly reliable. The exclusion of age as an independent factor influencing early death in patients with stage IV ovarian cancer may be closely related to the decline in estrogen levels after menopause. However, there is no evidence to support this conclusion, and more studies are needed.
We have successfully developed a nomogram that accurately predicts the probability of early death in patients with stage IV ovarian cancer, but we must recognize the limitations of this study. Firstly, as a retrospective study, the lack of intervention and confounding bias of the variables is unavoidable. Secondly, due to the limitation of the database, many new therapeutic tools (e.g., targeted drugs) were not included in this study. However, this predictive model can still provide reliable theoretical support for clinicians to choose the best treatment strategy.
Conclusions
We have successfully developed a nomogram that accurately predicts early death in patients with stage IV ovarian cancer. Grade, histological type, surgery, and chemotherapy are considered to be independent risk factors affecting early death in patients with stage IV ovarian cancer. Validation of the nomogram have indicated that it has a good predictive performance and can provide good benefits to patients. It can bring good benefits to patients. This can help clinicians to make better clinical decisions and provide a theoretical basis for future advances in related areas of research.
Acknowledgments
Funding: This work was supported by
Footnote
Reporting Checklist: The authors have completed the TRIPOD reporting checklist. Available at https://tcr.amegroups.com/article/view/10.21037/tcr-24-625/rc
Peer Review File: Available at https://tcr.amegroups.com/article/view/10.21037/tcr-24-625/prf
Conflicts of Interest: All authors have completed the ICMJE uniform disclosure form (available at https://tcr.amegroups.com/article/view/10.21037/tcr-24-625/coif). All authors report that this work was supported by Major Science and Technology Programs in Jinhua City (2021-3-030). The authors have no other conflicts of interest to declare.
Ethical Statement: The authors are accountable for all aspects of the work in ensuring that questions related to the accuracy or integrity of any part of the work are appropriately investigated and resolved. The study was conducted in accordance with the Declaration of Helsinki (as revised in 2013).
Open Access Statement: This is an Open Access article distributed in accordance with the Creative Commons Attribution-NonCommercial-NoDerivs 4.0 International License (CC BY-NC-ND 4.0), which permits the non-commercial replication and distribution of the article with the strict proviso that no changes or edits are made and the original work is properly cited (including links to both the formal publication through the relevant DOI and the license). See: https://creativecommons.org/licenses/by-nc-nd/4.0/.
References
- Roett MA, Evans P. Ovarian cancer: an overview. Am Fam Physician 2009;80:609-16. [PubMed]
- Jayson GC, Kohn EC, Kitchener HC, et al. Ovarian cancer. Lancet 2014;384:1376-88. [Crossref] [PubMed]
- Stewart C, Ralyea C, Lockwood S. Ovarian Cancer: An Integrated Review. Semin Oncol Nurs 2019;35:151-6. [Crossref] [PubMed]
- Elias KM, Guo J, Bast RC Jr. Early Detection of Ovarian Cancer. Hematol Oncol Clin North Am 2018;32:903-14. [Crossref] [PubMed]
- Brand AH, DiSilvestro PA, Sehouli J, et al. Cytoreductive surgery for ovarian cancer: quality assessment. Ann Oncol 2017;28:viii25-9. [Crossref] [PubMed]
- Polom K, Roviello G, Generali D, et al. Cytoreductive surgery and hyperthermic intraperitoneal chemotherapy for treatment of ovarian cancer. Int J Hyperthermia 2016;32:298-310. [Crossref] [PubMed]
- Elies A, Rivière S, Pouget N, et al. The role of neoadjuvant chemotherapy in ovarian cancer. Expert Rev Anticancer Ther 2018;18:555-66. [Crossref] [PubMed]
- Berek JS, Renz M, Kehoe S, et al. Cancer of the ovary, fallopian tube, and peritoneum: 2021 update. Int J Gynaecol Obstet 2021;155:61-85. [Crossref] [PubMed]
- Gao J, Wang S, Li F, et al. Nomograms for Predicting Overall Survival and Cancer-Specific Survival of Young Patients with Epithelial Ovarian Cancer: Analysis Based on SEER Program. Adv Ther 2022;39:257-85. [Crossref] [PubMed]
- Ma G, Zeng S, Zhao Y, et al. Development and validation of a nomogram to predict cancer-specific survival of mucinous epithelial ovarian cancer after cytoreductive surgery. J Ovarian Res 2023;16:120. [Crossref] [PubMed]
- Sun H, Yan L, Chen H, et al. Development of a nomogram to predict prognosis in ovarian cancer: a SEER-based study. Transl Cancer Res 2020;9:5829-42. [Crossref] [PubMed]
- Au KK, Josahkian JA, Francis JA, et al. Current state of biomarkers in ovarian cancer prognosis. Future Oncol 2015;11:3187-95. [Crossref] [PubMed]
- Marcus CS, Maxwell GL, Darcy KM, et al. Current approaches and challenges in managing and monitoring treatment response in ovarian cancer. J Cancer 2014;5:25-30. [Crossref] [PubMed]
- Bowtell DD, Böhm S, Ahmed AA, et al. Rethinking ovarian cancer II: reducing mortality from high-grade serous ovarian cancer. Nat Rev Cancer 2015;15:668-79. [Crossref] [PubMed]
- Lisio MA, Fu L, Goyeneche A, et al. High-Grade Serous Ovarian Cancer: Basic Sciences, Clinical and Therapeutic Standpoints. Int J Mol Sci 2019;20:952. [Crossref] [PubMed]
- Kaldawy A, Segev Y, Lavie O, et al. Low-grade serous ovarian cancer: A review. Gynecol Oncol 2016;143:433-8. [Crossref] [PubMed]
- Gadducci A, Multinu F, Cosio S, et al. Clear cell carcinoma of the ovary: Epidemiology, pathological and biological features, treatment options and clinical outcomes. Gynecol Oncol 2021;162:741-50. [Crossref] [PubMed]
- Esposito F, Cecere SC, Magazzino F, et al. Second-line chemotherapy in recurrent clear cell ovarian cancer: results from the multicenter italian trials in ovarian cancer (MITO-9). Oncology 2014;86:351-8. [Crossref] [PubMed]
- Morice P, Gouy S, Leary A. Mucinous Ovarian Carcinoma. N Engl J Med 2019;380:1256-66. [Crossref] [PubMed]
- Olaoye T, - A, Boyle W, et al. Investigating age and ethnicity as novel high-risk phenotypes in mucinous ovarian cancer: retrospective study in a multi-ethnic population. Int J Gynecol Cancer 2024;34:1399-407. [Crossref] [PubMed]
- Crane EK, Brown J. Early stage mucinous ovarian cancer: A review. Gynecol Oncol 2018;149:598-604. [Crossref] [PubMed]
- Chen L, Pang S, Shen Y, et al. Low-grade endometrioid carcinoma of the ovary associated with undifferentiated carcinoma: case report and review of the literature. Int J Clin Exp Pathol 2014;7:4422-7. [PubMed]
- Konstantinopoulos PA, Matulonis UA. Clinical and translational advances in ovarian cancer therapy. Nat Cancer 2023;4:1239-57. [Crossref] [PubMed]
- Abdallah R, Atallah D, Bitar N, et al. Consensus on the management of platinum-sensitive high-grade serous epithelial ovarian cancer in Lebanon. Gynecol Oncol Rep 2023;47:101186. [Crossref] [PubMed]
- Chan JK, Brady MF, Penson RT, et al. Weekly vs. Every-3-Week Paclitaxel and Carboplatin for Ovarian Cancer. N Engl J Med 2016;374:738-48. [Crossref] [PubMed]
- Armstrong DK, Bundy B, Wenzel L, et al. Intraperitoneal cisplatin and paclitaxel in ovarian cancer. N Engl J Med 2006;354:34-43. [Crossref] [PubMed]
- Pignata S, Scambia G, Katsaros D, et al. Carboplatin plus paclitaxel once a week versus every 3 weeks in patients with advanced ovarian cancer (MITO-7): a randomised, multicentre, open-label, phase 3 trial. Lancet Oncol 2014;15:396-405. [Crossref] [PubMed]
- Markman M, Bundy BN, Alberts DS, et al. Phase III trial of standard-dose intravenous cisplatin plus paclitaxel versus moderately high-dose carboplatin followed by intravenous paclitaxel and intraperitoneal cisplatin in small-volume stage III ovarian carcinoma: an intergroup study of the Gynecologic Oncology Group, Southwestern Oncology Group, and Eastern Cooperative Oncology Group. J Clin Oncol 2001;19:1001-7. [Crossref] [PubMed]
- Lu Z, Chen J. Introduction of Who Classification of Tumours of Female Reproductive Organs, Fourth Edition. Zhonghua Bing Li Xue Za Zhi 2014;43:649-50.
- Mosgaard BJ, Meaidi A, Høgdall C, et al. Risk factors for early death among ovarian cancer patients: a nationwide cohort study. J Gynecol Oncol 2020;31:e30. [Crossref] [PubMed]
- Tanha K, Mottaghi A, Nojomi M, et al. Investigation on factors associated with ovarian cancer: an umbrella review of systematic review and meta-analyses. J Ovarian Res 2021;14:153. [Crossref] [PubMed]