Novel cuprotosis-related gene signature: a prognostic indicator and regulator of the glioma immune microenvironment
Highlight box
Key findings
• We identified copper homeostasis–related genes and examined their correlation with glioma patient prognosis.
What is known, and what is new?
• Cuproptosis, an unusual form of cell death, is closely associated with tumor progression and the tumor immune microenvironment.
• We identified three molecular subgroups of copper homeostasis-related genes linked to prognosis and found that these subgroups exhibited distinct and unique immune and biological characteristics.
What is the implication, and what should change now?
• These biomarkers are useful for diagnostic and prognostic purposes and could be used to guide our understanding of the progression and the treatment of glioma tumorigenesis.
Introduction
Glioma is the most common primary malignant tumor of the central nervous system (1). Despite significant progress in treatment strategies such as targeted molecular therapy (2) and immunotherapy (3), the 5-year overall survival (OS) rate of glioma remains below 35% (4). Glioma has the characteristic of infiltrative growth, and surgery cannot completely eradicate the tumor, which can recur in a short period after surgery. Even with aggressive surgical resection and subsequent systemic chemoradiotherapy, the median survival time for patients is only approximately 14.6 months (4). Developments in molecular biotechnology and genetics (5,6) have facilitated isocitrate dehydrogenase gene 1 and 2 (IDH1/2) mutations (7) and O6-methylguanine DNA methyltransferase (MGMT) initiation. With the discovery of submethylation (8), these mutations and methylation status can serve as prognostic indicators for glioma. However, the prognosis of glioma patients is still poor, and existing prognostic indicators vary in accuracy, resulting in insufficient prognostic guidance for patients (8).
The complexity of glioma and its progression correlates patient prognosis with multiple biological pathways (9). Therefore, relying solely on existing prognostic indicators may not fully reflect the prognosis. The discovery of new prognostic indicators through broader bioinformatics analysis is important for improving the prognostic assessment of glioma.
Copper is an essential co-factor for all organisms. Copper can increase mitochondrial protein lipoylation, a post-translational modification of lysine that occurs in four enzymes [i.e., dihydrolipoamide branched chain transacylase E2, glycine cleavage system protein H, dihydrolipoamide S-succinyltransferase, and dihydrolipoamide S-acetyltransferase (DLAT)] that regulate carbon entry into the tricarboxylic acid (TCA) cycle (9). Copper directly binds to DLAT, facilitating the disulfide bond-dependent aggregation of lipoylated DLAT (9). Copper becomes toxic if concentrations exceed the threshold maintained by evolutionarily conserved homeostatic mechanisms. The toxicity of copper overload is caused by releasing reactive oxygen species via Fenton or Haber-Weiss reactions, damaging lipid, protein, DNA, and RNA (10).
A recent study by Tsvetkov et al. showed that copper-dependent death, termed cuproptosis, occurs by copper directly binding to lipoylated components of the TCA (11). Cuproptosis is a novel form of regulated cell death that differs from both zinc-induced cell death and ferroptosis. Cuproptosis is caused by the aggregation of lipoylated mitochondrial enzymes and the loss of Fe-S cluster proteins (11). Copper accumulation results in cell death, indicating the need for classical copper homeostatic mechanisms. Previous studies have shown that the dysfunction of genes associated with copper homeostasis leads to pathological cuproptosis (12,13), and copper overload disorder can cause cell death (14).
Some research reports have shown that cuproptosis plays an important role in tumorigenesis and the tumor immune microenvironment. For example, studies on ovarian cancer and pancreatic cancer have shown that cuproptosis may significantly affect tumor progression and microenvironment by regulating cell metabolism and immune responses. These findings highlight the importance of cuproptosis as a potential novel tumor biological mechanism and suggest that it may provide new targets for cancer diagnosis and treatment (11,15-17). However, the function of cuproptosis in glioma is not yet known. Therefore, we conducted a systematic analysis to investigate the gene expression levels of copper homeostasis in glioma. We also examined the effect of these genes on tumor-associated pathways and immuno-infiltration to determine the prognosis subsets of neurogliomas related to cuproptosis. Based on our findings, a glioma prognosis prediction model was developed and validated using an external test set. We present this article in accordance with the TRIPOD reporting checklist (available at https://tcr.amegroups.com/article/view/10.21037/tcr-24-1860/rc).
Methods
Data sets
RNA sequencing data and glioblastoma (GBM) samples were obtained from The Cancer Genome Atlas (TCGA) (https://portal.gdc.cancer.gov/) and Chinese Glioma Genome Atlas (CGGA) (http://www.cgga.org.cn/), respectively. In TCGA, a total of 669 samples were obtained, while in CGGA, 1,018 samples were obtained. The study was conducted in accordance with the Declaration of Helsinki (as revised in 2013).
CNV and mutation analysis of the copper homeostasis-related genes
In total, 51 copper homeostasis-related genes were obtained by searching the gene set enrichment analysis (GSEA) database (https://www.gsea-msigdb.org/). The copy number variation (CNV) analysis by R (version 4.1.2) and Perl software (version 5.34.0) showed the distribution of the copper homeostasis–related genes in chromosomes using the “RCircos” package, and a waterfall diagram was generated with the genetic mutations using the “maftools” package. The “ggplot2” package was used to visualize the significance of the links between the gene mutations and expression levels.
Prognosis analysis of copper homeostasis-related genes
We assessed the association between the copper homeostasis-related genes and patient prognosis using a fragment per kilobase million value >5 as the cut-off value. The prognosis correlation network and copper homeostasis-related genes were drawn by R packages “igraph”, “psych”, “reshape2”, and “RColorBrewer”. The subtype glioma clustering analysis was performed using the “ConsensusClusterPlus” R package, and the cumulative distribution function (CDF) consistency and the region of the CDF curve were used to identify selected genes. The “survival” package was used to generate the survival curve. A Kaplan-Meier analysis was conducted to investigate the prognosis of the subset. The “stats” package was used for the principal component analysis (PCA).
Gene function analysis
We performed an immune cell infiltration analysis and single-sample GSEA (ssGSEA) of the copper homeostasis-related genes to examine the function of the different subsets. We evaluated the percentage of immune and compared them between different subsets using the “GSEABase” package. The “TIMER 1.0” (11,15,16) database was used to assess the correlation between the expression of the copper homeostasis-related genes and the infiltration level of six immune cells in glioma. The “CIBERSORT” package was used to examine the characteristics of the tumor microenvironment and predict the infiltration status of the immune cells. The expression of the 22 infiltrating immune cells was compared between the risk groups. The expression of different signaling pathways was determined for each subtype using the “GSVA” package for the gene set variation analysis (GSVA). The “CellMiner” (18) platform was used for the drug sensitivity analysis.
Derivation and validation of the prognostic model
The prognostic model was based on TCGA data, which served as the training cohort. The CGGA data served as the test cohort and was used to examine the stability of the model. First, we inspected the relationship between the copper homeostasis-related genes and survival conditions by least absolute shrinkage and selection operator (LASSO) regression. Based on the penalty parameter (λ), nine genes were identified, which were then included in the multivariate Cox regression. To select the optimal genes, we identified the differentially expressed genes related to copper homeostasis in glioma using “limma”, “reshape2”, and “ggpubr” in R language. A multivariate Cox regression analysis was conducted to identify the most significantly connected common differentially expressed genes in glioma. Additionally, the Kaplan-Meier method was used to analyze survival curves in TCGA and CGGA data sets. The “survivalROC” package was used to represent the receiver operating characteristic (ROC) curves for 1-, 3-, and 5-year OS, and examine the prediction ability of our model.
Statistical analysis
Kaplan-Meier, LASSO, and multivariate Cox regression analyses were conducted to investigate the independent prognostic value of the risk model. R software (version 4.1.2) was used to conduct the statistical analyses and visualize the graphs. The overall flow diagram for the study is shown in Figure 1A.
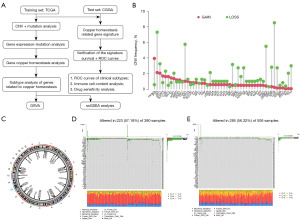
Results
Genetic variations in the copper homeostasis-related genes in glioma
Through GSEA, we identified a total of 51 genes related to copper homeostasis. First, we summarized the changes of these genes in glioma. The analysis results showed that in patients with glioma, the incidence of deletion of genes related to copper homeostasis was higher. Specifically, phosphatase and tensin homolog (PTEN), forkhead box transcription factor O1 (FOXO1), caspase 3 (CASP3), and ATPase beta (ATP7B) were particularly lost (Figure 1B,1C). These findings provide an important basis for us to construct mutational characteristics of genes related to copper homeostasis in glioma patients (Figure 1D,1E). Among them, key copper homeostasis-related genes include solute carrier family 31 member 1 (SLC31A1), microtubule-associated protein tau (MAPT), ATP7B, and prostate six-transmembrane epithelial antigen 3 (STEAP3).
Correlation between the expression of the copper homeostasis-related genes and prognosis
To explore the relationship between copper homeostasis and the prognosis of glioma patients, we conducted a comprehensive analysis of 51 genes related to copper homeostasis and evaluated their expression levels, interactions, and prognostic significance (Figure 2A). The results showed that most of the up- or down-regulated copper homeostasis-related genes had significant value in prognostic prediction.
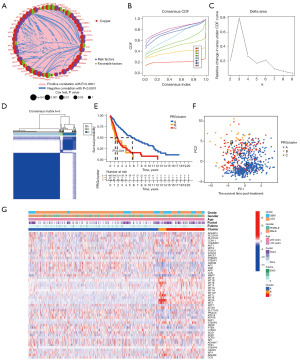
Next, we used a consensus clustering approach to classify glioma subtypes based on the expression of genes involved in copper homeostasis. By comparing the relative changes in the CDF area for each number of categories k and k-1, we found that when 3 clustering schemes (k=3) are selected, the growth rate of the CDF area is significantly accelerated (Figure 2B). When k=3, the growth rate of the CDF value is close to the peak. When the number of categories is increased from 2 to 8, the rising rate of the CDF curve slows down (Figure 2C). In addition, Figure 2D shows that the within-group relationship among different subtypes is strong, while the between-group relationship is moderate. Therefore, these results indicate that the classification of TCGA glioma samples based on the expression levels of genes related to copper homeostasis is reasonable.
At the same time, Figure 2E shows that the prognosis of patients with subtype B is significantly worse than that of patients with subtype A and subtype C, and subtype A has the most favorable prognosis for glioma patients (P<0.001). Furthermore, PCA results confirmed the clear distinguishability of the three subtypes in two-dimensional space (Figure 2F).
We also investigated phenotypic and genomic differences between these three subtypes. First, we generated heat maps demonstrating basic characteristics of glioma patients and differential expression of copper homeostasis-related genes in the TCGA cohort (Figure 2G). We found that patients with subtypes B and C had the worst survival prognosis. These subtypes accounted for the largest proportion of GBM-type gliomas and represented the less differentiated and more malignant subtypes in this study.
Copper homeostasis-related gene function analysis
Gene Ontology (GO) and Kyoto Encyclopedia of Genes and Genomes (KEGG) analyses were conducted to examine the biological functions of the copper homeostasis–related genes in glioma. The GO analysis revealed that the genes were mainly enriched in basic biological processes, such as the metal ion response and metal ion homeostasis transition (Figure 3A). While the KEGG analysis revealed that the genes were mainly related to mineral absorption and platinum drug resistance (Figure 3B).
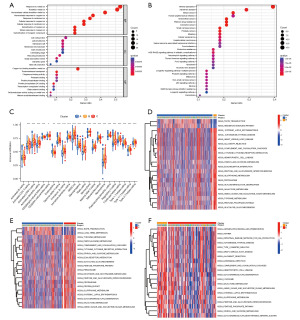
To explore the role of copper homeostasis in glioma immune response, we compared the immune activity of different subtypes by conducting a ssGSEA of TCGA cohort (Figure 3C). In relation to the enrichment scores of the 23 immune cell types, we discovered that subtype A exhibited a higher degree of infiltration compared to other clusters among these immune cells, particularly in activated cytotoxic T lymphocytes (CD8 T cells), immature B cells, and neutrophils. The cluster C subtype also exhibited higher enrichment scores for most of the immune cells compared to the cluster B subtype.
We conducted a GSVA to compare the cell functions and different pathways in the distinct different glioma subsets to evaluate their biological roles. Our analysis revealed that cluster A was significantly enriched in taste transduction and hedgehog signaling (Figure 3D), while Cluster C was significantly enriched in tyrosine and phenylalanine metabolism, the complement and coagulation cascades, and glutathione metabolism (Figure 3E), and Cluster B was significantly enriched in the intestinal immune network for immunoglobulin A (IgA) production, hematopoietic cell lineage, the cytokine receptor interaction, the pentose phosphate pathway, and glutathione metabolism (Figure 3F). Thus, classifying glioma based on copper homeostasis-related genes primarily revealed differences in various expression pathways, including those involved in immune response and metabolism.
Development of a prognostic model for glioma based on copper homeostasis-related genes
Table 1 sets out the clinicopathological characteristics of the glioma patients. Given the significance of copper homeostasis in tumors, we created a predictive prognostic model using TCGA data. First, to select the optimal genes, nine genes were identified based on the optimal λ value by LASSO regression analysis (Figure 4A,4B). We then identified six genes using the multivariate Cox regression analysis (Figure 4C). Next, using the “limma” package in R, 20 copper homeostasis-related genes were identified as being differentially expressed in glioma (Figure 4D). Finally, four optimal genes were identified using the earlier methods (Figure 4E and Table 2).
Table 1
Data | TCGA (training set; N=669) | CGGA (test set; N=1,018) |
---|---|---|
Age, years | ||
≥60 | 146 (21.8) | 116 (11.4) |
<60 | 463 (69.2) | 902 (88.6) |
Unknown | 60 (9.0) | – |
Gender | ||
Male | 355 (53.1) | 417 (41.0) |
Female | 254 (38.0) | 601 (59.0) |
Unknown | 60 (9.0) | |
OS (days) | 537 (264.0–1,011.0) | 836.5 (329.8–2,032.3) |
State | ||
Alive | 430 (64.3) | 362 (35.6) |
Dead | 239 (25.7) | 617 (60.6) |
Unknown | – | 39 (3.8) |
IDH mutation status | ||
Wild type | 233 (34.8) | 435 (42.7) |
Mutant | 429 (64.1) | 531 (52.2) |
Unknown | 7 (1.0) | 52 (5.1) |
1p19q codeletion status | ||
Codel | 169 (25.3) | 212 (20.8) |
Non-codel | 494 (73.8) | 728 (71.5) |
Unknown | 6 (0.9) | 78 (7.7) |
MGMTp methylation status | ||
Methylated | 477 (71.3) | 472 (46.4) |
Un-methylated | 161 (24.1) | 376 (36.9) |
Unknown | 31 (4.6) | 170 (16.7) |
Data are presented as n (%) or median (interquartile range). TCGA, The Cancer Genome Atlas; CGGA, Chinese Glioma Genome Atlas; OS, overall survival; IDH, isocitrate dehydrogenase; MGMT, O6-methylguanine DNA methyltransferase.
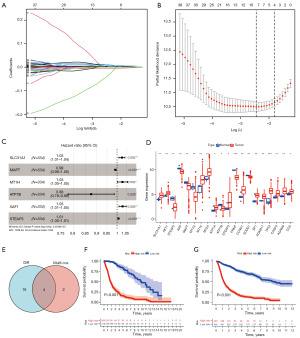
Table 2
ID | Coef | HR | HR.95L | HR.95H | P value |
---|---|---|---|---|---|
SLC31A1 | 0.025 | 1.054 | 1.045 | 1.064 | 7.52E−30 |
MAPT | −0.009 | 0.982 | 0.980 | 0.985 | 1.63E−39 |
ATP7B | −0.133 | 0.696 | 0.640 | 0.758 | 3.91E−17 |
STEAP3 | 0.007 | 1.015 | 1.013 | 1.017 | 5.23E−56 |
HR, hazard ratio; H, high; L, low.
The risk score was calculated as follows: risk score = (0.025 × SLC31A1 expression) + (−0.009 × MAPT expression) + (−0.133 × ATP7B expression) + (0.007 × STEAP3 expression). After completing the risk model, we used the CGGA data set to validate the performance of the prognostic model. TCGA and CGGA samples were separated into low- and high-risk subgroups according to the median risk score of TCGA cohort. Next, a survival analysis was conducted using the Kaplan-Meier curves. As Figure 4F,4G show, the high-risk group had the worst survival rate. As Figure 5A,5B show, the high-risk group of glioma patients had a higher risk score. Finally, we used the CGGA dataset as the test data set and showed that the predictive prognostic model was stable. The area under the curve (AUC) values of TCGA dataset were 0.900, 0.931, and 0.892 (Figure 5C). The areas under the ROC curves were 0.825, 0.876, and 0.886 for the 1-, 3-, and 5-year survival rates, respectively (Figure 5D). As Figure 5E shows, the gene model (AUC =0.881) outperformed age (AUC =0.803), gender (AUC =0.520), and grade (AUC =0.754) in the TCGA samples, while the gene model (AUC =0.822) outperformed age (AUC =0.616), gender (AUC =0.499), and grade (AUC =0.733) in the CGGA samples (Figure 5F).
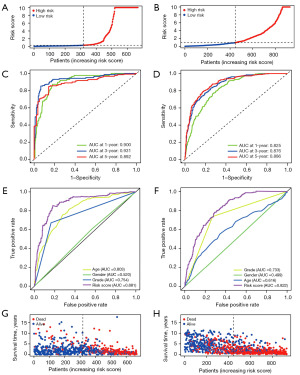
Further, according to the risk score survival time scatter plot, a difference was observed in the OS of the different risk score groups in TCGA (Figure 5G) and CGGA datasets (Figure 5H), and the survival status of the samples was positively correlated with the risk score. Specifically, our results showed that the high-risk subset had a higher mortality. Meanwhile, in TCGA and CGGA data sets, significant differences were found in the expression of the copper homeostasis-related genes in the distinct risk-score subsets. Specifically, in the high-risk model, SLC31A1 and STEAP3 expression were higher, and MAPT and ATP7B expression were lower (Figure 6A,6B). The results are consistent with those presented in Table 2. The expression of the copper homeostasis-related genes was assessed in the glioma tissues using data from the Human Protein Atlas (https://www.proteinatlas.org/) database. As Figure 6C-6F show, the MAPT, STEAP3, SLC31A1, and ATP7B expression levels differed significantly between the low-grade glioma (LGG) tissues and GBM specimens.
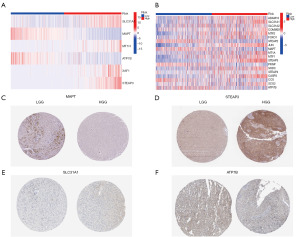
Tumor immune analysis of the prognostic model
To examine the correlation between the immune cells and the risk model, we estimated the composition of 22 immune cells in each sample. We compared the infiltration patterns among the different risk groups using CIBERSORT (Figure S1A,S1B). As Figure S1C-S1E show, the patterns of immune infiltration differed significantly between the low- and high-risk subgroups. Therefore, the findings indicate that the immune system has a significant influence on the regulatory mechanisms of the copper homeostasis genes.
The TIMER database was used to study the correlation between the expression of the copper homeostasis-related genes in the model and the immunization events in macrophages, B cells, CD4 T cells, mast cells, CD8 T cells, dendritic cells, and neutrophils (Figure 7A). Notably, we found that ATP7B and MAPT were positively correlated with central memory T cell (Tcm), Tgd, T follicular helper (TFH), eosinophils, and activated dendritic cells (aDCs) (Figure 7B,7C), and negatively correlated with macrophages and neutrophils. Additionally, we found that SLC31A1 and STEAP3 were positively correlated with Th2 cells, macrophages, eosinophils, neutrophils, and aDC (Figure 7D,7E). Figure 7F shows the correlation between the immune checkpoints and the copper homeostasis-related genes. Most of the genes in the high-risk model were significantly upregulated. It was negatively correlated with pDCs. This study revealed that STEAP3 and SLC31A1 were significantly positively correlated with macrophages, neutrophils, B cells, dendritic cells, and CD8 T cells in the LGG specimens (as shown in Figure S2). This suggests that STEAP3 and SLC31A1 are crucial genes involved in the tumor-immune interactions related to copper homeostasis.
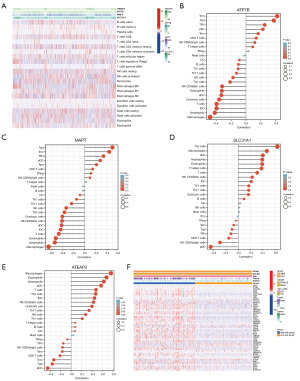
Copper homeostasis genes act as anti-tumor drug targets
We examined the association between the expression of the copper homeostasis-related genes and drug activity in 60 tumor cell lines. We also investigated the potential of the copper homeostasis-related genes as anti-tumor drug targets using the “CellMiner” database (Figure 8, Figures S3,S4) in pan-cancer. As shown in Figure 8, the expression levels of SLC31A1, MAPT, ATP7B, and STEAP3 in multiple anti-tumor drugs are associated with their anti-tumor effects. This suggests that the signaling pathways involved in pan-cancer and anti-tumor drug responses may be influenced by copper homeostasis.
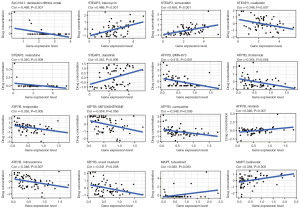
Discussion
Cuproptosis is a novel form of cell death. Studies have shown that cuproptosis might be involved in tumor immunity and treatment procedures (19-21). Copper, via various inflammatory factors, promotes the development and progression of tumors (such as IL-8, IL-6, and IL-1). Given the critical role of copper homeostasis in modulating the signaling pathways of anti-tumor drugs, as demonstrated in our study, copper emerges as a promising new target for cancer therapy. This insight could pave the way for the development of novel therapeutic strategies that leverage the regulation of copper to enhance the efficacy of existing treatments or to target resistant cancer cells.
Copper-induced cell death may be triggered by the binding of various drugs to proteins involved in maintaining copper homeostasis within tumor cells (19-21). Glioma is known for its particularly poor prognosis. However, to date, no studies have specifically investigated the influence of cuproptosis on the disease’s progression and patient outcomes.
Excess copper accumulation can induce cuproptosis (11). The mechanism of copper toxicity is associated with copper homeostasis, and it is essential to maintain copper homeostasis in cells. Currently, only 13 cuproptosis-related genes have been identified in the literature. These cuproptosis-related genes were unsuited for bioinformatic analysis, and the related results were unreliable. Thus, we downloaded copper homeostasis-related genes from the GSEA database in our study.
First, we analyzed the expression of 51 mutant genes and CNVs related to copper homeostasis in neuroglioma and found that their levels were relatively stable and conserved. Next, we identified the genes that were significantly associated with prognosis via the development of a network. To closely examine the prognostic value of the copper homeostasis regulators, we conducted a consensus clustering analysis and selected three subsets of neuroglioma samples according to copper homeostasis. The results revealed remarkable differences in the OS of the three subgroups of glioma patients. Meanwhile, the GSVA and ssGSEA results indicated that the copper homeostasis-related genes played a crucial role in certain immune-associated signaling pathways in tumor prognosis. In addition, the drug susceptibility analysis of the pan-cancer cell lines revealed multifarious relationships between copper homeostasis and the effects of anti-tumor drugs. We identified six copper homeostasis-related genes via LASSO and multivariate Cox analyses. The “limma” analysis identified 20 homeostasis-related genes. A Venn analysis was used to select the four optimal genes and examine their various characteristics in the clinical and distinct cohorts to create a risk model.
Analyses of multiple biological informatics and survival factors revealed that four cuproptosis-related genes (i.e., SLC31A1, ATP7B, MAPT, and STEAP3) were significantly associated with the prognosis of neuroglioma patients. SLC31A1 is a high-affinity plasma membrane copper importer that regulates copper homeostasis (22). Tsvetkov et al. reported that the overexpression of SLC31A1 increased susceptibility to cuproptosis in human embryonic kidney (HEK) 293T and ABC1 cells (11). Yu et al. (23) showed that SLC31AI expression was negatively correlated with the survival of pancreatic cancer patients. SLC31A1 has also been shown to promote tumor growth and inhibit the survival rate by enhancing the expression of PD-L1 in neuroblastoma and brain tumors (20).
ATP7B is crucial for maintaining cellular copper homeostasis, as it transports copper into vesicles for storage and facilitates the export of copper from cells, thus preventing the accumulation of excessive copper (24). A recent study showed that the deletion of ATP7B increased cuproptosis in a Wilson disease mouse model (11). Lukanović et al. revealed that the increased expression of ATP7B leads to poor survival and platinum resistance in ovarian cancer patients (25). However, to date, no study has examined the expression and function of ATP7B in glioma; thus, further research needs to be conducted.
STEAP3 is an oxidoreductase that is essential for copper uptake and homeostasis (26). In HEK293T cells, the overexpression of STEAP3 protein was shown to upregulate intracellular copper uptake (27). Recent studies showed that STEAP3 regulated ferroptosis in renal cell carcinoma (28,29). Thus, STEAP3 may be involved in cuproptosis. Studies have shown that the ablation of STEAP3 might inhibit cell proliferation and predicts favorable survival in glioma (30,31). In the present study, we verified that STEAP3 is a valuable prognostic biomarker for glioma patients, with higher expression levels being associated with a worse prognosis. Thus, this study confirmed the crucial role of STEAP3 in glioma.
MAPT is implicated in neurodegenerative disorders, such as Alzheimer’s disease, Parkinson’s disease, and progressive supranuclear palsy (32,33). Recent studies have suggested that the elevated expression of MAPT is beneficial to the survival outcomes of breast cancer, renal clear cell cancer, and LGG patients (34-36). A study on glioma found that the expression of MAPT depends on the genetic status of IDH1/2 and hinders the progression of tumors (37). However, to date, no research has been conducted on the relationship between MAPT and cuproptosis.
We also performed GSVA and ssGSEA to assess differences in the level of immune cell infiltration and the enrichment of pathways among distinct subtypes. The results showed that cuproptosis mainly affects the immune and inflammatory responses of glioma, which suggests that cuproptosis also affects the tumor-immune microenvironment.
Conclusions
We established an efficacious predictive model of prognostic glioma based on copper homeostasis-related genes and established a copper homeostasis-related gene prognostic signature with significant prognosis and differential clinical value. The genes in the model were significantly correlated to immune infiltration and drug susceptibility factors. Our risk model could provide guidance in the clinical management of glioma patients.
Acknowledgments
Funding: This work was supported by
Footnote
Reporting Checklist: The authors have completed the TRIPOD reporting checklist. Available at https://tcr.amegroups.com/article/view/10.21037/tcr-24-1860/rc
Peer Review File: Available at https://tcr.amegroups.com/article/view/10.21037/tcr-24-1860/prf
Conflicts of Interest: All authors have completed the ICMJE uniform disclosure form (available at https://tcr.amegroups.com/article/view/10.21037/tcr-24-1860/coif). The authors have no conflicts of interest to declare.
Ethical Statement: The authors are accountable for all aspects of the work in ensuring that questions related to the accuracy or integrity of any part of the work are appropriately investigated and resolved. The study was conducted in accordance with the Declaration of Helsinki (as revised in 2013).
Open Access Statement: This is an Open Access article distributed in accordance with the Creative Commons Attribution-NonCommercial-NoDerivs 4.0 International License (CC BY-NC-ND 4.0), which permits the non-commercial replication and distribution of the article with the strict proviso that no changes or edits are made and the original work is properly cited (including links to both the formal publication through the relevant DOI and the license). See: https://creativecommons.org/licenses/by-nc-nd/4.0/.
References
- Nussbaumer G, Benesch M, Grabovska Y, et al. Gliomatosis cerebri in children: A poor prognostic phenotype of diffuse gliomas with a distinct molecular profile. Neuro Oncol 2024;26:1723-37. [Crossref] [PubMed]
- Wang Z, Chen F, Cao Y, et al. An Engineered Nanoplatform with Tropism Toward Irradiated Glioblastoma Augments Its Radioimmunotherapy Efficacy. Adv Mater 2024;36:e2314197. [Crossref] [PubMed]
- Bao P, Gu HY, Jiang YC, et al. In Situ Sprayed Exosome-Cross-Linked Gel as Artificial Lymph Nodes for Postoperative Glioblastoma Immunotherapy. ACS Nano 2024;18:13266-76. [Crossref] [PubMed]
- Kidwell RL, Aghi MK. Lymphatic endothelial-like cells in the glioblastoma tumor niche drive metabolic alterations that promote stem cell proliferation and survival. Neuro Oncol 2024;26:783-4. [Crossref] [PubMed]
- Odia Y, Hall MD, Cloughesy TF, et al. Selective DRD2 antagonist and ClpP agonist ONC201 in a recurrent non-midline H3 K27M-mutant glioma cohort. Neuro Oncol 2024;26:S165-72. [Crossref] [PubMed]
- Ghosh S, Rothlin CV. TREM2 function in glioblastoma immune microenvironment: Can we distinguish reality from illusion? Neuro Oncol 2024;26:840-2. [Crossref] [PubMed]
- Wick W, Hartmann C, Engel C, et al. NOA-04 randomized phase III trial of sequential radiochemotherapy of anaplastic glioma with procarbazine, lomustine, and vincristine or temozolomide. J Clin Oncol 2009;27:5874-80. Erratum in: J Clin Oncol 2010;28:708. [Crossref] [PubMed]
- Weller M, Stupp R, Hegi ME, et al. Personalized care in neuro-oncology coming of age: why we need MGMT and 1p/19q testing for malignant glioma patients in clinical practice. Neuro Oncol 2012;14:iv100-8. [Crossref] [PubMed]
- Tang D, Chen X, Kroemer G. Cuproptosis: a copper-triggered modality of mitochondrial cell death. Cell Res 2022;32:417-8. [Crossref] [PubMed]
- Cervantes-Cervantes MP, Calderón-Salinas JV, Albores A, et al. Copper increases the damage to DNA and proteins caused by reactive oxygen species. Biol Trace Elem Res 2005;103:229-48. [Crossref] [PubMed]
- Tsvetkov P, Coy S, Petrova B, et al. Copper induces cell death by targeting lipoylated TCA cycle proteins. Science 2022;375:1254-61. [Crossref] [PubMed]
- Polishchuk EV, Merolla A, Lichtmannegger J, et al. Activation of Autophagy, Observed in Liver Tissues From Patients With Wilson Disease and From ATP7B-Deficient Animals, Protects Hepatocytes From Copper-Induced Apoptosis. Gastroenterology 2019;156:1173-1189.e5. [Crossref] [PubMed]
- Xia F, Fu Y, Xie H, et al. Suppression of ATG4B by copper inhibits autophagy and involves in Mallory body formation. Redox Biol 2022;52:102284. [Crossref] [PubMed]
- Kahlson MA, Dixon SJ. Copper-induced cell death. Science 2022;375:1231-2. [Crossref] [PubMed]
- Ishida S, Andreux P, Poitry-Yamate C, et al. Bioavailable copper modulates oxidative phosphorylation and growth of tumors. Proc Natl Acad Sci U S A 2013;110:19507-12. [Crossref] [PubMed]
- Shanbhag VC, Gudekar N, Jasmer K, et al. Copper metabolism as a unique vulnerability in cancer. Biochim Biophys Acta Mol Cell Res 2021;1868:118893. [Crossref] [PubMed]
- Li B, Severson E, Pignon JC, et al. Comprehensive analyses of tumor immunity: implications for cancer immunotherapy. Genome Biol 2016;17:174. [Crossref] [PubMed]
- Luna A, Elloumi F, Varma S, et al. CellMiner Cross-Database (CellMinerCDB) version 1.2: Exploration of patient-derived cancer cell line pharmacogenomics. Nucleic Acids Res 2021;49:D1083-93. [Crossref] [PubMed]
- Kannappan V, Ali M, Small B, et al. Recent Advances in Repurposing Disulfiram and Disulfiram Derivatives as Copper-Dependent Anticancer Agents. Front Mol Biosci 2021;8:741316. [Crossref] [PubMed]
- Voli F, Valli E, Lerra L, et al. Intratumoral Copper Modulates PD-L1 Expression and Influences Tumor Immune Evasion. Cancer Res 2020;80:4129-44. [Crossref] [PubMed]
- Liao Y, Zhao J, Bulek K, et al. Inflammation mobilizes copper metabolism to promote colon tumorigenesis via an IL-17-STEAP4-XIAP axis. Nat Commun 2020;11:900. [Crossref] [PubMed]
- Wu G, Peng H, Tang M, et al. ZNF711 down-regulation promotes CISPLATIN resistance in epithelial ovarian cancer via interacting with JHDM2A and suppressing SLC31A1 expression. EBioMedicine 2021;71:103558. [Crossref] [PubMed]
- Yu Z, Zhou R, Zhao Y, et al. Blockage of SLC31A1-dependent copper absorption increases pancreatic cancer cell autophagy to resist cell death. Cell Prolif 2019;52:e12568. [Crossref] [PubMed]
- Li Y, Chen H, Liao J, et al. Long-term copper exposure promotes apoptosis and autophagy by inducing oxidative stress in pig testis. Environ Sci Pollut Res Int 2021;28:55140-53. [Crossref] [PubMed]
- Lukanović D, Herzog M, Kobal B, et al. The contribution of copper efflux transporters ATP7A and ATP7B to chemoresistance and personalized medicine in ovarian cancer. Biomed Pharmacother 2020;129:110401. [Crossref] [PubMed]
- Wang LL, Luo J, He ZH, et al. STEAP3 promotes cancer cell proliferation by facilitating nuclear trafficking of EGFR to enhance RAC1-ERK-STAT3 signaling in hepatocellular carcinoma. Cell Death Dis 2021;12:1052. [Crossref] [PubMed]
- Ohgami RS, Campagna DR, McDonald A, et al. The Steap proteins are metalloreductases. Blood 2006;108:1388-94. [Crossref] [PubMed]
- Ye CL, Du Y, Yu X, et al. STEAP3 Affects Ferroptosis and Progression of Renal Cell Carcinoma Through the p53/xCT Pathway. Technol Cancer Res Treat 2022;21:15330338221078728. [Crossref] [PubMed]
- Passer BJ, Nancy-Portebois V, Amzallag N, et al. The p53-inducible TSAP6 gene product regulates apoptosis and the cell cycle and interacts with Nix and the Myt1 kinase. Proc Natl Acad Sci U S A 2003;100:2284-9. [Crossref] [PubMed]
- Zhao N, Huang Y, Wang YH, et al. Ferronostics: Measuring Tumoral Ferrous Iron with PET to Predict Sensitivity to Iron-Targeted Cancer Therapies. J Nucl Med 2021;62:949-55. [Crossref] [PubMed]
- Han M, Xu R, Wang S, et al. Six-Transmembrane Epithelial Antigen of Prostate 3 Predicts Poor Prognosis and Promotes Glioblastoma Growth and Invasion. Neoplasia 2018;20:543-54. [Crossref] [PubMed]
- Bloom GS. Amyloid-β and tau: the trigger and bullet in Alzheimer disease pathogenesis. JAMA Neurol 2014;71:505-8. [Crossref] [PubMed]
- Caillet-Boudin ML, Buée L, Sergeant N, et al. Regulation of human MAPT gene expression. Mol Neurodegener 2015;10:28. [Crossref] [PubMed]
- Bonneau C, Gurard-Levin ZA, Andre F, et al. Predictive and Prognostic Value of the TauProtein in Breast Cancer. Anticancer Res 2015;35:5179-84. [PubMed]
- Han X, Sekino Y, Babasaki T, et al. Microtubule-associated protein tau (MAPT) is a promising independent prognostic marker and tumor suppressive protein in clear cell renal cell carcinoma. Urol Oncol 2020;38:605.e9-605.e17. [Crossref] [PubMed]
- Zaman S, Chobrutskiy BI, Sikaria D, et al. MAPT (Tau) expression is a biomarker for an increased rate of survival for low-grade glioma. Oncol Rep 2019;41:1359-66. [PubMed]
- Gargini R, Segura-Collar B, Herránz B, et al. The IDH-TAU-EGFR triad defines the neovascular landscape of diffuse gliomas. Sci Transl Med 2020;12:eaax1501.