Radiomic signatures of brain metastases on MRI: utility in predicting pathological subtypes of lung cancer
Highlight box
Key findings
• In our study, radiomics models that are capable of predicting the pathological subtypes of lung cancer for patients with synchronous brain metastases (BMs) based on pre-treatment multimodal magnetic resonance imaging (MRI) images were constructed. Also, contrast-enhanced susceptibility weighted imaging (CE-SWI) can actually provide complementary radiomics discriminators between adenocarcinoma (AD) and small cell lung cancer (SCLC).
What is known and what is new?
• Previous studies have shown that radiomics features of BMs from contrast-enhanced computed tomography (CE-CT) and MRI images could predict the primary tumor types or differentiate pathological subtypes of lung cancers. However, CE-CT does not provide sufficient diagnostic power for BMs and radiomics features from CT images are not well generalizable. In comparison, MRI could show most of BMs in various sizes, yet the existing MRI radiomics-based analyses for differentiation are limited on conventional MRI sequences and performance of a single classifier, which lack in-depth exploration of additional information from other MRI sequences and classifiers.
• In the study, CE-SWI was incorporated into the radiomics models and deeply mined. The prediction performance of models using multiple classifiers were compared, and the model using extreme gradient boosting was proved to outperform other models for the differentiation task and recommended as a supportive decision tool.
What is the implication, and what should change now?
• This study demonstrated radiomics based on multiple magnetic resonance sequences showed excellent performance in distinguishing AD from SCLC in BMs of lung cancer. It is a non-invasive predictive tool to guide therapeutic strategies.
Introduction
Brain metastases (BMs) are the most common intracranial malignant tumors in adults (1). The primary tumors most likely to metastasize to the central nervous system are originating from lung (≥50%) (2), and approximately 50% of lung cancers develop BMs during the course of this systemic malignancy (3). Development of BMs substantially impairs neurological function and life quality of patients, and it is even a major cause of mortality (4,5). The high incidence and increasing rate of BMs from lung cancer impose a great burden on public health services.
Lung cancer is an entity of heterogeneous tumors, which is pathologically classified into non-small cell lung cancer (NSCLC) and small cell lung cancer (SCLC). NSCLC accounts for 80–85% of all cases, mainly containing adenocarcinoma (AD) and squamous cell carcinoma (SCC), and SCLC accounts for the remaining (6). Among these subtypes, non-SCC is an independent risk factor for BMs (7,8), implying that AD and SCLC are more likely to develop BMs (9). The pathological subtypes of lung cancers lead to various individual therapeutic strategies and indicate different prognosis (10). To date, the golden standard for diagnosis of subtypes is pathological examination of primary or metastatic lesions, which is an invasive and time-consuming procedure and even causes procedure-related complications, such as hemorrhage and operative infection (11). Therefore, it is of great clinical importance to non-invasively and timely identify pathological subtypes of lung cancer before confirmation by histological biopsy or cytology, especially in patients with synchronous presentation (BMs and primary tumor diagnosed at the same time).
Detection and characterization of BMs from lung cancer rely largely on contrast-enhanced brain magnetic resonance imaging (MRI). However, it could only offer limited information of BMs, such as the number, location, size or tumor enhancement, which lacks specificity for sub-classification of primary tumors. In recent years, radiomics as an emerging medical imaging analytic technique, which is able of extracting and analyzing massive quantitative imaging characteristics, has been introduced to distinguish tumor phenotypes for improving the interpretation of the biological properties and accelerating treatment initiation in brain tumors (12). Previous studies have shown that radiomics features of BMs from contrast-enhanced computed tomography (CE-CT) and MRI images could predict the primary tumor types or differentiate pathological subtypes of lung cancers for patients (13-16). However, smaller BMs on CE-CT are inconspicuous and easily missed, so CT does not provide sufficient diagnostic power for BMs and radiomics features from CT images are not well generalizable. In comparison, MRI could show most of BMs in various sizes, yet the existing MRI radiomics-based analyses for differentiation are limited on conventional MRI sequences, such as pre-contrast T1 weighted imaging (T1WI), fluid-attenuated inversion recovery (FLAIR) and contrast-enhanced T1WI (CE-T1WI), and there is no performance comparison of multiple classifiers. During the training of machine-learning (ML) models, the addition of complementary contrast with new information could expand the input parameter space, which might provide new features and result in a higher accuracy and reliability of classification. Contrast-enhanced susceptibility weighted imaging (CE-SWI) as an unconventional MRI sequence, which is more sensitive to iron, hemorrhage and vasculatures in the tumors than other conventional ones (17) and helpful in evaluating the pathophysiological profile of the tumors, could be incorporated into the radiomics models and deeply mined in BMs.
The aim of this study was to investigate the feasibility and accuracy of radiomics-based ML models to differentiate AD and SCLC for patients of lung cancer with synchronous BMs. It was hypothesized that prediction of the subtypes could be facilitated by imaging biomarkers of BMs derived from multiparametric MRI data including FLAIR, diffusion weighted imaging (DWI), CE-T1WI and CE-SWI. In addition, the performance of prediction in different classifiers were compared. We present this article in accordance with the TRIPOD reporting checklist (available at https://tcr.amegroups.com/article/view/10.21037/tcr-24-1147/rc).
Methods
Patients
The study was conducted in accordance with the Declaration of Helsinki (as revised in 2013). This study was approved by the Institutional Review Board (IRB) of Shanghai Chest Hospital, Shanghai Jiao Tong University (No. KS23008) and the requirement for written informed consent was waived. Patients of lung cancer with synchronous BMs treated in Shanghai Chest Hospital between January 2021 and December 2023 were retrospectively reviewed in this study. The inclusion criteria were as follows: (I) patients with diagnosis of primary lung cancer (finally confirmed by biopsy or cytology) and synchronous BMs; (II) patients underwent follow-up MRI within the next 6 months and the BMs were finally confirmed by overall consideration; (III) the metastatic lesions must be located in the brain parenchyma and identified both on CE-T1WI and CE-SWI, and the sizes of the lesions ≥3 mm. The exclusion criteria were as follows: (I) diagnosed with non-AD and non-SCLC; (II) patients with multiple malignant tumors, intracranial hemorrhage, brain radiation therapy or prior neurosurgery; (III) incomplete MRI images or image artifacts. The following clinical features were recorded: sex, age, smoking and the number of BMs. Eventually, 161 patients were enrolled into this study and 69 patients were excluded. A stratified random sampling approach was employed, where the patient cohort was first stratified based on the pathological subtypes of lung cancer—AD and SCLC. A total of 161 enrolled patients (121 with AD, 40 with SCLC) were divided into the training set and validation set randomly with a ratio of 4:1. The flowchart of patient recruitment for this study is shown in Figure 1.
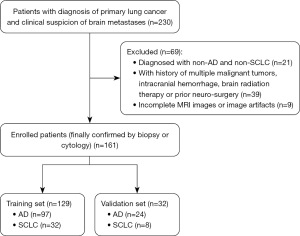
Magnetic resonance (MR) image acquisition and tumor region of interest (ROI) segmentation
Eligible patients were examined on the 3T MR units (Prisma or Vida; SIEMENS Medical Systems; Erlangen, Germany), and the sequences of FLAIR, DWI, CE-T1WI and CE-SWI were collected for feature extraction. CE-T1WI and CE-SWI were performed after injection of gadopentetate dimeglumine, which was administered at the standard dose of 0.1 mmol/kg of body weight. The scan parameters are shown in Table S1. On the axial CE-T1WI and CE-SWI sequences, the paired BMs were manually contoured slice-by-slice using the ITK-SNAP software (version 3.2), and the segmented regions of interest (ROIs) were verified on the consensus of two experienced neuroradiologists (with 12 and 23 years of clinical experience) and refined if necessary. Both of the neuroradiologists were blinded to all clinical information. The two masks were combined as the whole ROI mask, which was merged for subsequent feature extraction.
Image preprocessing
All images were resampled to voxel size of 1×1×1 mm3 by the Simple Insight Segmentation and Registration Toolkit (SimpleITK, https://github.com/SimpleITK/SimpleITK) package (18) to ensure the physical space consistency of different MR images. The N4 Bias Field Correction (19) was conducted to reduce the nonuniformity of the low-frequency signal intensity. And then FLAIR, DWI and CE-SWI were co-registered on the corresponding CE-T1WI using ANTsPy (https://github.com/ANTsX/ANTsPy) package (20). Subsequently, intensity normalization was utilized to all MRI images with the Z-score normalization method (21) for decreasing the effect of differences in image intensity.
Feature extraction
Three kinds of radiomics features from four MR sequences were extracted, including the shape [two-dimentional (2D), 3D] features, first-order features and high-order texture features. For the LoG filter, the sigma values were set to 3.0 and 5.0 mm, respectively. For the wavelet transform, all the images were filtered in the x, y, and z directions using a low and high bandpass filter. Eventually, 960 features were acquired, which included 14 shape features, 198 first-order features and 748 high-order texture features. The number distribution of features extracted in different image types is shown in Table S2.
Feature selection and model construction
The magnitudes of all the extracted features were uniformly converted into the same one for consistency before feature selection. For each feature vector, the mean and standard deviation in the training cohort were calculated, and then each feature vector was subtracted from the mean and divided by the standard deviation. The features’ correlation was evaluated by Pearson correlation.
Some redundant and irrelevant features were included in the initially extracted radiomics features. Mann-Whitney U tests were applied to select potentially informative features with P<0.05, and then the initially selected features were further filtered by the Least Absolute Shrinkage and Selection operator (LASSO) (22).
The selected informative features were inputted into four ML models, including logistic regression (LR) (23), random forest (RF) (24), support vector machine (25) (SVM) and extreme gradient boosting (XGBoost) (26). The Grid Search method and 5-fold cross-validation were applied to optimize the models and avoid overfitting in the training process. The performances of models were evaluated in the validation set. Hyperparameter values of models used in the prediction task are shown in Table S3. The feature selection and ML algorithms were implemented using the SciPy library (https://scipy.org/) and Scikit-learn Machine Learning library (https://scikit-learn.org/stable/) (25).
Statistical analysis
The statistical analysis was performed using SPSS (version 25.0; IBM Corp) and SciPy library (https://scipy.org/). Differences between groups were assessed using t-tests or Mann-Whitney U tests for continuous variables and Chi-squared tests for categorical variables. Accuracy (ACC), sensitivity (SEN), specificity (SPE), F1-score, the receiver operating characteristic curve (ROC) and the areas under the receiver operating characteristic curve (AUCs) were exploited for evaluating the ability of different models to predict subtypes of lung cancer. For the statistical analysis, P<0.05 was considered statistically significant.
Results
Patients’ clinical characteristics
One hundred and sixty-one patients (98 males and 63 females) were enrolled. There were 1,025 BMs (AD/SCLC: 714/311) in training set and 236 BMs (AD/SCLC: 105/131) in validation test. The pathological subtypes and clinical features of the enrolled patients in the training and validation sets are summarized in Table 1. As shown in Table 1, there were no significant differences in baseline characteristics between the training and validation sets.
Table 1
Characteristics | Training (n=129) | Validation (n=32) | P value |
---|---|---|---|
Subtypes (AD/SCLC) | 97/32 | 24/8 | 0.98 |
Gender (male/female) | 80/49 | 18/14 | 0.55 |
Age (years) | 62.3±7.1 | 59.9±8.6 | 0.43 |
Smokers | 46 (35.7) | 11 (34.4) | 0.89 |
BMs | 7.9±3.8 | 7.3±1.5 | 0.89 |
Location (AD/SCLC) | 1,025 (714/311) | 236 (105/131) | 0.08 |
Cerebrum | 863 (601/262) | 201 (81/120) | |
Cerebellum | 141 (101/40) | 33 (23/10) | |
Brain stem | 19 (11/8) | 2 (1/1) | |
Lateral ventricle | 2 (1/1) | 0 |
Data are presented as number, number (percentage) or mean ± SD. AD, adenocarcinoma; SCLC, small cell lung carcinoma; SD, standard deviation; BMs, brain metastases.
Feature selection and radiomics signature construction
The illustrative overview of the proposed subtype prediction pipeline of lung cancer is shown in Figure 2. Six key features on FLAIR, 21 on DWI, 6 on CE-T1WI and 8 on CE-SWI were finally selected. The optimal alpha in LASSO was set to 0.0124, 0.0180, 0.0164 and 0.0400 respectively according to the mean-square error (MSE) in the training process, and the process of LASSO feature selection is shown in Figure 3. The statistical analysis of the selected features in the training and validation sets is shown in Table S4. The key features extracted from FLAIR, DWI, CE-T1WI and CE-SWI in the bar chart were visualized and their normalized importance are shown in Figure 4. The heatmap of the feature correlation coefficient after feature selection is shown in Figure S1, in which higher color saturation indicated stronger inter-feature correlations and lower color saturation for lower inter-feature correlations.
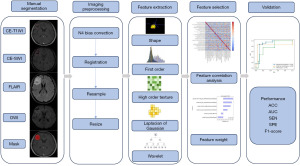
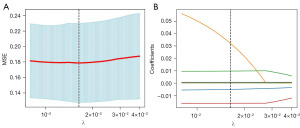
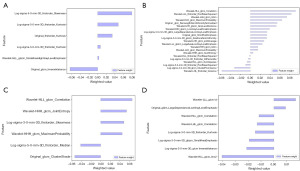
Model performance
The prediction performances of four ML models with different sets of features in the validation set are shown in Figure 5A,5B. It indicated that radiomics features alone showed excellent performance of prediction in the models of LR, RF and XGBoost, while incorporation of the clinical features only improved the prediction performance of SVM. The best prediction performance of radiomics features alone was achieved using the classifier of XGBoost, with ACC reaching 0.8750, SEN 0.8750, SPE 0.7108, F1 score 0.8686 and AUC 0.9062. The ROC curves of four ML models using radiomics features alone and radiomics plus clinical features in the validation set are shown in Figure 5C,5D. The confusion matrix of each ML model when predicting in the validation set is shown in Figure 6. In the best performing ML model of XGBoost, the combination model of conventional MRI sequences and CE-SWI showed better performance compared to the conventional MRI sequences with accuracy of 0.8750 vs. 0.8125, sensitivity of 0.8750 vs. 0.8125, specificity of 0.7108 vs. 0.6042, F1 score of 0.8686 vs. 0.8029 and AUC of 0.9062 vs. 0.7760, as shown in Figure 7.
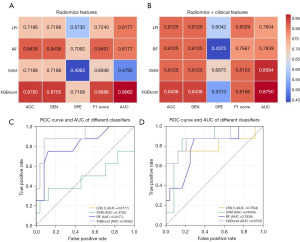
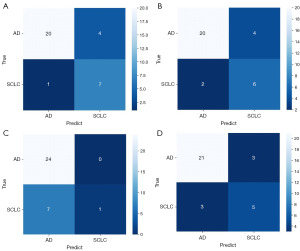
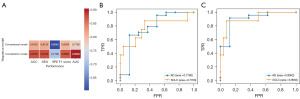
Discussion
In our study, radiomics models that are able of predicting the pathological subtypes of lung cancer for patients with synchronous BMs based on pre-treatment multimodal MRI images (combination of conventional MRI sequences and CE-SWI) were constructed. Also, CE-SWI can actually provide complementary radiomics discriminators between AD and SCLC. In the validation set with radiomics features alone, AUC values of 0.8177, 0.8177 and 0.9062 were achieved in the ML models using classifiers of LR, RF and XGBoost, while 0.4792 in the model using SVM. Hence, the proposed subtype prediction from BMs using the classifiers of LR, RF and XGBoost was promising, which could reduce diagnostic steps of lung cancers and expedite clinical practice. The model using XGBoost outperformed other models for the differentiation task presented in this study, which is recommended when utilized as a supportive decision and counseling tool.
The subtypes of lung cancer cannot be exactly predicted only by clinical characteristics, although patients with SCLC were strongly associated with male gender, senior age and smoking behavior (average age at diagnosis was 67.55±12.58 years) (27). In this study, we found that inclusion of basic clinical features (sex, age, smoking and the number of BMs) into radiomics features only improved the prediction performance of the SVM model, with AUC remarkably increasing from 0.4792 to 0.8594. The unsatisfactory performances in the other models might be related to the small sample size, and therefore we did not construct a nomogram due to the lack of practical significance.
When utilizing our recommended ML model of XGBoost, the combination of the conventional MRI sequences (FLAIR, DWI and CE-T1WI) and CE-SWI obtained higher performance of overall identification compared with the conventional MRI sequences only, yielding AUC of 0.9062 vs. 0.7760, accuracy of 0.8750 vs. 0.8125 and F1 score of 0.8686 vs. 0.8029 in the validation set. The F1 score as the weighted harmonic average of the precision and recall could supply a more practical evaluation for radiomics models, and it provides greater emphasis on the classification of interest when the dataset is imbalanced (the ratio of positive and negative classifications is different) (28,29). The F1 score of the combination model in this study was higher than that of the conventional MRI sequences in the validation set, which indicated that the combination of multiple MRI sequences may be more suitable and practical for differentiating AD from SCLC when the datasets were imbalanced.
To date, several studies have suggested that quantitative MRI radiomics features of BMs could be applied to predict subtypes in lung cancer (15,16). Whereas, they are mainly based on the conventional MRI sequences and lack in-depth exploration of additional information from other MRI sequences. It is reported that the potential future applications of SWI are texture analysis and radiomics (30). Petrujkić et al. (31) have shown that pre-contrast SWI could provide complementary discriminators of radiomics models in the differentiation of glioblastoma and solitary BM. The image quality of SWI was improved by application of contrast agent and higher field strength of MRI, and contrast enhancement and intralesional susceptibility effects can be assessed in one sequence of CE-SWI (32). In the radiologist readers’ view of CE-SWI images, the brain tumors could be enhanced as those shown on CE-T1WI, and the low-signal structures within a tumor defined as intra-tumoral susceptibility signals (ITSS) (33) reflecting microbleedings or neovessels were easily seen. However, visual observation of BMs on CE-SWI helps hardly to distinguish lung cancer subtypes. In our research, CE-SWI as an unconventional MRI sequence was introduced, and 8 key radiomics features from this sequence were detected. We found that the radiomics features extracted from the combination of conventional sequences and CE-SWI were more informative than conventional sequences in distinguishing AD from SCLC. The fact that neoplasm property caused by heterogeneous angiogenesis and microbleedings are more conspicuous on CE-SWI images than conventional MR images might be the probable explanation. Thus, the radiomics features extracted from CE-SWI potentially offer more information presenting the vascular-related changes, which might be correlated with tumor proliferation. We explored the heterogeneity of lung cancer by means of radiomics signatures in search for shape, textural and wavelet differences between BMs originating from AD and SCLC. To the best of our knowledge, our work is the first study to distinguish the two subtypes of lung cancer using radiomics features from CE-SWI images.
The following limitations remained in our study: (I) the study was conducted at a single medical center, and this would limit the generalizability of the findings; (II) only distinction between AD and SCLC restricts the utility of the model. Lung cancer also includes other subtypes, such as SCC, large cell carcinoma, carcinoid, sarcoma, although BMs from these subtypes are uncommon. The substantial expansion of the sample size, including multiple subtypes of lung cancer, would be helpful in improving the feasibility and accuracy of our model; (III) the lepto-meningeal and skull metastases were not included in this research, as ROI of the lepto-meningeal and skull metastases are hard to be outlined on CE-T1WI and/or CE-SWI although they are not rare; (IV) the areas of tumor enhancement and necrosis were not separately delineated, but heterogeneity of necrotic portion or ratio in analyzed groups may affect model performance. With the development of automatic segmentation technology, we are expected to further analyze the internal components of the tumor and calculate the necrotic portion or ratio in different subgroups in the future; (V) the clinical decisions regarding metastatic AD of the lung are based not only on pathological type of the tumor, but also on molecular features of the tumor such as presence/absence of driver mutations [e.g., epidermal growth factor receptor (EGFR), and anaplastic lymphoma kinase (ALK)] and programmed cell death-ligand 1 (PD-L1) expression. And we will focus on radiomics about some molecular features of lung cancer in the future.
Conclusions
This study demonstrated that radiomics features based on multiple MR sequences showed excellent performance in distinguishing AD from SCLC in BMs of lung cancer. It is a non-invasive predictive tool to complement established imaging and clinical measures that can be jointly applied to guide therapeutic strategies.
Acknowledgments
We would like to thank Jercy Chen for her help on polishing our paper.
Funding: This study has received funding from
Footnote
Reporting Checklist: The authors have completed the TRIPOD reporting checklist. Available at https://tcr.amegroups.com/article/view/10.21037/tcr-24-1147/rc
Data Sharing Statement: Available at https://tcr.amegroups.com/article/view/10.21037/tcr-24-1147/dss
Peer Review File: Available at https://tcr.amegroups.com/article/view/10.21037/tcr-24-1147/prf
Conflicts of Interest: All authors have completed the ICMJE uniform disclosure form (available at https://tcr.amegroups.com/article/view/10.21037/tcr-24-1147/coif). The authors have no conflicts of interest to declare.
Ethical Statement: The authors are accountable for all aspects of the work in ensuring that questions related to the accuracy or integrity of any part of the work are appropriately investigated and resolved. The study was conducted in accordance with the Declaration of Helsinki (as revised in 2013). This study was approved by the institutional review board (IRB) of Shanghai Chest Hospital, Shanghai Jiao Tong University (No. KS23008) and the requirement for written informed consent was waived.
Open Access Statement: This is an Open Access article distributed in accordance with the Creative Commons Attribution-NonCommercial-NoDerivs 4.0 International License (CC BY-NC-ND 4.0), which permits the non-commercial replication and distribution of the article with the strict proviso that no changes or edits are made and the original work is properly cited (including links to both the formal publication through the relevant DOI and the license). See: https://creativecommons.org/licenses/by-nc-nd/4.0/.
References
- Sacks P, Rahman M. Epidemiology of Brain Metastases. Neurosurg Clin N Am 2020;31:481-8. [Crossref] [PubMed]
- Soffietti R, Cornu P, Delattre JY, et al. EFNS Guidelines on diagnosis and treatment of brain metastases: report of an EFNS Task Force. Eur J Neurol 2006;13:674-81. [Crossref] [PubMed]
- Gavrilovic IT, Posner JB. Brain metastases: epidemiology and pathophysiology. J Neurooncol 2005;75:5-14. [Crossref] [PubMed]
- Sørensen JB, Hansen HH, Hansen M, et al. Brain metastases in adenocarcinoma of the lung: frequency, risk groups, and prognosis. J Clin Oncol 1988;6:1474-80. [Crossref] [PubMed]
- Mujoomdar A, Austin JH, Malhotra R, et al. Clinical predictors of metastatic disease to the brain from non-small cell lung carcinoma: primary tumor size, cell type, and lymph node metastases. Radiology 2007;242:882-8. [Crossref] [PubMed]
- Zheng M. Classification and Pathology of Lung Cancer. Surg Oncol Clin N Am 2016;25:447-68. [Crossref] [PubMed]
- Ji Z, Bi N, Wang J, et al. Risk factors for brain metastases in locally advanced non-small cell lung cancer with definitive chest radiation. Int J Radiat Oncol Biol Phys 2014;89:330-7. [Crossref] [PubMed]
- Derks SHAE, van der Veldt AAM, Smits M. Brain metastases: the role of clinical imaging. Br J Radiol 2022;95:20210944. [Crossref] [PubMed]
- Jin J, Zhou X, Liang X, et al. Brain metastases as the first symptom of lung cancer: a clinical study from an Asian medical center. J Cancer Res Clin Oncol 2013;139:403-8. [Crossref] [PubMed]
- Yousefi M, Bahrami T, Salmaninejad A, et al. Lung cancer-associated brain metastasis: Molecular mechanisms and therapeutic options. Cell Oncol (Dordr) 2017;40:419-41. [Crossref] [PubMed]
- Malone H, Yang J, Hershman DL, et al. Complications Following Stereotactic Needle Biopsy of Intracranial Tumors. World Neurosurg 2015;84:1084-9. [Crossref] [PubMed]
- Gillies RJ, Kinahan PE, Hricak H. Radiomics: Images Are More than Pictures, They Are Data. Radiology 2016;278:563-77. [Crossref] [PubMed]
- Zhang J, Jin J, Ai Y, et al. Differentiating the pathological subtypes of primary lung cancer for patients with brain metastases based on radiomics features from brain CT images. Eur Radiol 2021;31:1022-8. [Crossref] [PubMed]
- Béresová M, Larroza A, Arana E, et al. 2D and 3D texture analysis to differentiate brain metastases on MR images: proceed with caution. MAGMA 2018;31:285-94. [Crossref] [PubMed]
- Kniep HC, Madesta F, Schneider T, et al. Radiomics of Brain MRI: Utility in Prediction of Metastatic Tumor Type. Radiology 2019;290:479-87. [Crossref] [PubMed]
- Li Z, Mao Y, Li H, et al. Differentiating brain metastases from different pathological types of lung cancers using texture analysis of T1 postcontrast MR. Magn Reson Med 2016;76:1410-9. [Crossref] [PubMed]
- Sehgal V, Delproposto Z, Haddar D, et al. Susceptibility-weighted imaging to visualize blood products and improve tumor contrast in the study of brain masses. J Magn Reson Imaging 2006;24:41-51. [Crossref] [PubMed]
- Lowekamp BC, Chen DT, Ibáñez L, et al. The Design of SimpleITK. Front Neuroinform 2013;7:45. [Crossref] [PubMed]
- Tustison NJ, Avants BB, Cook PA, et al. N4ITK: improved N3 bias correction. IEEE Trans Med Imaging 2010;29:1310-20. [Crossref] [PubMed]
- Avants BB, Tustison N, Johnson H. Advanced Normalization Tools (ANTS), versions 2.x. Available online: https://psychiatry.ucsd.edu/research/programs-centers/snl/_files/ants2.pdf
- Fei N, Gao Y, Lu Z, et al. Z-Score Normalization, Hubness, and Few-Shot Learning. 2021 IEEE/CVF International Conference on Computer Vision (ICCV) 2021; 142-51.
- Kukreja SL, Löfberg J, Brenner MJ. A least absolute shrinkage and selection operator (lasso) for nonlinear system identification. IFAC Proc Vol 2006;39:814-9.
- Lever J, Krzywinski M, Altman N. Points of Significance: Model selection and overfitting. Nature Methods 2016;13:703. [Crossref]
- Breiman L. Random Forests. Machine Learning 2001;45:5-32. [Crossref]
- Hearst MA, Dumais ST, Osuna E, et al. Support vector machines. IEEE Intelligent Systems and their Applications 1998;13:18-28. [Crossref]
- Chen X, Huang L, Xie D, et al. EGBMMDA: Extreme Gradient Boosting Machine for MiRNA-Disease Association prediction. Cell Death Dis 2018;9:3. [Crossref] [PubMed]
- Tseng JS, Chiang CJ, Chen KC, et al. Association of Smoking With Patient Characteristics and Outcomes in Small Cell Lung Carcinoma, 2011-2018. JAMA Netw Open 2022;5:e224830. [Crossref] [PubMed]
- Saito T, Rehmsmeier M. The precision-recall plot is more informative than the ROC plot when evaluating binary classifiers on imbalanced datasets. PLoS One 2015;10:e0118432. [Crossref] [PubMed]
- DeVries Z, Locke E, Hoda M, et al. Using a national surgical database to predict complications following posterior lumbar surgery and comparing the area under the curve and F1-score for the assessment of prognostic capability. Spine J 2021;21:1135-42. [Crossref] [PubMed]
- Schwarz D, Bendszus M, Breckwoldt MO. Clinical Value of Susceptibility Weighted Imaging of Brain Metastases. Front Neurol 2020;11:55. [Crossref] [PubMed]
- Petrujkić K, Milošević N, Rajković N, et al. Computational quantitative MR image features - a potential useful tool in differentiating glioblastoma from solitary brain metastasis. Eur J Radiol 2019;119:108634. [Crossref] [PubMed]
- Pinker K, Noebauer-Huhmann IM, Stavrou I, et al. High-field, high-resolution, susceptibility-weighted magnetic resonance imaging: improved image quality by addition of contrast agent and higher field strength in patients with brain tumors. Neuroradiology 2008;50:9-16. [Crossref] [PubMed]
- Park MJ, Kim HS, Jahng GH, et al. Semiquantitative assessment of intratumoral susceptibility signals using non-contrast-enhanced high-field high-resolution susceptibility-weighted imaging in patients with gliomas: comparison with MR perfusion imaging. AJNR Am J Neuroradiol 2009;30:1402-8. [Crossref] [PubMed]