Construction of a cuproptosis-tricarboxylic acid cycle-associated lncRNA model to predict the prognosis of non-small cell lung cancer
Highlight box
Key findings
• Eleven cuproptosis-tricarboxylic acid-related long non-coding RNAs could serve as an independent factor to predict the prognosis of patients with non-small cell lung cancer (NSCLC) effectively.
What is known and what is new?
• Cuproptosis is a novel programmed cell death pathway that participates in tumor metabolism and immune response.
• The high-risk group of NSCLC had better response to immunotherapy than the low-risk group.
What is the implication, and what should change now?
• This is a new theoretical basis for understanding the molecular mechanism involved in the occurrence of NSCLC, which could lead to individualized treatments and prognostic assessments for patients suffering from the disease.
Introduction
The main pathological types of non-small cell lung cancer (NSCLC) are lung squamous cell carcinoma (LUSC) and lung adenocarcinoma (LUAD) (1). In addition, NSCLC makes up 85% of the total lung cancer incidence (2). Currently, cancer-related deaths are dominated by lung cancer, and only 21.7% of patients diagnosed with this disease survive for more than 5 years (3). Therefore, accurate predictions of NSCLC prognosis can help provide different treatment options.
On the basis numerous molecular-level studies using tumor cells, NSCLC treatment has changed from conventional radiotherapy and chemotherapy combined with surgery to molecular-targeted immunotherapy. In patients with epidermal growth factor receptor (EGFR)-mutated NSCLC, tyrosine kinase inhibitors have become the first-line treatment (4-6). These drugs can regulate the tumor microenvironment (TME) to enhance the ability of T cells to kill cancer cells (7), thereby achieving good efficacy. Accordingly, immunotherapy effectiveness in NSCLC patients is closely associated with TME (8). Therefore, studying the TME is beneficial for predicting the responsiveness of patients with NSCLC to immunotherapy.
Recently, a new method of programmed cell death has been reported, and this copper-dependent mechanism is known as cuproptosis. Copper is a trace element required for human biological functions, and an appropriate amount of copper, functioning as a cofactor, is required for metabolic processes in the body, such as superoxide disproportionation, oxygen free radical scavenging, pigmentation, and catecholamine metabolism. When copper ion homeostasis is disrupted, metabolism becomes abnormal. An excess of copper ions promotes the formation of tumors through the regulation of mitochondrial respiration, the immune system, antioxidant defense, and apoptosis (9). Antioxidant-1 stimulates the proliferation of NSCLC cells by transporting copper ions (10), leading to a significant increase in serum copper levels in patients with lung cancer (11). Further, water-soluble copper (II) complexes increase copper ion concentrations in the body and jointly promote lung cancer cell death, along with human copper transporter 1 (CTR1) (12). Copper ion metabolism imbalances are closely linked to lung cancer development and its immune microenvironment. Copper ions can affect the occurrence of LUAD and the TME through lysyl oxidase-like 2 (LOXL2), solute carrier family 31, member 2 (SLC31A2), and superoxide dismutase 3 (SOD3) (13). Further, high concentrations of copper ions in patients with lung cancer were found to promote tumor angiogenesis and metastasis (11,14), whereas Copper ion reduction can increase the infiltration of Immune cell (15) and improve cytotoxicity against tumor cells in the body. The downregulation of amine oxidase copper containing 3 expression in lung cancer cells can reduce the migration of CD4+ T cells to the lung tissue, thus promoting lung cancer progression (16). Studies on copper ion complexes as anticancer agents for the treatment of cancer have also been performed (17,18). Further, glucose restriction therapy can upregulate expression of the copper ion transporter CTR1, thus delaying the progression of NSCLC (19). Jiang et al. also found that copper nanoparticle reagents can inhibit lung cancer cell migration and improve cisplatin resistance by regulating the TME (20). In addition, it has been established that copper ions can serve as prognostic factors for patients with lung cancer. Specifically, studies have shown that copper levels in patients with advanced lung cancer are higher than those in patients with early lung cancer, suggesting that an increase in copper ion levels is related to the risk of lung cancer (21,22).
Cuproptosis is closely associated with the tricarboxylic acid (TCA) cycle, as excessive copper ions can bind to fatty acylation-associated components of TCA to inhibit the TCA cycle. This results in the loss of iron-sulfur (Fe-S)-cluster proteins, which then triggers protein-associated toxic stress and finally accelerates cell death (23). Excess acetyl-CoA in the TCA cycle competitively inhibits dihydrolipoamide s-acetyltransferase (DLAT) activity, resulting in cuproptosis, and these two factors influence each other. Copper ions can also regulate tumor growth by targeting TCA-cycle proteins. Tsvetkov et al. found that copper directly binds lipid acylated components of the TCA cycle, resulting in the aggregation of lipid acylated proteins and the loss of Fe-S-cluster proteins, which then increases toxic protein stress and ultimately accelerates cell death (23). Active TCA circulation is closely associated with lung cancer (24), and a direct relationship exists between TCA cycle gene expression and NSCLC occurrence and prognosis (25-27). Simultaneously, lactic acid participates in NSCLC tumor cell metabolism as a carbon source for the TCA cycle (28). Copper ions as well as the TCA cycle all play a role in NSCLC.
Long non-coding RNAs (lncRNAs) are transcripts of non-coding proteins that are longer than 200 nucleotides (29) and are closely related to proliferation, migration, and prognosis in NSCLC (30,31). Therefore, they have gradually emerged as novel biomarkers for predicting cancer prognosis. For example, lncRNA translation regulatory long non-coding RNA 1 (TRERNA1) can promote the proliferation of NSCLC cells by regulating forkhead box l1 (FOXL1) expression. In patients with this disease, TRERNA1 is linked to a poor prognosis (32). Numerous studies have also found that lncRNAs associated with cuproptosis can be used as prognostic factors for cancers, such as liver cancer, renal clear cell carcinoma, and soft tissue sarcoma (33-35). Cuproptosis-TCA-related lncRNAs are associated with tumor immunotherapeutic response and have an important role in predicting immunotherapeutic response. Binxiang Chu constructed a new risk-prognostic model and found that cuprotosis-associated lncRNAs were involved in the development of sarcoma and assisted in the formation of the tumor immune microenvironment, which could identify the prognosis of sarcoma patients (36). Four cuprotosis-TCA-associated lncRNAs, including GIHCG and AC145343.1, are high-risk lncRNAs in patients with hepatocellular carcinoma, and they are involved in the regulation of immune cell infiltration, and patients with their high expression are more responsive to immunosuppressants (33). AC145343.1, a cuproptosis-TCA-associated lncRNA, is associated with the TP53 mutation, is involved in the immune cell infiltration regulation and predicts the prognosis of patients with hepatocellular carcinoma. Cuproptosis-associated lncRNAs may affect the ability of tumor cells to escape immune surveillance by regulating the expression of immune infiltration-related molecules (37,38). If the expression of lncRNA XIST is downregulated in uterine corpus endometrial carcinoma (UCEC), high levels of XIST have a higher survival rate, and the XIST/miR-125a-5p/CDKN2A regulatory axis may be involved in the progression of UCEC (39). However, there are few studies on the association among cuproptosis, cuproptosis-TCA-related lncRNAs, the tumor immune microenvironment (TIME), and NSCLC prognosis. Therefore, a comprehensive analysis of cuproptosis-TCA-related lncRNAs would be beneficial for evaluating the treatment and prognosis of NSCLC and can provide new biomarkers for individualized immunotherapy of NSCLC.
Cuproptosis-TCA-associated lncRNAs in NSCLC were identified to construct a relatively accurate model to predict patient prognosis. This study was also undertaken to understand the role of cuproptosis-TCA-associated lncRNAs in the tumor immune microenvironment of NSCLC. Thus, this study elucidated the molecular mechanism by which cuproptosis-TCA-related lncRNAs affect NSCLC. And new immunotherapy theories will be developed from this research. We present this article in accordance with the TRIPOD reporting checklist (available at https://tcr.amegroups.com/article/view/10.21037/tcr-24-660/rc).
Methods
Data source processing
We used The Cancer Genome Atlas (TCGA) database (https://portal.gdc.cancer.gov/repository) to download the transcriptome studies and clinical data of 1,149 patients with NSCLC. RNA-seq data were normalized to transcripts per kilobase million values. TCGA database was used to download simple nucleotide variation data. In total, additional 123 patients were excluded for lack of clinical information, and clinical data from 1,026 patients with NSCLC were obtained (Table S1). Nineteen cuproptosis-related motifs (Table S2) were obtained from the document search (23,40-44). At the same time, the gene set enrichment analysis (GSEA) database (https://www.gsea-msigdb.org/gsea/index.jsp) was used to download 18 TCA-related genes (ACO2, CS, DLD, DLST, FH, IDH2, IDH3A, IDH3B, IDH3G, MDH2, OGDH, SDHA, SDHB, SDHC, SDHD, SUCLA2, SUCLG1 and SUCLG2), and after the removal of duplicated genes, 35 cuproptosis-TCA-related genes were obtained. The study was conducted in accordance with the Declaration of Helsinki (as revised in 2013).
Retrieval and identification of cuproptosis-TCA-related lncRNA data
To identify cuproptosis-TCA-related lncRNAs, we made use of pearson’s correlation analysis to evaluate the correlations between cuproptosis-TCA-related genes and lncRNAs using the “Perl” language. We set the criteria as a Pearson correlation coefficient >0.04 and a P value <0.001. Overlapping lncRNAs were identified as cuproptosis-TCA-related.
Construction and validation of cuproptosis-TCA-associated lncRNA signatures
Twenty-four tumor normal samples were excluded from the clinical data, and 1,002 selected patients were placed randomly in training and testing groups (Figure 1). In the training group, univariate Cox regression, least absolute shrinkage and selection operator (LASSO) Cox regression, and multivariate Cox regression analyses (P<0.05) were used to construct the cuproptosis-TCA-related lncRNA signatures. Finally, the multivariate Cox recurrence coefficient (β) was used to calculate the risk score, which was equal to ∑icoefficient(lncRNAi) × expression(lncRNAi). The training, test, and total patients were divided into high- and low-risk groups based on the median risk score. By using the R packages “timeROC” and “survival”, We use time-dependent receiver operating characteristic (time-ROC) curve analysis and Kaplan-Meier survival analysis to compare the overall survival (OS) of the training, trial, and low-risk group from all patients with NSCLC, as well as the clinically graded survival of patients with NSCLC. The signature’s prognostic accuracy was assessed using univariate and multivariate Cox analyses.
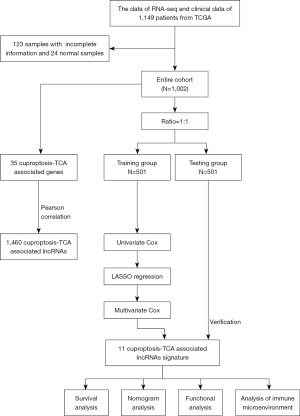
Prediction of nomogram structure
We then used the “rms” R package to establish a hybrid nomogram model that combined the cuproptosis-TCA-associated lncRNA risk score with clinicopathological features to predict 1-, 3-, and 5-year OS in patients with NSCLC. A calibration curve and consistency index were then used to determine the predictive capability of the nomogram.
Functional enrichment analysis
According to the risk score, all clinical patients were divided into high- and low-risk groups. The R package “enrichplot”, “org.Hs.eg.db”, “ggplot2” and “clusterProfiler” was used to analyze the Gene Ontology (GO) enrichment analysis comparing the high- and low-risk groups following the criteria |log2fold change (FC)| >2.0, based on a P value <0.01, in addition to Kyoto Encyclopedia of Genes and Genomes (KEGG) enrichment analysis, based on a P value <0.05.
Microenvironment analysis of tumor-free patients with NSCLC in high- and low-risk groups
Twenty-eight types of tumor-infiltrating cell gene sets were downloaded from the TISIDB database (http://cis.hku.hk/TISIDB/download.php). Cell-type Identification By Estimating Relative Subsets Of RNA Transcripts (CIBERSORT), Tumor Immune Estimation Resource (TIMER), Cell type enrichment analysis based on gene expression (Xcell), Quantitative Tissue Infiltrating Immune Estimation by RNA-seq (quanTIseq), Microenvironment Cell Populations-counter (MCP counter), Estimation of the Proportions of Immune and Cancer cells (EPIC), and Single-sample Gene Set Enrichment Analysis (ssGSEA) algorithms were applied to estimate the infiltration level of immune cell populations. For comparison of the differences between high- and low-risk groups in tumor immune cells and immune functions, the ssGSEA algorithm in gene set enrichment analysis (GSVA) was used.
Analysis of genetic variation in high- and low-risk groups
Using nucleotide variation data from patients with NSCLC downloaded from TCGA database, the R package “maftools” was used to evaluate genetic variation in the high- and low-risk groups of patients with NSCLC.
Immunotherapy-related analysis
Tumor mutation burden (TMB) reflects cancer mutations. The Tumor Immune Dysfunction and Exclusion (TIDE) computational framework was used to analyze TMB and TIDE scores in patients with NSCLC to predict the response to immunotherapy in high-risk groups. Waterfall plot showing the relationship between NSCLC patient risk score and TMB. Violin plot showing the relationship between NSCLC patient score and TIDE.
Cell culture
Human bronchial epithelial cell line (BEAS-2B) was purchased from Hunan Starfish Biotechnology Co. Ltd. and these cells were cultured in Starfish matching human bronchial epithelial cell complete medium (item No. TCH-G132). The NSCLC cell line NCI-H1299, which was gifted by the Hunan Key Laboratory of Chinese Medicine Founder’s Research on Conversion Medicine, was cultured in RPMI-1640 medium supplemented with 10% fetal calf serum and 1% (penicillin-streptomycin) double antiseptic sterilizing solution in RPMI-1640 medium at 37 ℃ under 5% CO2. The sample was incubated in RPMI-1640 medium supplemented with 10% fetal bovine serum and 1% (penicillin-streptomycin) double antiseptic solution at 37 ℃ under 5% CO2.
Total RNA extraction and real-time quantitative polymerase chain reaction (qRT-PCR)
To assess the expression level of AC004943.2 and LINC00996 between normal and cancer cells, total RNA was isolated from cells using TRIzol reagent. The complementary DNA (cDNA) was synthesized by reverse transcription using NovoScript® Plus All-in-one 1st Strand cDNA Synthesis SuperMix (gDNA Purge) (Lot No. 05305501). Then qRT-PCR was performed on SYBR Premix Ex Taq (novoprotein). Relative expression of lncRNA was normalized to the internal reference of GAPDH.
LINC00996 forward: 5'-CTCCCATCTTTTCTGCCGGT-3'; reverse: 5'-GATTGTGTCGGAAGCGGTTG-3'. AC004943.2 forward: 5'-CACTTCTGCAGGAACACCGA-3'; reverse: 5'-GTGGAGACTGAATGGCCCTC-3'. GAPDH forward: 5'-GTGAAGGTCGGAGTCAACGG-3'; reverse: 5'-GCAACAATATCCACTTTACCAGAGT-3'.
Statistical analysis
R software was used for all statistical analyses (v4.2.1). The validity of ROC curve analysis and Kaplan-Meier survival analysis was used to predict survival outcomes. The association between high- and low-risk groups of NSCLC and survival outcomes, as well as other clinical characteristics, was investigated using Cox proportional modeling. If the level of statistical significance was not clearly indicated, P<0.05 was used.
Results
After enrolling 1,002 patients, they were divided into a training group and a test group. In the training group, the cuproptosis-TCA-related lncRNAs prognostic model was constructed. Each patient’s risk score was calculated based on this prognostic model. The OS of high-low risk patients in the training group, the test group, and all patient groups was finally compared (Figure 1).
Construction of cuproptosis-TCA-associated lncRNA signature for NSCLC
Pearson correlation coefficients were calculated comparing lncRNA expression and cuproptosis-TCA-related gene expression in NSCLC, and corFilter =0.4 and pvalueFilter =0.001 were used as the criteria to obtain correlation results, as shown in Figure 2A. As a result, 28 genes, including ATP7A, FDX1, and GCSH, were significantly correlated with 1,460 copper-associated death and TCA-related lncRNAs. First, 62 cuproptosis-TCA-related lncRNAs associated with the prognosis of patients with NSCLC were identified using univariate Cox regression analysis in the training group from TCGA (P<0.05). LASSO regression analysis with 1,000 iterations was then adopted. Twenty-eight cuproptosis-TCA-associated lncRNAs were selected for prognostic assessments of the patients (Figure 2B,2C). Finally, 11 cuproptosis-TCA-associated lncRNAs were identified via multivariate Cox regression analysis. LncRNA risk scores were calculated as follows (Figure 2D): risk rating = AC022165.1 ´ 0.47718 + AC004943.2 ´ 0.52590 + AC009226.1 ´ 0.68043 − LINC00996 ´ 0.29947 − AC105389.2 ´ 0.38091 − AC025178.1 ´ 0.27865 − AC092807.3 ´ 0.79728 − AL122020.2 ´ 0.89487 − SNHG30 ´ 0.21668 − LINC02635 ´ 0.21324 − AP003032.1 ´ 0.21425. Analysis revealed that 35 cuproptosis-TCA-related genes were significantly correlated with 11 cuproptosis-TCA-related lncRNAs (Figure 2E). For example, the expression of AC004943.2 and GCSH were positively correlated.
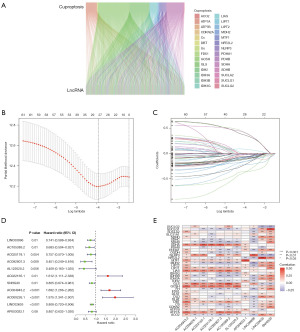
Validation of cuproptosis-TCA-associated lncRNAs in NSCLC
To verify the accuracy of the cuproptosis-TCA-related lncRNA labels, the aforementioned risk-scoring formula was used for the risk assessment based on all NSCLC patients in TCGA cohort, TCGA training, and TCGA test datasets. The risk states and lncRNA expression levels of TCGA queue (Figure 3A), training (Figure 3B), and test datasets (Figure 3C) were obtained. According to a Kaplan-Meier analysis, patients with low-risk scores were more likely to survive longer than those with high-risk scores in TCGA cohort (P<0.001), TCGA training (P<0.001), and TCGA test (P=0.01) datasets. This indicated that the prognosis of patients with high-risk NSCLC was worse than that of patients with low-risk NSCLC (Figure 3D-3F). Time-ROC analysis further showed that this feature had strong predictive ability; the AUCs of the cohort from TCGA were 0.682, 0.649, and 0.611, respectively, the AUCs of the training cohort were 0.710, 0.732, and 0.737, respectively, and the AUCs of the test cohort were 0.655, 0.569, and 0.483, respectively (Figure 3G-3I). Finally, we found that this feature might be an independent prognostic factor for patients with NSCLC. Clinical features and cuproptosis-TCA-associated lncRNA risk scores were also evaluated with single-factor and multi-factor Cox regression models. Univariate Cox regression analysis suggested that there was a significant impact of this factor on the prognosis of NSCLC patients [total TCGA dataset: hazard ratio (HR) =1.115, 95% confidence interval (CI): 1.071–1.160, P<0.001; Figure 4A]. Multivariate Cox regression analysis further showed that the cuproptosis-TCA-related lncRNA risk score was an independent predictor of OS for patients with NSCLC [total dataset: HR =1.121, 95% CI: 1.074–1.170; P<0.001; Figure 4B]. All genes involved in the distinction between the high- and low-risk groups (Figure 4C), 19 cuproptosis-TCA-related genes (Figure 4D), 1,460 cuproptosis-TCA-associated lncRNAs (Figure 4E), and 11 cuproptosis-TCA-associated lncRNA labels (Figure 4F) were used for principal component analysis. The results showed a wide range of gene expression changes between high- and low-risk patients.
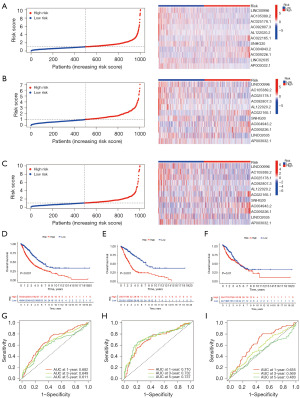
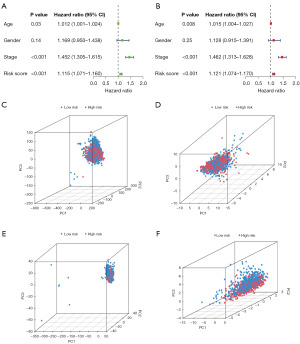
Establishment of a nomogram survival-prediction model
Because the cuproptosis-TCA-related lncRNA risk score could not intuitively predict OS in patients with clinical NSCLC, we combined the cuproptosis-TCA-related lncRNA risk score with clinicopathological features. A hybrid nomogram model was established to predict the 1-, 3-, and 5-year OS rates (Figure 5A). Predictors included risk score, age, and tumor grade. Simultaneously, calibration diagram indicated that the proposed model performed similarly to the ideal model (Figure 5B).
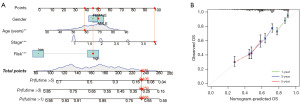
Functional enrichment analysis
To further investigate the mechanism underlying the effects of cuproptosis-TCA-related lncRNAs in NSCLC, GO (Figure S1) and KEGG (Figure S2) enrichment analyses were performed on differential genes in the high- and low-risk groups of patients with NSCLC. The cuproptosis-TCA-related lncRNA model was not only related to copper ion metabolism and transport but also to cellular and humoral immune responses, neuroactive ligand-receptor interactions, the IL-17 signaling pathway, and tryptophan metabolism.
Analysis of tumor immune microenvironment and immunotherapy response in patients with high- and low-risk NSCLC
In order to determine whether TME differs between patients with low- and high-risk NSCLC, 28 tumor-infiltrating immune cell gene sets were downloaded, and correlation analysis was performed using ssGSEA based on the c software package. The results showed that type 2 helper T cells, CD4+ T cells, natural killer T cells, CD56 (bright) natural killer cells, effector memory CD4 T cells, neutrophils, memory CD8+ T cells, and CD8+ T cells contributed a greater percentage to immune microenvironment in patients in the high-risk group. However, immature dendritic cells, B cells, mast cells, and eosinophils accounted for a lower proportion in patients in the high-risk group (Figure 6A). ssGSEA was also used to analyze differences in immune functions between the high- and low-risk groups, which showed that CCR, parainflammation, and MHC class I were further enriched in the high-risk group (Figure 6B). Changes in the distribution of somatic mutations in high- and low-risk patients were also analyzed. We found that 462 (95.06%) of the 486 high-risk samples had mutations, and the three genes with the highest mutation rates were TP53, TTN, and MUC16 (Figure 6C). Among the 486 low-risk samples, 440 (90.53%) had mutations, and the top three genes with the highest mutation rates were TP53, TTN, and MUC16 (Figure 6D). Mutation rates of TP53, TTN, and MUC16 in the high-risk group were higher than those in the low-risk group.
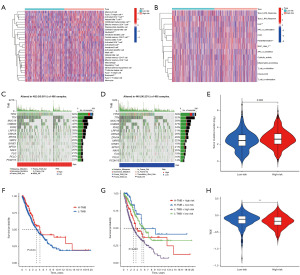
The TMB can be used as an important indicator of the therapeutic effects of immune checkpoint blockade (ICB). An analysis of the mutation data showed that the TMB was higher in high-risk patients than that in low-risk patients, suggesting that immunotherapy may be better suited for high-risk patients (Figure 6E). Overall, patients with a higher TMB had higher survival rates (Figure 6F). Further comparisons were performed between the groups with high- and low-risk TMBs. Results showed that patients in the low-risk group with a high TMB had the highest survival rate, whereas those in the high-risk group with a low TMB had the lowest (Figure 6G). TIDE is a computational framework that simulates two major mechanisms of tumor immune escape and provides predictive outcomes related to immunotherapy. To further demonstrate that this risk score is predictive of immunotherapy outcomes, TIDE scores were calculated. We found that TIDE scores were inversely associated with risk scores, suggesting better immunotherapy outcomes in the high-risk groups (Figure 6H).
Expression levels of AC004943.2 and LINC00996 in BEAS-2B, NCI-H520, and NCI-H1299
The expression of high-risk lncRNA AC004943.2 and low-risk lncRNA LINC00996 was examined in lung normal epithelial cells (BEAS-2B) and NSCLC cells (NCI-H520 and NCI-H1299). The results of the qRT-PCR analysis demonstrated that AC004943.2 is markedly upregulated in the NCI-H520 and NCI-H1299 cell lines, whereas its expression is significantly downregulated in the BEAS-2B cell line (P<0.05) (Figure 7A). In contrast, LINC00996 displays elevated expression levels in BEAS-2B, with downregulation observed in both NCI-H520 and NCI-H1299 (P<0.05, P<0.001) (Figure 7B).
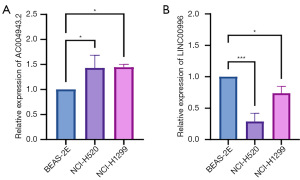
Discussion
In this study, 11 lncRNAs associated with cuproptosis and TCA in NSCLC were identified, including 3 high-risk lncRNAs and 8 low-risk lncRNAs. Further, a difference was noted in their expression between the high- and low-risk NSCLC groups, and the high-risk group had a short OS. By constructing an ROC curve and nomogram model, we found that these parameters could be used as independent predictors of NSCLC prognostic risk. In addition, by analyzing the differences in biological functions, the TME, and response to immunotherapy between the high- and low-risk groups, there was a better response to immunotherapy in the high-risk group of NSCLC than in the low-risk group.
Twenty-eight cuproptosis- and TCA-related lncRNA genes that were significantly correlated with OS in NSCLC were identified. These 28 genes included genes related to cuproptosis regulation, such as GLS, DLAT, and LIPT1, which were discovered by Tsvetkov et al. (23). The GLS gene encodes glutaminase, which helps the essential amino acid glutamine participate in the TCA cycle. Further, GLS promotes the proliferation of NSCLC cells through an oxidative stress response (45), and the inhibition of GLS expression can improve the survival of patients with prostate cancer (39). High expression of the DLAT glycolysis-associated gene is associated with the tumor volume and poor prognosis in patients with NSCLC (46). Meanwhile, LIPT1 participates in fatty acylation during the TCA cycle and affects the occurrence and prognosis of lung cancer (47). These genes are associated with the development and prognosis of lung cancer.
Several studies have found that lncRNAs, as important regulatory factors in biological processes, can regulate gene expression to contribute to tumor development and occurrence (48-50). A newly identified NSCLC-associated lncRNA, AL139294.1, enters the cell via extracellular vesicle (EV) transport and activates the Wnt and NF-κB2 pathways to promote cancer cell proliferation, differentiation, and migration (51). ACOXL-AS1, a cuproptosis-related lncRNA in the prognostic model of endometrial cancer, contributes to the proliferation of cancer cells by regulating the expression level of the miRror-421 to the cuproptosis related gene MTF1 expression level (52). Cuproptosis-associated lncRNA LINC01614 upregulates the expression level of SLC31A1, which regulates the degree of immune cell infiltration in breast cancer and predicts the prognosis of breast cancer patients (53). However, few studies have investigated the role of TCA-related lncRNAs in NSCLC. Using correlation analysis based on TCGA database, we found 1,460 cuproptosis-TCA-associated lncRNAs and created a prognostic model using these markers. In this study, we identified 11 TCA-associated lncRNA signatures associated with cuproptosis. AC004943.2, AC022165.1, and AC009226.1 were determined to be high-risk lncRNAs, whereas LINC02635, SNHG30, and LINC00996 were low-risk lncRNAs. AC004943.2 affects the tumor immunosuppressive microenvironment by activating the PTK2/PI3K pathway through sponge miR-135a-5p upregulation of protein tyrosine kinase 2 (PTK2) expression (54). Meanwhile AC004943.2 is a predictor in the cuproptosis-associated lncRNA model in head and neck squamous cell carcinoma (HNSCC) and osteosarcoma (55,56). AC004943.2 has been found to be upregulated in laryngeal squamous cell carcinoma, which promotes tumorigenesis, suggesting it as a potential biomarker for laryngeal squamous cell carcinoma (57). And in this study, AC004943.2 was highly expressed in NSCLC cells, verifying that it can be used as a predictor of NSCLC prognosis. LINC00996 is a tumor immune-infiltrating cell-associated lncRNA, which is lowly expressed in HNSCC high-risk patients and correlates with immunotherapy sensitivity (58). LINC00996, as an intermittent hypoxia-associated signal, is lowly expressed in LUAD, and acts as a tumor suppressor to inhibit tumor development and metastasis through multiple biological pathways, predicting the prognosis of LUAD (59,60), and also as a prognostic predictor of bladder, colorectal, and cervical cancers (61-63). Meanwhile, Yan identified LINC00996 as a potential target for NSCLC immunology (64). In this study, LINC00996 was lowly expressed in NSCLC, suggesting that it is a protective lncRNA for NSCLC patients. In addition, AC009226.1 can be used as one of the prognostic predictors for LUAD (65). However, the roles and molecular mechanisms of AC022165.1 and LINC02635 in NSCLC have not been reported. Therefore, in this study, it was determined that this model can be considered an independent prognostic factor for NSCLC and can be used to predict patient therapeutic responses to ICB therapy. Subsequently, a prediction nomogram was generated by combining the risk score of patients with NSCLC with age, sex, and tumor grade to further improve its usefulness and make the risk score easier to use.
Tumor immune microenvironments are influenced by lncRNAs, as demonstrated in several studies (66,67). Accordingly, our study showed that this model is bound up with the tumor immune microenvironment. We also found that the proportions of memory B cells and CD4+ T cells were significantly increased in high-risk patients, suggesting that cuproptosis regulates the development of NSCLC by increasing the infiltration of these immune cells. However, these preliminary findings need to be further verified, both in vivo and in vitro. The high-risk group also exhibited rich immunization-related features, such as CCR, parainflammation, and MHC class I.
The analysis of immune cell infiltration and immune functions in samples alone cannot reflect the effect of ICB treatment on patients with NSCLC, and a comprehensive analysis and evaluation of clinical characteristics is needed. Researchers found that patients with a higher TMB have a higher proportion of neoantigens and therefore respond better to immunotherapy (68-70). Our study showed that the TMB of high-risk patients was higher than that of low-risk patients, indicating a better immunotherapeutic effect in high-risk NSCLC patients. We also found that 462 (95.06%) of the 486 high-risk samples had mutations, and among 486 low-risk samples, 440 (90.53%) had mutations. The top three genes with the highest mutation rates in the two groups were TP53, TTN, and MUC16, and the mutation rates of these three genes in the high-risk group were higher than those in the low-risk group. This provides an indication that a high TP53 mutation burden can be beneficial for immunotherapy. This conclusion is consistent with that of previous studies. Wang et al. showed that a high TP53 mutation rate is conducive to ICB treatment in patients with NSCLC (71). Further, TIDE, a framework for computing two major mechanisms of tumor immune escape, can be used to predict immunotherapeutic responses (72,73). Here, this was applied to demonstrate the predictive power of the immunotherapy risk scores. We found a negative association between TIDE and risk scores, further suggesting that patients at a high risk for NSCLC might have better immunotherapy outcomes.
This prognostic prediction model provides a new biological predictive marker for NSCLC patient prognosis as well as responsiveness to immunotherapy-cuprotosis-TCA. The model is able to capture complex molecular features associated with tumors and is suitable for NSCLC prognostic assessment and treatment response prediction. These molecular features often go beyond the ability of traditional gene expression profiling to identify potential therapeutic targets. The biggest drawback of this model is that it is not yet possible to accurately predict the responsiveness of individual patients to specific different targeted drugs and chemotherapeutic agents. Meanwhile, how to effectively translate these models into clinical diagnostic or therapeutic tools still requires a lot of validation studies, which is also the biggest problem facing this model.
We must recognize the limitations of the current study. We lack in-depth mechanistic exploration of the key lncRNAs associated with cuprotosis-TCA in prognostic models. Further hard work on this topic is being carried out by our team.
Conclusions
We found that 11 cuproptosis-TCA-related lncRNAs could effectively predict the prognosis of patients with NSCLC, as independent factors, and the demonstrated on the TME of NSCLC. Simultaneously, they can predict the immunotherapy response. In summary, this is a new theoretical basis for understanding the molecular mechanism involved in the occurrence of NSCLC, which could lead to individualized treatments and prognostic assessments for patients suffering from the disease.
Acknowledgments
Funding: This work was supported by
Footnote
Reporting Checklist: The authors have completed the TRIPOD reporting checklist. Available at https://tcr.amegroups.com/article/view/10.21037/tcr-24-660/rc
Data Sharing Statement: Available at https://tcr.amegroups.com/article/view/10.21037/tcr-24-660/dss
Peer Review File: Available at https://tcr.amegroups.com/article/view/10.21037/tcr-24-660/prf
Conflicts of Interest: All authors have completed the ICMJE uniform disclosure form (available at https://tcr.amegroups.com/article/view/10.21037/tcr-24-660/coif). The authors have no conflicts of interest to declare.
Ethical Statement: The authors are accountable for all aspects of the work in ensuring that questions related to the accuracy or integrity of any part of the work are appropriately investigated and resolved. The study was conducted in accordance with the Declaration of Helsinki (as revised in 2013).
Open Access Statement: This is an Open Access article distributed in accordance with the Creative Commons Attribution-NonCommercial-NoDerivs 4.0 International License (CC BY-NC-ND 4.0), which permits the non-commercial replication and distribution of the article with the strict proviso that no changes or edits are made and the original work is properly cited (including links to both the formal publication through the relevant DOI and the license). See: https://creativecommons.org/licenses/by-nc-nd/4.0/.
References
- Zhang L, Zhang Y, Wang C, et al. Integrated single-cell RNA sequencing analysis reveals distinct cellular and transcriptional modules associated with survival in lung cancer. Signal Transduct Target Ther 2022;7:9. [Crossref] [PubMed]
- Lim JU, Yeo CD. Update on adjuvant therapy in completely resected NSCLC patients. Thorac Cancer 2022;13:277-83. [Crossref] [PubMed]
- Ettinger DS, Wood DE, Aisner DL, et al. Non-Small Cell Lung Cancer, Version 3.2022, NCCN Clinical Practice Guidelines in Oncology. J Natl Compr Canc Netw 2022;20:497-530. [Crossref] [PubMed]
- Remon J, Hendriks LE, Cabrera C, et al. Immunotherapy for oncogenic-driven advanced non-small cell lung cancers: Is the time ripe for a change? Cancer Treat Rev 2018;71:47-58. [Crossref] [PubMed]
- Hanna N, Johnson D, Temin S, et al. Systemic Therapy for Stage IV Non-Small-Cell Lung Cancer: American Society of Clinical Oncology Clinical Practice Guideline Update. J Clin Oncol 2017;35:3484-515. [Crossref] [PubMed]
- Ettinger DS, Wood DE, Aisner DL, et al. NCCN Guidelines Insights: Non-Small Cell Lung Cancer, Version 2.2021. J Natl Compr Canc Netw 2021;19:254-66. [Crossref] [PubMed]
- Jia Y, Li X, Jiang T, et al. EGFR-targeted therapy alters the tumor microenvironment in EGFR-driven lung tumors: Implications for combination therapies. Int J Cancer 2019;145:1432-44. [Crossref] [PubMed]
- Overacre-Delgoffe AE, Chikina M, Dadey RE, et al. Interferon-γ Drives T(reg) Fragility to Promote Anti-tumor Immunity. Cell 2017;169:1130-1141.e11. [Crossref] [PubMed]
- Shanbhag VC, Gudekar N, Jasmer K, et al. Copper metabolism as a unique vulnerability in cancer. Biochim Biophys Acta Mol Cell Res 2021;1868:118893. [Crossref] [PubMed]
- Cai H, Peng F. Knockdown of copper chaperone antioxidant-1 by RNA interference inhibits copper-stimulated proliferation of non-small cell lung carcinoma cells. Oncol Rep 2013;30:269-75. [Crossref] [PubMed]
- Zhang X, Yang Q. Association between serum copper levels and lung cancer risk: A meta-analysis. J Int Med Res 2018;46:4863-73. [Crossref] [PubMed]
- Terra WDS, Bull ÉS, Morcelli SR, et al. Antitumor activity via apoptotic cell death pathway of water soluble copper(II) complexes: effect of the diamino unit on selectivity against lung cancer NCI-H460 cell line. Biometals 2021;34:661-74. [Crossref] [PubMed]
- Chang W, Li H, Zhong L, et al. Development of a copper metabolism-related gene signature in lung adenocarcinoma. Front Immunol 2022;13:1040668. [Crossref] [PubMed]
- Oliveri V. Selective Targeting of Cancer Cells by Copper Ionophores: An Overview. Front Mol Biosci 2022;9:841814. [Crossref] [PubMed]
- Voli F, Valli E, Lerra L, et al. Intratumoral Copper Modulates PD-L1 Expression and Influences Tumor Immune Evasion. Cancer Res 2020;80:4129-44. [Crossref] [PubMed]
- Chang CY, Wu KL, Chang YY, et al. Amine oxidase, copper containing 3 exerts anti‑mesenchymal transformation and enhances CD4(+) T‑cell recruitment to prolong survival in lung cancer. Oncol Rep 2021;46:203. [Crossref] [PubMed]
- Zhou J, Yu Q, Song J, et al. Photothermally Triggered Copper Payload Release for Cuproptosis-Promoted Cancer Synergistic Therapy. Angew Chem Int Ed Engl 2023;62:e202213922. [Crossref] [PubMed]
- Zhang W, Zhang P, Xu X, et al. Synergy effects of copper ion in doxorubicin-based chelate prodrug for cancer chemo-chemodynamic combination therapy. Drug Deliv 2023;30:2219426. [Crossref] [PubMed]
- Zhang P, Li B, Chen Q, et al. Glucose restriction induces ROS-AMPK-mediated CTR1 expression and increases cisplatin efficiency in NSCLC. Cancer Lett 2022;543:215793. [Crossref] [PubMed]
- Jiang M, Zhang Z, Li W, et al. Developing a Copper(II) Agent Based on His-146 and His-242 Residues of Human Serum Albumin Nanoparticles: Integration To Overcome Cisplatin Resistance and Inhibit the Metastasis of Nonsmall Cell Lung Cancer. J Med Chem 2022;65:9447-58. [Crossref] [PubMed]
- Zhang L, Shao J, Tan SW, et al. Association between serum copper/zinc ratio and lung cancer: A systematic review with meta-analysis. J Trace Elem Med Biol 2022;74:127061. [Crossref] [PubMed]
- Yang M, Wu X, Hu J, et al. COMMD10 inhibits HIF1α/CP loop to enhance ferroptosis and radiosensitivity by disrupting Cu-Fe balance in hepatocellular carcinoma. J Hepatol 2022;76:1138-50. [Crossref] [PubMed]
- Tsvetkov P, Coy S, Petrova B, et al. Copper induces cell death by targeting lipoylated TCA cycle proteins. Science 2022;375:1254-61. [Crossref] [PubMed]
- Kikuchi N, Soga T, Nomura M, et al. Comparison of the ischemic and non-ischemic lung cancer metabolome reveals hyper activity of the TCA cycle and autophagy. Biochem Biophys Res Commun 2020;530:285-91. [Crossref] [PubMed]
- Geeraerts X, Fernández-Garcia J, Hartmann FJ, et al. Macrophages are metabolically heterogeneous within the tumor microenvironment. Cell Rep 2021;37:110171. [Crossref] [PubMed]
- Zhao D, Mu HJ, Shi HB, et al. Identification of therapeutic targets and mechanisms of tumorigenesis in non-small cell lung cancer using multiple-microarray analysis. Medicine (Baltimore) 2020;99:e22815. [Crossref] [PubMed]
- Guo X, Li D, Wu Y, et al. Genetic variants in genes of tricarboxylic acid cycle key enzymes are associated with prognosis of patients with non-small cell lung cancer. Lung Cancer 2015;87:162-8. [Crossref] [PubMed]
- Faubert B, Li KY, Cai L, et al. Lactate Metabolism in Human Lung Tumors. Cell 2017;171:358-371.e9. [Crossref] [PubMed]
- Bridges MC, Daulagala AC, Kourtidis A. LNCcation: lncRNA localization and function. J Cell Biol 2021;220:e202009045. [Crossref] [PubMed]
- Hua Q, Mi B, Xu F, et al. Hypoxia-induced lncRNA-AC020978 promotes proliferation and glycolytic metabolism of non-small cell lung cancer by regulating PKM2/HIF-1α axis. Theranostics 2020;10:4762-78. [Crossref] [PubMed]
- He Y, Jiang X, Duan L, et al. LncRNA PKMYT1AR promotes cancer stem cell maintenance in non-small cell lung cancer via activating Wnt signaling pathway. Mol Cancer 2021;20:156. [Crossref] [PubMed]
- Luo DB, Lv HB, Sun XH, et al. LncRNA TRERNA1 promotes malignant progression of NSCLC through targeting FOXL1. Eur Rev Med Pharmacol Sci 2020;24:1233-42. [PubMed]
- Zhang G, Sun J, Zhang X. A novel Cuproptosis-related LncRNA signature to predict prognosis in hepatocellular carcinoma. Sci Rep 2022;12:11325. [Crossref] [PubMed]
- Xu S, Liu D, Chang T, et al. Cuproptosis-Associated lncRNA Establishes New Prognostic Profile and Predicts Immunotherapy Response in Clear Cell Renal Cell Carcinoma. Front Genet 2022;13:938259. [Crossref] [PubMed]
- Han J, Hu Y, Liu S, et al. A Newly Established Cuproptosis-Associated Long Non-Coding RNA Signature for Predicting Prognosis and Indicating Immune Microenvironment Features in Soft Tissue Sarcoma. J Oncol 2022;2022:8489387. [Crossref] [PubMed]
- Chu B, Zheng H, Zheng X, et al. Cuproptosis-associated lncRNAs discern prognosis and immune microenvironment in sarcoma victims. Front Cell Dev Biol 2022;10:989882. [Crossref] [PubMed]
- Wu J, Ren X, Wang N, et al. A Mutation-Related Long Noncoding RNA Signature of Genome Instability Predicts Immune Infiltration and Hepatocellular Carcinoma Prognosis. Front Genet 2021;12:779554. [Crossref] [PubMed]
- Huang DP, Liao MM, Tong JJ, et al. Construction of a genome instability-derived lncRNA-based risk scoring system for the prognosis of hepatocellular carcinoma. Aging (Albany NY) 2021;13:24621-39. [Crossref] [PubMed]
- Chen Y. Identification and Validation of Cuproptosis-Related Prognostic Signature and Associated Regulatory Axis in Uterine Corpus Endometrial Carcinoma. Front Genet 2022;13:912037. [Crossref] [PubMed]
- Polishchuk EV, Merolla A, Lichtmannegger J, et al. Activation of Autophagy, Observed in Liver Tissues From Patients With Wilson Disease and From ATP7B-Deficient Animals, Protects Hepatocytes From Copper-Induced Apoptosis. Gastroenterology 2019;156:1173-1189.e5. [Crossref] [PubMed]
- Aubert L, Nandagopal N, Steinhart Z, et al. Copper bioavailability is a KRAS-specific vulnerability in colorectal cancer. Nat Commun 2020;11:3701. [Crossref] [PubMed]
- Kahlson MA, Dixon SJ. Copper-induced cell death. Science 2022;375:1231-2. [Crossref] [PubMed]
- Ren X, Li Y, Zhou Y, et al. Overcoming the compensatory elevation of NRF2 renders hepatocellular carcinoma cells more vulnerable to disulfiram/copper-induced ferroptosis. Redox Biol 2021;46:102122. [Crossref] [PubMed]
- Dong J, Wang X, Xu C, et al. Inhibiting NLRP3 inflammasome activation prevents copper-induced neuropathology in a murine model of Wilson's disease. Cell Death Dis 2021;12:87. [Crossref] [PubMed]
- Ulanet DB, Couto K, Jha A, et al. Mesenchymal phenotype predisposes lung cancer cells to impaired proliferation and redox stress in response to glutaminase inhibition. PLoS One 2014;9:e115144. [Crossref] [PubMed]
- Chen Q, Wang Y, Yang L, et al. PM2.5 promotes NSCLC carcinogenesis through translationally and transcriptionally activating DLAT-mediated glycolysis reprograming. J Exp Clin Cancer Res 2022;41:229. [Crossref] [PubMed]
- Liu Y, Luo G, Yan Y, et al. A pan-cancer analysis of copper homeostasis-related gene lipoyltransferase 1: Its potential biological functions and prognosis values. Front Genet 2022;13:1038174. [Crossref] [PubMed]
- Jiang S, Cheng SJ, Ren LC, et al. An expanded landscape of human long noncoding RNA. Nucleic Acids Res 2019;47:7842-56. [Crossref] [PubMed]
- Wang G, Zhang ZJ, Jian WG, et al. Novel long noncoding RNA OTUD6B-AS1 indicates poor prognosis and inhibits clear cell renal cell carcinoma proliferation via the Wnt/β-catenin signaling pathway. Mol Cancer 2019;18:15. [Crossref] [PubMed]
- Zhuo W, Liu Y, Li S, et al. Long Noncoding RNA GMAN, Up-regulated in Gastric Cancer Tissues, Is Associated With Metastasis in Patients and Promotes Translation of Ephrin A1 by Competitively Binding GMAN-AS. Gastroenterology 2019;156:676-691.e11. [Crossref] [PubMed]
- Ma X, Chen Z, Chen W, et al. LncRNA AL139294.1 can be transported by extracellular vesicles to promote the oncogenic behaviour of recipient cells through activation of the Wnt and NF-κB2 pathways in non-small-cell lung cancer. J Exp Clin Cancer Res 2024;43:20. [Crossref] [PubMed]
- Wu H, Lin R, Hong L. Two lncRNA signatures with cuproptosis as a novel prognostic model and clinicopathological value for endometrioid endometrial adenocarcinoma. Aging (Albany NY) 2023;15:14242-62. [Crossref] [PubMed]
- Wu JH, Cheng TC, Zhu B, et al. Identification of cuproptosis-related gene SLC31A1 and upstream LncRNA-miRNA regulatory axis in breast cancer. Sci Rep 2023;13:18390. [Crossref] [PubMed]
- Zhu X, Dong W, Zhang M. IncRNA AC004943.2 regulates miR-135a-5p and PTK2/P13K axis to promote laryngeal squamous cell carcinoma progression. J Cell Commun Signal 2024;18:e12016. [Crossref] [PubMed]
- Li YJ, Li HY, Zhang Q, et al. The prognostic value and immune landscape of a cuproptosis-related lncRNA signature in head and neck squamous cell carcinoma. Front Genet 2022;13:942785. [Crossref] [PubMed]
- Jiang J, Chu D, Lai X, et al. The Cuproptosis-Related Long Noncoding RNA Signature Predicts Prognosis and Tumour Immune Analysis in Osteosarcoma. Comput Math Methods Med 2022;2022:6314182. [Crossref] [PubMed]
- Jing Z, Guo S, Zhang P, et al. LncRNA-Associated ceRNA Network Reveals Novel Potential Biomarkers of Laryngeal Squamous Cell Carcinoma. Technol Cancer Res Treat 2020;19:1533033820985787. [Crossref] [PubMed]
- Cai Z, Tang B, Chen L, et al. Mast cell marker gene signature in head and neck squamous cell carcinoma. BMC Cancer 2022;22:577. [Crossref] [PubMed]
- Shen Z, Li X, Hu Z, et al. Linc00996 is a favorable prognostic factor in LUAD: Results from bioinformatics analysis and experimental validation. Front Genet 2022;13:932973. [Crossref] [PubMed]
- Liu P, Zhou L, Chen H, et al. Identification of a novel intermittent hypoxia-related prognostic lncRNA signature and the ceRNA of lncRNA GSEC/miR-873-3p/EGLN3 regulatory axis in lung adenocarcinoma. PeerJ 2023;11:e16242. [Crossref] [PubMed]
- Lin Z, Zou J, Sui X, et al. Necroptosis-related lncRNA signature predicts prognosis and immune response for cervical squamous cell carcinoma and endocervical adenocarcinomas. Sci Rep 2022;12:16285. [Crossref] [PubMed]
- Luan L, Dai Y, Shen T, et al. Development of a novel hypoxia-immune-related LncRNA risk signature for predicting the prognosis and immunotherapy response of colorectal cancer. Front Immunol 2022;13:951455. [Crossref] [PubMed]
- Bai Y, Zhang Q, Liu F, et al. A novel cuproptosis-related lncRNA signature predicts the prognosis and immune landscape in bladder cancer. Front Immunol 2022;13:1027449. [Crossref] [PubMed]
- Yan T, Ma G, Wang K, et al. The Immune Heterogeneity Between Pulmonary Adenocarcinoma and Squamous Cell Carcinoma: A Comprehensive Analysis Based on lncRNA Model. Front Immunol 2021;12:547333. [Crossref] [PubMed]
- Sun X, Song J, Lu C, et al. Characterization of cuproptosis-related lncRNA landscape for predicting the prognosis and aiding immunotherapy in lung adenocarcinoma patients. Am J Cancer Res 2023;13:778-801. [PubMed]
- Huang Q, Lin Y, Chen C, et al. Immune-Related LncRNAs Affect the Prognosis of Osteosarcoma, Which Are Related to the Tumor Immune Microenvironment. Front Cell Dev Biol 2021;9:731311. [Crossref] [PubMed]
- Qin M, Ma Y, Wang Z, et al. Using immune-related lncRNAs to construct novel biomarkers and investigate the immune landscape of breast cancer. Transl Cancer Res 2021;10:2991-3003. [Crossref] [PubMed]
- Zheng Y, Yao M, Yang Y. Higher Tumor Mutation Burden Was a Predictor for Better Outcome for NSCLC Patients Treated with PD-1 Antibodies: A Systematic Review and Meta-analysis. SLAS Technol 2021;26:605-14. [Crossref] [PubMed]
- Li Y, Ma Y, Wu Z, et al. Tumor Mutational Burden Predicting the Efficacy of Immune Checkpoint Inhibitors in Colorectal Cancer: A Systematic Review and Meta-Analysis. Front Immunol 2021;12:751407. [Crossref] [PubMed]
- Deng H, Zhao Y, Cai X, et al. PD-L1 expression and Tumor mutation burden as Pathological response biomarkers of Neoadjuvant immunotherapy for Early-stage Non-small cell lung cancer: A systematic review and meta-analysis. Crit Rev Oncol Hematol 2022;170:103582. [Crossref] [PubMed]
- Wang S, Jiang M, Yang Z, et al. The role of distinct co-mutation patterns with TP53 mutation in immunotherapy for NSCLC. Genes Dis 2020;9:245-51. [Crossref] [PubMed]
- Wu J, Li L, Zhang H, et al. A risk model developed based on tumor microenvironment predicts overall survival and associates with tumor immunity of patients with lung adenocarcinoma. Oncogene 2021;40:4413-24. [Crossref] [PubMed]
- Chen X, Xu R, He D, et al. CD8(+) T effector and immune checkpoint signatures predict prognosis and responsiveness to immunotherapy in bladder cancer. Oncogene 2021;40:6223-34. [Crossref] [PubMed]