Machine learning-based pan-cancer study of classification and mechanism of BRAF inhibitor resistance
Highlight box
Key findings
• AOX1 is first reported in our study to have a vital role in V-raf murine sarcoma viral oncogene homolog B1 (BRAF) inhibitor (BRAFi) metabolism and resistance. Further, we found higher expression of OXTR, H2AC13, TBX2 and lower expression of SLC2A4, which were independent risk factors for BRAFi resistance and were associated with poor prognosis.
What is known and what is new?
• BRAFi therapy resistance affects approximately 15% of cancer patients, leading to disease recurrence and poor prognosis. A reliable way to predict drug response to different inhibitors is needed in order to achieve true individualization of therapy.
• We established a gene-expression model using machine learning methods, consisting of 37 variables based on RNA-seq database, which was externally validated and could be used to predict BRAFi resistance.
What is the implication, and what should change now?
• Machine learning (ML) methods may be useful in predicting BRAF resistance, and the differential expression genes we identified in this study based on the methods may help us to better understand BRAF resistance and improve the prognosis of cancer patients.
Introduction
The V-raf murine sarcoma viral oncogene homolog B1 (BRAF) gene, which encodes BRAF kinase protein, has been identified as an oncogene and potential therapeutic target since 2002 (1). It belongs to the RAF family (ARAF, BRAF, and CRAF/Raf-1) which controls the duration and amplitude of MAPK signaling. Among RAF family members, BRAF has the highest mutation propensity. The high rate of mutations in cancer has led to several efforts for the development of inhibitors (2). In human cancers, BRAF mutations, mostly BRAFV600E, mainly occur in 40–60% melanoma, 45% thyroid cancer, 8–12% colorectal carcinoma, and 1–5% non-small cell lung cancer (3-6).
BRAFV600 leads to continuous activation of BRAF and downstream of MEK and ERK (7,8). MAPK pathway plays a crucial role in regulating cell growth, proliferation, and survival. MAPK pathway-targeted inhibitors are the main therapy for BRAFV600 mutant tumors (9). Currently, many BRAF inhibitors (BRAFi) such as vemurafenib, dabrafenib, and encorafenib have been approved by the US Food and Drug Administration (FDA) for clinical use. Unfortunately, approximately 50% of patients suffered from disease progression within 6–7 months of initiating treatment with a single BRAF inhibitor (10). BRAFi in combination with MEK inhibitors, which include trametinib, binimetinib, and cobimetinib completely blocks MAPK signaling and increases metastasis-free survival (MFS) and progression-free survival (PFS) compared to BRAF inhibitor monotherapy since the simultaneous blockade of BRAF and MEK protein in MAPK pathway suppresses and delays the occurrence of drug resistance (11,12). However, drug resistance still occurs in approximately 15% of patients during the treatment processes, which leads to disease recurrence or deterioration (13). Experimental data for melanoma in mice show that under continuous treatment with BRAFi, the pro-cancer macrophages and chemokine C-C motif chemokine ligand 2 (CCL2) initially decrease but eventually increase to above the original level. In contrast, the anticancer T cells continuously decrease (14). Therefore, a combination of anti-CCL2 or immune checkpoint inhibitors is becoming increasingly prevalent thereby eliminating or reducing BRAFi-acquired resistance. CCL2 is a chemokine involved in recruiting monocytes and macrophages to the tumor microenvironment, where they can promote tumor growth and resistance to therapies. Combining BRAFi with anti-CCL2 has been effective in melanoma by attenuating the immunosuppressive microenvironment and enhancing the direct anti-tumor effects of BRAFi (15). Another study has demonstrated that the combination of BRAFi with anti-PD-1 significantly improved PFS and overall survival (OS) in patients with melanoma compared to BRAFi alone (16). Cytotoxic T-lymphocyte-associated protein 4 (CTLA-4) is another immune checkpoint receptor that inhibits early stages of T-cell activation. Similar to PD-1, CTLA-4 prevents the immune system from attacking tumor cells effectively. By combining BRAFi with anti-CTLA-4 therapy, the immune system can be activated to recognize and destroy cancer cells. Although the combination can lead to increased immune-related adverse events, it has been associated with improved survival outcomes in patients with advanced disease, suggesting a synergistic effect between the two therapies (17). Therefore, a reliable way to predict drug response to different inhibitors is needed to achieve true individualization of therapy (18).
Machine learning (ML) is a subset of artificial intelligence and has been widely employed in drug screening, drug toxicity prediction, quantitative structure-activity relationship prediction, and anti-cancer synergy score prediction (19). Additionally, along with the advance of medical technology, big data such as genomic profiles of cell lines or patient samples, physical-chemical properties of drug molecules, tumor imaging information, healthcare insurance, social media, omics data, and traditional clinical trials are difficult to process using traditional methods and tools (20). Therefore, the applications of ML could enhance personalized medicine due to its important role in identifying potential objective biomarkers, genetic predictors, and new risk factors related to drug response (21). ML methods for drug response prediction including support vector machines, Bayesian multitask multiple kernel learning, Random forests, and neural network models have been reported (22). Currently, with the rapid development of next-generation high-throughput sequencing technologies, international large-scale cancer projects such as The Cancer Genome Atlas (TCGA), Cancer Cell Line Encyclopedia (CCLE) and Genomics of Drug Sensitivity in Cancer (GDSC) have offered large amount of multi-omics and clinical data based on different technologies, which are considered as the source of data to advance cancer studies (23).
To investigate the resistance mechanism, we obtained drug sensitivity data of five kind of BRAFi from the GDSC database and subsequently screened resistance-related genes by RNA-seq data from the CCLE database. Further, ML algorithms were used to select important genes related to drug resistance. Then we employed two external data to verify these variables based on an established classifier and resistance-susceptible gene variations. We could clearly distinguish resistant and sensitive patients according to our methods. The results of this study will provide a basis for individualized treatment with BRAF inhibitors. We present this article in accordance with the TRIPOD reporting checklist (available at https://tcr.amegroups.com/article/view/10.21037/tcr-24-961/rc).
Methods
Public databases and data preparation
The detailed information on cell lines was extracted from CCLE (https://depmap.org/portal/download/all/), which mainly includes cell line source, RNA expression, gene mutation, and copy number variation data. The cell line drug sensitive data including half maximal inhibitory concentration (IC50) and drug types were obtained from the GDSC database (https://www.cancerrxgene.org/downloads/anova). These datasets provide comprehensive genomic and transcriptomic profiles that are crucial for linking gene expression patterns to drug response. We downloaded the TCGA database, which includes information on patient status, survival time, drug treatment status, and gene expression for cancer patient data, from the public platform UCSC Xena (https://xenabrowser.net/datapages/). The study was conducted in accordance with the Declaration of Helsinki (as revised in 2013).
The RNA-seq data were log2 transformed before applying it to analysis, and the missing values were imputed with zero. All variables whose missing values were over 50% were omitted. Only the protein-coding RNAs were objects of study. To obtain a precise understanding of the expression discrepancy in cells, we conducted a principal component analysis (PCA) with expressional sequencing data from CCLE. The emerged two main clusters of cells were separated by unsupervised hierarchical clustering analysis (HCA). Finally, the larger cluster included 787 cells that were collected for further analysis (available online: https://cdn.amegroups.cn/static/public/tcr-24-961-1.xlsx).
BRAF inhibitor resistance score
We originated a method called the BRAFi resistance score (BRS) to evaluate the resistance potential of cell lines. First, five BRAF inhibitors (AZ628, Dabrafenib, HG6-64-1, PLX-4720, and SB590885) were selected. Then, we categorized cancer cell lines with drug responses, measured by IC50, into three bins, ranging from resistance (score =1), intermediate (score =0) to sensitive (score =−1). The BRSs were defined as the summing scores of five BRAF inhibitors in different cell lines. We removed cells with missing values over 50% at the very beginning and defined the rest of the missing values as score =0. The resistant cells were defined as BRS ≥3 and sensitive cells as score ≤−3. Finally, 235 cell lines were obtained for further study (available online: https://cdn.amegroups.cn/static/public/tcr-24-961-1.xlsx). RNA-seq data was then employed to assess differences in gene expression between the two groups.
Differential expression genes
The analysis of differential expressed mRNA between BRAF resistant and sensitive groups was performed by R software. Student’s t-test, Orthogonal Partial Least Squares-Discriminant Analysis (OPLS-DA), and expression fold change were used to compare the two groups. Differential expression genes were defined as P<0.05, |log2FC| >1, and VIP value >1. As a result, 990 genes were screened out with the above threshold. The volcano plot demonstrated the directions of these differential genes. The most common mutations of the two groups were displayed and visualized in an Oncoplot. To further explore the biological pathways involved, we performed pathway enrichment analysis on the differences such as Gene Ontology (GO), Kyoto Encyclopedia of Genes and Genomes (KEGG) analysis, and gene set enrichment analysis (GSEA). This analysis helps in mapping these genes to specific pathways, such as oxidative phosphorylation and metabolic pathways, which are known to be implicated in drug resistance mechanisms.
Feature selection process based on XGboost
Subsequently, the 990 differentially expressed genes were used to select important variables to predict drug response. The importance rank of the features was calculated from the Shapley Additive exPlanations (SHAP) mean score by the XGboost method. The training and test set was set at 7:3. Then the features whose importance score >0.1 were subsequently selected as inputs in model building. In addition, the SHAP approach was used to explain the effects of all feature contributions on the outcome of each patient (24). Totally 37 variables were inputted to develop a drug response model. These genes are key indicators of altered biological pathways contributing to drug resistance.
Drug sensitivity determination based on an established predictor
We applied the feature selection and prediction procedure derived from Bolis et al. (25) and further used to select features for drug-response prediction. For the 787 cell lines of cluster1, we firstly abandoned the mRNA data which was unexpressed in more than 1/3 of cell lines. Subsequently, we retained the genes based on the Spearman correlation coefficient P<0.01 with LnIC50. Then we used a ML algorithm of Bayesian ridge regression to predict IC50. The dataset was split into training and test sets with a ratio of 7:3, and a 10-fold cross-validation method was used to verify the accuracy of the model. Instead of the 10 times repeated Leave-Half-Out cross-validation procedure, we concurrently used data from the two most common BRAF inhibitors for clinical use, Dabrafenib, and Vemurafenib, to reduce data and classification error. We selected variables significantly correlated with LnIC50 (Spearman, P<0.05) for constructing the prediction model.
Bayesian Ridge regression model was used to predict the sensitivity of TCGA pan-cancer study patients. According to the expression of screened variables, the patients were classified into sensitive and resistant by the median of the predicted value. Only patients predicted to be sensitive or resistant to Dabrafenib and Vemurafenib concurrently were included in corresponding groups. Through the prediction and classification procedure, 3,014 BRAFi sensitive patients and 3,015 BRAFi resistant patients were selected from 10,535 patients for further study, detailed in available online: https://cdn.amegroups.cn/static/public/tcr-24-961-2.xlsx.
Literature evidence-based sensitivity classification
We next generated a sensitivity classification method based on published literature evidence (26,27). As previously reported, the appearance of BRAF inhibitor resistance is associated with some specific changes in a gene such as mutations and copy number variants. Thus, according to published literature, 17 gene alterations (EGFR, IGF1R, KRAS, MAP2K1, MAP2K2, MET, NF1, NRAS, PDGFRB, PTEN, RAF1, STAG3, CDKN2A, MAP3K8, CCND1, ARAF, STAG2) that influence BRAF inhibitor sensitivity the most were selected. Then the mutation and copy number data of the TCGA pan-cancer study patients were obtained. We considered the patients who carry BRAFV600 series mutations as sensitive to BRAF inhibitors, as most literature reported. Apart from BRAFV600 mutations, patients who concurrently carry specific gene changes or another BRAF site mutation were deemed resistant to BRAF inhibitors.
For the 17 specific gene alterations, patients carried mutations in EGFR, IGF1R, KRAS, MAP2K1, MAP2K2, MET, NF1, NRAS, PDGFRB, PTEN, RAF1, amplifications in RAF1, BRAF, MAP3K8, CCND1, ARAF, or deletions in STAG3, CDKN2A, PTEN, NF1, STAG2 were classified into the resistant group. We only considered the non-silent mutations as effective. Samples without RNA-seq data were omitted from the analysis. With this literature evidence-based classification method, 368 BRAF inhibitor sensitive and 185 resistant patients were selected for further validation (available online: https://cdn.amegroups.cn/static/public/tcr-24-961-3.xlsx).
Validation of 37-feature classification
To validate the predictive effect of the 37 features, we applied these features to external datasets. We predicted and established two datasets using predictor and literature evidence from TCGA. Multi ML algorithms such as linear regression, K-nearest neighbor, support vector machine, decision tree, random forest, Adaboost, Catboost, linear discriminant analysis, and XGboost were employed to predict drug resistance. The area under the curve (AUC) and the precision/recall (PR) curve were used to evaluate the performance of the models.
Quantitative real-time polymerase chain reaction (qRT-PCR)
Total RNA was isolated from the rat myocardium samples with RNA extraction kit (TaKaRa, Dalian, China) according to instructions. Approximately 2 µg of total RNA was used for first-strand cDNA synthesis by cDNA Synthesis Kit (TaKaRa, Osaka, Japan). Quantitative real-time PCR was performed using QuantStudio 6 Flex System (Thermo Fisher Scientific, Massachusetts, USA) with TB Green qPCR Kit (TaKaRa, Osaka, Japan). Specific primers of the cDNAs are displayed in Table 1. We chose the quantitation-comparative 2−ΔΔCT algorithm with the normalization of data to reference gene GAPDH.
Table 1
Gene | Forward | Reverse |
---|---|---|
OXTR | CCGAGGCTCCAGTGAGAGA | CGCAGGCGAACCTAAAGTTG |
SLC2A4 | GGCTGTTGTCATACTTCTCATGG | GCCAGGACATTGTTGACCAG |
TBX2 | CACGGCTTCACCATCCTAAAC | TGCGGAAGGTGCTGTAAGG |
H2AC13 | AGAAGACTCGCATCATCCCG | TCCAGGCTTCTACTTGCCCT |
GAPDH | GGAGCGAGATCCCTCCAAAAT | GGCTGTTGTCATACTTCTCATGG |
qRT-PCR, quantitative real-time polymerase chain reaction.
Statistical analysis
For unsupervised clustering, RNA-seq data were input into PCA and HCA. For orthogonal partial least square discriminant analysis (OPLS-DA), the variable importance in projection (VIP) value over 1.0 was considered meaningful to the model. The VIP value threshold combined with |log2FC| >1 and P<0.05 (Student’s t-test) constructed the differential expression genes’ inclusion criteria. For oncoplot, Chi-squared tests were performed between BRAFi resistance and sensitive group to judge the mutational difference, and P<0.01 was considered as significant. For SHAP by XGboost feature selection, the importance score >0.1 was considered significant for the classification model. For univariate logistic regression, multivariate logistic regression, and Kaplan-Meier survival analysis, P<0.05, Adjusted P<0.05, and Log-rank P<0.05 was considered significant.
Statical analyses were performed by R 4.2.1 and Python 3.6. HCA, OPLS-DA, enrichment analysis, oncoplot, forest plot, and Kaplan-Meier curve were performed by R packages ggtree, ropls, clusterProfiler, ComplexHeatmap, forestplot, and survminer respectively. Plot visualization and beautification were performed by R package ggplot2. ML models and feature selection involved in this study were implemented by sklearn and shap packages of python.
Results
Group division according to PCA, HCA, and OPLS-DA
The drug sensitivity and expression data of 952 cell lines were finally downloaded from the GDSC and CCLE database, which contained most of the cancer types (Figure 1A). Then, HCA was employed to calculate and separate the cells. Given that BRAF mutations are more prevalent in solid tumors, we ultimately selected the 787 cell line, which represents solid tumors within the larger group, for the subsequent study (Figure 1B cluster1). Two hundred and thirty-five cell lines were further utilized to investigate the potential presence of BRAF inhibitor resistance (Figure 1C). To control for the influence of huge differences among cell types, we tested the RNA expression dispersion of these cell lines by PCA. The result showed that these cell lines could be divided into two conspicuous groups, we subsequently found the samples were clearly distinguished by solid or hematological tumors (Figure 1D).
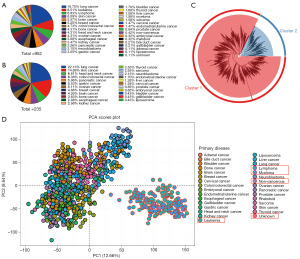
We next employed our original method BRS to evaluate the cells’ resistance potential and help grouping (Figure 2A). After that, 235 cell lines were extracted and divided into resistance group (n=163) and sensitive group (n=172) (available online: https://cdn.amegroups.cn/static/public/tcr-24-961-1.xlsx). This time, the PCA showed no remarkable subgroup generated except for a slight tendency (Figure 2B). To better demonstrate the differences between the two groups, we introduced a supervised clustering analysis OPLS-DA of RNA-seq data. Supervised clustering is aware of group information of samples, so the analysis is more result-oriented. A better separation trend of the two groups was seen from OPLS-DA (Figure 2C), which also indicated that the resistant difference of different cell lines could be properly illustrated by the RNA profile.
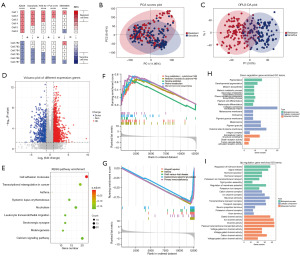
Differential expression genes analysis between BRAFi resistant and sensitive cells
To obtain an applicable difference between the two groups, we screened the differential expression genes by statistical approach and model contribution. Overall, after eliminating non-protein-coding RNA, 666 genes were upregulated and 324 genes were downregulated between the two groups. Figure 2D showed a volcano plot representing the results. To further elucidate the physiological function, the differential expression genes were subsequently subjected to GO enrichment analysis and KEGG pathway enrichment analysis. We employed all the differential expression genes in KEGG pathway enrichment, and the result shows that only the cell adhesion molecules pathway was significant (Figure 2E). Furthermore, the GSEA investigated the pathway changes of the two groups (Figure 2F,2G). The cytochrome P450 (CYP450), the key enzyme of drug metabolism, was significantly enriched in up-regulated genes. The important role of CYP450 isoenzymes in BRAF inhibitors metabolism was validated previously (27,28). Next, we separated the up- and down-regulated genes into different GO functional enrichment analysis. The up-regulated genes are generally enriched in ion channel activity and transportation, and the down-regulated genes are mainly involved in collagen-containing extracellular matrix (Figure 2H,2I). We also found that melanin metabolism and pigmentation were main results in down-regulated genes. This may indicate BRAFi resistance that is associated with the recession of melanin-related metabolic processes. In contrast, immune-related pathways were enriched in down-regulated genes, which indicated BRAFi resistant cells may have a potential for immune escape. We also noticed that hormone metabolism was enriched in both GO and GSEA results. Overall, the RNA changes of BRAFi resistance cells involved in ion channel transportation, melanocyte bioprocess, CYP450 and hormone metabolism.
To investigate the genetic profile of the two groups, we further plotted an oncoplot of gene panorama (Figure 3). From this plot, the gain of copy number in the resistant group was significantly more than the sensitive group (P=0.02). The commonest mutations were TP53, BRAF, PKHD1, and KRAS in order, with mutation frequency over 10%. Among all mutation types, missense mutation was the leading cause. Notably, a variety of components were statistically different between the two groups. For example, the rates of TP53 and KRAS mutation were significantly higher in resistant cells; mutations of BRAF, AOX1, and CASP8 were more common in the sensitive group. This result indicated that MAPK pathway activation (KRAS mutants), conformational changes of target gene (BRAF wild type) as well as broken tumor growth-apoptosis balance (TP53 and CASP8 mutants) may determine resistance occurrence. Besides, the possible role of AOX1, a promoter of peroxide and superoxide which was found to influence the resistance of PI3K inhibitors (29,30), in BRAFi resistance was first reported.
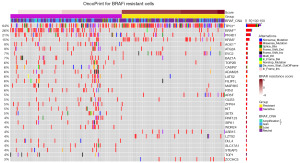
Feature selection and the 37-feature ML models
The 235 cell lines were grouped by the above method. We used the expression value of 990 differential expression genes as input variables in feature selection by the method of XGboost. To interpret ML models, SHAP values were used to visualize and explain how these features affect events according to the permutation importance method in the XGboost model (Figure 4A). Overall, 37 variables were selected based on the SHAP value >0.1 for the outcome (available online: https://cdn.amegroups.cn/static/public/tcr-24-961-4.xlsx). Then we built a ML model of BRAF inhibitor response classifier. In the selection of an optimal model, eight applicable algorithms were employed to calculate the predictive performance (Figure 4B,4C). For drug response, the discriminative performance of the nine ML models was displayed by the receiver operating characteristic (ROC) curves in Figure 4B. XGboost model exhibited the best discrimination analysis with AUC 0.99, followed by RF (AUC 0.98), CatBoost (AUC 0.98), SVM (AUC 0.96), LDA (AUC 0.95) and LR (AUC 0.95). The Decision Tree and Adaboost model performed the worst with AUC =0.77 and 0.87. In general, XGboost model had the best performance among the models when comprehensively evaluating the AUC, accuracy, specificity, sensitivity, precision, and F1 score. Also, the PR curve of all the models was shown in Figure 4C. According to the ROC curve, XGboost classifier was the best to predict BRAF response (AUC =0.99). Thus, we chose XGboost method to construct the response model.
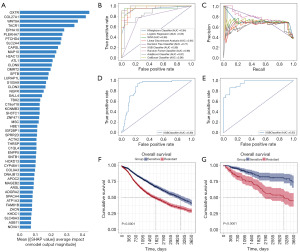
External validation of the 37 variables
Based on Bosil’s method, we validated the accuracy of the 37 variables. Bayesian Ridge regression was performed to predict IC50. According to the prediction model, we divided 6,029 patients from TCGA into resistant and sensitive groups. Then, we used 37 variables to predict drug response in this dataset. Strikingly, the AUC of XGboost achieved 0.88 based on 37 variables as in Figure 4D. The performance of the model in external validation datasets underscores the robustness and highlights the potential for using these gene expression profiles to identify high-risk patients. Meanwhile, based on well-proven genetical changes associated with drug response, 545 patients from TCGA database were also divided into the resistant and sensitive groups. The performance of XGboost model achieved 0.95, even higher than the above classification model (Figure 4E). Further, Kaplan-Meier (KM) curve was used to evaluate the prognosis of the two classified groups. The 10-year OS rate of the resistant group was dramatically poorer than the sensitive group (Figure 4F). Similarly, the prognosis in the resistant group was poorer than the sensitive group during the 10-year follow-up (Figure 4G). The poor prognosis of BRAFi resistant patients indicated the significant impact of drug resistance on disease progression and treatment.
Relationship between drug response and OS
To identify the effect of the 37 variables in BRAFi resistance, multivariate logistic regression analysis was applied to explore the potential risk factors of drug resistance as shown in available online: https://cdn.amegroups.cn/static/public/tcr-24-961-4.xlsx. There are 11 genes that were identified as independent risk and protective factors of BRAFi resistance (Figure 5A). ACTA2, MSC, SLC2A4, and SPTB are protective factors of resistance with adjusted P value =0.01, 0.007, 0.009, and 0.03, respectively; meanwhile, EPHA10, H2AC13, OXTR, TBX2, WNT9A, ZNF471, and THRSP are risk factors of resistance with adjusted P value =0.02, 0.04, 0.003, 0.002, 0.005, 0.03, and 0.03, respectively. The subsequent survival analysis aimed at demonstrating the direct effect of these factors on prognosis and the utility of our method. We obtained data of 10,434 patients from TCGA database and used the KM curve to identify the relationship between 37 variables and OS in the 10-year follow-up period (Figure S1). Notably, the high expression of OXTR, H2AC13 and TBX2, and low expression of SLC2A4 were associated with an unfavorable prognosis in cancer patients, echoed influences on resistance (Figure 5B-5E). The consistency of OXTR, H2AC13, TBX2, and SLC2A4 on resistance and prognosis may indicate the four factors report resistant status in a relatively direct way.
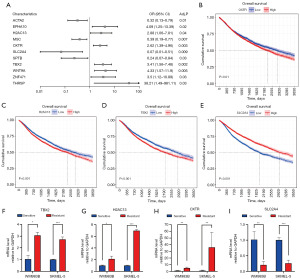
In order to verify the authenticity of the research findings, we employed the PCR technique to evaluate the mRNA expression level of TBX2, H2AC13, OXTR, and SLC2A4 (Figure 5F-5I). This analysis was conducted in both dabrafenib-generated BRAFi resistant and corresponding sensitive SKMEL-5 and WM983B cell lines (Figure S2). In concurrence with the outcomes of logistic regression and survival analysis, we observed significant upregulation of OXTR, H2AC13, and TBX2, while SLC2A4 exhibited notably diminished expression within both kinds of BRAFi resistant cell lines. These trends collectively indicate potential contributions of OXTR, H2AC13, TBX2, and SLC2A4 in influencing the phenomenon of BRAFi resistance, which may warrant a more in-depth validation process.
Discussion
Even though BRAFi-targeted therapies (e.g., dabrafenib) have improved the OS of patients, they are limited by heterogeneous response patterns and drug resistance. With the rising care costs, the development of tools to improve risk and drug response prediction is invaluable, as these tools may accurately identify patients who are at high risk (31). In this study, we used PCA, HCA, and OPLS-DA to select 235 resistant and sensitive cell lines of multiple cancer types from the public database. With analyses of RNA-seq data, we obtained resistant-prone changes from a transcription profile. In the age of precision medicine, ML is useful for converting extensive data into required structural data, making it possible to predict drug resistance timely and accurately (32). We established a gene-expression model using ML methods, consisting of 37 variables based on an RNA-seq database, which was externally validated and could be used to predict BRAFi resistance. Additionally, our approach effectively demonstrates how publicly available pharmacogenomic datasets can be utilized to identify and validate biological pathways contributing to drug resistance. By leveraging publicly available pharmacogenomic datasets such as CCLE and GDSC, we utilized a comprehensive set of RNA sequencing data from a variety of cancer cell lines. This broad dataset enhances the generalizability of the model across different cancer types. By integrating differential gene expression analysis, pathway enrichment, and ML, we were able to uncover novel insights into BRAFi resistance, which could guide the development of targeted therapies and improve patient outcomes. The machine-learning model developed in this study demonstrated robust predictive power, successfully classifying cell lines into BRAFi-resistant and sensitive groups. This predictive capability is a significant strength, as it provides a reliable method for identifying high-risk patients who may not respond well to BRAFi therapy.
Currently, the majority of studies have focused on the molecular mechanism of genomic or epigenetic abnormalities and tumor microenvironment which are related to drug resistance (13). Of that, the reactivation of the MAPK signal pathway plays an important role in drug resistance and has been intensively studied for BRAF inhibitors in recent years (26,33). When the MAPK pathway is reactivated, the resistance process begins to emerge. BRAFV600E splices isoform-induced drug resistance by forming BRAF dimers since homo- and heterodimers could activate MEK in the presence of BRAF inhibitors (34). In addition, alterations in COT, NRAS, KRAS, TP53 and NF1 also reactivate the MAPK pathway or alternative pathway (35,36). Meanwhile, a higher mutation frequency of TP53 and KRAS as well as less mutation of BRAF was found in resistance cell lines, which means the function of pathway molecules is still of great significance in our study. These gene-based alterations could potentially serve as inherited risk factors, contributing to tumor initiation and reduced therapeutic efficacy in subsequent generations. Additionally, cancer-associated fibroblasts and immune cells can secrete factors that protect tumor cells from treatment, facilitating resistance. Hypoxia within the TME also promotes drug resistance by stabilizing HIF-1α, which triggers survival pathways (37). Epigenetic modifications such as DNA methylation and histone acetylation can alter gene expression, leading to drug resistance. These changes may silence tumor suppressor genes or activate oncogenes, allowing cancer cells to survive despite therapy (38). Overexpression of efflux transporters like P-glycoprotein (P-gp) reduces intracellular drug concentrations, decreasing the effectiveness of chemotherapy. Moreover, the B7-H1/PD-1 signaling axis is recognized as a central mechanism by which tumors evade immune surveillance. Upon encountering tumor antigens, effector T cells specific to the tumor upregulate PD-1 expression and secrete interferon-γ (IFN-γ), which in turn induces B7-H1 expression in tumor and myeloid cells within the tumor microenvironment. B7-H1 binds to PD-1 on T cells, leading to their functional inhibition and disruption of antitumor activity. This localized immunosuppression facilitates tumor immune evasion, a process termed ‘adaptive immune resistance’, where the tumor environment manipulates immune checkpoints to escape immune-mediated destruction (39). The PD-1/PD-L1 signaling pathway is critical in regulating immune tolerance and facilitating immune escape within the tumor microenvironment. The interaction between PD-1 receptors, expressed on activated T cells, and PD-L1 receptors, found on the surface of cancer cells, suppresses the cytotoxic activity of T-lymphocytes. This suppression impairs the immune system’s ability to attack malignant cells, thereby promoting immune evasion and contributing to tumor survival (40). Cellular plasticity, including epithelial-mesenchymal transition (EMT), transdifferentiation, and phenotypic switching, represents a key mechanism of targeted therapy resistance in various cancers. This plasticity enables tumor cells to adopt alternative phenotypic states that no longer rely on drug-targeted pathways. As a result, these drug-resistant cells form a population of slow-cycling cells that may either regain drug sensitivity when treatment is paused or develop permanent resistance, ultimately leading to disease relapse (41). CYP450 is a well-established enzymatic system that controls the phase I metabolic conversion of xenobiotics. Approximately 80% of oxidative metabolism and 50% of common drug elimination can be attributed to the CYP450 family (42). As drug metabolism causes a decrease in therapeutic effects, the high enrichment of the CYP450 pathway in the resistant group is explicable. Overactivation of CYP450 enzymes could induce rapid drug clearance, and a higher dose of inhibitor is needed to maintain effective plasma concentration.
As the clinical use of BRAFi has expanded, addressing BRAFi resistance is crucial for improving patient outcomes, especially in cancers like melanoma where BRAFv600E mutations. Combining BRAF inhibitors with MEK inhibitors is a well-established approach that has shown efficacy in delaying resistance. Resistance to BRAFi can arise through the activation of alternative pathways, such as the PI3K/AKT pathway. Combining BRAFi with inhibitors of these parallel pathways could prevent the cancer cells from bypassing the blocked BRAF pathway (43). Combining BRAFi with immune checkpoint inhibitors like anti-PD-1 or anti-CTLA-4 or cytokines anti-CCL2 has shown promising results. Drugs that reverse epigenetic changes, such as histone deacetylase (HDAC) inhibitors or DNA methyltransferase inhibitors, could restore sensitivity to BRAFi (44). Emerging gene-editing technologies like CRISPR/Cas9 could correct epigenetic modifications or directly target resistance-conferring mutations, providing a more personalized approach to overcoming BRAFi resistance (45). Ongoing research aims to identify biomarkers that predict resistance to BRAFi. These biomarkers could be used to tailor therapy regimens, allowing for earlier intervention with alternative therapies in patients likely to develop resistance (46). Monitoring ctDNA can provide real-time insights into the development of resistance, allowing for timely adjustments to treatment strategies (47). Synthetic lethality targeting a second gene, along with the BRAF pathway, leads to cell death. This approach could be particularly effective in cells that have developed resistance through alternative survival pathways (48). Advanced drug delivery systems using nanoparticles can enhance the delivery of BRAFi and co-delivered drugs to the tumor site, improving efficacy and overcoming drug resistance by ensuring sustained and targeted delivery (49). Modulating the TME, such as inhibiting CAFs or reducing hypoxia, can make tumors more susceptible to treatment. Normalizing blood vessels within tumors can also improve drug delivery and reduce resistance. Developing inhibitors of efflux transporters like P-gp can increase the intracellular concentration of chemotherapy drugs, thereby enhancing their effectiveness. Tailoring treatments based on specific genetic and tumor molecular profiles can help in reducing resistance. Continued research and clinical trials will be crucial to refine these strategies and integrate them into standard cancer care, ultimately improving outcomes for patients with BRAFi resistance.
Our study identified for the first time the involvement of AOX1 in BRAFi resistance. Aldehyde oxidase (AOX) has become increasingly important in drug metabolism. It can oxidize aldehyde into corresponding carboxylic acids, which is a crucial process in xenobiotic and drug metabolism (50). In cancers such as glioblastoma and breast cancer, overexpression of AOX1 could be exploited to activate anti-neoplastic prodrugs in situ. However, for urogenital, ovarian, and prostate carcinoma, AOX1 may inactivate targets for antitumor agents (46). It has been reported that the loss of AOX1 expression is related to a poorer prognosis of bladder cancer and renal cell carcinoma (51,52). When it comes to BRAFi resistance, a higher frequency of mutation, mostly missense, was observed in sensitive cell lines. In concert with endoplasmic CYP450, AOX1 could play a part in inactivating and eliminating BRAF inhibitors via hepatic clearance. Nonetheless, the effect of AOX1 should be more complicated, due to the uncertainty of mutation, its two-sided potential for drug activity, as well as different cancer responses. A clear role of AOX1 in BRAFi resistance needs to be further investigated.
BRAF resistance may lead to poor prognosis in cancer patients, which our research has proved. By multivariate logistic regression and survival analysis, 4 genes showed consistency of drug-resistant prone and prognostic risk. A factor that influences prognosis may induce resistance in a more direct manner. Among all the features, OXTR owns the highest SHAP score, endowing it with the most important factor. OXTR is a G-protein coupled receptor binding to oxytocin hormone, which could activate the proliferation pathway via ERK1/2 phosphorylation (53). ERK reactivation is a manifestation of BRAFi resistance. The high expression of OXTR is associated with the enrichment of hormone metabolism. It has been reported that OXTR genetic alterations have led to poorer OS in hepatocellular carcinoma and pancreatic cancer (53,54). TBX2 gene is a member of the T-box family of transcription factors and has been reported to play critical roles in embryonic development and cell cycle of cancer. TBX2 is reported to regulate WNT signal proteins to induce bone metastasis of prostate cancer (55). Its ability to suppress the expression of cell cycle regulators p21 and p16 could promote malignant growth, and depletion of TBX2 leads to cell cycle arrest and senescence (56). It is well known that glycolysis plays an important role in tumor progression. Solute carrier family-2 (SLC2) can encode glucose transporter (GLUT) protein. Among them, SLC2A4 is significantly downregulated in many types of cancers such as lung cancer, liver cancer, and stomach cancer (57). Additionally, SLC2A4 could be an important prognosis biomarker for cancer in our study. H2AC13, a necroptosis-related gene signature, has been reported to relate to poorer prognosis of cervical cancer patients (58). In our study, we first found that H2AC13 directly contributes to drug resistance and poor prognosis. Our findings warrant further studies on the poorly defined molecular mechanism underlying the influence of OXTR, TBX2, SLC2A4, and H2AC13 in BRAFi resistance. The study identified AOX1 and other genes (OXTR, H2AC13, TBX2, SLC2A4) as potential biomarkers associated with BRAFi resistance. The findings contribute to a deeper understanding of the molecular mechanisms underlying resistance and provide potential targets for future therapeutic interventions.
Our study still has some limitations. First, although the ML model showed robust performance in external validation datasets, its applicability to diverse patients remains to be fully validated. The cell line-based model may not fully capture the complexity of tumors, where factors such as tumor heterogeneity and the tumor microenvironment play significant roles. Second, given the large number of features (37 genes) used in the model, there is a risk of overfitting, where the model may perform well on the training data but less effectively on new, unseen data. Third, while the model was validated using cell lines, it has not yet been validated in vivo. This gap may raise questions about the model’s relevance to clinical practice. Fourth, integrating data from different sources posed challenges in consistency and integrity across datasets. Variability in experimental conditions, data processing, and measurement techniques could introduce biases. Therefore, further validation in diverse patients and in vivo of the model is necessary. Regularization or the inclusion of more diverse training data is valuable to mitigate overfitting. The identified biomarkers suggest the directions of future research and integrating data from the tumor microenvironment could further improve the accuracy of the model.
Conclusions
In this study, the 37 variants-based BRAFi resistance classification method passed external validation successfully. Apart from previously reported genetic factors, AOX1 is first reported in our study to have a vital role in BRAFi metabolism and resistance. OXTR, H2AC13, TBX2, and SLC2A4 showed consistency of drug-resistant prone and prognostic risk. These findings provide potential biomarkers for clinical use and enhance our understanding of the underlying biological pathways that contribute to BRAFi resistance. Overall, the ML algorithm may be useful in predicting BRAF resistance and helping us to better understand BRAF resistance and improve the prognosis of cancer patients.
Acknowledgments
Funding: This research was supported by
Footnote
Reporting Checklist: The authors have completed the TRIPOD reporting checklist. Available at https://tcr.amegroups.com/article/view/10.21037/tcr-24-961/rc
Peer Review File: Available at https://tcr.amegroups.com/article/view/10.21037/tcr-24-961/prf
Conflicts of Interest: All authors have completed the ICMJE uniform disclosure form (available at https://tcr.amegroups.com/article/view/10.21037/tcr-24-961/coif). The authors have no conflicts of interest to declare.
Ethical Statement: The authors are accountable for all aspects of the work in ensuring that questions related to the accuracy or integrity of any part of the work are appropriately investigated and resolved. The study was conducted in accordance with the Declaration of Helsinki (as revised in 2013).
Open Access Statement: This is an Open Access article distributed in accordance with the Creative Commons Attribution-NonCommercial-NoDerivs 4.0 International License (CC BY-NC-ND 4.0), which permits the non-commercial replication and distribution of the article with the strict proviso that no changes or edits are made and the original work is properly cited (including links to both the formal publication through the relevant DOI and the license). See: https://creativecommons.org/licenses/by-nc-nd/4.0/.
References
- Sakai T, Matsumoto S, Ueda Y, et al. Clinicogenomic Features and Targetable Mutations in NSCLCs Harboring BRAF Non-V600E Mutations: A Multi-Institutional Genomic Screening Study (LC-SCRUM-Asia). J Thorac Oncol 2023;18:1538-49. [Crossref] [PubMed]
- Gunderwala A, Cope N, Wang Z. Mechanism and inhibition of BRAF kinase. Curr Opin Chem Biol 2022;71:102205. [Crossref] [PubMed]
- Halle BR, Johnson DB. Defining and Targeting BRAF Mutations in Solid Tumors. Curr Treat Options Oncol 2021;22:30. [Crossref] [PubMed]
- Gan X, Shen F, Deng X, et al. Prognostic implications of the BRAF-V600(E) mutation in papillary thyroid carcinoma based on a new cut-off age stratification. Oncol Lett 2020;19:631-40. [PubMed]
- Yaeger R, Kotani D, Mondaca S, et al. Response to Anti-EGFR Therapy in Patients with BRAF non-V600-Mutant Metastatic Colorectal Cancer. Clin Cancer Res 2019;25:7089-97. [Crossref] [PubMed]
- Mazieres J, Cropet C, Montané L, et al. Vemurafenib in non-small-cell lung cancer patients with BRAF(V600) and BRAF(nonV600) mutations. Ann Oncol 2020;31:289-94. [Crossref] [PubMed]
- Ottaviano M, Giunta EF, Tortora M, et al. BRAF Gene and Melanoma: Back to the Future. Int J Mol Sci 2021;22:3474. [Crossref] [PubMed]
- Jung T, Haist M, Kuske M, et al. Immunomodulatory Properties of BRAF and MEK Inhibitors Used for Melanoma Therapy-Paradoxical ERK Activation and Beyond. Int J Mol Sci 2021;22:9890. [Crossref] [PubMed]
- Bai X, Flaherty KT. Targeted and immunotherapies in BRAF mutant melanoma: where we stand and what to expect. Br J Dermatol 2021;185:253-62. [Crossref] [PubMed]
- Subbiah V, Baik C, Kirkwood JM. Clinical Development of BRAF plus MEK Inhibitor Combinations. Trends Cancer 2020;6:797-810. [Crossref] [PubMed]
- Zhong J, Yan W, Wang C, et al. BRAF Inhibitor Resistance in Melanoma: Mechanisms and Alternative Therapeutic Strategies. Curr Treat Options Oncol 2022;23:1503-21. [Crossref] [PubMed]
- Czarnecka AM, Bartnik E, Fiedorowicz M, et al. Targeted Therapy in Melanoma and Mechanisms of Resistance. Int J Mol Sci 2020;21:4576. [Crossref] [PubMed]
- Alqathama A. BRAF in malignant melanoma progression and metastasis: potentials and challenges. Am J Cancer Res 2020;10:1103-14. [PubMed]
- Ren Z, Xu Z, Chang X, et al. STC1 competitively binding βPIX enhances melanoma progression via YAP nuclear translocation and M2 macrophage recruitment through the YAP/CCL2/VEGFA/AKT feedback loop. Pharmacol Res 2024;204:107218. [Crossref] [PubMed]
- Friedman A, Siewe N. Overcoming Drug Resistance to BRAF Inhibitor. Bull Math Biol 2020;82:8. [Crossref] [PubMed]
- Cui JW, Li Y, Yang Y, et al. Tumor immunotherapy resistance: Revealing the mechanism of PD-1 / PD-L1-mediated tumor immune escape. Biomed Pharmacother 2024;171:116203. [Crossref] [PubMed]
- Dohm AE, Nakashima JY, Kalagotla H, et al. Stereotactic radiosurgery and anti-PD-1 + CTLA-4 therapy, anti-PD-1 therapy, anti-CTLA-4 therapy, BRAF/MEK inhibitors, BRAF inhibitors, or conventional chemotherapy for the management of melanoma brain metastases. Eur J Cancer 2023;192:113287. [Crossref] [PubMed]
- Hakeem H, Feng W, Chen Z, et al. Development and Validation of a Deep Learning Model for Predicting Treatment Response in Patients With Newly Diagnosed Epilepsy. JAMA Neurol 2022;79:986-96. [Crossref] [PubMed]
- Haug CJ, Drazen JM. Artificial Intelligence and Machine Learning in Clinical Medicine, 2023. N Engl J Med 2023;388:1201-8. [Crossref] [PubMed]
- An X, Chen X, Yi D, et al. Representation of molecules for drug response prediction. Brief Bioinform 2022;23:bbab393. [Crossref] [PubMed]
- Goyal RK, Kalaria SN, McElroy SL, et al. An exploratory machine learning approach to identify placebo responders in pharmacological binge eating disorder trials. Clin Transl Sci 2022;15:2878-87. [Crossref] [PubMed]
- Kuenzi BM, Park J, Fong SH, et al. Predicting Drug Response and Synergy Using a Deep Learning Model of Human Cancer Cells. Cancer Cell 2020;38:672-684.e6. [Crossref] [PubMed]
- Yang H, Gan L, Chen R, et al. From multi-omics data to the cancer druggable gene discovery: a novel machine learning-based approach. Brief Bioinform 2023;24:bbac528. [Crossref] [PubMed]
- Wang Y, Chen H, Sun T, et al. Risk predicting for acute coronary syndrome based on machine learning model with kinetic plaque features from serial coronary computed tomography angiography. Eur Heart J Cardiovasc Imaging 2022;23:800-10. [Crossref] [PubMed]
- Bolis M, Garattini E, Paroni G, et al. Network-guided modeling allows tumor-type independent prediction of sensitivity to all-trans-retinoic acid. Ann Oncol 2017;28:611-21. [Crossref] [PubMed]
- Proietti I, Skroza N, Bernardini N, et al. Mechanisms of Acquired BRAF Inhibitor Resistance in Melanoma: A Systematic Review. Cancers (Basel) 2020;12:2801. [Crossref] [PubMed]
- Dulgar O, Kutuk T, Eroglu Z. Mechanisms of Resistance to BRAF-Targeted Melanoma Therapies. Am J Clin Dermatol 2021;22:1-10. [Crossref] [PubMed]
- Sorf A, Vagiannis D, Ahmed F, et al. Dabrafenib inhibits ABCG2 and cytochrome P450 isoenzymes; potential implications for combination anticancer therapy. Toxicol Appl Pharmacol 2022;434:115797. [Crossref] [PubMed]
- Narci K, Kahraman DC, Koyas A, et al. Context dependent isoform specific PI3K inhibition confers drug resistance in hepatocellular carcinoma cells. BMC Cancer 2022;22:320. [Crossref] [PubMed]
- Davis BH, Beasley TM, Amaral M, et al. Pharmacogenetic Predictors of Cannabidiol Response and Tolerability in Treatment-Resistant Epilepsy. Clin Pharmacol Ther 2021;110:1368-80. [Crossref] [PubMed]
- Freeman M, Laks S. Surveillance imaging for metastasis in high-risk melanoma: importance in individualized patient care and survivorship. Melanoma Manag 2019;6:MMT12. [Crossref] [PubMed]
- Katta MR, Kalluru PKR, Bavishi DA, et al. Artificial intelligence in pancreatic cancer: diagnosis, limitations, and the future prospects-a narrative review. J Cancer Res Clin Oncol 2023;149:6743-51. [Crossref] [PubMed]
- Oliveira ÉA, Chauhan J, Silva JRD, et al. TOP1 modulation during melanoma progression and in adaptative resistance to BRAF and MEK inhibitors. Pharmacol Res 2021;173:105911. [Crossref] [PubMed]
- Vido MJ, Le K, Hartsough EJ, et al. BRAF Splice Variant Resistance to RAF Inhibitor Requires Enhanced MEK Association. Cell Rep 2018;25:1501-1510.e3. [Crossref] [PubMed]
- Kozar I, Margue C, Rothengatter S, et al. Many ways to resistance: How melanoma cells evade targeted therapies. Biochim Biophys Acta Rev Cancer 2019;1871:313-22. [Crossref] [PubMed]
- Vlašić I, Horvat A, Tadijan A, et al. p53 Family in Resistance to Targeted Therapy of Melanoma. Int J Mol Sci 2022;24:65. [Crossref] [PubMed]
- Wu Q, You L, Nepovimova E, et al. Hypoxia-inducible factors: master regulators of hypoxic tumor immune escape. J Hematol Oncol 2022;15:77. [Crossref] [PubMed]
- Hong Z, Liu F, Zhang Z. Ubiquitin modification in the regulation of tumor immunotherapy resistance mechanisms and potential therapeutic targets. Exp Hematol Oncol 2024;13:91. [Crossref] [PubMed]
- Sanmamed MF, Chen L. A Paradigm Shift in Cancer Immunotherapy: From Enhancement to Normalization. Cell 2018;175:313-26. [Crossref] [PubMed]
- Rogala P, Czarnecka AM, Cybulska-Stopa B, et al. Long Term Results and Prognostic Biomarkers for Anti-PD1 Immunotherapy Used after BRAFi/MEKi Combination in Advanced Cutaneous Melanoma Patients. Cancers (Basel) 2022;14:2123. [Crossref] [PubMed]
- Boumahdi S, de Sauvage FJ. The great escape: tumour cell plasticity in resistance to targeted therapy. Nat Rev Drug Discov 2020;19:39-56. [Crossref] [PubMed]
- Zhao M, Ma J, Li M, et al. Cytochrome P450 Enzymes and Drug Metabolism in Humans. Int J Mol Sci 2021;22:12808. [Crossref] [PubMed]
- Arasi MB, De Luca G, Chronopoulou L, et al. MiR126-targeted-nanoparticles combined with PI3K/AKT inhibitor as a new strategy to overcome melanoma resistance. Mol Ther 2024;32:152-67. [Crossref] [PubMed]
- Embaby A, Huijberts SCFA, Wang L, et al. A Proof-of-Concept Study of Sequential Treatment with the HDAC Inhibitor Vorinostat following BRAF and MEK Inhibitors in BRAFV600-Mutated Melanoma. Clin Cancer Res 2024;30:3157-66. [Crossref] [PubMed]
- Goh CJH, Wong JH, El Farran C, et al. Identification of pathways modulating vemurafenib resistance in melanoma cells via a genome-wide CRISPR/Cas9 screen. G3 (Bethesda) 2021;11:jkaa069. [Crossref] [PubMed]
- Mansoori B, Mohammadi A, Davudian S, et al. The Different Mechanisms of Cancer Drug Resistance: A Brief Review. Adv Pharm Bull 2017;7:339-48. [Crossref] [PubMed]
- Di Nardo L, Del Regno L, Di Stefani A, et al. The dynamics of circulating tumour DNA (ctDNA) during treatment reflects tumour response in advanced melanoma patients. Exp Dermatol 2023;32:1785-93. [Crossref] [PubMed]
- Karpel-Massler G, Ishida CT, Bianchetti E, et al. Inhibition of Mitochondrial Matrix Chaperones and Antiapoptotic Bcl-2 Family Proteins Empower Antitumor Therapeutic Responses. Cancer Res 2017;77:3513-26. [Crossref] [PubMed]
- Wang C, Li Q, Xiao J, et al. Nanomedicine-based combination therapies for overcoming temozolomide resistance in glioblastomas. Cancer Biol Med 2023;20:325-43. [Crossref] [PubMed]
- Gajula SNR, Nathani TN, Patil RM, et al. Aldehyde oxidase mediated drug metabolism: an underpredicted obstacle in drug discovery and development. Drug Metab Rev 2022;54:427-48. [Crossref] [PubMed]
- Vantaku V, Putluri V, Bader DA, et al. Epigenetic loss of AOX1 expression via EZH2 leads to metabolic deregulations and promotes bladder cancer progression. Oncogene 2020;39:6265-85. [Crossref] [PubMed]
- Xiong L, Feng Y, Hu W, et al. Expression of AOX1 Predicts Prognosis of Clear Cell Renal Cell Carcinoma. Front Genet 2021;12:683173. [Crossref] [PubMed]
- Harricharran T, Ogunwobi OO. Oxytocin and oxytocin receptor alterations, decreased survival, and increased chemoresistance in patients with pancreatic cancer. Hepatobiliary Pancreat Dis Int 2020;19:175-80. [Crossref] [PubMed]
- Hu B, Yang XB, Sang XT. Molecular subtypes based on immune-related genes predict the prognosis for hepatocellular carcinoma patients. Int Immunopharmacol 2021;90:107164. [Crossref] [PubMed]
- Nandana S, Tripathi M, Duan P, et al. Bone Metastasis of Prostate Cancer Can Be Therapeutically Targeted at the TBX2-WNT Signaling Axis. Cancer Res 2017;77:1331-44. [Crossref] [PubMed]
- Lu S, Louphrasitthiphol P, Goradia N, et al. TBX2 controls a proproliferative gene expression program in melanoma. Genes Dev 2021;35:1657-77. [Crossref] [PubMed]
- Shi Z, Liu J, Wang F, et al. Integrated analysis of Solute carrier family-2 members reveals SLC2A4 as an independent favorable prognostic biomarker for breast cancer. Channels (Austin) 2021;15:555-68. [Crossref] [PubMed]
- Xing X, Tian Y, Jin X. Immune infiltration and a necroptosis-related gene signature for predicting the prognosis of patients with cervical cancer. Front Genet 2023;13:1061107. [Crossref] [PubMed]