Construction of a prognostic survival model with tumor immune-related genes for breast cancer
Highlight box
Key findings
• Our research developed a six-gene model for prognosticating the overall survival of patients with breast cancer. Additionally, we identified the C-X-C motif chemokine ligand 9 (CXCL9) as a pivotal factor in immune cell infiltration within breast cancer tissues, suggesting its potential as a crucial biomarker for assessing immune response and therapeutic efficacy in breast cancer management.
What is known and what is new?
• Numerous studies have demonstrated that CXCL9 is implicated in macrophage polarization, CD8+ T-cell infiltration, and other forms of immune cell infiltration during the progression of breast cancer. Additionally, CXCL9 has been associated with favorable prognostic outcomes.
• Our research identified that a prognostic model incorporating six genes, including CXCL9, is capable of predicting breast cancer outcomes.
What is the implication, and what should change now?
• Our findings hold significant implications for the understanding of immunotherapy response and may inform the prediction of immune checkpoint inhibitor efficacy in patients with breast cancer. Notably, CXCL9 appears to play a crucial role in the immunotherapy of breast cancer by modulating immune cell infiltration. Consequently, CXCL9 may emerge as a potential biomarker for immunotherapy.
Introduction
Breast cancer is a highly heterogeneous malignancy characterized by the interaction of various immune cell clusters, including T cells, innate lymphoid cells, and myeloid cells, with cancer cells, epithelial cells, mesenchymal cells, and fibroblasts (1-5). These interactions contribute to the tumor’s resistance to radiotherapy and chemotherapy, immune evasion, and nonresponsiveness to immunotherapy (3,4,6), which result in substantial variations in prognostic survival times among different breast cancer subtypes and treatment conditions (7-9). Therefore, it is imperative to develop novel prognostic models that can accurately predict clinical outcomes and survival time in patients undergoing treatment for breast cancer.
With the widespread adoption of programmed cell death protein-1 (PD-1)/programmed death ligand 1 (PD-L1) immunosuppressants in clinical practice, patients with breast cancer may benefit from therapeutic advantages from PD-1/PD-L1 immunotherapy (10-12). Recent study has identified PD-1/PD-L1 pathway-related subtypes of breast cancer, which are closely linked to the immune microenvironment, ferroptosis status, and m6A modifications in breast cancer patients (13). The gene signature associated with the PD-1/PD-L1 pathway may aid in distinguishing subtypes and predicting prognosis in breast cancer patients (13). Currently, the expression of PD-L1 in tumor tissue is not a dependable biomarker for predicting the efficacy and prognosis of neoadjuvant chemotherapy or immunotherapy (14-17). Whole-genome sequencing and whole-exome sequencing contribute to the identification of predisposition genes, facilitate risk stratification, and uncover rare single nucleotide polymorphisms (18). These technologies also help to identifying novel mutations that could potentially inform personalized treatments in the future (18). A recent genomic analysis in breast cancer underscores the complexity related to this disease, as an inverse relationship was discovered between the PD-L1 gene and the estrogen receptor 1 (ESR1) gene (15), which reduces sensitivity to endocrine therapy and is especially detrimental in the context of metastatic breast cancer (19). However, the ESR1 mutation has the potential to enhance sensitivity to immunotherapy, which is attributed to the increased presence of PD-L1+ macrophages (20). Furthermore, there have been inconsistent findings regarding the influence of PD-L1 expression on breast cancer survival (14,16). In one study, high PD-L1 expression was linked to poorer relapse-free survival [hazard ratio (HR) =1.824; P=0.01] and overall survival (OS) (HR =2.585; P=0.001) in patients with local advanced breast cancer with low levels of total tumor-infiltrating lymphocytes (TILs) (14). In contrast, PD-L1 messenger RNA (mRNA) expression has been detected in approximately 60% of breast tumors and is correlated with elevated levels of TILs and prolonged recurrence-free survival (16). Furthermore, immunohistochemical staining results indicate that PD-L1 expression in breast cancer ranges from approximately 10% to 30%, with significant variability contingent upon tumor stage and molecular subtype. Triple-negative breast cancer (TNBC) exhibits the highest proportion of PD-L1 positivity, whereas hormone receptor-positive breast cancers demonstrate minimal expression, observed in only 0% to 10% of cases (21).
Given the variability in results across different studies (14,16), caution should be exercised when employing PD-L1 expression as a prognostic marker. Consequently, the development of an undifferentiated prognostic model for breast cancer is instrumental in predicting patient survival and facilitating the formulation of personalized treatment plans, thereby enhancing patient survival rates and quality of life. In this study, our objective was to develop a prognostic model for undifferentiated breast cancer based on immune-related markers. We used data from The Cancer Genome Atlas (TCGA) and the GeneCards database to identify prognostic genes and immune-related markers, respectively. Subsequently, we employed least absolute shrinkage and selection operator (LASSO) and Cox proportional hazards regression models to construct a six-gene prognostic model centered on cancer immune-related markers, aimed at predicting the survival outcomes of patients with breast cancer. We present this article in accordance with the TRIPOD reporting checklist (available at https://tcr.amegroups.com/article/view/10.21037/tcr-24-2137/rc).
Methods
Data collection
Differentially expressed genes (DEGs) between normal tissues (n=113) and breast tumor tissues (n=1,113) were selected and downloaded from the TCGA database (https://portal.gdc.cancer.gov). DEGs were selected according to the criteria of |log2(fold change)| ≥2 and an adjusted P value (P.adj) <0.05. Breast cancer (n=1,113) related prognostic factors were screened according to the TCGA database as described previously (22,23). The GeneCards database (https://www.genecards.org/) was used to acquire comprehensive information regarding cancer immune infiltration-related genes. The study was conducted in accordance with the Declaration of Helsinki (as revised in 2013).
Association between prognostic factors and immune infiltration
The single-sample gene set enrichment analysis (ssGSEA) and CIBERSOFT algorithms were used to investigate the association between prognostic indicators and immune infiltration in patients with breast cancer. The ssGSEA algorithm, provided by the “GSVA” package in R version 1.46.0 (The R Foundation for Statistical Computing, Vienna, Austria) was used to calculate immune infiltration based on markers for 24 immune cells, as described previously (24,25). The CIBERSORT algorithm, provided by the CIBERSORTx website (https://cibersortx.stanford.edu/), was used to calculate immune cell infiltration based on markers of 22 types of immune cells, as described previously (26,27).
Functional enrichment analysis
We used the TCGA database to identify C-X-C motif chemokine ligand 9 (CXCL9)-related genes for GSEA using clusterProfiler version 4.4.4. A total of 178 CXCL9-related genes with |log2(fold change)| ≥2 and P.adj <0.05 were selected for Gene Ontology (GO) analysis and Kyoto Encyclopedia of Genes and Genomes (KEGG) analysis with GOplot version 1.0.2 and clusterProfiler version 4.4.4, as described previously (28,29).
Statistical analysis
The data are presented as the mean ± standard deviation. LASSO and Cox proportional hazards regression were employed to select prognostic indicators with the “glmnet” package (version 4.1.7), “survival” package (version 3.3.1), and “rms” package (version 6.3-0) in R software version 4.2.1. Pearson correlation coefficient analysis was used to assess correlations. The Wilcoxon rank-sum test was used for measurable factors, and the chi-square test or Fisher exact test was employed for categorical factors via the “ggplot2” package (version 3.3.6), “stats” package (version 4.2.1), and “car” package (version 3.1-0) in R. The R packages “DESeq2” (version 1.36.0) and “edgeR” (version 3.38.2) were used to analyze the DEGs in breast cancer. The R “survival” package (version 3.3.1) was used to select the prognostic factors in breast cancer. Kaplan-Meier survival analysis was used to evaluate the association of prognostic indicators with OS and disease-specific survival (DSS) using the “survival” (version 3.3.1), “survminer” (version 0.4.9), and “ggplot2 (version 3.3.6) packages. Nomogram and calibration related models were built and visualized using the R “rms” package (version 6.3-0). A P value <0.05 indicated statistical significance.
Results
Screening of prognostic indicators for breast cancer
DEGs and prognostic factors were identified from the TCGA database, with the criteria of |log2(fold change)| ≥2 and P.adj <0.05 being applied. Cancer immune-associated genes were filtered according to a relevance score exceeding 10 via the GeneCards database. As illustrated in Figure 1A, a total of 92 key genes meeting these stringent criteria were selected for further analysis. A volcano plot was used to illustrate the six genes ultimately included based on LASSO and Cox regression analyses (Figure 1B). The results from the LASSO coefficient screening (Figure 1C), assessed using deviance, along with the LASSO variable locus graph (Figure 1D), indicated a total of 23 nonzero selected parameters. Subsequently, we employed Cox univariate (Figure 1E) and multivariate (Figure 1F) analyses to identify six prognostic genes. Our findings indicated that elevated expression levels of zic family member 2 (ZIC2) [HR =1.598; 95% confidence interval (CI): 1.126–2.267; P=0.009] and solute carrier family 7 member 5 (SLC7A5) (HR =1.592; 95% CI: 1.078–2.350; P=0.02) were associated with poorer OS in breast cancer, whereas increased expression of forkhead box J1 (FOXJ1) (HR =0.699; 95% CI: 0.493–0.989; P=0.043), CXCL9 (HR =0.559; 95% CI: 0.340–0.918; P=0.02), tumor necrosis factor receptor superfamily member 18 (TNFRSF18) (HR =0.607; 95% CI: 0.429–0.857; P=0.005), and serine protease 2 (PRSS2) (HR =0.593; 95% CI: 0.418–0.841; P=0.003) were found to be protective factors for OS in patients with breast cancer (Figure 1E). In addition, we determined the correlation of six prognostic indicators with the following clinical characteristics: age; pathologic T stage; M stage and N stage; pathologic stage; histological type; progesterone receptor (PR), estrogen receptor (ER), and human epidermal growth factor receptor 2 (HER2) status; menopause status; and the prediction analysis of microarray 50 (PAM50) (Table 1).
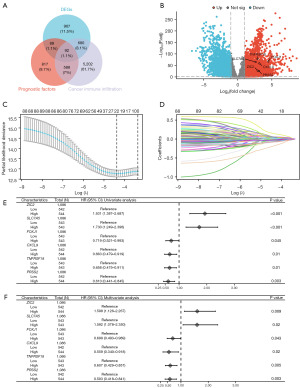
Table 1
Characteristics | ZIC2 | SLC7A5 | FOXJ1 | CXCL9 | TNFRSF18 | PRSS2 | |||||||||||||||||
---|---|---|---|---|---|---|---|---|---|---|---|---|---|---|---|---|---|---|---|---|---|---|---|
L- (n=543) | H- (n=544) | P value | L- (n=543) | H- (n=544) | P value | L- (n=543) | H- (n=544) | P value | L- (n=543) | H- (n=544) | P value | L- (n=543) | H- (n=544) | P value | L- (n=543) | H- (n=544) | P value | ||||||
Age (years) (n=1,087) | <0.001 | 0.14 | 0.92 | 0.02 | 0.98 | 0.04 | |||||||||||||||||
≤60 | 337 (31.0) | 266 (24.5) | 289 (26.6) | 314 (28.9) | 302 (27.8) | 301 (27.7) | 282 (25.9) | 321 (29.5) | 301 (27.7) | 302 (27.8) | 284 (26.1) | 319 (29.3) | |||||||||||
>60 | 206 (19.0) | 278 (25.6) | 254 (23.4) | 230 (21.2) | 241 (22.2) | 243 (22.4) | 261 (24.0) | 223 (20.5) | 242 (22.3) | 242 (22.3) | 259 (23.8) | 225 (20.7) | |||||||||||
Pathologic T stage (n=1,084) | 0.003 | 0.16 | 0.27 | 0.051 | 0.46 | 0.11 | |||||||||||||||||
T1 & T2 | 471 (43.5) | 438 (40.4) | 446 (41.1) | 463 (42.7) | 447 (41.2) | 462 (42.6) | 441 (40.7) | 468 (43.2) | 459 (42.3) | 450 (41.5) | 444 (41.0) | 465 (42.9) | |||||||||||
T3 & T4 | 69 (6.4) | 106 (9.8) | 96 (8.9) | 79 (7.3) | 94 (8.7) | 81 (7.5) | 99 (9.1) | 76 (7.0) | 83 (7.7) | 92 (8.5) | 97 (8.9) | 78 (7.2) | |||||||||||
Pathologic N stage (n=1,068) | 0.03 | 0.14 | 0.08 | 0.55 | 0.43 | 0.18 | |||||||||||||||||
N0 & N1 | 449 (42.0) | 426 (39.9) | 446 (41.8) | 429 (40.2) | 424 (39.7) | 451 (42.2) | 438 (41.0) | 437 (40.9) | 435 (40.7) | 440 (41.2) | 425 (39.8) | 450 (42.1) | |||||||||||
N2 & N3 | 82 (7.7) | 111 (10.4) | 87 (8.1) | 106 (9.9) | 107 (10.0) | 86 (8.1) | 92 (8.6) | 101 (9.5) | 102 (9.6) | 91 (8.5) | 104 (9.7) | 89 (8.3) | |||||||||||
Pathologic M stage (n=925) | 0.07 | 0.42 | 0.70 | 0.057 | 0.89 | 0.14 | |||||||||||||||||
M0 | 459 (49.6) | 446 (48.2) | 444 (48.0) | 461 (49.8) | 447 (48.3) | 458 (49.5) | 439 (47.5) | 466 (50.4) | 467 (50.5) | 438 (47.4) | 437 (47.2) | 468 (50.6) | |||||||||||
M1 | 6 (0.6) | 14 (1.5) | 8 (0.9) | 12 (1.3) | 9 (1.0) | 11 (1.2) | 14 (1.5) | 6 (0.6) | 10 (1.1) | 10 (1.1) | 13 (1.4) | 7 (0.8) | |||||||||||
Pathologic stage (n=1,063) | 0.006 | 0.53 | 0.07 | 0.45 | 0.66 | 0.40 | |||||||||||||||||
Stage I & II | 417 (39.2) | 384 (36.1) | 403 (37.9) | 398 (37.4) | 388 (36.5) | 413 (38.9) | 394 (37.1) | 407 (38.3) | 397 (37.3) | 404 (38.0) | 395 (37.2) | 406 (38.2) | |||||||||||
Stage III & IV | 111 (10.4) | 151 (14.2) | 126 (11.9) | 136 (12.8) | 144 (13.5) | 118 (11.1) | 136 (12.8) | 126 (11.9) | 134 (12.6) | 128 (12.0) | 137 (12.9) | 125 (11.8) | |||||||||||
Histological type (n=1,027) | 0.053 | <0.001 | 0.84 | 0.01 | 0.54 | <0.001 | |||||||||||||||||
Infiltrating ductal carcinoma | 392 (38.2) | 384 (37.4) | 328 (31.9) | 448 (43.6) | 388 (37.8) | 388 (37.8) | 377 (36.7) | 399 (38.9) | 396 (38.6) | 380 (37.0) | 356 (34.7) | 420 (40.9) | |||||||||||
Infiltrating lobular carcinoma | 90 (8.8) | 115 (11.2) | 160 (15.6) | 45 (4.4) | 102 (9.9) | 103 (10.0) | 96 (9.3) | 109 (10.6) | 93 (9.1) | 112 (10.9) | 129 (12.6) | 76 (7.4) | |||||||||||
Mixed histology | 18 (1.8) | 11 (1.1) | 20 (1.9) | 9 (0.9) | 16 (1.6) | 13 (1.3) | 15 (1.5) | 14 (1.4) | 14 (1.4) | 15 (1.5) | 18 (1.8) | 11 (1.1) | |||||||||||
Mucinous carcinoma | 12 (1.2) | 5 (0.5) | 8 (0.8) | 9 (0.9) | 7 (0.7) | 10 (1.0) | 15 (1.5) | 2 (0.2) | 8 (0.8) | 9 (0.9) | 8 (0.8) | 9 (0.9) | |||||||||||
PR status (n=1,034) | 0.29 | <0.001 | <0.001 | <0.001 | <0.001 | 0.19 | |||||||||||||||||
Negative | 163 (15.8) | 179 (17.3) | 88 (8.5) | 254 (24.6) | 223 (21.6) | 119 (11.5) | 143 (13.8) | 199 (19.2) | 199 (19.2) | 143 (13.8) | 181 (17.5) | 161 (15.6) | |||||||||||
Positive | 354 (34.2) | 338 (32.7) | 430 (41.6) | 262 (25.3) | 294 (28.4) | 398 (38.5) | 369 (35.7) | 323 (31.2) | 312 (30.2) | 380 (36.8) | 336 (32.5) | 356 (34.4) | |||||||||||
ER status (n=1,037) | 0.76 | <0.001 | <0.001 | <0.001 | <0.001 | 0.31 | |||||||||||||||||
Negative | 122 (11.8) | 118 (11.4) | 30 (2.9) | 210 (20.3) | 166 (16.0) | 74 (7.1) | 84 (8.1) | 156 (15.0) | 148 (14.3) | 92 (8.9) | 113 (10.9) | 127 (12.2) | |||||||||||
Positive | 396 (38.2) | 401 (38.7) | 490 (47.3) | 307 (29.6) | 352 (33.9) | 445 (42.9) | 431 (41.6) | 366 (35.3) | 367 (35.4) | 430 (41.5) | 405 (39.1) | 392 (37.8) | |||||||||||
HER2 status (n=717) | <0.001 | 0.06 | 0.17 | 0.10 | 0.11 | 0.005 | |||||||||||||||||
Negative | 295 (41.1) | 265 (37.0) | 283 (39.5) | 277 (38.6) | 279 (38.9) | 281 (39.2) | 270 (37.7) | 290 (40.4) | 284 (39.6) | 276 (38.5) | 264 (36.8) | 296 (41.3) | |||||||||||
Positive | 57 (7.9) | 100 (13.9) | 66 (9.2) | 91 (12.7) | 88 (12.3) | 69 (9.6) | 64 (8.9) | 93 (13.0) | 91 (12.7) | 66 (9.2) | 94 (13.1) | 63 (8.8) | |||||||||||
Menopause status (n=976) | <0.001 | 0.53 | 0.92 | 0.18 | 0.24 | 0.03 | |||||||||||||||||
Pre | 140 (14.3) | 90 (9.2) | 108 (11.1) | 122 (12.5) | 112 (11.5) | 118 (12.1) | 103 (10.6) | 127 (13.0) | 118 (12.1) | 112 (11.5) | 97 (9.9) | 133 (13.6) | |||||||||||
Peri | 21 (2.2) | 19 (1.9) | 22 (2.3) | 18 (1.8) | 20 (2.0) | 20 (2.0) | 19 (1.9) | 21 (2.2) | 25 (2.6) | 15 (1.5) | 19 (1.9) | 21 (2.2) | |||||||||||
Post | 325 (33.3) | 381 (39.0) | 356 (36.5) | 350 (35.9) | 355 (36.4) | 351 (36.0) | 365 (37.4) | 341 (34.9) | 347 (35.6) | 359 (36.8) | 370 (37.9) | 336 (34.4) | |||||||||||
PAM50 (n=1,087) | <0.001 | <0.001 | <0.001 | <0.001 | <0.001 | <0.001 | |||||||||||||||||
Normal | 20 (1.8) | 20 (1.8) | 23 (2.1) | 17 (1.6) | 26 (2.4) | 14 (1.3) | 16 (1.5) | 24 (2.2) | 23 (2.1) | 17 (1.6) | 26 (2.4) | 14 (1.3) | |||||||||||
LumA | 297 (27.3) | 267 (24.6) | 403 (37.1) | 161 (14.8) | 254 (23.4) | 310 (28.5) | 328 (30.2) | 236 (21.7) | 257 (23.6) | 307 (28.2) | 265 (24.4) | 299 (27.5) | |||||||||||
LumB | 89 (8.2) | 117 (10.8) | 84 (7.7) | 122 (11.2) | 74 (6.8) | 132 (12.1) | 105 (9.7) | 101 (9.3) | 92 (8.5) | 114 (10.5) | 114 (10.5) | 92 (8.5) | |||||||||||
Her2 | 24 (2.2) | 58 (5.3) | 22 (2.0) | 60 (5.5) | 48 (4.4) | 34 (3.1) | 19 (1.7) | 63 (5.8) | 55 (5.1) | 27 (2.5) | 61 (5.6) | 21 (1.9) | |||||||||||
Basal | 113 (10.4) | 82 (7.5) | 11 (1.0) | 184 (16.9) | 141 (13.0) | 54 (5.0) | 75 (6.9) | 120 (11.0) | 116 (10.7) | 79 (7.3) | 77 (7.1) | 118 (10.9) |
Data are presented as n (%). ZIC2, zic family member 2; SLC7A5, solute carrier family 7 member 5; FOXJ1, forkhead box J1; CXCL9, C-X-C motif chemokine ligand 9; TNFRSF18, tumor necrosis factor receptor superfamily member 18; PRSS2, serine protease 2; L, low expression; H, high expression; PR, progesterone receptor; ER, estrogen receptor; HER2, human epidermal growth factor receptor 2; PAM50, prediction analysis of microarray 50.
The association of six genes with OS and DSS in breast cancer
The Kaplan-Meier survival analysis results indicated that patients exhibiting high expression levels of ZIC2 (Figure 2A) or SLC7A5 (Figure 2B) experienced significantly reduced OS relative to those with low expression levels of these genes. Furthermore, the analysis demonstrated that patients with elevated expression of ZIC2 (Figure 2A) or SLC7A5 (Figure 2B) also had reduced DSS compared to their counterparts with lower expression levels. Conversely, patients exhibiting high expression levels of FOXJ1 (Figure 2C), CXCL9 (Figure 2D), TNFRSF18 (Figure 2E), or PRSS2 (Figure 2F) demonstrated prolonged OS compared to those with low expression levels of these genes. Specifically, elevated expression of FOXJ1 (Figure 2C) or PRSS2 (Figure 2F) was associated with extended DSS compared to lower expression levels. However, the expression levels of CXCL9 (Figure 2D) and TNFRSF18 (Figure 2E) did not appear to be significantly associated with DSS.
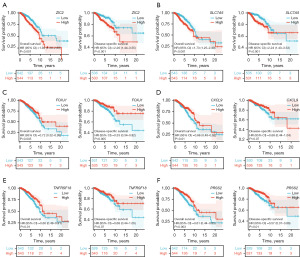
A nomogram model to predict OS and DSS for breast cancer
We developed a prognostic nomogram model for OS based on six prognostic factors. The expression levels of each indicator were used to calculate total points. Subsequently, a vertical line corresponding to the total points was drawn to illustrate the 1-, 5-, and 10-year OS survival probabilities for patients with breast cancer (Figure 3A). Prognostic calibration curves illustrated the discrepancy between the predicted probabilities and the observed probabilities of the model at 1- (Figure 3B), 5- (Figure 3C), and 10-year (Figure 3D) intervals for OS of patients with breast cancer. Statistical analyses (concordance index =0.692, 95% CI: 0.666–0.718; likelihood ratio test =58.84, P<0.001; Wald test =55.1, P<0.001) indicated that our prognostic model had good fit. The application of Cox regression was predicated on the assumption that the independent variables adhere to the proportional hazards assumption and that there was an absence of collinearity among the parameters. Analysis with the Cox univariate (Figure 4A) and multivariate (Figure 4B) proportional hazards model identified ZIC2 (HR =2.036; 95% CI: 1.293–3.205; P=0.002), SLC7A5 (HR =2.181; 95% CI: 1.378–3.452; P<0.001), FOXJ1 (HR =0.523; 95% CI: 0.335–0.817; P=0.004), and PRSS2 (HR =0.535; 95% CI: 0.344–0.833; P=0.006) as the independent risk factors for DSS in patients with breast cancer. We also developed a prognostic nomogram model for DSS using four prognostic factors. The expression levels of each indicator were used to calculate total points. Subsequently, a vertical line corresponding to the total points was drawn to illustrate the 1-, 5-, and 10-year DSS survival probabilities for patients with breast cancer (Figure 4C). Prognostic calibration curves illustrated the discrepancy between the predicted probabilities and the observed probabilities of the model at 1- (Figure 4D), 5- (Figure 4E), and 10-year (Figure 4F) intervals for DSS in patients with breast cancer. Furthermore, we developed risk factor maps for OS (Figure 5A) and DSS (Figure 5B) based on the prognostic indicators. Our findings suggested that elevated risk scores may be correlated with reduced survival duration.
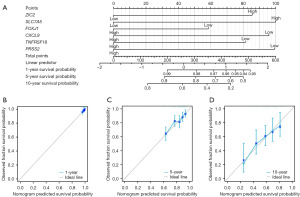
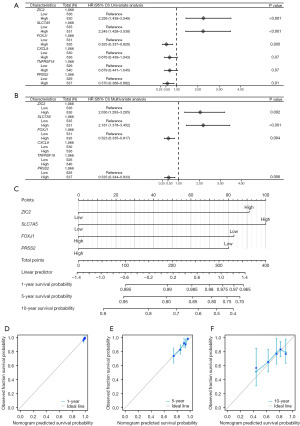
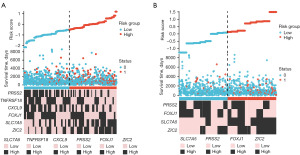
The association of prognostic indicators with immune infiltration in breast cancer
We conducted an in-depth examination of the relationship between prognostic indicators and immune infiltration in breast cancer. Using the ssGSEA algorithm (Figure 6A) and the CIBERSORT algorithm (Figure 6B), we analyzed the correlation between six prognostic indicators and immune cell populations within tumor tissues. Our findings revealed that all six prognostic indicators exhibited correlations with various immune cell types, with CXCL9 demonstrating the most significant association with immune cell infiltration. Via the ssGSEA algorithm, expression of CXCL9 was found to be significantly positively correlated with T cells (r=0.854; P<0.001), cytotoxic cells (r=0.767; P<0.001), and CD8 T cells (r=0.489; P<0.001) (Figure 6A). Furthermore, according to the CIBERSORT algorithm, CXCL9 expression exhibited positive correlations with activated memory CD4 T cells (r=0.631; P<0.001), M1 macrophages (r=0.629; P<0.001), and CD8 T cells (r=0.484; P<0.001) (Figure 6B).
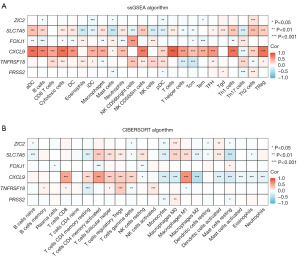
Ru et al. (30) conducted a comprehensive screening of major immunomodulators and classified them into three distinct categories: immunoinhibitors, immunostimulators, and major histocompatibility complex (MHC) molecules. The primary objective of this study was to assess the correlation between relevant genes and these immunomodulators (30). In our study, immunomodulators were employed to investigate the correlation between prognostic indicators and immunomodulators in breast cancer. The relationship between prognostic indicators and breast cancer immune infiltration was assessed at the molecular level. Notably, among the six prognostic indicators examined, CXCL9 exhibited a strong correlation with immunoinhibitors (Figure 7A), immunostimulators (Figure 7B), and MHC molecules (Figure 7C). These findings suggest that CXCL9 may play a significant role in the prognosis and efficacy evaluation of breast cancer immune infiltration and immunotherapy.
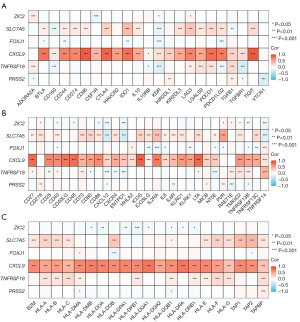
We subsequently conducted an in-depth investigation into the role of CXCL9 in breast cancer. Using the ssGSEA algorithm, we observed that the enrichment scores for CD8 T cells, cytotoxic cells, and natural killer (NK) CD56dim cells were significantly elevated in the CXCL9-high expression group compared to the CXCL9-low expression group (Figure 8A). Additionally, employing the CIBERSORT algorithm, we found that the enrichment scores for activated memory CD4 T cells, M1 macrophages, and CD8 T cells were markedly increased in the CXCL9-high expression group relative to the CXCL9-low expression group (Figure 8B). Subsequently, we used the TCGA database to identify CXCL9-related genes for GSEA (Figure 8C). Additionally, 178 CXCL9-related genes [|log2(fold change)| ≥2 and P.adj <0.05] were selected for GO (Figure 8D) and KEGG (Figure 8E) pathway analysis. Our findings indicated that CXCL9-related genes are predominantly involved in immune regulation, B-cell receptor signaling, NK cell-mediated cytotoxicity, and other immunoregulatory signaling pathways. These findings further indicated that CXCL9 may be integral to the immunomodulation and infiltration of immune cells within breast tissue. Consequently, CXCL9 may serve as a significant biomarker for assessing the prognosis and therapeutic efficacy of immunotherapy in breast cancer.
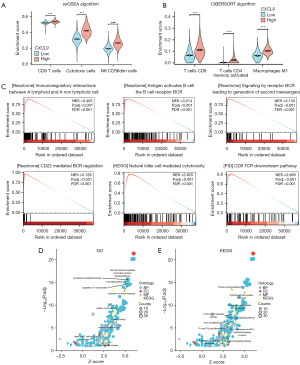
Discussion
In our study, we established a six-gene prognostic model for predicting the OS patients with breast cancer using multiple screening mechanisms, LASSO regression analysis, and Cox proportional hazards models. Notably, CXCL9 exhibited a significant correlation with CD8+ T cells, cytotoxic cells, and the M1 macrophage phenotype in breast cancer tissues. These immune cell types are generally recognized for their roles in tumor cell recognition and clearance, as well as their participation in antitumor immune responses (31,32). Therefore, it is plausible that CXCL9 may not only be a prognostic marker for breast cancer but also a biomarker for assessing the efficacy of immunotherapy in patients with breast cancer.
The phenotypes of immune cells play a crucial role in tumor development, prognosis, and therapeutic efficacy in breast cancer (33). Different immune cell phenotypes frequently mediate breast cancer progression through diverse mechanisms (33). Neutrophils are increasingly recognized as critical contributors to BC progression (34). Accumulating evidence suggests that neutrophils exhibit a dual role in BC by differentiating into either an anti-tumor (N1) or pro-tumor (N2) phenotype (35). Li et al. (36) incorporated four neutrophil-associated genes into the construction of a prognostic model centered on neutrophil activity. Subsequent multivariate Cox regression analysis demonstrated that this model serves as an independent prognostic factor for OS (36). The prognostic model developed based on tumor-associated neutrophil-related genes demonstrates outstanding predictive performance in patients with BC. Furthermore, this model is significantly associated with the prediction of survival outcomes, immune activity, and treatment response in BC patients (37). A study employing Mendelian randomization and meta-analysis demonstrated that CD28+CD4−CD8− T cells confer protection against breast cancer by enhancing immune surveillance, recognizing and eliminating tumor cells, and facilitating the secretion of cytokines, the activation of apoptotic pathways, and the stimulation of other immune cells, collectively augmenting the overall immune response (33). In one study, the presence of CD8+ TILs exhibiting a tissue-resident memory T-cell phenotype was significantly correlated with enhanced clinical outcomes in patients with TNBC undergoing treatment with checkpoint inhibitors (38). Although TILs exhibit prognostic and therapeutic predictive value, the precise function of distinct immune cell subsets remains a subject of ongoing debate. The infiltration of T cells and B cells is significantly correlated with a favorable prognosis in breast cancer, particularly within the HER2+ molecular subtype (39). Yang et al. (40) developed a prognostic scoring model incorporating M2 macrophages, CD8+ T cells, and memory CD4+ T cells. The model revealed that a high enrichment score of M2 macrophages correlated with a poor prognosis in TNBC. Conversely, elevated enrichment scores of CD8+ T cells and memory CD4+ T cells were associated with improved survival outcomes (40). Even without the assessment of PD-L1 protein, the quantification of TILs can predict the clinical efficacy of PD-1/PD-L1 inhibition (41). In patients with advanced TNBC and HER2-positive breast cancer, TIL cutoff values of 5% or 10%, when combined with PD-L1 expression evaluation, can identify immune-enriched tumors, thereby optimizing the clinical application of immunotherapy (41).
In addition to prognostic models predicated on immune cell scores, there exists a body of research on employing immune cell-related regulatory genes for the development of prognostic models in breast cancer (42,43). Li et al. (42) developed a prognostic macrophage marker gene signature (MMGS) comprising seven genes. A high score on the MMGS model was associated with poor survival (42). Another risk score model, derived from the expression profiles of five genes associated with NK cells, stratified patients with TNBC into high-risk and low-risk categories. Notably, patients classified within the low-risk group exhibited a more favorable clinical prognosis (43). Due to the limited functionality of individual immune cells in the pathogenesis of breast tumor progression, the prognostic prediction of breast cancer using single immune cell-related markers is susceptible to bias. Our research, however, is predicated on cancer immune-related genes without restriction on those associated with specific immune cells. These markers may be directly or indirectly involved in cancer immunity, thereby broadening the spectrum of markers available for screening. Ultimately, we identified six tumor immune-related genes—ZIC2, SLC7A5, FOXJ1, CXCL9, TNFRSF18, and PRSS2—for the development of a prognostic model for patients with breast cancer. Notably, the results of the correlation analysis indicated that CXCL9 was associated with antitumor immune cells, including CD8+ T cells, cytotoxic cells, M1 macrophages, activated memory CD4 T cells, and the enrichment of NK CD56dim cells. Furthermore, CXCL9 exhibited a significant negative association with the tumor-promoting M2 macrophage phenotype.
CXCL9 is a chemokine that plays a pivotal role in tumor immunity by recruiting immune cells, facilitating T-cell and NK infiltration, and regulating macrophage polarization within the tumor microenvironment (44-47). CXCL9 is a potential biomarker of immune infiltration and is correlated with favorable prognosis in ER-negative breast cancer (47). An early elevation in serum level of CXCL9 in patients with NK cell-rich tumors was found to be indicative favorable responses to anti-HER2 antibody-based neoadjuvant therapy (45). Cao et al. (48) found that high mRNA and protein expression of CXCL9 was associated with improved prognosis in patients with TNBC, which may be correlated with by immune cell infiltration (46). Consistent with these findings, our results indicated that elevated CXCL9 gene expression correlates with prolonged OS in patients with breast cancer. Concurrently, CXCL9 expression was associated with the enrichment of various antitumor immune cells in patients with breast cancer.
Although the development of a six-gene prognostic model for breast cancer produced encouraging results, it is important to acknowledge certain limitations of our study. Firstly, we are currently unable to ascertain the applicability of this model to breast cancer treatment outcomes, including chemotherapy, targeted therapy, and immunotherapy. Secondly, our prognostic model is derived from the TCGA database, which is limited by a relatively small sample size, potentially introducing bias in predicting the survival outcomes of patients with breast cancer. Thirdly, the relationship between primary and distant lesions and peripheral blood immune profiles in patients with breast cancer was not elucidated in our study. Knowing the relationship between primary and distant lesions and peripheral blood immune profiles might be points for further exploration. Finally, we did not conduct experimental validation to establish the correlation between these genes and immune cell infiltration. In subsequent research, we intend to investigate the potential of CXCL9 as a predictive biomarker for the efficacy of immunotherapy in breast cancer treatment.
Conclusions
We established a six-gene model for predicting breast cancer prognosis. Furthermore, we unexpectedly discovered that CXCL9 was significantly positively associated with the enrichment of antitumor immune cells and exhibited an excellent correlation with numerous immunoregulators. There is substantial evidence suggesting that CXCL9 is integral to immune infiltration in breast cancer and may thus serve as a critical biomarker for evaluating immune response and therapeutic efficacy in breast cancer treatment.
Acknowledgments
Funding: None.
Footnote
Reporting Checklist: The authors have completed the TRIPOD reporting checklist. Available at https://tcr.amegroups.com/article/view/10.21037/tcr-24-2137/rc
Peer Review File: Available at https://tcr.amegroups.com/article/view/10.21037/tcr-24-2137/prf
Conflicts of Interest: All authors have completed the ICMJE uniform disclosure form (available at https://tcr.amegroups.com/article/view/10.21037/tcr-24-2137/coif). M.T. is supported by the grants from Chugai, Takeda, Pfizer, Taiho, JBCRG association, KBCRN association, Eisai, Eli-Lilly and companies, Daiichi-Sankyo, AstraZeneca, Astellas, Shimadzu, Yakult, Nippon Kayaku, AFI technology, Luxonus, Shionogi, GL Science, Sanwa Shurui; receives lecture honoraria from Chugai, Takeda, Pfizer, Kyowa-Kirin, Taiho, Eisai, Daiichi-Sankyo, AstraZeneca, Eli Lilly and companies, MSD, Exact Science, Novartis, Shimadzu, Yakult, Nippon Kayaku, Devicore Medical Japan, Sysmex; holds leadership roles at Daiichi-Sankyo, Eli Lilly and companies, BMS, Bertis, Terumo, Kansai Medical Net, JBCRG Association, KBCRN Association, NPO org. OOTR, JBCS Association; and serves as Associate Editor in British Journal of Cancer, Scientific Reports, Breast Cancer Research and Treatment, Cancer Science, Asian Journal of Surgery, Asian Journal of Breast Surgery. The other authors have no conflicts of interest to declare.
Ethical Statement: The authors are accountable for all aspects of the work in ensuring that questions related to the accuracy or integrity of any part of the work are appropriately investigated and resolved. The study was conducted in accordance with the Declaration of Helsinki (as revised in 2013).
Open Access Statement: This is an Open Access article distributed in accordance with the Creative Commons Attribution-NonCommercial-NoDerivs 4.0 International License (CC BY-NC-ND 4.0), which permits the non-commercial replication and distribution of the article with the strict proviso that no changes or edits are made and the original work is properly cited (including links to both the formal publication through the relevant DOI and the license). See: https://creativecommons.org/licenses/by-nc-nd/4.0/.
References
- Zhao S, Chen DP, Fu T, et al. Single-cell morphological and topological atlas reveals the ecosystem diversity of human breast cancer. Nat Commun 2023;14:6796. [Crossref] [PubMed]
- Wu SZ, Al-Eryani G, Roden DL, et al. A single-cell and spatially resolved atlas of human breast cancers. Nat Genet 2021;53:1334-47. [Crossref] [PubMed]
- Xu L, Saunders K, Huang SP, et al. A comprehensive single-cell breast tumor atlas defines epithelial and immune heterogeneity and interactions predicting anti-PD-1 therapy response. Cell Rep Med 2024;5:101511. [Crossref] [PubMed]
- Yofe I, Shami T, Cohen N, et al. Spatial and Temporal Mapping of Breast Cancer Lung Metastases Identify TREM2 Macrophages as Regulators of the Metastatic Boundary. Cancer Discov 2023;13:2610-31. [Crossref] [PubMed]
- Sain B, Gupta A, Ghose A, et al. Clinico-Pathological Factors Determining Recurrence of Phyllodes Tumors of the Breast: The 25-Year Experience at a Tertiary Cancer Centre. J Pers Med 2023;13:866. [Crossref] [PubMed]
- Shiao SL, Gouin KH 3rd, Ing N, et al. Single-cell and spatial profiling identify three response trajectories to pembrolizumab and radiation therapy in triple negative breast cancer. Cancer Cell 2024;42:70-84.e8. [Crossref] [PubMed]
- Rayson VC, Harris MA, Savas P, et al. The anti-cancer immune response in breast cancer: current and emerging biomarkers and treatments. Trends Cancer 2024;10:490-506. [Crossref] [PubMed]
- Song F, Tarantino P, Garrido-Castro A, et al. Immunotherapy for Early-Stage Triple Negative Breast Cancer: Is Earlier Better? Curr Oncol Rep 2024;26:21-33. [Crossref] [PubMed]
- Ding XH, Xiao Y, Chen F, et al. The HLA-I landscape confers prognosis and antitumor immunity in breast cancer. Brief Bioinform 2024;25:bbae151. [Crossref] [PubMed]
- Cortes J, Rugo HS, Cescon DW, et al. Pembrolizumab plus Chemotherapy in Advanced Triple-Negative Breast Cancer. N Engl J Med 2022;387:217-26. [Crossref] [PubMed]
- Schmid P, Cortes J, Dent R, et al. Event-free Survival with Pembrolizumab in Early Triple-Negative Breast Cancer. N Engl J Med 2022;386:556-67. [Crossref] [PubMed]
- Loibl S, Schneeweiss A, Huober J, et al. Neoadjuvant durvalumab improves survival in early triple-negative breast cancer independent of pathological complete response. Ann Oncol 2022;33:1149-58. [Crossref] [PubMed]
- Zhang P, Yang J, Zhong X, et al. A novel PD-1/PD-L1 pathway-related seven-gene signature for the development and validation of the prognosis prediction model for breast cancer. Transl Cancer Res 2024;13:1554-66. [Crossref] [PubMed]
- Chen S, Wang RX, Liu Y, et al. PD-L1 expression of the residual tumor serves as a prognostic marker in local advanced breast cancer after neoadjuvant chemotherapy. Int J Cancer 2017;140:1384-95. [Crossref] [PubMed]
- Liu L, Shen Y, Zhu X, et al. ERα is a negative regulator of PD-L1 gene transcription in breast cancer. Biochem Biophys Res Commun 2018;505:157-61. [Crossref] [PubMed]
- Schalper KA, Velcheti V, Carvajal D, et al. In situ tumor PD-L1 mRNA expression is associated with increased TILs and better outcome in breast carcinomas. Clin Cancer Res 2014;20:2773-82. [Crossref] [PubMed]
- Vranic S, Cyprian FS, Gatalica Z, et al. PD-L1 status in breast cancer: Current view and perspectives. Semin Cancer Biol 2021;72:146-54. [Crossref] [PubMed]
- Ganatra H, Tan JK, Simmons A, et al. Applying whole-genome and whole-exome sequencing in breast cancer: a review of the landscape. Breast Cancer 2024;31:999-1009. [Crossref] [PubMed]
- Herzog SK, Fuqua SAW. ESR1 mutations and therapeutic resistance in metastatic breast cancer: progress and remaining challenges. Br J Cancer 2022;126:174-86. [Crossref] [PubMed]
- Williams MM, Spoelstra NS, Arnesen S, et al. Steroid Hormone Receptor and Infiltrating Immune Cell Status Reveals Therapeutic Vulnerabilities of ESR1-Mutant Breast Cancer. Cancer Res 2021;81:732-46. [Crossref] [PubMed]
- Angelico G, Broggi G, Tinnirello G, et al. Tumor Infiltrating Lymphocytes (TILS) and PD-L1 Expression in Breast Cancer: A Review of Current Evidence and Prognostic Implications from Pathologist's Perspective. Cancers (Basel) 2023;15:4479. [Crossref] [PubMed]
- Berger AC, Korkut A, Kanchi RS, et al. A Comprehensive Pan-Cancer Molecular Study of Gynecologic and Breast Cancers. Cancer Cell 2018;33:690-705.e9. [Crossref] [PubMed]
- Liu J, Lichtenberg T, Hoadley KA, et al. An Integrated TCGA Pan-Cancer Clinical Data Resource to Drive High-Quality Survival Outcome Analytics. Cell 2018;173:400-416.e11. [Crossref] [PubMed]
- Yoshihara K, Shahmoradgoli M, Martínez E, et al. Inferring tumour purity and stromal and immune cell admixture from expression data. Nat Commun 2013;4:2612. [Crossref] [PubMed]
- Bindea G, Mlecnik B, Tosolini M, et al. Spatiotemporal dynamics of intratumoral immune cells reveal the immune landscape in human cancer. Immunity 2013;39:782-95. [Crossref] [PubMed]
- Chen B, Khodadoust MS, Liu CL, et al. Profiling Tumor Infiltrating Immune Cells with CIBERSORT. Methods Mol Biol 2018;1711:243-59. [Crossref] [PubMed]
- Newman AM, Liu CL, Green MR, et al. Robust enumeration of cell subsets from tissue expression profiles. Nat Methods 2015;12:453-7. [Crossref] [PubMed]
- Yu G, Wang LG, Han Y, et al. clusterProfiler: an R package for comparing biological themes among gene clusters. OMICS 2012;16:284-7. [Crossref] [PubMed]
- Walter W, Sánchez-Cabo F, Ricote M. GOplot: an R package for visually combining expression data with functional analysis. Bioinformatics 2015;31:2912-4. [Crossref] [PubMed]
- Ru B, Wong CN, Tong Y, et al. TISIDB: an integrated repository portal for tumor-immune system interactions. Bioinformatics 2019;35:4200-2. [Crossref] [PubMed]
- Li M, Yang Y, Xiong L, et al. Metabolism, metabolites, and macrophages in cancer. J Hematol Oncol 2023;16:80. [Crossref] [PubMed]
- Reina-Campos M, Scharping NE, Goldrath AW. CD8(+) T cell metabolism in infection and cancer. Nat Rev Immunol 2021;21:718-38. [Crossref] [PubMed]
- Xu W, Zhang T, Zhu Z, et al. The association between immune cells and breast cancer: insights from mendelian randomization and meta-analysis. Int J Surg 2024; Epub ahead of print. [Crossref] [PubMed]
- Yang C, Li L, Ye Z, et al. Mechanisms underlying neutrophils adhesion to triple-negative breast cancer cells via CD11b-ICAM1 in promoting breast cancer progression. Cell Commun Signal 2024;22:340. [Crossref] [PubMed]
- Gong YT, Zhang LJ, Liu YC, et al. Neutrophils as potential therapeutic targets for breast cancer. Pharmacol Res 2023;198:106996. [Crossref] [PubMed]
- Li S, Qian Y, Xie W, et al. Identification and validation of neutrophils-related subtypes and prognosis model in triple negative breast cancer. J Cancer Res Clin Oncol 2024;150:149. [Crossref] [PubMed]
- Zhang J, Wang X, Zhang Z, et al. A novel tumor-associated neutrophil gene signature for predicting prognosis, tumor immune microenvironment, and therapeutic response in breast cancer. Sci Rep 2024;14:5339. [Crossref] [PubMed]
- Virassamy B, Caramia F, Savas P, et al. Intratumoral CD8(+) T cells with a tissue-resident memory phenotype mediate local immunity and immune checkpoint responses in breast cancer. Cancer Cell 2023;41:585-601.e8. [Crossref] [PubMed]
- Myllys M. Highlight report: Relevance of T-cells, B-cells and immune checkpoint factors for prognosis of breast cancer. EXCLI J 2019;18:253-5. [PubMed]
- Yang A, Wu M, Ni M, et al. A risk scoring system based on tumor microenvironment cells to predict prognosis and immune activity in triple-negative breast cancer. Breast Cancer 2022;29:468-77. [Crossref] [PubMed]
- Loi S, Michiels S, Adams S, et al. The journey of tumor-infiltrating lymphocytes as a biomarker in breast cancer: clinical utility in an era of checkpoint inhibition. Ann Oncol 2021;32:1236-44. [Crossref] [PubMed]
- Li Y, Zhao X, Liu Q, et al. Bioinformatics reveal macrophages marker genes signature in breast cancer to predict prognosis. Ann Med 2021;53:1019-31. [Crossref] [PubMed]
- Liu Z, Ding M, Qiu P, et al. Natural killer cell-related prognostic risk model predicts prognosis and treatment outcomes in triple-negative breast cancer. Front Immunol 2023;14:1200282. [Crossref] [PubMed]
- Bill R, Wirapati P, Messemaker M, et al. CXCL9:SPP1 macrophage polarity identifies a network of cellular programs that control human cancers. Science 2023;381:515-24. [Crossref] [PubMed]
- Santana-Hernández S, Suarez-Olmos J, Servitja S, et al. NK cell-triggered CCL5/IFNγ-CXCL9/10 axis underlies the clinical efficacy of neoadjuvant anti-HER2 antibodies in breast cancer. J Exp Clin Cancer Res 2024;43:10. [Crossref] [PubMed]
- Cao X, Song Y, Wu H, et al. C-X-C Motif Chemokine Ligand 9 Correlates with Favorable Prognosis in Triple-Negative Breast Cancer by Promoting Immune Cell Infiltration. Mol Cancer Ther 2023;22:1493-502. [Crossref] [PubMed]
- Liang YK, Deng ZK, Chen MT, et al. CXCL9 Is a Potential Biomarker of Immune Infiltration Associated With Favorable Prognosis in ER-Negative Breast Cancer. Front Oncol 2021;11:710286. [Crossref] [PubMed]
- Cao X, Song Y, Wu H, et al. Quantifying spatial CXCL9 distribution with image analysis predicts improved prognosis of triple-negative breast cancer. Front Genet 2024;15:1421573. [Crossref] [PubMed]
(English Language Editor: J. Gray)