Multi-slice computed tomography radiomics combined with serum alpha-L-fucosidase: a potential biomarker for precise identification of pleomorphic adenoma and Warthin tumor
Introduction
The incidence rate of salivary gland tumors is increasing every year, most of which occur in parotid glands. The vast majority of parotid gland tumors are pleomorphic adenoma (PMA) and Warthin tumor (WT) (1). The currently applied standard of treatment for PMA is superficial parotidectomy or partial superficial parotidectomy, while WT is treated with extracapsular dissection (2). Extracapsular dissection is not appropriate for PMA because it increases the likelihood of postoperative recurrence. Therefore, it is of great significance to clearly distinguish PMA from WT before surgery.
Radiological examination plays a pivotal role in the preoperative diagnosis of parotid gland tumors (3,4). Multi-slice computed tomography (MSCT) is being increasingly used in the clinical setting due to its advantages of low cost, shorter operation time, and the ability to clearly visualize the location of tumor in relation to surrounding soft and hard tissues, as well as important blood vessels (5). Physicians ordinarily make a qualitative diagnosis of parotid tumors on radiological images through visual analysis. Such subjective assessment is prone to misdiagnosis. In recent years, radiomics has recently gained interest as a research subject due to the innovation in medical imaging diagnostics brought about by advances in computer technology. Based on artificial intelligence, radiomics can automatically extract repeatable image features that far exceed those obtained by physicians’ visual analysis and transform them into high-dimensional data to evaluate, which effectively compensates for the shortcoming of traditional diagnosis depending solely on visual analysis of radiological images (6). Radiomics was first presented in 2012 by the Dutch scholar Lambin, who considered that the spatiotemporal heterogeneous of solid tumors can be exhibited by modern medical imaging, i.e., high-resolution imaging devices can capture both intra-tumoral features and instant pathophysiological information in a non-invasive way, and thus he defined radiomics as the process of high-throughput extraction of image features from radiographic images (7). Radiomics belongs to a typical medical-industrial interdisciplinary field, with five essential steps: image acquisition, image segmentation, feature extraction, feature screening, and model development. In recent years, radiomics have been demonstrated for the diagnosis of many types of cancer, including lung cancer and liver cancer (8). In oral diseases, radiomics can be used for diagnosis of jaw cysts, prognosis analysis of head and neck cancer, and prediction analysis of dry mouth symptoms after radiotherapy. Furthermore, several studies have shown that radiomics can be utilized for preoperative diagnosis of parotid tumors. However, there remains a research gap in identifying parotid tumors using a combination of radiomics and biochemical markers.
Alpha-L-fucosidase (AFU), a lysosomal acid hydrolase found in abundance in liver and kidney tissue cells, is mainly engaged in the catabolism of macromolecular substances such as various glycolipids of fucose and glycoprotein (9). Since Deugnier discovered that serum AFU levels were higher in patients with primary liver cancer in 1980 (10), the relationship between serum AFU and tumor has been extensively researched. Serum AFU level was lower in a range of cancer patients, including ovarian cancer and colorectal cancer, and low serum AFU expression was generally associated with high malignancy grade and poor prognosis (11,12). However, a study by Shah et al. showed that serum AFU activity was positively correlated with the severity of oral cancer (13). PMA is a benign tumor that is prone to recurrence and has potential for malignant transformation. To date, no published studies have investigated serum AFU levels in patients with PMA and WT, indicating a notable gap in the literature.
The aim of this study was to develop a logistic regression model combining MSCT radiomics and serum AFU to assess the accuracy of distinguishing PMA from WT for assisting in clinic decision-making. We present this study in accordance with the TRIPOD reporting checklist (14) (available at https://tcr.amegroups.com/article/view/10.21037/tcr-24-871/rc).
Methods
Figure 1 illustrates the flow chart of this study.
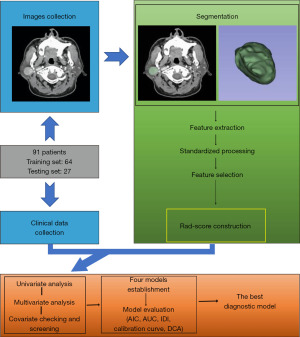
Patients
Preoperative enhanced MSCT images, age, gender, and required biochemical indexes [glutamate oxalyl acetate transaminase (AST)/glutamate pyruvate transaminase (ALT), X-protein/globin (X/G), AFU, urea/Cr (U/C), uric acid (UA), high density lipoprotein (HDL), and lactate dehydrogenase (LDH)] were collected from 91 patients with PMA or WT who had parotid tumor resection between December 2017 and December 2021. Exclusion criteria: (I) history of tumor; (II) preoperative radiotherapy or chemotherapy; (III) recurrent parotid tumor; (IV) no surgical treatment or unclear pathological results; and (V) all types of hepatitis.
These patients were randomized into a training cohort and a validation cohort at a ratio of 7:3 (64 vs. 27). The institutional ethics committee of the First Hospital of Lanzhou University approved this study (No. LDYYLL2022-374). The need for informed consent was waived because of the retrospective nature of the study. This study was conducted in accordance with the Declaration of Helsinki (as revised in 2013).
MSCT images acquisition
All patients underwent a preoperative examination by a dual-source 64-row MSCT scanner (SIEMENS, Erlangen, Germany) with following scanning parameters: tube voltage, 110 kVp; tube current, 130 mAs; matrix, 350×350; gantry rotation time, 0.5 s; pitch, 0.98; collimation, 24 mm × 1.2 mm; scanning slice thickness, 5 mm; reconstruction slice thickness, 1 mm; scanning range, skull base to cervical root. Ioversol was used as the contrast medium with a specification of 50 mL:33.9 g (320 mg iodine per 1 mL), injected through the elbow vein at a rate of 3 mL/s, and the arterial phase images were scanned 30 s after the injection. All images were acquired according to the existing quality control standards and saved in Digital Imaging and Communications in Medicine (DICOM) format.
Image segmentation
Image segmentation includes outlining a lesion from a computed tomography (CT) image, separating it from the surrounding normal tissue, and forming a region of interest (ROI) (15). In this study, two radiologists with more than ten years of experience in head and neck imaging manually outlined the ROI of tumors on each slice of arterial phase images to generate a three-dimensional ROI (Figure 2) using 3D Slicer software (version 4.13; http://www.slicer.org). In order to avoid the surrounding normal tissues from being outlined, the two radiologists must draw along the inner 1 mm of the tumor boundary.
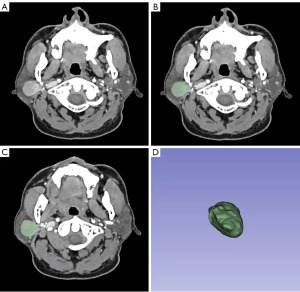
Feature extraction
The PyRadiomics software package in Python (version 3.9; http://www.python.org) was used to extract radiomics features from each three-dimensional ROI (16). The features were then standardized via Z-score standardization to minimize the influence on the outcomes of subsequent data analysis caused by significant discrepancies in value between features.
Inter- and intra-observer reproducible assessment
To assess intra-observer repeatability, each radiologist followed the same procedure to generate radiomics features twice. Intra-and-inter class correlation (ICC) was used to evaluate the intra- and inter-observer agreement in feature extraction. ICC >0.8 represented good repeatability (17).
Feature screening and calculation of Rad-score
The extracted features with ICC >0.8 in the training cohort were screened using the least absolute shrinkage and selection operator (LASSO) and 5-fold cross-validation. With the addition of penalty parameters to the linear regression model, LASSO, which is based on the least square approach, is used to compress the regression coefficients of variables and some of which may even be compressed to zero. As a consequence, the variables that had a bigger influence on the findings are screened out, and a simpler model is created (18). The regression coefficients are determined by minimizing the following functional equation:
is the penalty parameter of the objective function, λ is the penalty parameter coefficient, and is the L1 norm of the regression coefficient β, denoting the sum of the absolute values of all regression coefficients. λ determines the penalty strength of the regression coefficients and controls the goodness-of-fit of the model. λ can be determined by 5-fold cross-validation, and the value of λ that ultimately maximizes the area under the curve (AUC) of the model is the optimal value. The screened features and their corresponding regression coefficients will be obtained once the optimal λ has been determined. Then, the Rad-score for each patient could be calculated by a linear equation consisting of the screened features multiplied by the corresponding regression coefficients.
Model establishment
In the training cohort, the collected variables (AST/ALT, X/G, AFU, U/C, UA, HDL, LDH, gender, and age) and Rad-score were subjected to a univariate logistic regression analysis. Variables with P<0.05 were then used as confounders, and Rad-score as an exposure variable for covariate checking and screening. The confounders that changed the estimate of Rad-score on diagnostic outcomes by more than 10% or were significantly associated with diagnostic outcomes were selected (19). These selected variables were subjected to a multivariate logistic regression analysis along with Rad-score to obtain variables with P<0.05. Four diagnostic models were then created using these variables via logistic regression model. As a multivariate analysis method to study the relationship between dichotomous outcomes and some influencing factors, logistic regression model has the objective function as follows (20,21):
P is the probability of the event occurring, is the intercept, is the regression coefficient of the independent variable . When Y varies between , P varies between 0 and 1.
Comparison of diagnostic performance and goodness-of-fit
The validation cohort was used to verify the performance of the four models. The Akaike’s information criterion (AIC) as an international standard was used to measure the complexity and goodness-of-fit of the models. The smaller the AIC, the lesser the complexity and the greater the goodness-of-fit (22). To assess the models’ diagnostic performance, we compared the receiver operating characteristic (ROC) curve, the AUC, accuracy, sensitivity, specificity, positive predictive value, and negative predictive value. The ROC curve was mainly to evaluate the diagnostic performance of dichotomous models. In general, the more convex the curve to the upper left corner, the higher the diagnostic capability. The AUC, whose value ranges from 0.5 to 1, is the area enclosed by the ROC curve and the horizontal and vertical coordinates. Models with AUC values between 0.7 and 0.8 are often considered acceptable, while values between 0.8 and 0.9 indicate moderate diagnostic ability and greater than 0.9 implies excellent diagnostic ability (23). Then, the calibration plot accompanied by Hosmer-Lemeshow test was drawn to evaluate models’ goodness-of-fit. Finally, the best model was chosen.
Evaluation of clinical application value
The decision curve analysis (DCA) was plotted to assess the clinical application value of the best model (24). And the nomogram was drawn for the clinical work.
Statistical analysis
All statistical analyses were performed by R software (version 3.6.3; http://www.r-project.org) (25). Categorical variables were compared by Chi-square test. Continuous variables were first tested by the Kolmogorov-Smimov test for conformity to the normal distribution. Student’s t test, analysis of variance (ANOVA), or Mann-Whitney U test was then used for comparison between groups in the corresponding conditions. P<0.05 was considered to be statistically significant.
Results
Patient characteristics
We collected the baseline characteristics (Table 1) of the patients in the training and validation cohorts. The training cohort contained 47 PMA and 17 WT cases. Female number and U/C were significantly higher among PMA cases than in WT cases. Male number, AFU, UA, HDL, LDH, and age were significantly higher among WT cases than in PMA cases (P<0.05).
Table 1
Clinical factors | Training cohort (n=64) | Validation cohort (n=27) | P value |
---|---|---|---|
AST/ALT | 1.13 (0.86–1.57) | 0.87 (0.71–1.31) | 0.02* |
X/G | 1.70 (1.50–1.90) | 1.60 (1.50–1.80) | 0.52 |
AFU (U/L) | 18.00 (15.00–22.00) | 24.00 (21.00–34.00) | <0.001* |
U/C | 77.50 (63.00–90.75) | 71.00 (62.50–84.00) | 0.85 |
UA (μmol/L) | 304.00 (246.00–376.50) | 339.00 (263.00–395.00) | 0.28 |
HDL (mmol/L) | 1.08 (0.98–1.29) | 1.04 (0.94–1.20) | 0.30 |
LDH (U/L) | 173.50 (154.75–201.50) | 174.00 (162.00–196.00) | 0.90 |
Age (years) | 51.00 (40.75–59.00) | 51.00 (41.00–56.00) | 0.89 |
Diagnosis | 0.76 | ||
PMA | 47 (73.44) | 19 (70.37) | |
WT | 17 (26.56) | 8 (29.63) | |
Gender | 0.91 | ||
Male | 34 (53.12) | 14 (51.85) | |
Female | 30 (46.88) | 13 (48.15) |
Continuous data are presented as median (interquartile range), while categorical data are presented as counts (percentages). *, P<0.05. AST/ALT, glutamate oxalyl acetate transaminase/glutamate pyruvate transaminase; X/G, X-protein/globin; AFU, alpha-L-fucosidase; U/C, urea/Cr; UA, uric acid; HDL, high density lipoprotein; LDH, lactate dehydrogenase; PMA, pleomorphic adenomas; WT, Warthin tumors.
The validation cohort included 19 PMA cases and 8 WT cases. Similar to the training group, AFU, Age and male number were significantly higher in WT cases. However, different from the training cohort, there was no statistically significant change in U/C, UA, HDL, or LDH between PMA and WT patients. Additionally, in both cohorts, the difference in AST/ALT and X/G between PMA and WT was not significant.
Feature extraction, screening, and calculation of Rad-score
A total of 112 features with ICC >0.8 were extracted from each three-dimensional ROI, including 19 morphological features (the largest three-dimensional diameter, surface area, volume, shape, voxel number, etc.), 18 grayscale histogram features (skewness, uniformity, mean, median, energy, etc.), and 75 texture features [Grey Level Co-occurrence Matrix (GLCM), Gray Level Size Zone Matrix (GLSZM), Gray Level Run-length Matrix (GLRLM), Neighbourhood Gray-tone Difference Matrix (NGTDM), Grey Level Dependence Matrix (GLDM)] (26).
LASSO regression results show that, as the value of λ increased, the absolute values of the regression coefficients of all features gradually approach 0 and stabilize when λ is around −3 (Figure 3A). 5-fold cross-validation results (Figure 3B) show that when the minimum value (0.080) is taken for λ, the AUC is the largest and 5 features are screened out. The AUCs of the LASSO regression model in the training and validation cohorts are 0.8116 and 0.8388, respectively, indicating that the model has good diagnostic performance (Figure 4). The 5 features and their corresponding regression coefficients are shown in Table 2. The Rad-score for each patient is calculated via the following equation:
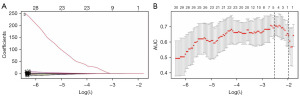
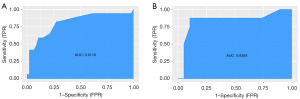
Table 2
Features | Coefficients |
---|---|
Intercept | −1.083 |
Diagnostics_Image.original_Mean | 0.104 |
Original_shape_Flatness | −0.124 |
Original_shape_Maximum3DDiameter | 0.076 |
Original_firstorder_Median | 0.417 |
Original_glszm_LargeAreaLowGrayLevelEmphasis | −0.109 |
Univariate and multivariate logistic regression analysis
Univariate analysis results (Table 3) showed that AFU, U/C, UA, HDL, LDH, Rad-score, gender, and Age were significantly associated with the identification of PMA and WT (P<0.05). The odds ratio (OR) revealed that U/C, HDL, and gender were risk factors, AFU, UA, LDH, Rad-score, and age were protective factors. The risk of a parotid tumor being WT increased by 1.05 times for every year of age increase, by 1.15 times for each 1 U/L increase in AFU, by 1.01 times for each 1 µmol/L increase in UA, by 1.02 times for each 1 U/L increase in LDH, and by 12.76 times for each increase in Rad-score. Furthermore, the risk of WT in men was 25 times greater than in women. Covariate checking and screening and multivariate logistic regression analysis results (Table 3) showed only AFU, Rad-score, and gender were independent diagnostic factors for distinguishing PMA from WT (P<0.05).
Table 3
Variables | Univariate analysis | Multivariate analysis | |||||
---|---|---|---|---|---|---|---|
OR | 95% CI | P value | OR | 95% CI | P value | ||
AST/ALT | 0.86 | 0.27–2.74 | 0.79 | NA | |||
X/G | 1.61 | 0.17–15.45 | 0.68 | NA | |||
AFU (U/L) | 1.15 | 1.03–1.28 | 0.01* | 1.18 | 1.02–1.38 | 0.03* | |
U/C | 0.96 | 0.93–0.99 | 0.01* | NA | |||
UA (μmol/L) | 1.01 | 1.00–1.01 | 0.01* | 1.01 | 1.00–1.01 | 0.17 | |
HDL (mmol/L) | 0.05 | 0.00–0.90 | 0.04* | NA | |||
LDH (U/L) | 1.02 | 1.00–1.04 | 0.04* | NA | |||
Rad-score | 12.76 | 3.03–53.81 | 0.001* | 74.34 | 4.93–1,120.13 | 0.002* | |
Gender | 0.04 | 0.00–0.32 | 0.003* | 0.05 | 0.00–0.88 | 0.04* | |
Age | 1.05 | 1.00–1.09 | 0.03* | NA |
*, P<0.05. CI, confidence interval; AST/ALT, glutamate oxalyl acetate transaminase/glutamate pyruvate transaminase; OR, odds ratio; X/G, X-protein/globin; AFU, alpha-L-fucosidase; U/C, urea/Cr; UA, uric acid; HDL, high density lipoprotein; LDH, lactate dehydrogenase; NA, not applicable.
The establishment of four models
Four diagnostic models were established based on AFU, gender, or Rad-score via logistic regression model (Table 4). The formulas of four models were shown as follows:
Table 4
Variables | Model A | Model B | |||||||
---|---|---|---|---|---|---|---|---|---|
Coefficient | 95% CI | P value | AIC | Coefficient | 95% CI | P value | AIC | ||
Model 1 | 61.75 | 59.76 | |||||||
AFU | – | – | – | 0.083 | 0.964–1.224 | 0.17 | |||
Gender | – | – | – | −2.992 | 0.006–0.424 | 0.006* | |||
Rad-score | 2.546 | 3.424–63.350 | <0.001* | – | – | – | |||
Model 2 | 46.50 | 43.33 | |||||||
AFU | – | – | – | 0.160 | 1.016–1.356 | 0.03* | |||
Gender | −3.843 | 0.001–0.176 | 0.003* | −3.590 | 0.001–0.273 | 0.01* | |||
Rad-score | 3.187 | 3.271–179.151 | 0.002* | 3.681 | 4.260–369.867 | 0.001* |
Model A1, (Rad-score); model A2, (Rad-score, gender); model B1, (AFU, gender); model B2, (AFU, Rad-score, gender) (27). *, P<0.05. AFU, alpha-L-fucosidase; AIC, Akaike’s information criterion; CI, confidence interval.
These formulas also applied to the validation cohort.
Evaluation on diagnostic performance and goodness-of-fit of models
Table 4 shows the AIC of model B2 is the smallest. ROC curves in the training and validation cohorts (Figure 5A,5B) shows the ROC curve of model B2 is most convex to the upper left corner. Table 5 shows in both cohorts, the AUC sizes of the four models, ranked in descending order, are as follows: model B2 > model A2 > model B1 > model A1. The accuracies of model A2 (0.906) and B2 (0.906) are greater than that of model A1 (0.781) and model B1 (0.781) in the training cohort. In the validation cohort, the accuracies of model A1, A2, and B1 are all 0.889, which are lower than that of model B2 (0.963), indicating that model A2 and B2 had higher diagnostic performance than the other two models, and model B2 had the greatest. The calibration plots of model A2 and B2 (Figure 5C,5D) shows that the calibration curve of model B2 is closer to the diagonal dotted line, indicating the predicting effect of model B2 is better than that of model A2. Hosmer-Lemeshow test shows model B2 (χ2=0.055, df =2, P=0.75) has better goodness-of-fit than that of model A2 (χ2=4.123, df =2, P=0.12). Thus, model B2 performs best among the four models.
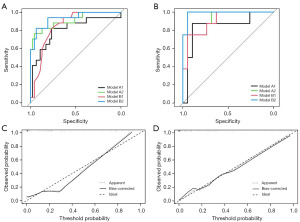
Table 5
Model | AUC (95% CI) | Specificity | Sensitivity | Best threshold | Accuracy | Positive-pv | Negative-pv |
---|---|---|---|---|---|---|---|
Training cohort | |||||||
A1 | 0.807 (0.670–0.945) | 0.766 | 0.824 | −0.988 | 0.781 | 0.560 | 0.923 |
A2 | 0.910 (0.820–1.000) | 0.957 | 0.765 | −3.861 | 0.906 | 0.867 | 0.918 |
B1 | 0.848 (0.754–0.942) | 0.766 | 0.824 | −3.450 | 0.781 | 0.560 | 0.923 |
B2 | 0.934 (0.863–1.000) | 0.936 | 0.824 | −4.060 | 0.906 | 0.824 | 0.936 |
Validation cohort | |||||||
A1 | 0.842 (0.649–1.000) | 0.895 | 0.875 | −0.578 | 0.889 | 0.778 | 0.944 |
A2 | 0.908 (0.794–1.000) | 0.947 | 0.750 | −3.764 | 0.889 | 0.857 | 0.900 |
B1 | 0.901 (0.780–1.000) | 0.947 | 0.750 | −2.414 | 0.889 | 0.857 | 0.900 |
B2 | 0.987 (0.956–1.000) | 0.947 | 1.000 | −3.587 | 0.963 | 0.889 | 1.000 |
Model A1, (Rad-score); model A2, (Rad-score, gender); model B1, (AFU, gender); model B2, (AFU, Rad-score, gender) (27). AUC, area under the curve; CI, confidence interval; Positive-pv, positive predict value; Negative-pv, negative predict value.
Evaluation of clinical value of model B2
The nomogram of model B2 is illustrated in Figure 6A. The best threshold of the nomogram in the training cohort was 0.385 (Table 5), then the best probability value P was calculated via Eq. [5]:

As a result, when the rate of WT calculated by the nomogram was greater than 0.595, the tumor could be diagnosed as WT.
The DCA curves of four models in the training and validation cohorts (Figure 6B,6C) shows that within the threshold probability (10–60%), all models are available, and the net benefit of model B2 was greater than that of the others in the validation cohort, indicating that model B2 had the highest clinical application value.
Discussion
The most common benign tumors of parotid glands are PMA and WT (28), the former is more prone to malignant transformation and recurrence than the latter, resulting in significant differences in surgical therapies (1). Therefore, accurate differentiation of PMA from WT is vital for the prognosis of patient. Ultrasound, as the preferred method of body surface imaging, plays an important role in the differential diagnosis of parotid masses. However, ultrasound requires the operators to have possess a high level of examination skills and it is difficult to assess the nature of lesions in deeper sites. In addition to ultrasound, other imaging examinations such as CT and magnetic resonance imaging (MRI) are also widely used in the preoperative diagnosis of parotid tumors. Among different examination methods, CT is minimally invasive, affordable, low-risk, and can locate the three-dimensional position of the mass and analyze the relationship between the mass and the surrounding tissues, which is becoming the main choice for preoperative examination of parotid tumors in China (29). CT has been proven to have a high degree of accuracy in identifying parotid tumors, but the majority of this evidence has relied on the visual assessment of qualitative or semi-quantitative features such as tumor morphology, border, location, diameter, and density values in the images, which is a highly subjective approach that may lead to misdiagnosis (2). In contrast, the diagnostic model we developed is based on objective variables such as serum AFU, gender, and Rad-score. In this retrospective study, we developed and validated a MSCT-based radiomics model that integrates AFU and gender for the differentiation of PMA from WT. The presented radiomics model showed powerful discrimination efficacy in both the training cohort (AUC 0.934) and the validation cohort (AUC 0.987).
Clinically, we found a significant difference in the values of some blood examination indices, especially AFU, between PMA and WT patients. Serum AFU is a common blood examination index that is currently used to assess liver function. Previous research has shown that serum AFU levels were significantly associated with favorable progression-free survival in breast cancer patients treated with trastuzumab, and therefore serum AFU may be considered as a biomarker for predicting trastuzumab sensitivity (30). In addition, serum AFU levels were found to be significantly different in patients with oral precancerous lesions and oral cancer compared to normal subjects, suggesting that serum AFU could be used as a biomarker for early oral cancer screening and tracking therapy effectiveness (31). However, studies on serum AFU levels in patients with parotid tumors have not been reported. Therefore, in this study, we analyzed these blood examination indices (Table 1) by univariate and multivariate logistic regression analysis, and the results showed that only AFU was an independent diagnostic factor in the differentiation between PMA and WT. We found that patients with PMA had lower serum AFU levels than patients with WT, and the difference was statistically significant (Table 3). Considering that PMA has the property of being susceptible to malignant transformation and recurrence, we believe that serum AFU levels can be used as a biomarker to predict the probability of recurrence or malignant transformation in PMA (32).
Radiomics is a hotspot of current research as an emerging technology (33). In about 10 years since it was initially presented in 2012, radiomics has been demonstrated to be useful for the prediction of preoperative lymph node metastasis and vascular infiltration in cancer patients (34), as well as the prognosis of cancer and cerebral hemorrhage patients (35). In this study, we screened 112 extracted radiomics features, but only 5 of them—3 morphological features and 2 textural features—were ultimately connected to the differentiation of PMA and WT. The remaining features were redundant or unrelated, which is consistent with the findings of previous studies. The large difference in textural features between PMA and WT may be due to the fact that the former is mainly composed of glandular duct-like, mucus-like, or cartilaginous tissue, whereas the latter is mainly composed of glandular epithelial and lymphatic tissue, and the latter has a slightly higher probability of cystic degeneration.
Herein, we developed and validated a prediction model based on AFU, gender and Rad-score for differentiating PMA and WT. The diagnostic performance of this model was superior to the remaining three models both in the training and validating cohorts. Therefore, the nomogram based on the best model in this study can be used as an auxiliary tool for clinicians to differentiate PMA and WT preoperatively.
There are some limitations in this study that should be acknowledged. First, it was possible that this study had sampling bias. In the process of collecting patient data, we excluded more cases, such as recurrent tumors, cases without preoperative MSCT-enhanced scans, cases in which the MSCT images were so unclear that the tumor borders could not be identified, etc. Second, this study was a single-center one with a relatively small sample size. In the future, we will continue to collect relevant data to validate the clinical value of the diagnostic model developed in this study. Third, this study lacks external validation, which limits the generalizability of the diagnostic model to other clinical settings. Future studies will aim to validate this model with external datasets from multiple centers to ensure its robustness and applicability across different populations.
Conclusions
In conclusion, we found that preoperative serum AFU levels could be used as a useful biomarker to differentiate PMA from WT. We further developed and validated a nomogram based on Rad-score, gender and serum AFU, which was proved to be highly accurate in distinguishing PMA from WT. This work could give clinicians fresh perspectives and techniques for figuring out the kind of parotid tumors before surgery.
Acknowledgments
We thank all of those who helped us with this retrospective study.
Funding: This work was supported by grants from
Footnote
Reporting Checklist: The authors have completed the TRIPOD reporting checklist. Available at https://tcr.amegroups.com/article/view/10.21037/tcr-24-871/rc
Data Sharing Statement: Available at https://tcr.amegroups.com/article/view/10.21037/tcr-24-871/dss
Peer Review File: Available at https://tcr.amegroups.com/article/view/10.21037/tcr-24-871/prf
Conflicts of Interest: All authors have completed the ICMJE uniform disclosure form (available at https://tcr.amegroups.com/article/view/10.21037/tcr-24-871/coif). The authors have no conflicts of interest to declare.
Ethical Statement: The authors are accountable for all aspects of the work in ensuring that questions related to the accuracy or integrity of any part of the work are appropriately investigated and resolved.
Open Access Statement: This is an Open Access article distributed in accordance with the Creative Commons Attribution-NonCommercial-NoDerivs 4.0 International License (CC BY-NC-ND 4.0), which permits the non-commercial replication and distribution of the article with the strict proviso that no changes or edits are made and the original work is properly cited (including links to both the formal publication through the relevant DOI and the license). See: https://creativecommons.org/licenses/by-nc-nd/4.0/.
References
- Zheng YM, Chen J, Xu Q, et al. Development and validation of an MRI-based radiomics nomogram for distinguishing Warthin's tumour from pleomorphic adenomas of the parotid gland. Dentomaxillofac Radiol 2021;50:20210023. [Crossref] [PubMed]
- Zhang D, Li X, Lv L, et al. Improving the diagnosis of common parotid tumors via the combination of CT image biomarkers and clinical parameters. BMC Med Imaging 2020;20:38. [Crossref] [PubMed]
- Hou Z, Li S, Jiang Y, et al. Benefits of intraoral stents for sparing normal tissue in radiotherapy of nasopharyngeal carcinoma: a radiobiological model-based quantitative analysis. Transl Cancer Res 2021;10:4281-9. [Crossref] [PubMed]
- Li ZQ, Gao JN, Xu S, et al. Multimodal magnetic resonance imaging for the diagnosis of parotid gland malignancies: systematic review and meta-analysis. Transl Cancer Res 2022;11:2275-82. [Crossref] [PubMed]
- Cheng PC, Chang CM, Huang CC, et al. The diagnostic performance of ultrasonography and computerized tomography in differentiating superficial from deep lobe parotid tumours. Clin Otolaryngol 2019;44:286-92. [Crossref] [PubMed]
- Mayerhoefer ME, Materka A, Langs G, et al. Introduction to Radiomics. J Nucl Med 2020;61:488-95. [Crossref] [PubMed]
- Lambin P, Rios-Velazquez E, Leijenaar R, et al. Radiomics: extracting more information from medical images using advanced feature analysis. Eur J Cancer 2012;48:441-6. [Crossref] [PubMed]
- Avanzo M, Stancanello J, Pirrone G, et al. Radiomics and deep learning in lung cancer. Strahlenther Onkol 2020;196:879-87. [Crossref] [PubMed]
- Hu Y, Tang D, Zhang P. Prognostic and immunological role of alpha-L-fucosidase 2 (FUCA2) in hepatocellular carcinoma. Transl Cancer Res 2023;12:257-72. [Crossref] [PubMed]
- Deugnier Y, Le Treut A, Glaise D, et al. A study of lysosomal enzyme activities in serum and leukocytes in chronic hepatic disease (author's transl). Clin Chim Acta 1980;108:385-92. [Crossref] [PubMed]
- Ezawa I, Sawai Y, Kawase T, et al. Novel p53 target gene FUCA1 encodes a fucosidase and regulates growth and survival of cancer cells. Cancer Sci 2016;107:734-45. [Crossref] [PubMed]
- Otero-Estévez O, Martínez-Fernández M, Vázquez-Iglesias L, et al. Decreased expression of alpha-L-fucosidase gene FUCA1 in human colorectal tumors. Int J Mol Sci 2013;14:16986-98. [Crossref] [PubMed]
- Shah M, Telang S, Raval G, et al. Serum fucosylation changes in oral cancer and oral precancerous conditions: alpha-L-fucosidase as a marker. Cancer 2008;113:336-46. [Crossref] [PubMed]
- Moons KG, Altman DG, Reitsma JB, et al. New Guideline for the Reporting of Studies Developing, Validating, or Updating a Multivariable Clinical Prediction Model: The TRIPOD Statement. Adv Anat Pathol 2015;22:303-5. [Crossref] [PubMed]
- Sun L, Fu Y, Zhao J, et al. MAS-CL: An End-to-End Multi-Atlas Supervised Contrastive Learning Framework for Brain ROI Segmentation. IEEE Trans Image Process 2024;33:4319-33. [Crossref] [PubMed]
- Wang T, She Y, Yang Y, et al. Radiomics for Survival Risk Stratification of Clinical and Pathologic Stage IA Pure-Solid Non-Small Cell Lung Cancer. Radiology 2022;302:425-34. [Crossref] [PubMed]
- Shrout PE, Fleiss JL. Intraclass correlations: uses in assessing rater reliability. Psychol Bull 1979;86:420-8. [Crossref] [PubMed]
- Trayanova NA, Popescu DM, Shade JK. Machine Learning in Arrhythmia and Electrophysiology. Circ Res 2021;128:544-66. [Crossref] [PubMed]
- Jaddoe VW, de Jonge LL, Hofman A, et al. First trimester fetal growth restriction and cardiovascular risk factors in school age children: population based cohort study. BMJ 2014;348:g14. [Crossref] [PubMed]
- Meurer WJ, Tolles J. Logistic Regression Diagnostics: Understanding How Well a Model Predicts Outcomes. JAMA 2017;317:1068-9. [Crossref] [PubMed]
- Liu P, Xing Z, Peng X, et al. Machine learning versus multivariate logistic regression for predicting severe COVID-19 in hospitalized children with Omicron variant infection. J Med Virol 2024;96:e29447. [Crossref] [PubMed]
- Portet S. A primer on model selection using the Akaike Information Criterion. Infect Dis Model 2020;5:111-28. [Crossref] [PubMed]
- Messina A, Chew M, Cecconi M. Rotten and gold apples: inside and outside the gray zone of a ROC curve. Crit Care 2024;28:22. [Crossref] [PubMed]
- Adamson AS, Abraham I. Decision Curve Analysis and the Net Benefit of Novel Tests. JAMA Dermatol 2022;158:684. [Crossref] [PubMed]
- Zhang D, Zhu W, Guo J, et al. Application of artificial intelligence in glioma researches: A bibliometric analysis. Front Oncol 2022;12:978427. [Crossref] [PubMed]
- Demircioğlu A. Radiomics-AI-based image analysis. Pathologe 2019;40:271-6. [PubMed]
- Kushima M, Kojima R, Shinohara R, et al. Association Between Screen Time Exposure in Children at 1 Year of Age and Autism Spectrum Disorder at 3 Years of Age: The Japan Environment and Children's Study. JAMA Pediatr 2022;176:384-91. [Crossref] [PubMed]
- Liu Y, Zheng J, Lu X, et al. Radiomics-based comparison of MRI and CT for differentiating pleomorphic adenomas and Warthin tumors of the parotid gland: a retrospective study. Oral Surg Oral Med Oral Pathol Oral Radiol 2021;131:591-9. [Crossref] [PubMed]
- Li Q, Jiang T, Zhang C, et al. A nomogram based on clinical information, conventional ultrasound and radiomics improves prediction of malignant parotid gland lesions. Cancer Lett 2022;527:107-14. [Crossref] [PubMed]
- Matsumoto K, Shimizu C, Arao T, et al. Identification of predictive biomarkers for response to trastuzumab using plasma FUCA activity and N-glycan identified by MALDI-TOF-MS. J Proteome Res 2009;8:457-62. [Crossref] [PubMed]
- Vajaria BN, Patel KA, Patel PS. Role of aberrant glycosylation enzymes in oral cancer progression. J Carcinog 2018;17:5. [Crossref] [PubMed]
- Levyn H, Subramanian T, Eagan A, et al. Risk of Carcinoma in Pleomorphic Adenomas of the Parotid. JAMA Otolaryngol Head Neck Surg 2023;149:1034-41. [Crossref] [PubMed]
- Sunnetci KM, Kaba E, Celiker FB, et al. Deep Network-Based Comprehensive Parotid Gland Tumor Detection. Acad Radiol 2024;31:157-67. [Crossref] [PubMed]
- Chen J, He B, Dong D, et al. Noninvasive CT radiomic model for preoperative prediction of lymph node metastasis in early cervical carcinoma. Br J Radiol 2020;93:20190558. [Crossref] [PubMed]
- Mayerhoefer ME, Riedl CC, Kumar A, et al. Radiomic features of glucose metabolism enable prediction of outcome in mantle cell lymphoma. Eur J Nucl Med Mol Imaging 2019;46:2760-9. [Crossref] [PubMed]