Prognostic value of CTF1 in glioma and its role in the tumor microenvironment
Highlight box
Key findings
• Cardiotrophin-1 (CTF1) was established as an effective tool for glioma screening, diagnosis, and prognostic assessment. A nomogram was developed and validated based on the CTF1 to predict the overall survival of glioma patients.
What is known and what is new?
• High CTF1 expression is associated with poor prognosis in cancers, including gastric cancer, breast cancer and uveal melanoma, etc.
• This study found that CTF1 was significantly overexpressed in tumor tissues and significantly correlated with patient prognosis, suggesting that CTF1 could be used as an effective differentiating tool and therapeutic target for glioma. A nomogram based on CTF1 and clinicopathological factors was constructed to fully investigate the role of CTF1 in glioma progression.
What is the implication, and what should change now?
• The potential of CTF1 as a predictive biomarker for glioma is confirmed.
• The efficacy and safety of this nomogram need to be verified by further large-scale clinical trials.
Introduction
The prevalence of primary malignant brain tumors is estimated to be approximately 7 cases per 100,000 individuals, with a higher incidence observed in older age groups (1). Glioma is recognized as the most common form of primary brain tumor, known for its invasive characteristics and aggressive tendencies (2). Gliomas are classified by the World Health Organization (WHO) into WHO grades 1 through 4 according to both histological and molecular features, which are essential for determining the diagnosis, treatment plan, and prognosis for the illness (3). Glioblastoma (GBM) is known to be a very aggressive type of brain cancer that is usually treated with surgery, radiation, and chemotherapy (4). According to the WHO 2021 Classification of Central Nervous System Tumors, the diagnosis of GBM is reserved for IDH wild-type gliomas (WHO grade 4). The aggressive nature, frequent return, and treatment resistance of GBM lead to an average survival time of just 14 months. Approximately 75% of GBM patients treated with temozolomide (TMZ) succumb to the disease within 2 years due to recurrence (5,6). As a result, GBM continues to be a significant contributor to cancer-related mortality. Hence, clinicians and researchers must urgently discover molecular biomarkers linked to GBM in order to create better treatment plans and predict patient survival more accurately, ultimately enhancing prognostic outcomes.
Cardiotrophin-1 (CTF1) belongs to the interleukin 6 (IL6) family of cytokines and has been shown to stimulate compensatory hypertrophy in heart muscle cells, preserve heart function, prevent cell death, shield against damage from lack of oxygen followed by restoration of blood flow in the heart, kidney, and liver, and enhance the growth of nerve tissue (7-11). Currently, most studies have found that CTF1 has a protective effect on various solid organs, but its role in tumors may be quite the opposite. CTF1 has been investigated in different types of cancers to understand its expression and importance in clinical settings. CTF1 expression has been demonstrated to enhance the movement, infiltration, and spread of breast cancer cells by activating STAT3 phosphorylation and movement into the nucleus, triggering the transcriptional activation of important autophagy proteins (12). The prognosis of patients with uveal melanoma can be predicted by CTF1 as an immune-related gene signature (13). However, the prognostic significance of CTF1 and its correlation with immune infiltrates in glioma remain unclear.
Limited information is available regarding the effectiveness of CTF1 in treating glioma, as it has not been extensively studied in this situation. Our data were sourced from The Cancer Genome Atlas (TCGA) and Chinese Glioma Genome Atlas (CGGA). We first validated the Messenger RNA (mRNA) expression levels of CTF1 using TCGA and CGGA Messenger RNA Sequencing (mRNA-seq) data. Independent prognostic clinical-pathological factors were identified using Kaplan-Meier and Cox regression analyses. The CIBERSORT technique, commonly utilized to assess the various types of immune cells present in the tumor microenvironment, was used to examine ratios of immune cells infiltrating the tumor. We employed similar techniques to evaluate the correlation between CTF1 expression and immune cell infiltration. Furthermore, we developed and Valided a novel nomogram and calibration curves to forecast the likelihood of survival for individuals with glioma, while also examining the theoretical connections and associations between CTF1 and the interaction of tumor immunity. This study focuses on the significance of CTF1 in glioma survival prognosis, laying the foundation for clinical improvement of glioma prognosis. We present this article in accordance with the TRIPOD reporting checklist (available at https://tcr.amegroups.com/article/view/10.21037/tcr-24-1258/rc).
Methods
Acquiring data and processing
The mRNA expression data and relatively complete clinical information (such as age, gender, WHO grade and survival status) of glioma patients in the TCGA training cohort were obtained from the University of California, Santa Cruz (UCSC) Xena (https://xena.ucsc.edu/). These included 689 glioma tissue samples (tumour) and 1157 normal tissue samples(normal). To independently validate the diagnostic value, prognostic value and clinical value of CTF1, we used the CGGA validation cohort from the Chinese Glioma Genome Atlas (http://www.cgga.org.cn) as an external independent validation cohort. The CGGA 325 cohort involves a multicenter study including 325 glioma patients from grade 2 to 4, sourced from institutions such as the Beijing Neurosurgical Institute and Beijing Tiantan Hospital, Capital Medical University. The gene expression levels of both the TCGA training cohort and the CGGA validation cohort were converted to a log2 scale. The study was conducted in accordance with the Declaration of Helsinki (as revised in 2013).
Co-expression analysis
CTF1 gene co-expression was analyzed in GBM data. The correlation coefficient had a filtering condition of 0.4, with a P value of 0.05. After screening the most significant genes with CTF1 expression, the CTF1 correlation analysis circle and heat map were drawn with “corrplot” and “circlize” packages.
Immune cell infiltration analysis
CIBERSORT is a widely used technique for assessing various types of immune cells in tumor microenvironment. The deconvolution analysis of the immune cell subtype expression matrix was conducted using support vector regression principles. Using the 547 genes from the LM22 signature file, 22 types human immune cells were distinguished, including T cells, B cells, plasma cells, and myeloid cells. CIBERSORT algorithm was used to analyze RNA-seq data from glioma patients, estimating the distribution of these 22 immune cell types. Further, a correlation analysis was conducted to investigate the relationship between CTF1 expression and immune cell composition, with a significance level of less than 0.05 considered statistically significant.
Gene set variation analysis (GSVA)
GSVA converts gene-level variations into pathway-level changes, thereby assessing the biological functions of samples based on gene set variation analysis. Based on the Molecular Signatures Database, gene sets were obtained and analyzed by GSVA. With this approach, we were able to calculate comprehensive scores for each gene set, enabling us to assess potential functional changes across samples.
Gene set enrichment analysis (GSEA)
GSEA was used to analyze the signaling pathway variances between high-expression and low-expression groups based on CTF1 expression levels. Gene sets from version 7.0 of MsigDB database were used as background gene sets for annotating subtype pathways. Pathway analysis was conducted to compare gene expression differences between subtypes, and gene sets that were significantly enriched (P<0.05) were identified based on consistency scores. GSEA analysis is frequently utilized to investigate the intimate relationship between disease categorization and biological relevance.
Drug sensitivity analysis
Using the Genomics of Drug Sensitivity in Cancer (GDSC) database, we utilized the R software package ‘pRRophetic’ to forecast the responsiveness of individual tumor samples to chemotherapy. Half maximal inhibitory concentration (IC50) predictions for each chemotherapy drug were derived through regression analysis, and the accuracy of the regression and predictions were validated through 10 cross-validations using the GDSC training set. All parameters were assigned default values, such as ‘combat’ for batch effects removal and calculating the mean of gene expression repetitions.
Development and evaluation of a nomogram
Using the RMS package, a nomogram was constructed using CTF1 expression level, isocitrate dehydrogenase 1 (IDH1) mutation status, O6-methylguanine-DNA methyltransferase (MGMT) methylation status, WHO grade, Age and Gender for the TCGA training cohort. To independently verify the accuracy of OS prediction of our established nomogram, calibration curves were calculated to evaluate the accuracy of 1- and 2-year survival rates. In general, the consistency of the predicted 1-, 2-year OS with the actual OS is presented by calibration curves. The feasibility of the nomogram was confirmed by external validation using the CGGA dataset.
Weighted gene co-expression network analysis (WGCNA)
We constructed a weighted gene co-expression network and explored the correlation between the gene network and CTF1 and the key genes. A co-expression network of all genes in the glioma dataset was created using the WGCNA-R package, and the algorithm identified the top 5,000 variograms. A threshold of 9 was established as the soft limit. A weighted adjacency matrix converted to a topological overlap matrix (TOM) allows the estimation of network connectivity, followed by hierarchical clustering to create the TOM matrix cluster tree. Various sections of the cluster tree symbolize distinct gene groups, with varying colors indicating separate modules. Genes were categorized based on their expression patterns using the weighted correlation coefficient. Genes exhibiting comparable patterns were clustered together in a single module, while the rest of the genes were categorized into various modules based on their expression patterns.
Analysis of gene modules for functional enrichment
Using the R package ClusterProfiler, the biological functions and signaling pathways associated with WGCNA key modules were analyzed, with the brown module showing the strongest correlation with CTF1. This analysis aimed to provide a comprehensive understanding of the functional relationships among the genes within this module. Functional categories were evaluated using the Gene Ontology (GO) and the Kyoto Encyclopedia of Genes and Genomes (KEGG). Enrichment pathways from GO and KEGG were deemed significant if their P and q values <0.05.
Statistical analysis
Analyses were conducted using R language (version 4.3.0), with significance defined as P<0.05. An unpaired t test was used to determine the significance of differences between the two groups; one-way analysis of variance (ANOVA) was used to compare the differences between three or more groups. To assess the significance of the difference in prognosis between the CTF1 high-expression group and the CTF low-expression group, KM curves were generated using the log-rank test. The P value of Pearson correlation analysis had been corrected by Bonferroni.
Results
Clinical value and prognostic value of CTF1
The specific mechanism of CTF1 in Glioma remains unclear. To explore the association between CTF1 and glioma. The original mRNA expression data for glioma was obtained and incorporated from the TCGA database. Analysis revealed a significant difference in CTF1 expression between normal and cancerous tissues, with a notable increase in cancerous tissues (Figure 1A). Examining survival rates by categorizing high and low CTF1 gene expression levels revealed a significant P<0.001 at the median value of CTF1 (Figure 1B,1C). By performing receiver operating characteristic (ROC) curve analysis to discriminate tumor tissue from normal tissue samples, we found that area under the curve (AUC) of the CTF1 expression level was 0.889 in TCGA (Figure 1D) and was 0.664 in CGGA (Figure 1E). We further evaluated the correlation between CTF1 expression levels and glioma grades. In the TCGA dataset, no significant difference was observed between Grade 2 and 3 (G2 vs. G3) (P=0.15). However, there was a significant difference between G2 vs. G4, and G3 vs. G4 (P<0.001) (Figure 1F). In the CGGA dataset (14-17), A significant statistical difference was found between G2 and G3 (P=0.03), G3 and G4 (P=0.003), and G2 and G4 (P<0.001) (Figure 1G). Univariate and multivariate Cox regression models were created, using clinical data and CTF1 gene expression levels. CTF1 was identified as a risk factor in Glioma patients based on the results of both univariate and multivariate Cox regression analysis (Table 1).
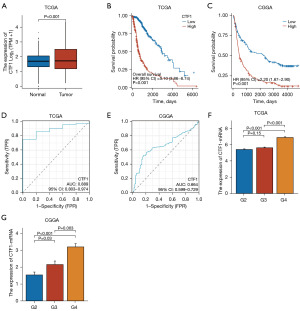
Table 1
Characteristics | Total (N) | Univariate analysis | Multivariate analysis | |||
---|---|---|---|---|---|---|
HR (95% CI) | P value | HR (95% CI) | P value | |||
Age | 567 | |||||
≤65 years | 493 | Reference | Reference | |||
>65 years | 74 | 8.000 (5.473–11.694) | <0.001 | 3.809 (2.492–5.821) | <0.001 | |
Gender | 567 | |||||
Male | 322 | Reference | ||||
Female | 245 | 1.062 (0.771–1.463) | 0.71 | |||
WHO grades | 567 | |||||
G4 | 118 | Reference | Reference | |||
G2 | 211 | 0.047 (0.028–0.079) | <0.001 | 0.200 (0.105–0.382) | <0.001 | |
G3 | 238 | 0.155 (0.106–0.227) | <0.001 | 0.437 (0.280–0.683) | <0.001 | |
IDH mutation status | 567 | |||||
Mutant | 372 | Reference | Reference | |||
Wildtype | 195 | 10.461 (7.267–15.060) | <0.001 | 3.283 (1.778–6.060) | <0.001 | |
MGMT methylation status | 567 | |||||
Methylated | 421 | Reference | Reference | |||
Un-methylated | 146 | 3.310 (2.381–4.601) | <0.001 | 0.985 (0.671–1.448) | 0.94 | |
CTF1 | 567 | 2.077 (1.797–2.400) | <0.001 | 1.233 (1.025–1.484) | 0.03 |
CTF1, cardiotrophin-1; TCGA, The Cancer Genome Atlas; HR, hazard ratio; CI, confidence interval; WHO, World Health Organization; IDH1, isocitrate dehydrogenase 1; MGMT, O6-methylguanine-DNA methyltransferase.
Correlation analysis between CTF1 expression and clinical characteristics of glioma
The clinical and gene expression characteristics of 567 Glioma patients were collected from the TCGA-GBMLGG database. Based on the average CTF1 expression level, Glioma patients were categorized into a high-expressing group consisting of 283 individuals and a low-expressing group consisting of 284 individuals. The correlation between clinicopathological characteristics of Glioma and CTF1 expression level was analyzed using the Chi-square test. Upon examination of the connection between CTF1 expression and various clinical parameters, we discovered a significant correlation with WHO grade (P<0.001), IDH1 mutation status (P<0.001), MGMT methylation status (P<0.001), age (P<0.001), while no significant correlation was found with gender (P=0.77) (Table 2).
Table 2
Variable | Overall , N=567 | CTF1 | Statistics | P value† | |
---|---|---|---|---|---|
High, N=283 (50%) | Low, N=284 (50%) | ||||
Age (years) | 46.00 [34.00, 59.00] | 53.00 [38.50, 63.00] | 39.00 [32.00, 51.00] | 55,374.50 | <0.001 |
MGMT methylation status | 100.27 | <0.001 | |||
Methylated | 421 (74.25) | 158 (55.83) | 263 (92.61) | ||
Un-methylated | 146 (25.75) | 125 (44.17) | 21 (7.39) | ||
IDH1 mutation status | 224.15 | <0.001 | |||
Mutant | 372 (65.61) | 101 (35.69) | 271 (95.42) | ||
Wildtype | 195 (34.39) | 182 (64.31) | 13 (4.58) | ||
Gender | 0.08 | 0.77 | |||
Female | 245 (43.21) | 124 (43.82) | 121 (42.61) | ||
Male | 322 (56.79) | 159 (56.18) | 163 (57.39) | ||
WHO grades | 93.24 | <0.001 | |||
Grade 2 | 211 (37.21) | 71 (25.09) | 140 (49.30) | ||
Grade 3 | 238 (41.98) | 108 (38.16) | 130 (45.77) | ||
Grade 4 | 118 (20.81) | 104 (36.75) | 14 (4.93) |
Statistics: statistical test results for intergroup differences. Age: Mann-Whitney U test. Categorical variables were primarily analyzed using the Chi-square test. If the group frequency <5, Fisher’s exact test was used instead. Data are presented as median [interquartile range] or n (%). †, Wilcoxon rank sum test; Pearson’s Chi-squared test. CTF1, cardiotrophin-1; IDH1, isocitrate dehydrogenase 1; MGMT, O6-methylguanine-DNA methyltransferase; WHO, World Health Organization.
Identification of CTF1 co-expressed genes
In gliomas, GBM is the most malignant form. Therefore, we focused our attention on GBM in this study. We analyzed the correlation between CTF1 and gene expression patterns of GBM patients in TCGA database to explore their co-expression network. The correlation coefficient threshold was set at 0.4 with a significance level of 0.05. A total of 573 genes exhibiting significant correlations with CTF1 expression were identified, and heatmaps displaying the top 10 genes with positive and negative correlation coefficients, as well as gene co-expression correlation circle maps, were generated (Figure 2A,2B).
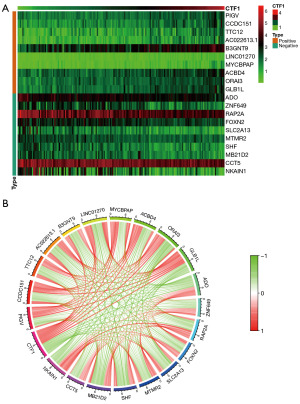
Immuno-infiltration analysis
This study analyzed the connection between GBM and tumor immune invasion by studying the TCGA dataset, focusing on the immune cell components in individual patients and the interactions among various immune cell types (Figure 3A,3B). Simultaneously, we observed significant differences in the activation of Dendritic cells, Macrophages M0, Monocytes, Neutrophils, and T-cells CD8 between the two groups (Figure 3C). This research delved deeper into the connection between the CTF1 gene and immune cells, finding a strong positive correlation between CTF1 and resting Neutrophils, Monocytes, and Dendritic cells. There was a strong negative correlation with Macrophages M0 (Figure 3D).
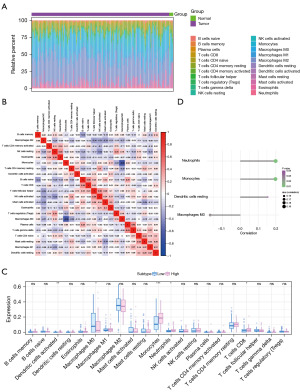
GSVA and GSEA analysis of CTF1
Our research explored the signaling pathway linked to CTF1 and analyzed the molecular processes by which the CTF1 gene could influence the advancement of tumors. GSVA results showed that individuals with increased CTF1 levels displayed enhancement in IL2_STAT5_SIGNALING, P53_PATHWAY, IL6_JAK_STAT3_SIGNALING, and various other pathways (Figure 4A). Additionally, GSEA findings suggested that CTF1 could enhance the IL-17 signaling pathway, TNF signaling pathway, and Pentose phosphate pathway (Figure 4B,4C). The findings indicate that CTF1 could potentially impact the progression of GBM by affecting these pathways.
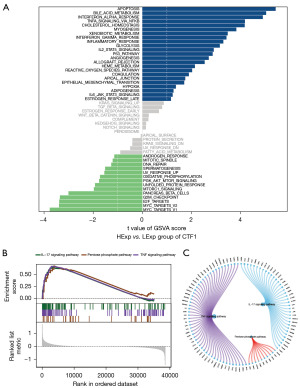
The relationship between CTF1 and GBM genomic instability
Since CTF1 was correlated with DNA damage repair (DDR) as revealed by GSVA analyses, we further analyzed the relationship between CTF1 and the genomic instability of GBM. After obtaining the processed GBM data related to single nucleotide polymorphisms (SNPs), we chose the top 30 genes with a high mutation rate for visualization. We analyzed the differences between the two groups and created a mutation map using R ComplexHeatmap. The high-expression group had a notably reduced percentage of TP53 gene mutations compared to the low-expression group (Figure 5A). Our research utilized the drug sensitivity information from the GDSC database to forecast the responsiveness of individual tumor samples to chemotherapy. This was achieved through the utilization of the R package ‘pRRophetic’ to delve deeper into the sensitivity of CTF1 and widely used chemotherapy medications. The findings indicated a strong difference in responsiveness to Gemcitabine, Dasatinib, BIRB.0796, BMS.536924, Bexarotene, and Bicalutamide between the high and low expression groups of CTF1 (Figure 5B).
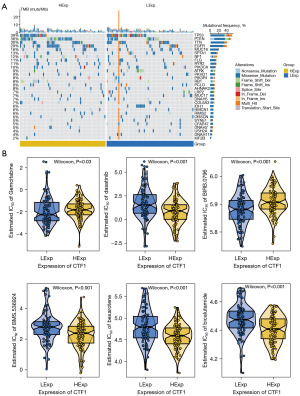
Establishment and validation of the prognostic nomogram
IDH1 mutation status and MGMT methylation status are established prognostic factors for glioma, as identified in previous studies (3,18). The significance of these biomarkers has been underscored by various analyses, including a comprehensive examination of molecular prognostic factors in gliomas, which highlights the importance of genetic and epigenetic alterations in predicting patient outcomes (19). Specifically, the combination of IDH1 mutations and MGMT methylation has been shown to provide a more accurate prediction of survival in GBM patients compared to either factor alone (20). Furthermore, recent research has demonstrated that MGMT promoter methylation serves as an independent prognostic biomarker in high-risk glioma patients treated with radiotherapy and temozolomide, reinforcing its clinical relevance (21). The association between MGMT methylation and patient age, as well as its relationship with 1p/19q status in IDH-mutant gliomas, further illustrates the complexity of glioma biology and the need for a multifaceted approach to patient stratification (22). Overall, the integration of these molecular markers into clinical practice is crucial for improving treatment strategies and patient management in glioma cases, as they provide valuable insights into tumor behavior and patient prognosis (23). Therefore, we incorporated these two factors into the Nomogram model. The regression analysis findings were displayed in a bar chart illustrating the levels of CTF1 expression. The results indicated that various clinical indicators for glioma and the distribution of CTF1 expression contributed differently to the overall scoring process across all samples (Figure 6A). Additionally, we performed a prognostic analysis of overall survival (OS) at 1- and 2-year, revealing a strong correlation between the predicted and observed OS. The Nomogram model demonstrated excellent predictive accuracy, which was further validated using the CGGA database (Figure 6B-6E).
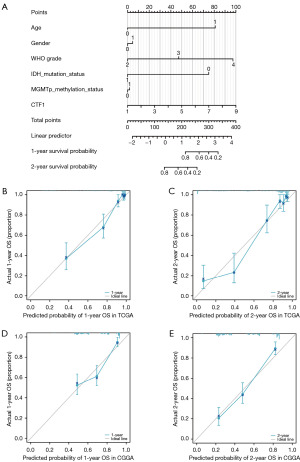
Identification of CTF1 relative genes (CRGs) and analysis of GO and KEGG pathways
We further constructed WGCNA network based on database to explore the CTF1-related regulatory network in GBM. A soft threshold of 9 was applied (Figure 7A) to detect gene modules using the tom matrix. A total of 8 gene modules were detected in GBM (Figure 7B). They are black [145], blue [720], brown [627], green [1,427], grey [1,399], pink [57], red [183] and yellow [442]. After conducting additional analysis on modules and characteristics, it was discovered that the brown module exhibited the strongest correlation with CTF1 (Figure 7C). The brown module gene was also utilized for pathway analysis, revealing enrichment in various pathways such as regulation of trans-synaptic signaling, synaptic vesicle cycle, and signal release from synapse according to the GO results (Figure 7D). The KEGG analysis indicated that the gene was predominantly involved in the Oxytocin signaling pathway, cAMP signaling pathway, neurotrophin signaling pathway, and various other pathways (Figure 7E).
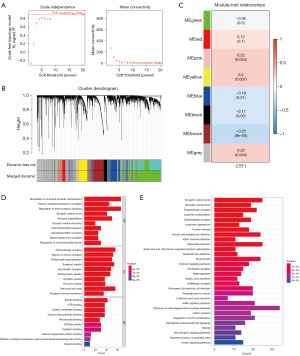
Discussion
Secreted CTF1 demonstrates expression across various types of central nervous system cells and exhibits evolutionary conservation. Wang et al. (24) demonstrated that CTF1 gene expression promotes glucose uptake in the brain by constructing transgenic mice with brain specific CTF1 expression. Meanwhile, CTF1 has been identified as a promoter of angiogenesis via the ADMA/DDAH pathway (25). Therefore, we suggest that CTF1 could be involved in the development and advancement of tumors. Research on CTF1 in the field of cancer is currently limited. CTF1 has shown varying levels of expression in lung adenocarcinoma and is identified as a prognostic indicator for this type of cancer (26). In breast cancer, gastric cancer, melanoma, and endometrial cancer, CTF1 is recognized as a factor that promotes tumor progression (12,13,27,28). Yet, there is a scarcity of literature regarding the possible predictive significance of CTF1 in glioma. Consequently, we conducted a study to investigate the potential involvement of CTF1 in glioma. This study analyzed CTF1 expression in glioma patients. We combined RNA-seq data with clinical data for retrospective analysis of 567 patients with Glioma. CTF1 mRNA levels were found to be associated with tumor grade in Glioma.
In this study, it was observed that changes in the level of CTF1 expression are associated with the outcome of glioma. High CTF1 expression was correlated with a negative prognosis in glioma. Increased expression of CTF1 was a standalone predictor of poor prognosis. Furthermore, our research indicated a connection between immune infiltration levels and CTF1 expression in GBM. Therefore, we propose that CTF1 could potentially impact GBM immunology. Furthermore, it has the potential to serve as a valuable indicator for glioma. CTF1 expression was found to vary between normal and tumor tissues in glioma. TCGA and CGGA datasets were used to investigate the mechanisms and connections of CTF1 expression in cancer. Analysis of multiple variables indicated that the expression of CTF1 was a significant independent predictor of prognosis in patients with glioma.
Given these preliminary findings, we constructed a nomogram based on CTF1 expression and various clinicopathological factors to predict survival in glioma patients. IDH1 mutation status and MGMT methylation status, both established as prognostic factors for glioma as identified in previous studies, were incorporated into the model (29). Additionally, since age is a significant factor affecting prognosis, it was also included alongside tumor grade and gender. Li et al. (30) constructed a model based on four SUMOylation regulator-related signatures, but did not incorporate glioma-related clinical factors. In our nomogram, we included key clinical factors that influence glioma prognosis. This comprehensive nomogram, which integrates CTF1 expression, tumor stage, age, gender, IDH1 mutation status, and MGMT methylation status, demonstrated superior accuracy compared to models based on single clinical factors. The advantage of our nomogram lies in its development from a relatively large cohort and its independent validation in an external database with a substantial sample size, ensuring robust generalizability and clinical applicability. The model is user-friendly and serves as a rapid and effective tool for personalized prognosis prediction and treatment guidance in glioma patients.
The growth of tumors is influenced by the surrounding environment, which has the ability to alter the formation of blood vessels in tumors through the control of the immune environment and the release of cytokines (31). Tumor-associated fibroblasts, immune cells, extracellular matrix, growth factors, inflammatory factors, and distinct physical and chemical traits make up the tumor microenvironment, influencing tumor diagnosis, survival rates, and treatment response (32,33). The intricate nature of the tumor microenvironment in GBM is well recognized. The GBM immune microenvironment is demonstrated as profoundly immunosuppressive, presenting a significant challenge in eliciting immune-mediated eradication of cancerous cells (34,35). Recent research has shown that CTF1 could impact tumor microenvironment by triggering autophagy, which in turn enhances invasion and spread in breast cancer (12). Therefore, we hypothesize that CTF1 may influence tumor progression and patient prognosis in GBM by impacting the tumor microenvironment. In GBM, CTF1 expression and immune infiltration have a notable relationship. A CIBERSORT analysis showed a significant variance between groups with high and low CTF1 expression, along with a direct correlation between them. Previously, it has been demonstrated that inflammatory monocytes contribute to glioma genesis (36). Neutrophils have been identified as a marker of poor prognosis in GBM (37). We found significant positive correlations between CTF1 expression levels and infiltrating neutrophils, monocytes, and dendritic cells in GBM. CTF1 had a significant impact on immune infiltration in our study, and this further corroborates our findings.
Studies examining the influence of tumor-infiltrating immune cells (TIICs) in humans frequently focus on evaluating T-cells, especially in relation to their reaction to immune checkpoint blockade and resulting survival rates (38,39). T-cells have been demonstrated to play a positive role in tumor prognosis (40). Patients diagnosed recently with glioma typically exhibit improved prognoses when they display higher levels of CD8+ T cell infiltration compared to those with lower level (41). Therefore, the influence of CTF1 on GBM prognosis could be due to the high levels of both T cells.
To further investigate the functions of CTF1 in GBM, through GSEA and GSVA analyses, we further explored the role of CTF1 in GBM. According to GSVA findings, individuals with elevated CTF1 levels exhibited enrichment in pathways like IL2_STAT5_SIGNALING, P53_PATHWAY, and IL6_JAK_STAT3_SIGNALING. The IL-2/STAT5 signaling pathway may activate immunosuppressive mechanisms, such as the recruitment of regulatory T cells (Tregs) and myeloid-derived suppressor cells (MDSCs), thereby promoting tumor progression (42). Additionally, activation of the IL-2/STAT5 signaling pathway is associated with increased tumor cell proliferation and resistance to apoptosis, which leads to poorer clinical outcomes (43). Therefore, modulating the IL-2/STAT5 signaling pathway could potentially enhance the efficacy of anti-tumor immunotherapies and reduce immune escape. The p53 pathway is associated with promoting tumor cell proliferation and inhibiting apoptosis, and has been shown to correlate with clinical pathological parameters and glioma prognosis. Notably, poorly differentiated gliomas exhibit high Ki-67 expression, and p53-positive gliomas show a significant correlation with elevated Ki-67 levels (44). However, the relationship between CTF1 and the p53 pathway has not been extensively studied. Enrichment analysis suggests a close association between CTF1 and the p53 pathway, indicating that further exploration of this relationship is warranted. Furthermore, the GSEA findings showed that CTF1 enriched signaling pathways like the IL-17 signaling pathway, TNF signaling pathway, and Pentose phosphate pathway in KEGG. Results from the GO analysis indicated that genes were primarily enriched in pathways related to the regulation of trans-synaptic signaling, synaptic vesicle cycle, and synaptic signal release.
Overall, although there may be a link between synaptic transmission and neuronal function abnormalities and the development of certain tumors, the direct relationship to tumor advancement is still being actively studied and needs more research in both experimental and clinical settings. All these indications imply that CTF1 could be a valuable prognostic indicator and target for treatment in GBM. Then, another important issue to discuss is whether increased CTF1 expression directly leads to malignant tumor progression or merely serves as a marker associated with inherent malignancy factors in tumors. CTF1 may promote tumor growth by activating specific signaling pathways, such as IL-2 or p53, or it could simply be an accompanying phenomenon of tumor deterioration due to inherent poor prognostic factors (such as higher invasiveness). This remains to be explored in our future research.
There are several limitations in this study. Some clinicopathological factors, such as tumor recurrence/metastasis, chemotherapy, and radiotherapy, are incomplete or missing in the TCGA and CGGA databases. Consequently, large-scale clinical trials are needed to fully assess the prognostic value of CTF1 in glioma.
Conclusions
In recent years, the incidence of glioma has been steadily increasing. Despite significant advances in diagnosis and treatment, patient survival remains limited. Identifying new biomarkers with diagnostic and prognostic value is a crucial research direction to improve early detection rates and outcomes in glioma. This study revealed that CTF1 was significantly overexpressed in glioma tissues, making it an effective differentiating tool for glioma. Furthermore, CTF1 expression was significantly correlated with the survival and prognosis of glioma patients, suggesting that CTF1 could serve as a novel therapeutic target for glioma.
We also investigated the role of CTF1 in immune infiltration within GBM and explored its relationship with gene mutations and drug sensitivity. Subsequently, a nomogram based on CTF1 expression and clinicopathological factors was constructed. Our study not only examined the impact of CTF1 on the diagnosis and prognosis of glioma but also provided a foundation for further investigation into the molecular functions of CTF1. These findings offer new insights into the mechanistic exploration and treatment of glioma.
Acknowledgments
We express our gratitude to the public databases, specifically the TCGA and CGGA, for their invaluable contributions to human medicine. The datasets used and analysed in this study, including TCGA-GBMLGG, are available in the University of California, Santa Cruz (UCSC) repository. Additionally, the CGGA 325 datasets utilized in our research can be accessed via the Chinese Glioma Genome Atlas at http://www.cgga.org.cn.
Funding: This work was supported by
Footnote
Reporting Checklist: The authors have completed the TRIPOD reporting checklist. Available at https://tcr.amegroups.com/article/view/10.21037/tcr-24-1258/rc
Peer Review File: Available at https://tcr.amegroups.com/article/view/10.21037/tcr-24-1258/prf
Conflicts of Interest: All authors have completed the ICMJE uniform disclosure form (available at https://tcr.amegroups.com/article/view/10.21037/tcr-24-1258/coif). The authors have no conflicts of interest to declare.
Ethical Statement: The authors are accountable for all aspects of the work in ensuring that questions related to the accuracy or integrity of any part of the work are appropriately investigated and resolved. The study was conducted in accordance with the Declaration of Helsinki (as revised in 2013).
Open Access Statement: This is an Open Access article distributed in accordance with the Creative Commons Attribution-NonCommercial-NoDerivs 4.0 International License (CC BY-NC-ND 4.0), which permits the non-commercial replication and distribution of the article with the strict proviso that no changes or edits are made and the original work is properly cited (including links to both the formal publication through the relevant DOI and the license). See: https://creativecommons.org/licenses/by-nc-nd/4.0/.
References
- Hdeib A, Sloan AE. Convection-enhanced delivery of 131I-chTNT-1/B mAB for treatment of high-grade adult gliomas. Expert Opin Biol Ther 2011;11:799-806. [Crossref] [PubMed]
- Li H, Xue Y, Ma J, et al. SNHG1 promotes malignant biological behaviors of glioma cells via microRNA-154-5p/miR-376b-3p- FOXP2- KDM5B participating positive feedback loop. J Exp Clin Cancer Res 2019;38:59. [Crossref] [PubMed]
- Torp SH, Solheim O, Skjulsvik AJ. The WHO 2021 Classification of Central Nervous System tumours: a practical update on what neurosurgeons need to know-a minireview. Acta Neurochir (Wien) 2022;164:2453-64. [Crossref] [PubMed]
- Li S, He Y, Chen K, et al. RSL3 Drives Ferroptosis through NF-κB Pathway Activation and GPX4 Depletion in Glioblastoma. Oxid Med Cell Longev 2021;2021:2915019. [Crossref] [PubMed]
- Ramakrishnan V, Xu B, Akers J, et al. Radiation-induced extracellular vesicle (EV) release of miR-603 promotes IGF1-mediated stem cell state in glioblastomas. EBioMedicine 2020;55:102736. [Crossref] [PubMed]
- Brandes AA, Tosoni A, Franceschi E, et al. Recurrence pattern after temozolomide concomitant with and adjuvant to radiotherapy in newly diagnosed patients with glioblastoma: correlation With MGMT promoter methylation status. J Clin Oncol 2009;27:1275-9. [Crossref] [PubMed]
- Zelt JGE, Chaudhary KR, Cadete VJ, et al. Medical Therapy for Heart Failure Associated With Pulmonary Hypertension. Circ Res 2019;124:1551-67. [Crossref] [PubMed]
- López-Yoldi M, Moreno-Aliaga MJ, Bustos M. Cardiotrophin-1: A multifaceted cytokine. Cytokine Growth Factor Rev 2015;26:523-32. [Crossref] [PubMed]
- Stephanou A, Brar B, Heads R, et al. Cardiotrophin-1 induces heat shock protein accumulation in cultured cardiac cells and protects them from stressful stimuli. J Mol Cell Cardiol 1998;30:849-55. [Crossref] [PubMed]
- Pennica D, Arce V, Swanson TA, et al. Cardiotrophin-1, a cytokine present in embryonic muscle, supports long-term survival of spinal motoneurons. Neuron 1996;17:63-74. [Crossref] [PubMed]
- Craig R, Larkin A, Mingo AM, et al. p38 MAPK and NF-kappa B collaborate to induce interleukin-6 gene expression and release. Evidence for a cytoprotective autocrine signaling pathway in a cardiac myocyte model system. J Biol Chem 2000;275:23814-24. [Crossref] [PubMed]
- Akkoc Y, Dalci K, Karakas HE, et al. Tumor-derived CTF1 (cardiotrophin 1) is a critical mediator of stroma-assisted and autophagy-dependent breast cancer cell migration, invasion and metastasis. Autophagy 2023;19:306-23. [Crossref] [PubMed]
- Wang W, Wang S. The prognostic value of immune-related genes AZGP1, SLCO5A1, and CTF1 in Uveal melanoma. Front Oncol 2022;12:918230. [Crossref] [PubMed]
- Zhao Z, Zhang KN, Wang Q, et al. Chinese Glioma Genome Atlas (CGGA): A Comprehensive Resource with Functional Genomic Data from Chinese Glioma Patients. Genomics Proteomics Bioinformatics 2021;19:1-12. [Crossref] [PubMed]
- Zhao Z, Meng F, Wang W, et al. Comprehensive RNA-seq transcriptomic profiling in the malignant progression of gliomas. Sci Data 2017;4:170024. [Crossref] [PubMed]
- Bao ZS, Chen HM, Yang MY, et al. RNA-seq of 272 gliomas revealed a novel, recurrent PTPRZ1-MET fusion transcript in secondary glioblastomas. Genome Res 2014;24:1765-73. [Crossref] [PubMed]
- Zhang K, Liu X, Li G, et al. Clinical management and survival outcomes of patients with different molecular subtypes of diffuse gliomas in China (2011-2017): a multicenter retrospective study from CGGA. Cancer Biol Med 2022;19:1460-76. [Crossref] [PubMed]
- Altieri R, Zenga F, Ducati A, et al. Tumor location and patient age predict biological signatures of high-grade gliomas. Neurosurg Rev 2018;41:599-604. [Crossref] [PubMed]
- Aquilanti E, Miller J, Santagata S, et al. Updates in prognostic markers for gliomas. Neuro Oncol 2018;20:vii17-26. [Crossref] [PubMed]
- Molenaar RJ, Verbaan D, Lamba S, et al. The combination of IDH1 mutations and MGMT methylation status predicts survival in glioblastoma better than either IDH1 or MGMT alone. Neuro Oncol 2014;16:1263-73. [Crossref] [PubMed]
- Bell EH, Zhang P, Fisher BJ, et al. Association of MGMT Promoter Methylation Status With Survival Outcomes in Patients With High-Risk Glioma Treated With Radiotherapy and Temozolomide: An Analysis From the NRG Oncology/RTOG 0424 Trial. JAMA Oncol 2018;4:1405-9. [Crossref] [PubMed]
- Horbinski C, McCortney K, Stupp R. MGMT promoter methylation is associated with patient age and 1p/19q status in IDH-mutant gliomas. Neuro Oncol 2021;23:858-60. [Crossref] [PubMed]
- Miller JJ, Cahill DP. MGMT promoter methylation and hypermutant recurrence in IDH mutant lower-grade glioma. Neuro Oncol 2020;22:1553-4. [Crossref] [PubMed]
- Wang D, Li X, Gao K, et al. Cardiotrophin-1 (CTF1) ameliorates glucose-uptake defects and improves memory and learning deficits in a transgenic mouse model of Alzheimer’s disease. Pharmacol Biochem Behav 2013;107:48-57. [Crossref] [PubMed]
- Fiedler LR, Bachetti T, Leiper J, et al. The ADMA/DDAH pathway regulates VEGF-mediated angiogenesis. Arterioscler Thromb Vasc Biol 2009;29:2117-24. [Crossref] [PubMed]
- Gao L, Zhang L. Construction and comprehensive analysis of a ceRNA network to reveal potential prognostic biomarkers for lung adenocarcinoma. BMC Cancer 2021;21:849. [Crossref] [PubMed]
- Ding W, Wu L, Li X, et al. Comprehensive analysis of competitive endogenous RNAs network: Identification and validation of prediction model composed of mRNA signature and miRNA signature in gastric cancer. Oncol Lett 2022;23:150. [Crossref] [PubMed]
- Liu J, Chen X, Jiang Y, et al. Development of an immune gene prognostic classifier for survival prediction and respond to immunocheckpoint inhibitor therapy/chemotherapy in endometrial cancer. Int Immunopharmacol 2020;86:106735. [Crossref] [PubMed]
- Zeng HL, Li H, Yang Q, et al. Transcriptomic Characterization of Copper-Binding Proteins for Predicting Prognosis in Glioma. Brain Sci 2023;13:1460. [Crossref] [PubMed]
- Li X, Meng Y. Construction of a SUMOylation regulator-based prognostic model in low-grade glioma. J Cell Mol Med 2021;25:5434-42. [Crossref] [PubMed]
- Guo W, Yin G, Liu H, et al. Analysis of vascular-associated factors and the prognosis of poorly differentiated hypopharyngeal carcinoma. Oncol Lett 2020;20:271. [Crossref] [PubMed]
- Ollauri-Ibáñez C, Ayuso-Íñigo B, Pericacho M. Hot and Cold Tumors: Is Endoglin (CD105) a Potential Target for Vessel Normalization? Cancers (Basel) 2021;13:1552. [Crossref] [PubMed]
- Li J, Gu X, Gao C, et al. Six MicroRNA Prognostic Models for Overall Survival of Lung Adenocarcinoma. Genet Res (Camb) 2022;2022:5955052. [Crossref] [PubMed]
- Pombo Antunes AR, Scheyltjens I, Duerinck J, et al. Understanding the glioblastoma immune microenvironment as basis for the development of new immunotherapeutic strategies. Elife 2020;9:e52176. [Crossref] [PubMed]
- Bekeschus S, Ispirjan M, Freund E, et al. Gas Plasma Exposure of Glioblastoma Is Cytotoxic and Immunomodulatory in Patient-Derived GBM Tissue. Cancers (Basel) 2022;14:813. [Crossref] [PubMed]
- Maximov V, Chen Z, Wei Y, et al. Tumour-associated macrophages exhibit anti-tumoural properties in Sonic Hedgehog medulloblastoma. Nat Commun 2019;10:2410. [Crossref] [PubMed]
- Zha C, Meng X, Li L, et al. Neutrophil extracellular traps mediate the crosstalk between glioma progression and the tumor microenvironment via the HMGB1/RAGE/IL-8 axis. Cancer Biol Med 2020;17:154-68. [Crossref] [PubMed]
- Powles T, Eder JP, Fine GD, et al. MPDL3280A (anti-PD-L1) treatment leads to clinical activity in metastatic bladder cancer. Nature 2014;515:558-62. [Crossref] [PubMed]
- Mahmoud SM, Paish EC, Powe DG, et al. Tumor-infiltrating CD8+ lymphocytes predict clinical outcome in breast cancer. J Clin Oncol 2011;29:1949-55. [Crossref] [PubMed]
- Lu P, Wang Y, Liu X, et al. Malignant gliomas induce and exploit astrocytic mesenchymal-like transition by activating canonical Wnt/β-catenin signaling. Med Oncol 2016;33:66. [Crossref] [PubMed]
- Zhang F, Cai HB, Liu HZ, et al. High Expression of CISD2 in Relation to Adverse Outcome and Abnormal Immune Cell Infiltration in Glioma. Dis Markers 2022;2022:8133505. [Crossref] [PubMed]
- Zheng YX, Shi S, Jiang XH, et al. Comprehensive analysis of protein phosphatase 1 regulatory inhibitor subunit 14B, a molecule related to tumorigenesis, poor prognosis, and immune cell infiltration in lung adenocarcinoma. Am J Transl Res 2023;15:858-77. [PubMed]
- Kavarthapu R, Dufau ML. Prolactin receptor gene transcriptional control, regulatory modalities relevant to breast cancer resistance and invasiveness. Front Endocrinol (Lausanne) 2022;13:949396. [Crossref] [PubMed]
- Li B, Li H, Zhang L, et al. Expression analysis of human glioma susceptibility gene and P53 in human glioma and its clinical significance based on bioinformatics. Ann Transl Med 2023;11:53. [Crossref] [PubMed]