Regulatory T cell-associated gene signature correlates with prognostic risk and immune infiltration in patients with breast cancer
Highlight box
Key findings
• This study developed a novel regulatory T cell (Treg)-associated gene signature, which could predict breast cancer prognosis and was related to immunotherapy response and chemotherapy drug sensitivity.
What is known and what is new?
• Tregs are crucial in the development, prognosis, and treatment of breast cancer.
• Six Treg-associated genes including TBC1D4, PMAIP1, IFNG, LEF1, MZB1 and EZR were identified to construct a prognostic signature for breast cancer.
What is the implication, and what should change now?
• The Treg-associated gene signature may be a promising prognostic factor in breast cancer, which contributes to accurate prognosis evaluation and individualized treatment for breast cancer.
Introduction
Breast cancer is the most commonly diagnosed cancer worldwide, with an estimated 2.3 million new cases (11.7% of total cancer cases) and 685,000 deaths (6.9% of all deaths caused by cancers) from breast cancer in 2020 (1). Being a heterogeneous disorder, breast cancer is divided into different histologic (primarily lobular and ductal) and molecular subtypes, with diverse biological and clinical features as well as treatment options and outcomes (2-4). Such heterogeneity makes the classification and treatment of breast cancer enter the era of precision therapy. Although the use of adjuvant chemotherapy, radiotherapy, targeted therapy, and endocrine therapy has significantly reduced the risk of death in patients, some patients, especially those with advanced metastatic disease, are still unable to benefit from existing treatments (5-7). Recently, increasing evidence supports the close involvement of the immune system in the development and progression of breast cancer, and its critical role in determining patient response to treatment and their long-term survival (8-10).
Regulatory T cells (Tregs) are subset of CD4+ T cell populations, which specialize in suppressing abnormal/excessive immune responses to maintain immune tolerance and homeostasis (11,12). Emerging evidence indicates that Treg cells abundantly infiltrate into tumor tissues and involves in tumor development and progression by suppressing effective tumor immunity, which is frequently linked to worse prognosis of cancer patients (13-15). Consequently, depletion of tumor-resident Treg cells and/or modulating Treg activity have been proposed as hopeful immunotherapy strategies in breast cancer (16,17). For example, annexin A1 was found to be linked to poor survival of breast cancer by enhancing the inhibition function of Treg cells, while repressing Treg cell function by targeting annexin A1 could decrease tumor size (18). Focal radiotherapy and dual blockade of activin A and TGF-β was linked to decreased tumor recurrence rate in breast cancer by reducing Treg-mediated immunosuppression (19).
Considering the close association between Tregs and prognosis of patients with breast cancer, we intended to identify a Treg-associated gene signature to predict the prognosis of breast cancer patients, which has not been investigated in breast cancer research. Based on the single-cell RNA-sequencing (scRNA-seq) data in TISCH2 database and the expression and clinical data in The Cancer Genome Altas (TCGA) database, a Treg-associated prognostic signature was identified, which stratified breast cancer patients into different risk groups with different prognosis. In addition, the associations of this signature with clinical features, immune infiltrates, molecular pathways, mutation and drug sensitivity were further explored. This study contributes to make a comprehensive understanding of the role of Tregs in the development and prognosis of breast cancer. We present this article in accordance with the TRIPOD reporting checklist (available at https://tcr.amegroups.com/article/view/10.21037/tcr-24-1118/rc).
Methods
Data acquisition and preprocessing
Data of RNA-seq, clinical phenotype and survival for breast cancer samples in TCGA database were downloaded. Totally 1,113 tumor tissues and 113 normal tissue samples were obtained. Tumor samples without survival data or samples with a follow-up less than 30 days were removed, and 1,047 tumor samples were finally included. The detail information of samples, including age, stage, and histological types were listed in the online table (available at https://cdn.amegroups.cn/static/public/tcr-24-1118-1.pdf).
Two microarray datasets (GSE58812 and GSE42568) of breast cancer were also obtained from the Gene Expression Omnibus (GEO) database. Only the tumor samples with survival data were included in this study. Probes were annotated into gene symbols, and the mean value was regarded as the expression level of this gene when multiple probes matched to the same gene symbol. Samples in the two datasets were merged into a large one after eliminating batch effect using the Combat function of SVA package (version 3.46.0). This merged dataset was used as an external validation dataset. The study was conducted in accordance with the Declaration of Helsinki (as revised in 2013).
TISCH2 database
TISCH2 is a scRNA-seq database containing 190 datasets from 50 cancer types and 20 tissue types, which provides detailed cell-type annotation at the single-cell level (20). Two breast cancer-associated datasets (GSE110686 and GSE114727) that contained Treg cell annotations were selected from TISCH2 database, and differentially expressed gene (DEG) list at Treg cell level in these two datasets were downloaded.
Differential expression analysis
The DEGs in tumor vs. normal samples in TCGA cohort were screened by differential expression analysis using DESeq2 package (version 1.38.3), with false discovery rate (FDR) <0.05 and |log2 fold change (FC)| >1 used as cut-off values.
Treg-associated genes
The shared genes between DEGs screened in TCGA-cohort and the Treg-DEGs screened using TISCH2 were selected as Treg-associated genes and were used for subsequent analysis. The interactions among these genes were analyzed based on STRING database with a minimum required interaction score setting as 0.4, and a protein-protein interaction (PPI) network was constructed using Cytoscape software (version 3.9.1).
Establishment and validation of a Treg-associated gene signature
The tumor samples in TCGA-cohort were randomly assigned into TCGA-training and TCGA-validation sets at a ratio of 7:3. Based on TCGA-training set, prognostic value of Treg-associated genes was explored using both univariate cox regression and Kaplan-Meier (KM) survival analysis of the survival package (version 3.5-5). Only the genes with P<0.05 in both univariate cox and KM survival analysis were selected as prognostic genes. Next, the most contributing prognostic gene were identified by the least absolute shrinkage and selection operator (LASSO) cox regression with 10-fold cross-validation using glmnet package (version 4.1-7). A prognostic risk model, named Treg-associated gene signature, was established using multivariable COX stepwise regression as per the formula: , in which βgene and Expgene represent the regression coefficient and the expression level of genes, respectively. Risk score of samples were calculated to assign tumor samples into two risk groups based on their median value. Survival probability between the two risk groups was compared using KM survival analysis. The prognostic model was evaluated by receiver operating characteristic (ROC) curves using survivalROC package (version 1.0.3.1). The prognostic model was validated in both TCGA-validation and GEO external validation sets.
Establishment and evaluation of clinical nomogram model
To investigate whether the risk score of the Treg-associated gene signature was an independent prognostic factor from various clinical factors, univariate and multivariate Cox regression analyses for risk score and clinical factors were conducted to identify the independent prognostic factors. The identified factors were further used to establish a prognostic nomogram using rms package (version 6.7-0). A nomoScore was calculated for each sample based on the nomogram model, and then was used to assign patients into high- and low-nomoScore groups according to their median value. Survival probability between two nomoScore groups was compared using KM survival analysis. The predictive power and the accuracy of the nomogram model were evaluated by ROC, calibration and decision curves using rms (version 6.7-0) and ggDCA packages (version 1.2).
Evaluation of immune infiltrates
The association between the Treg-associated gene signature and immune infiltration was analyzed. Fractions of infiltrating immune cells in tumor microenvironment were evaluated using both single-sample gene set enrichment analysis (ssGSEA) method in gene set variation analysis (GSVA) package (21). This algorithm was commonly used for evaluating fractions of 28 kinds of immune cells, respectively. Moreover, stromal and immune scores were calculated by means of Estimation of STromal and Immune cells in MAlignant Tumor tissues using Expression data (ESTIMATE) algorithm (22), and the sum of these two score were ESTIMATE score, which could indirectly reflect the tumor purity. The differences on immune infiltrates and scores between the two risk groups were compared using Wilcoxon test.
Gene set enrichment analysis (GSEA)
To explore the biological mechanisms underlying the Treg-associated gene signature, differential expression analysis in high- vs. low-risk groups was conducted using DESeq2, and all the genes were ranked according their log2FC value. Then, the differed Kyoto Encyclopedia of Genes and Genomes (KEGG) pathways were explored by GSEA analysis using ClusterProfiler package (version 4.7.1.003).
Somatic mutation and drug sensitivity analyses for two risk groups
Based on the somatic mutation profiles provided in TCGA, the mutation frequency of genes was analyzed, and the top 20 mutated genes were visualized in Waterfall plots using Maftools package (version 2.14.0). Then, tumor mutation burden (TMB) was calculated. Sensitivity of each sample to chemotherapeutic drugs was estimated based on the Genomics of Drug Sensitivity in Cancer (GDSC) database, and was quantified as half maximal inhibitory concentration (IC50) value using pRRophetic package (version 0.5). Differences on TMB and drug sensitivity between two risk groups were compared using Wilcoxon test.
Statistical analysis
The bioinformatics analyses were performed with R software (version 4.2.3), with P<0.05 indicating a statistically significant difference.
Results
Identification of Treg-associated genes
Based on TISCH2 database, two breast cancer-associated datasets (GSE110686 and GSE114727) containing Treg cell annotations were selected (Figure 1A). DEG list at Treg cell level in these two datasets were downloaded, and 769 Treg-DEGs were obtained after removing the repeated genes. Meanwhile, 5055 DEGs were screened in tumor vs. normal in TCGA-cohort by differential analysis, with the cutoff value of FDR <0.05 and |log2FC| >1 (Figure 1B). Finally, Venn diagram revealed 169 shared genes between DEGs screened in TCGA-cohort and the Treg-DEGs screened by TISCH2 database (Figure 1C). These 169 genes were considered as Treg-associated genes, and were used for subsequent analysis.
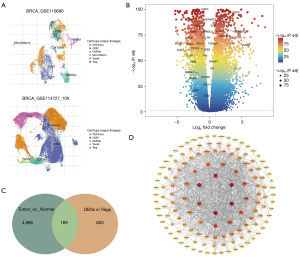
PPI network indicated close interactions among these Treg-associated genes (Figure 1D). In this network, genes such as IFNG, STAT1, FOXP3, FOXP3, GAPDH and CCL5 exhibited interactions with much more genes.
Establishment and validation of a Treg-associated gene signature
Among the 169 Treg-associated genes, 29 prognostic genes were identified by both univariate cox and KM survival analysis (all P<0.05). As shown in the forest plot, these genes seemed to be protective genes in breast cancer with hazard ratio (HR) less than 1, in exception to EZR whose HR was 1.236 (Figure 2A). Further LASSO-Cox identified 10 most contributing prognostic gene (Figure 2B,2C). Next, multivariable Cox stepwise regression identified a Treg-associated 6-gene prognostic signature (Figure 2D), including TBC1D4, PMAIP1, IFNG, LEF1, MZB1 and EZR. Risk score of each sample was then calculated according to the formula: TBC1D4*(−0.191432846) + PMAIP1*(−0.191675111) + IFNG*(−0.335955314) + LEF1*(−0.254642836) + MZB1*(−0.194623458) + EZR*0.23483348. Patients in high-risk group exhibited a worse survival probability than those in low-risk group (P<0.001, Figure 3A). The Treg-associated gene signature showed a moderate predictive power for 1-, 3- and 5-year survival for breast cancer patients with an area under the curve (AUC) of 0.705, 0.678 and 0.668, respectively (Figure 3B). The prognostic signature was further evaluated in two validation datasets, and similar results were observed (Figure 3C-3F). Patients with high risk had poor survival probability than patients with low risk in both TCGA-validation set (P=0.003, Figure 3C) and GEO external validation set (P=0.003, Figure 3E). The prognostic signature showed a moderate predictive power for 1-, 3- and 5-year survival for breast cancer patients with an AUC of 0.788, 0.698 and 0.648 in TCGA-validation set (Figure 3D) and an AUC of 0.616, 0.677 and 0.692 in GEO external validation set (Figure 3F).
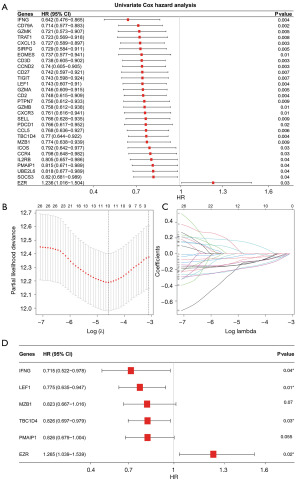
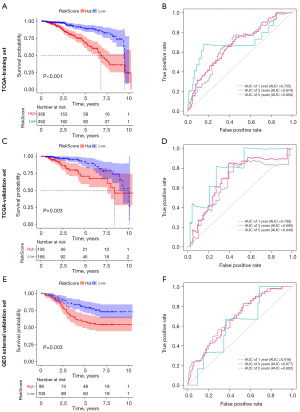
Associations of the Treg-associated gene signature with clinical pathological factors
Breast cancer patients in high-risk were relatively older with a median age over 60 years (Figure 4A). In terms of the associations between the Treg-associated gene signature and tumor staging system, American Joint Committee on Cancer (AJCC)_T4 patients seemed to have higher risk score than AJCC_T1–T3 patients (all P<0.05, Figure 4B), whereas no associations were observed between risk score and lymph node (AJCC_N, Figure 4C) and metastasis (AJCC_M, Figure 4D) and tumor stages (Figure 4E). Meanwhile, associations of the Treg-associated gene signature with breast cancer subtypes were further analyzed. No significant difference on risk score was observed between progesterone receptor (PR)-positive and PR-negative patients (Figure 4F). Interestingly, estrogen receptor (ER)-positive patients showed higher risk score than ER-negative patients (Figure 4G), and human epidermal growth factor receptor-2 (HER-2)-positive patients showed higher risk score than HER-2-negative patients (Figure 4H). Moreover, significant difference on risk score was also observed between non-triple-negative breast cancer (TNBC) and TNBC patients (Figure 4I). These results indicated a close association between the Treg-associated gene signature with clinical pathological factors.
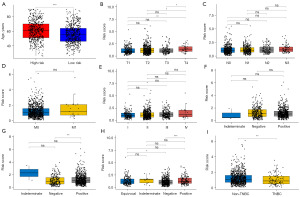
Establishment and evaluation of clinical nomogram model
Three independent prognostic factors were further identified based on univariate and multivariate Cox regression analyses, including age, tumor stages and risk score (Figure 5A,5B). Therefore, age, tumor stage, and risk score were combined to further establish a nomogram model (Figure 5C). Patients with high-nomoScore exhibited obviously worse survival outcomes than patients with low-nomoScore (P<0.001, Figure 5D). ROC curves for nomogram model showed an AUC for predicting 1-, 3- and 5-year survival of 0.861, 0.774 and 0.747, respectively (Figure 5E), indicating an improved predictive power of nomogram model than the Treg-associated gene signature alone. This was confirmed by decision curves, that was, the nomogram model had the largest net benefit than the three factors alone (Figure 5F). Calibration curves indicated a high consistency between the estimated and actual survival rates (Figure 5G). The above results suggested that nomogram model performed well for predicting survival for breast cancer patients.
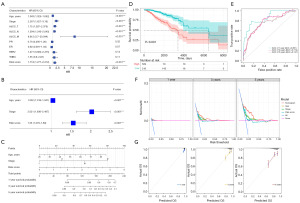
Associations of the Treg-associated gene signature with immune infiltrates
Immune infiltrates were evaluated by ssGSEA algorithms. ssGSEA algorithm estimated the infiltration fraction of 28 immune cells, among which 21 immune cells were significantly differed (Figure 6A). For example, samples in low-risk group showed higher infiltration fraction of activated B cell/CD8+ T cell/CD4+ T cell, effector memory CD8+ T cell, immature B cell, myeloid-derived suppressor cell (MDSC) and Treg cells. Whereas samples in high-risk group exhibited higher infiltration fraction type 17 T helper cell, neutrophil, immature dendritic cell, CD56dim/CD56bright natural killer cell. Abundance of the majority of infiltrating immune cells in tumor microenvironment of samples was differed between high- and low-risk groups, indicating that risk score was associated with immune infiltrates. This was confirmed by the significant differences on immune and stromal scores between two risk groups (Figure 6B).
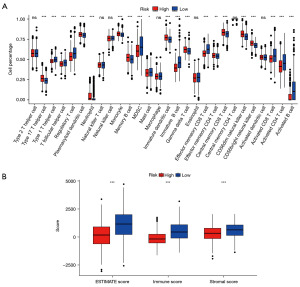
Potential pathways associated with the Treg-associated gene signature
GSEA revealed 99 pathways that significantly differed between two risk groups, of which 23 pathways were markedly enriched in high-risk group, such as oxidative phosphorylation, ribosome and chemical carcinogenesis-reactive oxygen species (Figure 7A). Conversely, 76 pathways were markedly enriched in low-risk groups, such as antigen processing and presentation, cytokine-cytokine receptor interaction and primary immunodeficiency (Figure 7B).
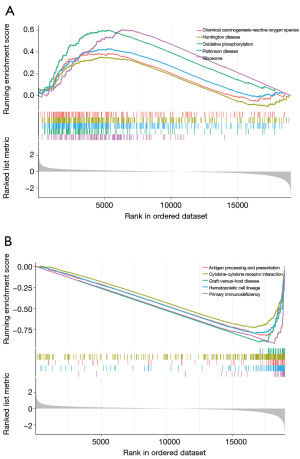
Associations of the Treg-associated gene signature with somatic mutation
Mutation frequency of genes was analyzed using Maftools package. The top 20 mutated genes are shown in Figure S1A,S1B, samples in high- and low-risk groups shared 15 mutated genes. TP53 (32% vs. 35%), PIK3CA (34% vs. 35%) and TTN (16% vs. 19%) were the top 3 mutated genes in samples in both two groups. TMB was further calculated, which showed no significant differences between two risk groups (Figure S1C).
Associations of the Treg-associated gene signature with drug sensitivity
Sensitivity of each sample to 128 chemotherapeutic drugs was estimated based on GDSC database, and was quantified as IC50 value. The IC50 value of 83 chemotherapeutic drugs was significantly differed between the two risk groups. For example, samples in high-risk group showed lower IC50 to lapatinib, while showed higher IC50 to methotrexate, gefitinib, cyclopamine, pyrimethamine and parthenolide than samples in low-risk group (all P<0.001, Figure 8). This indicated that patients in high-risk groups might be more sensitive to lapatinib, while patients in low-risk groups might be more sensitive to methotrexate, gefitinib, cyclopamine, pyrimethamine, and parthenolide.
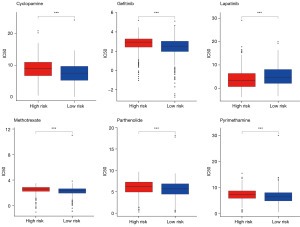
Discussion
Tregs acts crucial roles in tumor microenvironment, especially in inducing immune evasion, which is immunosuppressive in breast cancer to facilitate tumor growth and metastasis (23). Depletion of tumor-resident Treg cells and/or modulating Treg activity have been proposed as hopeful immunotherapy strategies in breast cancer (16,17). In this study, based on the integrated analysis of scRNA-seq and transcriptome data, we developed a Tregs-associated prognostic signature (TBC1D4, PMAIP1, IFNG, LEF1, MZB1 and EZR) for patients with breast cancer, which was related to prognosis, immune infiltration, and drug sensitivity.
TBC1D4, also named AS160, encodes a Rab-GTPase-activating protein. TBC1D4 phosphorylation has been linked to regulate glucose transport, while dysregulated energy metabolism, in particular massive cellular glucose uptake is a hallmark of malignant tumors (24,25). Phosphorylation level of TBC1D4 was found to frequently increase in breast cancer (26). PMAIP1, also known as NOXA, belongs to the BH3-only pro-apoptotic protein of the Bcl-2 family, which determines whether a cell commits to apoptosis (27). IFNG [interferon-gamma (IFN-γ)] has long been documented as an important modulator of anti-tumor immunomodulatory, which can be hijacked by tumor cells to initiate IFN-γ resistance (28,29). In breast cancer, SFV/IFN-γ vector could inhibit tumor growth in orthotopic 4T1 mouse model by inducing anti-tumor T-cell response (decreased Treg and increased CD4+ and CD8+ cells populations) (30). LEF1 could potentiate anti-tumor activity of natural killer T cells by driving a central memory program (31,32). Elevated LEF1 levels was observed in invasive micropapillary breast carcinoma, which was linked to lymphovascular invasion and nodal metastasis (33). LEF1 regulated glutathione metabolism and intracellular reactive oxygen species in breast cancer, thus contributing metastatic brain colonization (34). MZB1 encodes a molecular chaperone primarily expressing in endoplasmic reticulum of B lymphocytes, which involves in cancer progression by its roles in endoplasmic reticulum stress (35,36). MZB1 was highly expressed in ER-positive breast cancer, and its expression frequently indicated a shorter survival and a more advanced tumor stage (36). EZR (ezrin) is a well-known oncogenic gene regulating cell adhesion and migration. Expression of EZR was markedly elevated in breast cancer indicating a poor survival (37), and EZR knockdown repressed metastasis and chemoresistance in breast cancer (38). Findings from the above studies highlighted the prognostic associations of these six genes in breast cancer.
The risk score model developed based on this 6-gene prognostic signature showed a moderate predictive power for survival of breast cancer patients (Figure 3), and was an independent prognostic factor (Figure 5B). This prognostic signature showed close associations with breast cancer clinicopathologic features, in particular, with molecular subtypes (Figure 4G-4I). Therefore, we further established a nomogram by combining risk score and independent prognostic clinical factors, including age and tumor stage. In oncology, nomogram is widely employed for predicting patient outcomes. This model can combine a range of prognostic and determinant variables to produce individual probabilities for clinical events, thus fulfilling the demand for integrated models that merge biological and clinical data. With the user-friendly digital interfaces, nomogram facilitates quick calculations and delivers results that are easily interpretable (39,40). Nomogram has been confirmed as an efficient tool to provide prognostic prediction for breast cancer patients (41,42). In this study, the nomogram showed an improved predictive power for patients with breast cancer than the Treg-associated gene signature alone, as indicated by the AUC of 0.861, 0.774 and 0.747 for predicting 1-, 3- and 5-year survival, respectively (Figure 5E). Therefore, we conclude that the established nomogram may be a useful tool for predicating breast cancer prognosis and aiding in individualized healthcare decision-making.
The 6-gene Treg-associated prognostic signature showed close associations with immune infiltrates. The risk groups divided by prognostic risk score showed significantly different tumor-infiltrating immune cells, such as activated B cell/CD8+ T cell/CD4+ T cell, effector memory CD8+ T cell, type 17 T helper cell, neutrophil, immature dendritic cell, and natural killer cells (Figure 6A). These immune cells play important roles in tumor microenvironment, thus mediating tumor progression and response to treatments (43-47). For example, CD8+ T cells, the major anti-tumor effector cells, its tissue-resident memory phenotype was related to better outcomes in TNBC patients, which exhibited enhanced cytotoxic capacity than exhaustion phenotype in anti-cytotoxic T-lymphocyte antigen 4 (CTLA-4) and anti-programmed death-1 (PD-1) therapy, and provided local immune protection (48). Additionally, breast cancer patients with low-risk had relatively higher immune and stromal scores, indicating an immunoactivated state. Consistently, multiple immune related pathways were activated in low-risk groups, such as antigen processing and presentation, cytokine-cytokine receptor interaction and primary immunodeficiency (Figure 7B). Moreover, the 6-gene Treg-associated prognostic signature also showed tight connections with the sensitivity to chemotherapeutic drugs. The estimated IC50 value of many chemotherapeutic drugs was significantly differed between the two risk groups. For example, breast cancer patients with high-risk showed lower IC50 to lapatinib, indicating a more sensitive to lapatinib for these patients (Figure 8). All these findings suggested that the Treg-associated gene signature might contribute to identify the breast cancer subpopulations who might benefit from immunotherapy or chemotherapeutic drugs.
Conclusions
In summary, a six-gene prognostic signature based on Treg-associated genes was identified to predict prognosis in breast cancer patients, which could be an independent prognostic factor in breast cancer. This Treg-associated prognostic signature showed close associations with immune infiltrates and therapeutic responses, which contributed to make clinical decision regarding individualized treatment of breast cancer patients who could benefit from immunotherapy and/or chemotherapy.
Acknowledgments
Funding: This work was supported by
Footnote
Reporting Checklist: The authors have completed the TRIPOD reporting checklist. Available at https://tcr.amegroups.com/article/view/10.21037/tcr-24-1118/rc
Peer Review File: Available at https://tcr.amegroups.com/article/view/10.21037/tcr-24-1118/prf
Conflicts of Interest: All authors have completed the ICMJE uniform disclosure form (available at https://tcr.amegroups.com/article/view/10.21037/tcr-24-1118/coif). The authors have no conflicts of interest to declare.
Ethical Statement: The authors are accountable for all aspects of the work in ensuring that questions related to the accuracy or integrity of any part of the work are appropriately investigated and resolved. The study was conducted in accordance with the Declaration of Helsinki (as revised in 2013).
Open Access Statement: This is an Open Access article distributed in accordance with the Creative Commons Attribution-NonCommercial-NoDerivs 4.0 International License (CC BY-NC-ND 4.0), which permits the non-commercial replication and distribution of the article with the strict proviso that no changes or edits are made and the original work is properly cited (including links to both the formal publication through the relevant DOI and the license). See: https://creativecommons.org/licenses/by-nc-nd/4.0/.
References
- Sung H, Ferlay J, Siegel RL, et al. Global Cancer Statistics 2020: GLOBOCAN Estimates of Incidence and Mortality Worldwide for 36 Cancers in 185 Countries. CA Cancer J Clin 2021;71:209-49. [Crossref] [PubMed]
- Li Y, Zhang H, Merkher Y, et al. Recent advances in therapeutic strategies for triple-negative breast cancer. J Hematol Oncol 2022;15:121. [Crossref] [PubMed]
- Nicolò E, Tarantino P, Curigliano G. Biology and Treatment of HER2-Low Breast Cancer. Hematol Oncol Clin North Am 2023;37:117-32. [Crossref] [PubMed]
- Onkar SS, Carleton NM, Lucas PC, et al. The Great Immune Escape: Understanding the Divergent Immune Response in Breast Cancer Subtypes. Cancer Discov 2023;13:23-40. [Crossref] [PubMed]
- Nolan E, Lindeman GJ, Visvader JE. Deciphering breast cancer: from biology to the clinic. Cell 2023;186:1708-28. [Crossref] [PubMed]
- Hurvitz SA, Hegg R, Chung WP, et al. Trastuzumab deruxtecan versus trastuzumab emtansine in patients with HER2-positive metastatic breast cancer: updated results from DESTINY-Breast03, a randomised, open-label, phase 3 trial. Lancet 2023;401:105-17. [Crossref] [PubMed]
- Ben-Dror J, Shalamov M, Sonnenblick A. The History of Early Breast Cancer Treatment. Genes (Basel) 2022;13:960. [Crossref] [PubMed]
- Pilipow K, Darwich A, Losurdo A. T-cell-based breast cancer immunotherapy. Semin Cancer Biol 2021;72:90-101. [Crossref] [PubMed]
- Barzaman K, Moradi-Kalbolandi S, Hosseinzadeh A, et al. Breast cancer immunotherapy: Current and novel approaches. Int Immunopharmacol 2021;98:107886. [Crossref] [PubMed]
- Dvir K, Giordano S, Leone JP. Immunotherapy in Breast Cancer. Int J Mol Sci 2024;25:7517. [Crossref] [PubMed]
- Bluestone JA, McKenzie BS, Beilke J, et al. Opportunities for Treg cell therapy for the treatment of human disease. Front Immunol 2023;14:1166135. [Crossref] [PubMed]
- Song X, Chen R, Li J, et al. Fragile Treg cells: Traitors in immune homeostasis? Pharmacol Res 2024;206:107297. [Crossref] [PubMed]
- Deng G. Tumor-infiltrating regulatory T cells: origins and features. Am J Clin Exp Immunol 2018;7:81-7. [PubMed]
- Dikiy S, Rudensky AY. Principles of regulatory T cell function. Immunity 2023;56:240-55. [Crossref] [PubMed]
- Tanaka A, Sakaguchi S. Targeting Treg cells in cancer immunotherapy. Eur J Immunol 2019;49:1140-6. [Crossref] [PubMed]
- Plitas G, Konopacki C, Wu K, et al. Regulatory T Cells Exhibit Distinct Features in Human Breast Cancer. Immunity 2016;45:1122-34. [Crossref] [PubMed]
- Malla RR, Vasudevaraju P, Vempati RK, et al. Regulatory T cells: Their role in triple-negative breast cancer progression and metastasis. Cancer 2022;128:1171-83. [Crossref] [PubMed]
- Bai F, Zhang P, Fu Y, et al. Targeting ANXA1 abrogates Treg-mediated immune suppression in triple-negative breast cancer. J Immunother Cancer 2020;8:e000169. [Crossref] [PubMed]
- De Martino M, Daviaud C, Diamond JM, et al. Activin A Promotes Regulatory T-cell-Mediated Immunosuppression in Irradiated Breast Cancer. Cancer Immunol Res 2021;9:89-102. [Crossref] [PubMed]
- Han Y, Wang Y, Dong X, et al. TISCH2: expanded datasets and new tools for single-cell transcriptome analyses of the tumor microenvironment. Nucleic Acids Res 2023;51:D1425-31. [Crossref] [PubMed]
- Hänzelmann S, Castelo R, Guinney J. GSVA: gene set variation analysis for microarray and RNA-seq data. BMC Bioinformatics 2013;14:7. [Crossref] [PubMed]
- Yoshihara K, Shahmoradgoli M, Martínez E, et al. Inferring tumour purity and stromal and immune cell admixture from expression data. Nat Commun 2013;4:2612. [Crossref] [PubMed]
- Hinshaw DC, Benavides GA, Metge BJ, et al. Hedgehog Signaling Regulates Treg to Th17 Conversion Through Metabolic Rewiring in Breast Cancer. Cancer Immunol Res 2023;11:687-702. [Crossref] [PubMed]
- Fukushi A, Kim HD, Chang YC, et al. Revisited Metabolic Control and Reprogramming Cancers by Means of the Warburg Effect in Tumor Cells. Int J Mol Sci 2022;23:10037. [Crossref] [PubMed]
- Wang H, Zheng A, Arias EB, et al. AS160 expression, but not AS160 Serine-588, Threonine-642, and Serine-704 phosphorylation, is essential for elevated insulin-stimulated glucose uptake by skeletal muscle from female rats after acute exercise. FASEB J 2023;37:e23021. [Crossref] [PubMed]
- Jiang XH, Sun JW, Xu M, et al. Frequent hyperphosphorylation of AS160 in breast cancer. Cancer Biol Ther 2010;10:362-7. [Crossref] [PubMed]
- Morsi RZ, Hage-Sleiman R, Kobeissy H, et al. Noxa: Role in Cancer Pathogenesis and Treatment. Curr Cancer Drug Targets 2018;18:914-28. [Crossref] [PubMed]
- Han J, Wu M, Liu Z. Dysregulation in IFN-γ signaling and response: the barricade to tumor immunotherapy. Front Immunol 2023;14:1190333. [Crossref] [PubMed]
- Burke JD, Young HA. IFN-γ: A cytokine at the right time, is in the right place. Semin Immunol 2019;43:101280. [Crossref] [PubMed]
- Trofimova O, Korotkaja K, Skrastina D, et al. Alphavirus-Driven Interferon Gamma (IFNg) Expression Inhibits Tumor Growth in Orthotopic 4T1 Breast Cancer Model. Vaccines (Basel) 2021;9:1247. [Crossref] [PubMed]
- Van Kaer L. LEF1 Creates Memories in iNKT Cells That Potentiate Antitumor Immunity. Cancer Immunol Res 2023;11:144. [Crossref] [PubMed]
- Ngai H, Barragan GA, Tian G, et al. LEF1 Drives a Central Memory Program and Supports Antitumor Activity of Natural Killer T Cells. Cancer Immunol Res 2023;11:171-83. [Crossref] [PubMed]
- Dolezal D, Zhang X, Harigopal M. Increased Expression of LEF1 and β-Catenin in Invasive Micropapillary Carcinoma of the Breast is Associated With Lymphovascular Invasion and Lymph Node Metastasis. Appl Immunohistochem Mol Morphol 2022;30:557-65. [Crossref] [PubMed]
- Blazquez R, Rietkötter E, Wenske B, et al. LEF1 supports metastatic brain colonization by regulating glutathione metabolism and increasing ROS resistance in breast cancer. Int J Cancer 2020;146:3170-83. [Crossref] [PubMed]
- Rosenbaum M, Andreani V, Kapoor T, et al. MZB1 is a GRP94 cochaperone that enables proper immunoglobulin heavy chain biosynthesis upon ER stress. Genes Dev 2014;28:1165-78. [Crossref] [PubMed]
- Watanabe M, Shibata M, Inaishi T, et al. MZB1 expression indicates poor prognosis in estrogen receptor-positive breast cancer. Oncol Lett 2020;20:198. [Crossref] [PubMed]
- Zhang R, Zhang S, Xing R, et al. High expression of EZR (ezrin) gene is correlated with the poor overall survival of breast cancer patients. Thorac Cancer 2019;10:1953-61. [Crossref] [PubMed]
- Xiao G, Cheng F, Yuan J, et al. Integrative multiomics analysis identifies a metastasis-related gene signature and the potential oncogenic role of EZR in breast cancer. Oncol Res 2022;30:35-51. [Crossref] [PubMed]
- Balachandran VP, Gonen M, Smith JJ, et al. Nomograms in oncology: more than meets the eye. Lancet Oncol 2015;16:e173-80. [Crossref] [PubMed]
- Iasonos A, Schrag D, Raj GV, et al. How to build and interpret a nomogram for cancer prognosis. J Clin Oncol 2008;26:1364-70. [Crossref] [PubMed]
- Lin S, Mo H, Li Y, et al. Development and validation of a nomogram for predicting survival of advanced breast cancer patients in China. Breast 2020;53:172-80. [Crossref] [PubMed]
- Chen MS, Liu PC, Yi JZ, et al. Development and validation of nomograms for predicting survival in patients with de novo metastatic triple-negative breast cancer. Sci Rep 2022;12:14659. [Crossref] [PubMed]
- Park J, Hsueh PC, Li Z, et al. Microenvironment-driven metabolic adaptations guiding CD8(+) T cell anti-tumor immunity. Immunity 2023;56:32-42. [Crossref] [PubMed]
- Blomberg OS, Spagnuolo L, Garner H, et al. IL-5-producing CD4(+) T cells and eosinophils cooperate to enhance response to immune checkpoint blockade in breast cancer. Cancer Cell 2023;41:106-123.e10. [Crossref] [PubMed]
- Shibabaw T, Teferi B, Ayelign B. The role of Th-17 cells and IL-17 in the metastatic spread of breast cancer: As a means of prognosis and therapeutic target. Front Immunol 2023;14:1094823. [Crossref] [PubMed]
- Razeghian E, Kameh MC, Shafiee S, et al. The role of the natural killer (NK) cell modulation in breast cancer incidence and progress. Mol Biol Rep 2022;49:10935-48. [Crossref] [PubMed]
- Rodríguez-Bejarano OH, Parra-López C, Patarroyo MA. A review concerning the breast cancer-related tumour microenvironment. Crit Rev Oncol Hematol 2024;199:104389. [Crossref] [PubMed]
- Virassamy B, Caramia F, Savas P, et al. Intratumoral CD8(+) T cells with a tissue-resident memory phenotype mediate local immunity and immune checkpoint responses in breast cancer. Cancer Cell 2023;41:585-601.e8. [Crossref] [PubMed]