scRNA-seq reveals chemotherapy-induced tumor microenvironment changes in pancreatic ductal adenocarcinoma
Highlight box
Key findings
• The results of our single-cell RNA sequencing (scRNA-seq) data analysis showed that chemotherapy altered the mechanism of antigen presentation and modulated the tumor microenvironment (TME), which may lead to tumor drug resistance and recurrence. LYZ was significantly increased in the tumor cells in response to chemotherapy. Moreover, chemotherapy affected the receptor of polio virus receptor (PVR) signaling, which shifted from TIGIT in T cells to CD226 in myeloid cells.
What is known and what is new?
• Poor outcomes have fueled ongoing efforts to exploit the TME for therapeutic strategies, which have largely failed. Chemotherapy may generate favorable tumor neoantigens to induce T-cell responses and potentiate antitumor activity, thereby enhancing the efficacy of immuno-therapies.
• LYZ is usually used as a myeloid marker, and has been overlooked in the past due to the huge amount of data generated from scRNA-seq. We described the characteristics of cross-cell type marker expression in pancreatic ductal adenocarcinoma (PDAC). Chemotherapy altered the TME balance, changing the tumor antigen presentation and PVR signaling.
What is the implication, and what should change now?
• These pathways and key genes may be the treatment targets in the future. Combining the appropriate chemical and immune therapies could improve the immune therapy response in PDAC.
Introduction
Pancreatic ductal adenocarcinoma (PDAC) is a highly aggressive tumor that demonstrates to be highly fatal in humans. Resection therapies are currently the only effective interventional strategies for PDAC. When combined with chemotherapeutics like gemcitabine and capecitabine or Gem and FOLFIRINOX, resection-based interventional therapies can achieve a 5-year survival rate of up to 18%. Unfortunately, this approach is only suitable for patients diagnosed at an early stage, who constitute fewer than one-fifth of all cases (1,2).
Immunotherapy has been shown to be remarkably effective in treating various types of tumors, and has revolutionized cancer treatments; however, thus far, it has failed to benefit PDAC patients (3-5). Chemotherapy may generate favorable tumor neoantigens to induce T-cell responses and potentiate antitumor activity, thereby enhancing the efficacy of immunotherapies (6,7). The combination of chemotherapy and immunotherapy represents a potentially promising clinical treatment strategy. However, research needs to be conducted to establish how cellular interactions change in the PDAC tumor microenvironment (TME) under chemotherapy conditions (8,9). The neoadjuvant chemotherapy research in pancreatic cancer revealed that the clonotype of T cell receptors (TCRs) was increasingly expanded in PDAC samples and the level of infiltrating CD8+ T cells was increased, which indicated that chemotherapy-induced metabolic reprogramming promotes anti-tumor immunity and chemo-response TCR sequencing analysis revealed enhanced reactivity of intratumoral immune components to eliminate tumor cells after chemotherapy (10).
This study used publicly available single-cell RNA sequencing (scRNA-seq) data (11) to investigate the standard chemotherapy effect on the PDAC TME. We analyzed a series of cell markers expressed in PDAC immune cells. We also established how chemotherapy reshapes signaling patterns in the PDAC TME. The implications for a rational treatment application are discussed. We present this article in accordance with the MDAR reporting checklist (available at https://tcr.amegroups.com/article/view/10.21037/tcr-2025-103/rc).
Methods
Data resources
All the scRNA-seq data used in this analysis were downloaded from the Gene Expression Omnibus (https://www.ncbi.nlm.nih.gov/geo/). The GSE20513 (11) dataset was used for the discovery cohort, while the GSE212966 (12) and GSE197177 (13) datasets were used for the validation cohort. To avoid sampling bias, the GSE20513, GSE212966, and GSE197177 datasets were filtered to use as training datasets, and only primary site samples were used for validation.
An immunohistochemistry staining image analysis and bulk gene expression analysis were conducted using data from the Human Protein Atlas database (https://www.proteinatlas.org/) and the Gene Expression Profiling Interactive Analysis (GEPIA) database (http://gepia.cancer-pku.cn/index.html), respectively. Additionally, the following human PDAC spatial transcriptome datasets were obtained: GSE233254 (GSM7421790, GSM7421791, and GSM7421792) (14), and GSE203612 (GSM6177618) (15). The study was conducted in accordance with the Declaration of Helsinki (as revised in 2013).
Data processing
Seurat 4.3.0.1 (16) on the R 4.3.0 platform was used to process and annotate the scRNA datasets and the spatial transcriptome data. scRNA-seq data were processed and analyzed using the Seurat package (version 4.3.0.1) in R (version 4.3.0). The raw gene expression matrix was loaded, and a Seurat object was created with genes detected in at least three cells and cells expressing at least 200 genes were retained. Quality control was performed by calculating the percentage of mitochondrial genes and filtering out cells with high mitochondrial gene content (>15%) or extreme gene counts. Data normalization was performed using the LogNormalize method with a scale factor of 10,000. Highly variable genes were identified using the variance-stabilizing transformation (VST) method, and 2,000 top variable genes were selected for downstream analysis. The data were scaled to regress out the effects of sequencing depth and mitochondrial gene content. Principal component analysis (PCA) was performed, and the top 20 principal components were used for cell clustering via the shared nearest neighbor (SNN) modularity optimization-based algorithm at a resolution of 0.5. Nonlinear dimensionality reduction was performed using Uniform Manifold Approximation and Projection (UMAP). Cluster-specific marker genes were identified using the FindAllMarkers function (log-fold change threshold >0.25, minimum fraction of cells >25%). Cell type annotation was performed based on known marker genes or using automated annotation tools.
In the present study, Harmony 0.1.1 (17), a preeminent data integration algorithm extensively utilized in single-cell analysis, was implemented. Specifically engineered to address the critical issue of batch effects in multi-batch single-cell datasets, Harmony 0.1.1 harnesses advanced statistical and computational methodologies. Through these means, it facilitates the seamless integration of heterogeneous single-cell data sources. This integration is pivotal in guaranteeing the precision and dependability of subsequent downstream analyses, including cell clustering and differential gene expression analysis with 20 dimensions used for reduction. DubletFinder 2.0.3 (18) was utilized in this research. It is specifically designed to precisely detect doublets within single-cell datasets. By implementing advanced computational algorithms and statistical frameworks, DubletFinder 2.0.3 can effectively discern doublets from authentic single-cell entities.
Well-known gene markers were used to conduct a comprehensive analysis: tumor cells: KRT119, EPCAM, CD24, and SOX9; endothelial cells: VWF and PECAM1; myeloid cells: CD14, CD68, CD163, C1QA, ITGAM, CKDKN1C, FCGR3A, and lysozyme (LYZ); cancer-associated fibroblasts (CAFs): DCN, COL1A1, and ACTA2 (CD74 and HLA-DRA were also used to characterize antigen presentation); natural killer cells: NKG7, GNLY, and CD34; T cells: CD3D, CD3E, CD4, CD8A, and CD8B; B cells: CD79A, MS4A1, and CD19; plasma cells: IGHM, IGHG1, and JCHAIN; mast cells: TPAB2, KIT, and TPAAB1; proliferation state: MKI67 and TOP2A.
Data assay
Transcriptomic profiling and functional enrichment analysis
The differentially expressed genes (DEGs) between the two cell groups were identified using the “FindMarkers” function (Seurat v5.1.0) with default parameters (log-fold change threshold =0.25, adjusted P<0.05). The top 100 genes ranked by statistical significance were subsequently subjected to pathway enrichment analysis using the Kyoto Encyclopedia of Genes and Genomes (KEGG) and Gene Ontology (GO) enrichment analyses with clusterProfiler 4.8.2 (19). Hallmark variation activity differences were quantified using Gene Set Variation Analysis (GSVA) 1.48.1 package (20), with normalized gene expression matrices an input.
Cell-cell communication mapping
The intercellular signaling networks were reconstructed using CellChat 1.6.1 (21) through systematic interrogation of ligand-receptor (LR) pair dynamics. The spatial transcriptome datasets underwent preprocessing via merging and Single Cell Transform normalization (Seurat v5.1.0), followed by reference-based cell type annotation using pre-trained classifiers. Cell type predictions were projected onto tissue sections via the FindTransferAnchors algorithm with default transfer learning parameters. No novel computational algorithms were developed during this study. All analytical workflows exclusively utilized established open-source tools as referenced.
Results
Typical markers expressed across different cell types in the PDAC TME
Our analysis utilized the scRNA-seq dataset GSE20513 (11) from the Gene Expression Omnibus. After applying stringent quality control measures and doublet filtering (see Methods), 56,871 high-quality cells were retained for downstream analysis. We identified 34 distinct cell clusters, most of which were annotated using well-established markers (Figure 1A,1B). These clusters comprised tumor cells (Clusters 0, 2, 4, 7, 11, 17, 19, 21, 22, 26, 27, 28, and 30), endothelial cells (Clusters 10 and 20), T cells (Clusters 1, 3, 15, 24, 31, and 32), B cells and plasma cells (Clusters 13 and 18), myeloid cells (Clusters 5, 8, 12, 14, and 23), CAFs (Clusters 6, 9, 16, 29, and 33), and mast cells (Cluster 25).
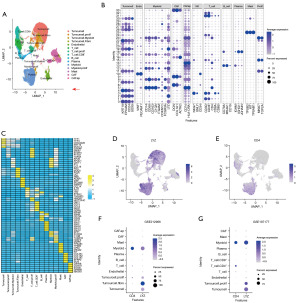
The cell markers exhibited notable cross-cellular expression (Figure1B,1C). For instance, nearly all the tumor cell clusters demonstrated high proportions and expression intensity of the myeloid marker LYZ (Figure 1B,1D). Cluster 17 co-expressed tumor and myeloid cell markers (APOE, APOC1, CHIT1, FTL, CCL18) and was designated “Tumor.Myeloid.”. Similarly, Cluster 19 co-expressed tumor and CAF markers (KRT19, EPCAM, CD24, DCN, and COL1A1) and was labeled “Tumor.Fibro”, with its top markers being PGC, MUC6, PIGR, LYZ, and HBB.
The cross-cellular expression of markers was also evident in immune cells. For instance, only a minor subset of T cells (CD3D+and CD3E+) expressed CD4 and/or CD8 (Figure 1B,1E). Among these, Cluster 3 exhibited prominent CD8 expression and was annotated as CD8+ T cells (“T_cell.CD8+”), consistent with prior findings (11). Notably, the myeloid cells displayed high CD4 and endothelial marker PECAM1 expression but minimal general T cell markers (Figure 1B,1E). The widespread CD4 expression across T cells and myeloid cells suggests a potential role for innate immune mechanisms in the PDAC TME.
LYZ expression was upregulated in tumor cells under chemotherapy, and is implicated in multiple metabolic processes
To confirm whether the cross-cellular expression of LYZ and CD4 could be attributable to the sample source, we analyzed two additional independent scRNA-seq datasets containing primary PDAC samples [GSE212966 (12) and GSE197177 (13)] using identical quality control and annotation protocols. The cross-cellular expression of CD4 and LYZ was consistent with our initial observations (Figure 1F,1G). Furthermore, LYZ expression was also detected across tumor cells and myeloid cell using an independent annotation approach (13). These findings suggest that LYZ might exert critical regulatory functions in the TME.
Data from the GEPIA and Human Protein Atlas databases confirmed that the LYZ gene was highly expressed in pancreatic cancer at both the bulk RNA and protein levels (Figure 2A,2B). Specifically, in the chemotherapy-treated group, the expression of the LYZ gene was significantly upregulated in the tumor cells, particularly in the “Tumorcell.Fibro” cluster (Cluster 19), which we previously identified as a marker-containing cluster for LYZ. In contrast, chemotherapy did not significantly affect LYZ expression in myeloid cells or the “Tumorcell.Myeloid” cluster (Figure 2C).
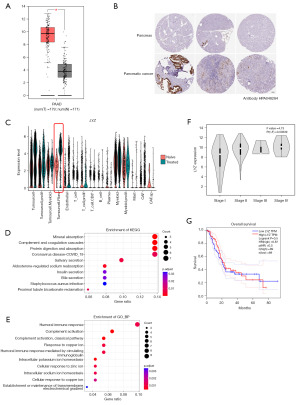
LYZ, a secretory protein with antibacterial function generally expressed in monocytes/macrophages, has been implicated in the prognostic of various tumors and possesses antimicrobial function (22). Recent evidence from a gene knockout study demonstrated that LYZ enhances the proliferation and migration of hepatocellular carcinoma cells via epithelial differentiation and lipid metabolism (23). However, the role of LYZ remains poorly characterized in the PDAC TME.
Given the limited information on LYZ in the PDAC TME, we conducted KEGG and GO enrichment analyses on the Cluster 19 of the chemotherapy-treated group. These analyses revealed that these genes primarily mediated cellular phagocytosis and possessed secretion functions (Figure 2D,2E). The tumor cells in Cluster 19 exhibited a highly active metabolic state, particularly in reactions involving copper, iron, and zinc ions.
Given its function in tumor progression (23), the high expression of LYZ in the chemotherapy-treated TME may facilitate the evolution of tumor cells, enabling them to rapidly adapt to the chemotherapy environment and subsequently develop the drug resistance. Although LYZ expression is associated with the late-stage grading of pancreatic cancer; however, it does not significantly correlate with the overall survival rates in the pancreatic cancer patients (Figure 2F,2G).
Totally, LYZ exhibited differential expression patterns within the PDAC tumor microenvironment. Further research is required to elucidate whether and how LYZ mediates tumor cell differentiation under chemotherapy conditions.
Chemotherapy altered the signal transmission and antigen presentation in TME
To elucidate the cellular crosstalk dynamics, we utilized CellChat (21) to identify the dominant signal transmitters. We observed that the number of interactions between the cells exhibited modest upregulation in the chemotherapy-treated group, while the intensity decreased slightly. Specifically, the interactions involving tumor cells and myeloid cells were limited in the naïve group/chemotherapy-untreated group, whereas the level of CAFs and other cells was slightly high (Figure 3A,3B), aligning with a previous report (11). Notably, the signaling output from the “Tumorcell.Fibro” cells decreased in the chemotherapy-treated group, compared to the naïve group, while the proliferative T cells received more signals (Figure 3B). Collectively, these results indicate that the interactions between different cells in the tumor tend to increase in response to chemotherapy.
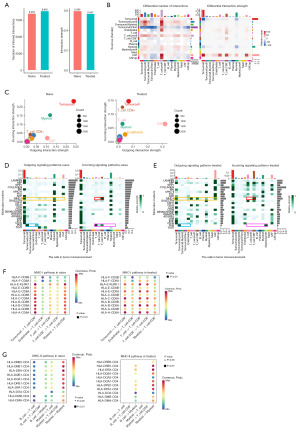
The incoming signal and outgoing modules revealed a distinct remodeling of intercellular communication in PDAC cells under chemotherapy. In the naïve group, interactions in the PDAC TME were mainly driven by tumor cells and myeloid cells (24), consistent with the established patterns. Notably, the interaction strengthened in the total T cells, CD8+ T cells, myeloid cells, endothelial cells, especially with CAFs enhanced after the chemotherapy (Figure 3C). The shift highlights significant remodeling of the PDAC TME following chemotherapy.
To investigate the signal transmission patterns in the PDAC TME, we identified the top 20 signal patterns in the naïve group as the benchmark and analyzed their alterations under treatment (Figure 3D,3E). Among these, we focused on the major histocompatibility complex (MHC)-I and MHC-II signaling due to their critical roles in the immune responses. The MHC-I signaling was predominantly emitted by tumor cells and myeloid cells. However, chemotherapy redirected the MHC-I signal to the T cells, especially the CD8+T cells and endothelial cells, with a slightly increase in the CAFs of the chemotherapy-treated group (Figure 3D,3E, yellow frame). The CD8+T cells remained the exclusive signal recipients regardless of treatment (Figure 3D,3E, red frame). Further, the HLA-A/B/C/E-CD8A LR pairs were the primary mediators of the MHC-I signal. Notably, while the overall MHC-I signaling increased, the interaction strength of the HLA-A/B/C/E-CD8B LR pair in the chemotherapy-treated group was significantly reduced (Figure 3F). In contrast, the MHC-II signal was primarily mediated by myeloid cells, both in the chemotherapy-treated and untreated (naïve) groups, with minimal involvement of B cells observed only after chemotherapy (Figure 3D,3E,3G).
Previous research has demonstrated that SPP1 and CXCL also undergo significant rearrangements (11). For instance, the cellular receptors of the SPP1 signal were originally emitted by the myeloid cells after chemotherapy. However, the signal received by CAFs and non-proliferative T cells remained relatively weak. In contrast, the CXCL signal, which was undetected in the naïve group, ranked higher in the chemotherapy-treated group with CAFs as the emitter. The cerebrospinal fluid (CSF) signal, which was originally emitted by CAFs and mast cells, and was mainly received by myeloid cells, changed, such that the mast cells became the signal emitter. Further investigation into these dynamic changes is warranted to elucidate their underlying mechanisms and implications.
Chemotherapy remodeled immune checkpoint signaling
Prior studies have established the dominant ligand-receptor axis mediating tumor-T cell communication, with reported downregulation following chemotherapy (11). Our single-cell analysis identified that the proliferating tumor cells (“Tumor.Prolif” cells) were the strongest senders of the polio virus receptor (PVR) signal in both the naïve and chemotherapy-treated groups. The PVR signal receiver in the naïve group included all states of T cells, but the PVR signal was downregulated or even lost in the chemotherapy-treated group (Figure 3D,3E, blue frame). The PVR signaling axis underwent functional compartmentalization, shifting from T cell targeting in naïve tumors to preferential engagement with proliferating myeloid populations following chemotherapy (Figure 3D,3E, magenta frame). Meanwhile, the TIGIT signal, which originally came from the T cells and its receivers, was completely lost after chemotherapy treatment. Further, in the naïve group, the primary contributor of the PVR signal was PVR-TIGIT, but later switched to PVR-CD226 (Figure 4A). PVR, TIGIT, and CD226 were expressed in both the naïve and chemotherapy-treated groups (Figure 4B).
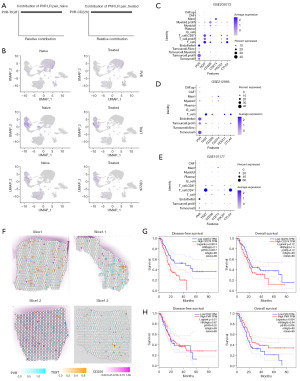
Our investigation extended to another typical checkpoint molecule, CD274, revealing unexpected compartmentalization of its biological activity. Single-cell profiling demonstrated that mast cells maintained significant programmed death-ligand 1 (PD-L1) signaling potency despite low baseline CD274 expression (Figure 4C). This observation was further validated across the GSE212966 and GSE197177 datasets (Figures 4D,4E). High CD274 expression in mast cells was found to inhibit the activity of effector CD8+ T cells in a skin cancer animal model (25), and was also found to be associated with an immunosuppressive microenvironment in stomach cancer (26). Cross-dataset analysis of three independent repositories revealed conserved patterns in PDAC. Tumor microenvironment exhibited PVR predominance over CD274 (PVR:CD274 ratio >2.8). The immune compartment showed preferential expression of inhibitory receptors (TIGIT, CTLA4) versus activation markers (CD226) (Figure 4C-4E).
To further investigate the expression of immune checkpoints in PDAC, we analyzed the spatial transcriptome data of PDAC samples from two independent available online GSE233254 (14), and GSE203612 (15) datasets. The analysis revealed that very few regions had adjacent spots of PVR-TIGIT and PVR-CD226 (Figure 4F). Similarly, high-abundance spots for PD-L1 (CD274) and programmed cell death protein 1 (PD-1; PDCD1) were relatively rare and clearly distant from each other. Notably, the PD-1 expression alteration due to the chemotherapy treatment was modest; however, according to the GEPIA database, CD274 was a more significant prognostic indicator of disease-free survival and overall survival in pancreatic cancer than PVR (Figure 4G,4H). Further research needs to be conducted to examine effects of CD274 on immune therapy in PDAC.
Notably, high CD226 expression was predominantly localized to T cell populations, particularly CD8+ T cells, rather than myeloid lineages (Figure 4C-4E), and the proportion of proliferating myeloid cells in the chemotherapy treatment group was limited. Prior study revealed that myeloid cells form physical barriers in the TME, isolating T cells from the tumor core and limiting their cytotoxic effects on tumor cells (27); however, the biological function of CD226 and its clinical relevance require further study.
Discussion
To date, no significant immunotherapeutic breakthroughs have been achieved in PDAC, primarily due to its highly complex immunosuppressive tumor microenvironment. In the present study, we observed the cross-cell type expression of markers typically associated with specific cell types. Among these, the cross-expression of LYZ emerged as a notable candidate between myeloid cells and tumor cells. LYZ is often used to label myeloid cells, and functional significance has previously been established in prior study (13). LYZ encodes lysozyme, which is best known for its anti-infection function. Previous studies have demonstrated that LYZ is highly expressed in Hepatocellular carcinoma, and can serve as a potential marker of tumor progression (23). LYZ has been identified as an adverse prognostic marker in breast cancer (28) and cervical cancer (29). Moreover, the protein-protein interaction network comprising CCL2, LYZ, MMP2, LOX, and GZMB appears to be closely associated to lung cancer brain metastasis (30). To the best of our knowledge, this study represents the investigation on the unique comprehensive expression pattern of LYZ in the PDAC TME. We found that LYZ was highly expressed in PDAC tumor cells under chemotherapy conditions, and accompanied by robust dysregulation of metal ion homeostasis and secretion functions (Figure 2). These findings suggest that LYZ may play a role in the adaptive response of PDAC cells to chemotherapy, potentially influencing cellular metabolism and signaling pathways. Further research is required to elucidate the underlying mechanisms and to determine the prognostic significance of LYZ upregulation in the context of PDAC treatment.
Antigen effective presentation is a fundamental process in the initiation of adaptive immune response, which is related to various cells, including macrophages, dendritic cells, and B cells that present antigens to T cells through MHC molecules. Both the density and spatial distribution of native myeloid cells in PDAC tissues exhibit high heterogeneity, and the location and density of immunosuppressive M2 polarization have a significant effect on patient prognosis (27). The key role of myeloid cells is to phagocytose and present antigens to CD4+ or CD8+ T cells via MHC molecules. CD4 often indicates a specific set of innate immune cells (30,31). The expression of CD4 in myeloid cells has been described previously, and appears to be related to viral infection (31,32). The expression of CD4 in blood monocytes initiates their differentiation into macrophages and makes them more susceptible to human immunodeficiency virus infection (33). A recent report found that CD4 expression in monocytes decreases their migratory capability and inhibits T cell activity (34). In our analysis, we observed substantial expression of CD4 in myeloid cells within PDAC tissues. However, the antigen presentation signaling pathways in these cells did not appear to be significantly affected. This suggests that while CD4 may be expressed in myeloid cells, its impact on antigen presentation in the context of PDAC may be limited or context-dependent. Further investigation is required to elucidate the functional significance of CD4 expression in myeloid cells within the PDAC tumor microenvironment.
MHC-I is primarily responsible for presenting endogenous antigens (35), and the upregulation of MHC-I in tumor cells may aid in the immunotherapy of PDAC (36). However, we found that the signal strength of MHC-I emitted by tumor cells was relatively lower after chemotherapy. Conversely, in the chemotherapy-treated group, the MHC-I emission was upregulated in CAFs, myeloid cells, and T cells (Figure 3D,3E, yellow frame). This suggests that chemotherapy may downregulate the immunogenicity of tumor cells and facilitate the self-antigen presentation of CAFs, myeloid cells, and T cells. This observation leads us to hypothesize that the tumor’s antigen presentation strategy in the TME may be suppressed.
The mechanistic basis remains to be fully elucidated on how endogenous antigens in the TME interfere with immune responses and whether the process of apoptosis after chemotherapy masks or suppresses the immunity. Based on our analysis, chemotherapy has limited effects on the MHC-II antigen presentation pathway. However, the selective expression of CD4 in myeloid cells appears to redirect the MHC-II antigen presentation pathway to function more as a self-secretory or paracrine signal in myeloid cells rather than T cells (Figure 3F,3G).
The immune checkpoint blockade is one of the main targets of immunotherapy, and its typical targets include PD-1/PD-L1 and CTLA4. Due to its demonstrated clinical efficacy in biomarker-selected cohorts, it has become the standard treatment for various tumors. However, this represents an insufficient foundation for mechanistic interpretation for the immunotherapy of PDAC. Researchers are striving to find more targets to meet clinical needs (37,38). PVR (also known as CD155) has been identified as a common ligand for CD226 and TIGIT (39). Besides PVR, CD112 has been demonstrated to competitively bind to CD226 and TIGIT, but our transcriptomic profiling failed to detect significant CD112 expression across the examined cellular populations. CD226 is an immunoglobulin superfamily member on the cell membrane surface, and is expressed in various immune cells, but its precise functional mechanisms remain incompletely characterized (40). With the discovery of additional factors related to CD226, such as PD-1, CD96, and PVGIG, there is increasing evidence that CD226 is another key player in immunotherapy, alongside established targets like PD-1/PD-L1 and CTLA4 (41).
In PDAC, comparative transcriptomic analysis across three independent cohorts revealed that PVR demonstrated consistently elevated expression levels relative to CD274 (PD-L1) (Figure 4C-4E). Additionally, the dynamic changes in TIGIT signaling following chemotherapy suggest that these signals or the cells emitting them may be particularly sensitive to chemotherapy treatment. The stronger and more stable PVR expression suggests that PVR might be a more reasonable target for PDAC. Notably, biochemical characterization of PVR-mediated interactions revealed limited binding affinities with TIGIT/CD226 receptors.
The stromal complexity of PDAC is further amplified by CAFs, of which its cellular ontogeny remains controversial (42), and Single-cell transcriptomic studies have identified molecularly characterized subtypes, such as myogenic (CAFmye), inflammatory (CAFinfla), and the relatively rare antigen-presenting (CAFap), adipogenic (CAFadi), and endothelial-mesenchymal (CAFEndMT). There seems to be a possibility of mutual transformations among these phenotypes (43). However, systematic interrogation of clinical specimens failed to detect CAFap transcriptional signatures in the analyzed sample cohort (11).
Through comprehensive single-cell transcriptomic profiling, we identified a special sub-population, Cluster 29 (Figure 1B) that was not recognized by conventional cell markers but exhibited limited expression of the CAF marker COL1A1. Transcriptomic characterization revealed that the top five genes expressed in this cluster (CRYAB, CDH19, S100B, GPM68, and NRXN1) are all included in the key signature of antigen-presenting CAFs (44). HLA-DRA and CD74 have been previously characterized as specific markers for CAFap (45). These two genes displayed ubiquitous expression patterns across multiple cell populations without apparent specificity in our dataset (Figure 1B). Based on these molecular features, we classified this cluster as a potential antigen-presenting phenotype of CAFs, which we designated as “CAF.ap.” Notably, a similar gene signature (S100B, CDH19, GPM6B, ITGB8, and CRYAB) was used to identify Schwann cells in the PDAC TME (12). Interestingly, this cluster also exhibited markers commonly associated with Schwann cells, such as MPZ and NCAM1 (46). This observation aligns with recent experimental evidence demonstrating CAF-to-Schwann cell transdifferentiation in vivo (47).
In conclusion, the high phenotypic plasticity of components in the TME adds complexity to their roles in PDAC progression and prognosis, highlighting the need for new technologies and theoretical frameworks for accurate classification. There are a number of limitations in this study. First, while we validated our main findings with independent datasets, the strong heterogeneity of PDAC suggests that some of our conclusions may require further validation through larger-scale studies. Second, the extent to which chemotherapy affects cells in the TME, as well as how these cellular states influence the accuracy of sequencing analyses, remains unclear. Nevertheless, our analysis sheds light on this challenging cancer treatment.
Conclusions
Through systematic analysis of scRNA-seq data, we investigated the chemotherapy-induced alterations in antigen presentation machinery and the significant remodeling of the tumor microenvironment. Further, the present results revealed that LYZ expression significantly increased in tumor cells and myeloid cells in response to chemotherapy. Moreover, chemotherapy induced a fundamental reorganization of PVR signaling, characterized by a cellular redistribution from TIGIT-dominant signaling in T cells to CD226-predominant signaling in myeloid cells. This treatment-mediated shift disrupted the immunosuppressive equilibrium of the TME while simultaneously enhancing tumor antigen presentation capacity and modifying PVR signaling networks. These findings suggest that strategic integration of chemotherapy with immune therapies could be a path to enhance treatment responsiveness in PDAC.
Acknowledgments
None.
Footnote
Reporting Checklist: The authors have completed the MDAR reporting checklist. Available at https://tcr.amegroups.com/article/view/10.21037/tcr-2025-103/rc
Peer Review File: Available at https://tcr.amegroups.com/article/view/10.21037/tcr-2025-103/prf
Funding: None.
Conflicts of Interest: All authors have completed the ICMJE uniform disclosure form (available at https://tcr.amegroups.com/article/view/10.21037/tcr-2025-103/coif). Y.L. and Y.Z. are from MyGene Diagnostics Co., Ltd. The other authors have no conflicts of interest to declare.
Ethical Statement: The authors are accountable for all aspects of the work in ensuring that questions related to the accuracy or integrity of any part of the work are appropriately investigated and resolved. The study was conducted in accordance with the Declaration of Helsinki (as revised in 2013).
Open Access Statement: This is an Open Access article distributed in accordance with the Creative Commons Attribution-NonCommercial-NoDerivs 4.0 International License (CC BY-NC-ND 4.0), which permits the non-commercial replication and distribution of the article with the strict proviso that no changes or edits are made and the original work is properly cited (including links to both the formal publication through the relevant DOI and the license). See: https://creativecommons.org/licenses/by-nc-nd/4.0/.
References
- Siegel RL, Miller KD, Wagle NS, et al. Cancer statistics, 2023. CA Cancer J Clin 2023;73:17-48. [PubMed]
- Jiang Y, Sohal DPS. Pancreatic Adenocarcinoma Management. JCO Oncol Pract 2023;19:19-32. [PubMed]
- Feng M, Xiong G, Cao Z, et al. PD-1/PD-L1 and immunotherapy for pancreatic cancer. Cancer Lett 2017;407:57-65. [Crossref] [PubMed]
- Bockorny B, Grossman JE, Hidalgo M. Facts and Hopes in Immunotherapy of Pancreatic Cancer. Clin Cancer Res 2022;28:4606-17. [Crossref] [PubMed]
- Du X, Lu X, Cao X. Gantt chart for updated OS and PFS after cancer targeted therapy. The Innovation Medicine 2023;1:100008. [Crossref]
- Xie N, Shen G, Gao W, et al. Neoantigens: promising targets for cancer therapy. Signal Transduct Target Ther 2023;8:9. [Crossref] [PubMed]
- Chen S, Li J, Dong A, et al. Nab-paclitaxel and gemcitabine plus camrelizumab and radiotherapy versus nab-paclitaxel and gemcitabine alone for locally advanced pancreatic adenocarcinoma: a prospective cohort study. J Hematol Oncol 2023;16:26. [Crossref] [PubMed]
- Wu X, Liang Y. Screening and Prognostic Analysis of Immune-Related Genes in Pancreatic Cancer. Front Genet 2021;12:721419. [Crossref] [PubMed]
- Peng J, Madduri S, Clontz AD, et al. Clinical trial-identified inflammatory biomarkers in breast and pancreatic cancers. Front Endocrinol (Lausanne) 2023;14:1106520. [Crossref] [PubMed]
- Tang R, Xu J, Wang W, et al. Targeting neoadjuvant chemotherapy-induced metabolic reprogramming in pancreatic cancer promotes anti-tumor immunity and chemo-response. Cell Rep Med 2023;4:101234. [Crossref] [PubMed]
- Werba G, Weissinger D, Kawaler EA, et al. Single-cell RNA sequencing reveals the effects of chemotherapy on human pancreatic adenocarcinoma and its tumor microenvironment. Nat Commun 2023;14:797. [Crossref] [PubMed]
- Chen K, Wang Q, Liu X, et al. Immune profiling and prognostic model of pancreatic cancer using quantitative pathology and single-cell RNA sequencing. J Transl Med 2023;21:210. [Crossref] [PubMed]
- Zhang S, Fang W, Zhou S, et al. Single cell transcriptomic analyses implicate an immunosuppressive tumor microenvironment in pancreatic cancer liver metastasis. Nat Commun 2023;14:5123. [PubMed]
- Sans M, Makino Y, Min J, et al. Spatial Transcriptomics of Intraductal Papillary Mucinous Neoplasms of the Pancreas Identifies NKX6-2 as a Driver of Gastric Differentiation and Indolent Biological Potential. Cancer Discov 2023;13:1844-61. [PubMed]
- Barkley D, Moncada R, Pour M, et al. Cancer cell states recur across tumor types and form specific interactions with the tumor microenvironment. Nat Genet 2022;54:1192-201. [PubMed]
- Hao Y, Hao S, Andersen-Nissen E, et al. Integrated analysis of multimodal single-cell data. Cell 2021;184:3573-3587.e29. [PubMed]
- Korsunsky I, Millard N, Fan J, et al. Fast, sensitive and accurate integration of single-cell data with Harmony. Nat Methods 2019;16:1289-96. [PubMed]
- McGinnis CS, Murrow LM, Gartner ZJ. DoubletFinder: Doublet Detection in Single-Cell RNA Sequencing Data Using Artificial Nearest Neighbors. Cell Syst 2019;8:329-337.e4. [PubMed]
- Wu T, Hu E, Xu S, et al. clusterProfiler 4.0: A universal enrichment tool for interpreting omics data. Innovation (Camb) 2021;2:100141. [PubMed]
- Hänzelmann S, Castelo R, Guinney J. GSVA: gene set variation analysis for microarray and RNA-seq data. BMC Bioinformatics 2013;14:7. [PubMed]
- Jin S, Guerrero-Juarez CF, Zhang L, et al. Inference and analysis of cell-cell communication using CellChat. Nat Commun 2021;12:1088. [PubMed]
- Ferraboschi P, Ciceri S, Grisenti P. Applications of Lysozyme, an Innate Immune Defense Factor, as an Alternative Antibiotic. Antibiotics (Basel) 2021;10:1534. [PubMed]
- Gu Z, Wang L, Dong Q, et al. Aberrant LYZ expression in tumor cells serves as the potential biomarker and target for HCC and promotes tumor progression via csGRP78. Proc Natl Acad Sci U S A 2023;120:e2215744120. [PubMed]
- Jin MZ, Jin WL. The updated landscape of tumor microenvironment and drug repurposing. Signal Transduct Target Ther 2020;5:166. [PubMed]
- Hirano T, Honda T, Kanameishi S, et al. PD-L1 on mast cells suppresses effector CD8(+) T-cell activation in the skin in murine contact hypersensitivity. J Allergy Clin Immunol 2021;148:563-573.e7. [Crossref] [PubMed]
- Lv Y, Zhao Y, Wang X, et al. Increased intratumoral mast cells foster immune suppression and gastric cancer progression through TNF-α-PD-L1 pathway. J Immunother Cancer 2019;7:54. Erratum in: J Immunother Cancer 2020;8:e0530-3corr1. [Crossref] [PubMed]
- Väyrynen SA, Zhang J, Yuan C, et al. Composition, Spatial Characteristics, and Prognostic Significance of Myeloid Cell Infiltration in Pancreatic Cancer. Clin Cancer Res 2021;27:1069-81. [Crossref] [PubMed]
- Serra C, Vizoso F, Alonso L, et al. Expression and prognostic significance of lysozyme in male breast cancer. Breast Cancer Res 2002;4:R16. [Crossref] [PubMed]
- Zhong PQ, Yan XX, Wang WJ, et al. Identification and Validation of LYZ and CCL19 as Prognostic Genes in the Cervical Cancer Micro-Environment. Clin Exp Obstet Gynecol 2022;49:144. [Crossref]
- Su H, Lin Z, Peng W, et al. Identification of potential biomarkers of lung adenocarcinoma brain metastases via microarray analysis of cDNA expression profiles. Oncol Lett 2019;17:2228-36. [PubMed]
- Gibbings D, Befus AD. CD4 and CD8: an inside-out coreceptor model for innate immune cells. J Leukoc Biol 2009;86:251-9. [Crossref] [PubMed]
- Kazazi F, Mathijs JM, Foley P, et al. Variations in CD4 expression by human monocytes and macrophages and their relationships to infection with the human immunodeficiency virus. J Gen Virol 1989;70:2661-72. [Crossref] [PubMed]
- Zhen A, Krutzik SR, Levin BR, et al. CD4 ligation on human blood monocytes triggers macrophage differentiation and enhances HIV infection. J Virol 2014;88:9934-46. [Crossref] [PubMed]
- Laopajon W, Cheyasawan P, Pata S, et al. The ligation of CD4 molecules, expressed on monocytes by an anti-CD4 monoclonal antibody, inhibits T cell activation and monocyte mobility. Asian Pac J Allergy Immunol 2023; Epub ahead of print. [Crossref] [PubMed]
- Pishesha N, Harmand TJ, Ploegh HL. A guide to antigen processing and presentation. Nat Rev Immunol 2022;22:751-64. [Crossref] [PubMed]
- Yamamoto K, Venida A, Yano J, et al. Autophagy promotes immune evasion of pancreatic cancer by degrading MHC-I. Nature 2020;581:100-5. [Crossref] [PubMed]
- Waldman AD, Fritz JM, Lenardo MJ. A guide to cancer immunotherapy: from T cell basic science to clinical practice. Nat Rev Immunol 2020;20:651-68. [Crossref] [PubMed]
- Bagchi S, Yuan R, Engleman EG. Immune Checkpoint Inhibitors for the Treatment of Cancer: Clinical Impact and Mechanisms of Response and Resistance. Annu Rev Pathol 2021;16:223-49. [Crossref] [PubMed]
- Wu B, Zhong C, Lang Q, et al. Poliovirus receptor (PVR)-like protein cosignaling network: new opportunities for cancer immunotherapy. J Exp Clin Cancer Res 2021;40:267. [Crossref] [PubMed]
- Huang Z, Qi G, Miller JS, et al. CD226: An Emerging Role in Immunologic Diseases. Front Cell Dev Biol 2020;8:564. [Crossref] [PubMed]
- Conner M, Hance KW, Yadavilli S, et al. Emergence of the CD226 Axis in Cancer Immunotherapy. Front Immunol 2022;13:914406. [Crossref] [PubMed]
- Sahai E, Astsaturov I, Cukierman E, et al. A framework for advancing our understanding of cancer-associated fibroblasts. Nat Rev Cancer 2020;20:174-86. [Crossref] [PubMed]
- Luo H, Xia X, Huang LB, et al. Pan-cancer single-cell analysis reveals the heterogeneity and plasticity of cancer-associated fibroblasts in the tumor microenvironment. Nat Commun 2022;13:6619. [Crossref] [PubMed]
- Cui Zhou D, Jayasinghe RG, Chen S, et al. Spatially restricted drivers and transitional cell populations cooperate with the microenvironment in untreated and chemo-resistant pancreatic cancer. Nat Genet 2022;54:1390-405. [Crossref] [PubMed]
- Elyada E, Bolisetty M, Laise P, et al. Cross-Species Single-Cell Analysis of Pancreatic Ductal Adenocarcinoma Reveals Antigen-Presenting Cancer-Associated Fibroblasts. Cancer Discov 2019;9:1102-23. [Crossref] [PubMed]
- Liu Z, Jin YQ, Chen L, et al. Specific marker expression and cell state of Schwann cells during culture in vitro. PLoS One 2015;10:e0123278. [Crossref] [PubMed]
- Sowa Y, Kishida T, Tomita K, et al. Direct Conversion of Human Fibroblasts into Schwann Cells that Facilitate Regeneration of Injured Peripheral Nerve In Vivo. Stem Cells Transl Med 2017;6:1207-16. [Crossref] [PubMed]
(English Language Editor: L. Huleatt)