Prediction of mortality in patients with retinoblastoma based on random survival forest: a retrospective cohort analysis using SEER database
Highlight box
Key findings
• We have developed a random survival forest (RSF) model with strong predictive performance, which was constructed and optimized by analyzing the retinoblastoma patient’s clinical characteristics, treatment methods, and survival outcomes in the Surveillance, Epidemiology, and End Results (SEER) database.
What is known and what is new?
• Retinoblastoma (RB) is the most prevalent malignant intraocular tumor in children, there is no validated prediction tool for long-term survival of RB patients. The applicability of the RSF model for predicting prognosis in patients with RB is unclear.
• We innovatively constructed a random forest model based on patient data from the SEER database to predict the risk of mortality in RB patients, providing a basis for the clinical evaluation of high-risk RB patients.
What is the implication, and what should change now?
• The RSF model was constructed and optimized by analyzing the patient’s clinical characteristics, treatment methods, and survival outcomes, with the aim of achieving highly effective and accurate mortality prediction. This would help clinical physicians identify high-risk RB patients early, optimize treatment decisions, and improve patients’ survival rates and quality of life.
Introduction
Background
Retinoblastoma (RB) is the most prevalent malignant intraocular tumor in children, with an incidence rate of approximately 1/17,000. It is caused by mutations in the retinoblastoma gene (RB1) located on chromosome 13q14.2 (1,2). The survival rate for RB is about 90% in developed countries, 70% in middle-income countries, and 40% in low-income countries. Mortality from the disease remains high in developing countries (3). The findings of Abdelazeem et al. show a significant decrease in the trend of 5- and 10-year relative survival in patients with RB from 2000 to 2018 (4). In addition, due to the relative rarity of the disease and the small sample size of previous studies, there is currently no analysis of prognosis and risk factors based on large sample data of the population. According to Yin et al., age, sex, stage, radiotherapy, income, and diagnostic certainty are independent prognostic factors for overall survival in adult RB patients (5), but it remains unclear whether these findings are applicable to children. Moreover, there is no validated prediction tool for the long-term survival of RB patients.
Rationale and knowledge gap
Survival analyses in previous medical studies are mostly conducted by using traditional proportional hazard models such as COX regression combined with nomogram plots (6-10). The advantage of the COX regression model is that it can handle censored data and control the interference of confounding factors (11). However, the included variables need to comply with the proportional hazard assumption, and there is no way to capture non-linear relationship data for analysis (12). Random survival forest (RSF) is a nonparametric statistical method based on ensemble learning. It is capable of handling data with high dimensionality and complex features and is widely used in the field of survival analysis (13). RSF is highly accurate and robust in predicting a patient’s survival time and mortality risk by constructing a large decision tree to capture the complex relationships between variables (14).
Objective
The aim of this study was to leverage the RSF model to predict the mortality of patients with RB based on patient data from the Surveillance, Epidemiology, and End Results (SEER) database. The RSF model was constructed and optimized by analyzing the patient’s clinical characteristics, treatment methods, and survival outcomes, with the aim of achieving highly effective and accurate mortality prediction. This would help clinical physicians identify high-risk RB patients early, optimize treatment decisions, and improve patients’ survival rates and quality of life. The study results would provide important scientific evidence for personalized treatment of RB and promote the development of precision medicine. We present this article in accordance with the TRIPOD + AI reporting checklist (available at https://tcr.amegroups.com/article/view/10.21037/tcr-24-1760/rc).
Methods
Data collection and pre-processing
Data collection
A total of 1,470 patients diagnosed with RB between 2000 and 2019 were extracted from the Surveillance, Epidemiology, and End Results (SEER) database (https://seer.cancer.gov). The SEER database is the largest cancer patient database in the U.S., covering approximately 48% of U.S. cancer patients and collecting clinical data from approximately 22 U.S. administrative regions (15). The study was conducted in accordance with the Declaration of Helsinki (as revised in 2013).
The total number of patients was 1,470. Patients with zero follow-up time (n=18), unknown diagnostic methods (n=21), and missing data (n=854) were excluded. Variables used for further analysis obtained from the SEER database were as follows: demographic information (sex, age, race, residence, household income), clinical characteristics (year of diagnosis, tumor stage, tumor size, tumor laterality, and whether or not the tumor was metastatic), treatment modalities (surgery, radiation, chemotherapy), timing of surgery (systemic therapy surgery sequence), timing of treatment, and survival data (such as survival time, follow-up time). The primary outcome was mortality due to RB. Follow-up for the study population started at the time of RB diagnosis and ended at the time of patient death.
Data cleaning
Missing data and outliers were addressed to ensure data integrity and consistency.
Data segmentation
To avoid overfitting, the dataset was split into a training set and a validation set at a ratio of 7:3 using the createDataPartition function from the R package caret, with randomization to ensure the representativeness of model training and validation.
Statistical analysis
The entire study cohort was allocated to a training set and a validation set. Continuous variables with normal distribution were expressed as mean ± standard deviation, and independent t-tests were leveraged for group comparisons. For continuous variables not following a normal distribution, the median (interquartile range) was employed, and between-group comparisons were executed by means of the Mann-Whitney U test. For categorical variables, data were presented as sample size (percentage), and Pearson’s Chi-squared test was leveraged to compare groups.
Feature selection
Based on the framework of the RSF model, the included variables were ranked in importance. In the importance ranking, variables with an importance value greater than 0 were considered beneficial to the model, while those with an importance value less than 0 were considered to lose model performance. Therefore, variables with an importance value greater than 0 were selected as model variables.
Parameter selection
The optimal mtry and nodesize tuning parameters for the random forest model were found by calculating the out-of-sample error using the tune function. Based on the above selected parameters, learning curves were plotted. The optimal number of learning trees (ntree) was selected through the learning curve. Based on the above parameters, a random forest model was developed.
Validation
The C-index, receiver operating characteristic (ROC) curve, and area under the ROC curve (AUC) were employed to validate the model. The AUC was primarily leveraged to appraise binary classification models, and its value ranged from 0.5 (random prediction) to 1 (perfect prediction). Generally, an AUC greater than 0.7 indicated favorable discriminative power, greater than 0.8 indicated excellent performance, and greater than 0.9 signified outstanding performance (15,16). In survival analysis, the C-index is an extended metric analogous to AUC, reflecting the time-dependent consistency of model predictions. C-index =0.5 corresponded to random prediction, while C-index =1 signified perfect prediction. A C-index greater than 0.7 was commonly viewed as an acceptable level of model performance (15,16). All analyses were completed using R version 4.4.0, and statistical significance was defined as a two-tailed P value <0.05.
Results
Population distribution
A total of 577 patients were included in the study. The dataset was split into a training set (n=411) and a validation set (n=166) at a ratio of 7:3. The median follow-up time in the total study cohort was 57 months. A total of 17 patients died from RB. The total study cohort consisted of 52% females and 48% males. The age distribution was highest among children aged 1–5 years old. There were no significant differences in the baseline characteristics between the training and validation sets, as shown in Table 1.
Table 1
Characteristic | Overall (N=577) | Training set (N=411) | Validation set (N=166) | P value |
---|---|---|---|---|
Survival months | 57 [25, 101] | 56 [26, 102] | 60 [23, 100] | >0.90a |
Death | 17 [2.9] | 14 [3.4] | 3 [1.8] | 0.42b |
Sex | 0.21b | |||
Female | 298 [52] | 220 [54] | 78 [47] | |
Male | 279 [48] | 191 [46] | 88 [53] | |
Laterality | 0.4b | |||
Bilateral | 180 [31] | 124 [30] | 56 [34] | |
Only one side | 397 [69] | 287 [70] | 110 [66] | |
Stage | 0.35b | |||
Distant | 7 [1.2] | 6 [1.5] | 1 [0.6] | |
Localized | 492 [85] | 344 [84] | 148 [89] | |
Regional | 78 [14] | 61 [15] | 17 [10] | |
T stage | 0.21b | |||
T1 | 253 [44] | 172 [42] | 81 [49] | |
T2 | 201 [35] | 147 [36] | 54 [33] | |
T3 | 85 [15] | 60 [15] | 25 [15] | |
T4 | 28 [4.9] | 25 [6.1] | 3 [1.8] | |
TX | 10 [1.7] | 7 [1.7] | 3 [1.8] | |
M stage | >0.90b | |||
M0 | 570 [99] | 405 [99] | 165 [99] | |
M1 | 5 [0.9] | 4 [1.0] | 1 [0.6] | |
MX | 2 [0.3] | 2 [0.5] | 0 [0] | |
Surgery radiation sequence | 0.90b | |||
No | 566 [98] | 402 [98] | 164 [99] | |
Other | 1 [0.2] | 1 [0.2] | 0 [0] | |
Radiation after surgery | 7 [1.2] | 5 [1.2] | 2 [1.2] | |
Radiation prior to surgery | 3 [0.5] | 3 [0.7] | 0 [0] | |
Surgery | 495 [86] | 351 [85] | 144 [87] | 0.74b |
Radiation | 14 [2.4] | 12 [2.9] | 2 [1.2] | 0.42b |
Chemotherapy | 376 [65] | 275 [67] | 101 [61] | 0.23b |
Systemic therapy surgery sequence | 0.48b | |||
Intraoperative systemic therapy | 8 [1.4] | 6 [1.5] | 2 [1.2] | |
No | 282 [49] | 195 [47] | 87 [52] | |
Other | 73 [13] | 48 [12] | 25 [15] | |
Systemic therapy after surgery | 138 [24] | 106 [26] | 32 [19] | |
Systemic therapy before surgery | 76 [13] | 56 [14] | 20 [12] | |
Months from diagnosis to treatment | 0.81b | |||
≤1 month | 391 [68] | 277 [67] | 114 [69] | |
>1 month | 186 [32] | 134 [33] | 52 [31] | |
Size (cm) | 0.43b | |||
<1 | 83 [14] | 54 [13] | 29 [17] | |
1–2 | 392 [68] | 282 [69] | 110 [66] | |
>2 | 102 [18] | 75 [18] | 27 [16] | |
Primary sequence | 0.34b | |||
One primary only | 569 [99] | 405 [99] | 164 [99] | |
1st of 2 or more primaries | 7 [1.2] | 6 [1.5] | 1 [0.6] | |
Other | 1 [0.2] | 0 [0] | 1 [0.6] | |
Total number of malignant tumors | >0.90b | |||
≥2 | 8 [1.4] | 6 [1.5] | 2 [1.2] | |
1 | 569 [99] | 405 [99] | 164 [99] | |
Age (years) | 0.45b | |||
<1 | 260 [45] | 187 [45] | 73 [44] | |
1–5 | 304 [53] | 212 [52] | 92 [55] | |
6–10 | 11 [1.9] | 10 [2.4] | 1 [0.6] | |
>10 | 2 [0.3] | 2 [0.5] | 0 [0] | |
Race | 0.41b | |||
Asian | 63 [11] | 44 [11] | 19 [11] | |
Black | 85 [15] | 67 [16] | 18 [11] | |
White | 422 [73] | 295 [72] | 127 [77] | |
Other | 7 [1.2] | 5 [1.2] | 2 [1.2] | |
Median household income | 0.20b | |||
<$50,000 | 99 [17] | 78 [19] | 21 [13] | |
$50,000–$75,000 | 333 [58] | 235 [57] | 98 [59] | |
$75,000+ | 145 [25] | 98 [24] | 47 [28] | |
Residence | 0.47b | |||
Metropolitan | 510 [88] | 366 [89] | 144 [87] | |
Nonmetropolitan | 67 [12] | 45 [11] | 22 [13] |
Data are presented as median [IQR] or n [%]. a, Wilcoxon rank sum test. b, Fisher’s exact test; Pearson’s Chi-squared test. IQR, interquartile range.
Feature selection
A total of 13 variables were beneficial to the model, including stage, T stage, M stage, surgery radiation sequence, radiation, systemic therapy surgery sequence, age, chemotherapy, surgery, race, residence, sex, and primary sequence, as shown in Figure 1.
Parameter selection and modeling
The out-of-sample error was used to find the optimal mtry and nodesize tuning parameters (mtry =10, nodesize =25) for the random forest model, as shown in Figure 2. The optimal ntree (ntree =160) was subsequently selected through the learning curve (Figure 3). Based on the above selected parameters, a random survival model was developed.
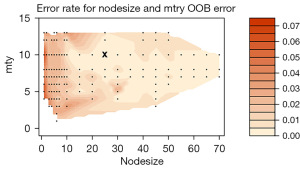
Model validation
The C-index value of the training cohort was 0.9803 and the C-index value of the validation cohort was 0.9122. The AUCs of the model for predicting mortality at 3, 5, and 10 years were 0.983, 0.986, and 0.996 in the training set, and 0.892, 0.910, and 0.904 in the validation cohort. The calibration curve results showed that the model did not significantly underestimate or overestimate the mortality risk of RB patients (Figure 4).
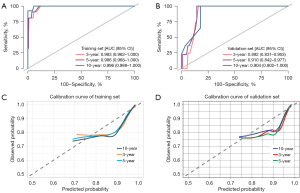
Partial univariate survival analysis
The variables, stage, T, and M, which were beneficial to the model, were chosen to draw their survival analysis images, respectively (Figures 5-7). The results indicated that the survival rates decreased in the following order within the subcategories of stage: localized, regional, and distant. The survival rates in the subcategories of T stage were arranged in descending order as follows: T1, T2, TX, T3, and T4. In addition, the survival rates in the subcategories of M stage were ranked in descending order as MX, M0, and M1.
Discussion
Key findings
RB is a rare and invasive childhood retinal tumor. Moreover, it is the most frequent primary intraocular tumor in both childhood and infancy, with 8,000 new cases of retinal malignancy diagnosed worldwide every year (16,17). Due to the low incidence of the disease, the fact that early diagnosis improves patient prognosis (16,17), and the risk of direct tissue biopsy triggering tumor dissemination in patients with RB, there is still no reliable method to determine a valid predictive tool for long-term survival in patients with RB. In this study, an RSF model for patients with RB is developed based on population data from the SEER database. 13 variables in the importance ranking such as stage, T stage, M stage, and race, are beneficial to the model. The performance of the model reaches an AUC of more than 0.9. The model has a good predictive performance that helps in the clinical assessment of high-risk RB patients.
The SEER database project was initiated on January 1, 1973 by President Richard Nixon (15). It is funded by the National Cancer Institute to reduce the burden of cancer in the U.S. by making cancer data available to the public for clinical study (18). As one of the premier cancer registries in the U.S., the SEER database has achieved high quality clinical data with a continuous quality control and improvement program. It’s a great source of real-world data (15). Using appropriate analytical methods and tools, real-world studies based on the SEER database can generate valuable real-world evidence (19). Using the SEER database for retrospective cohort analysis allows access to large-scale and diverse patient data, providing a solid foundation for the construction and validation of machine learning models. Previous studies on RB patients in the SEER database have predominantly utilized COX regression analysis to explore the relevant prognostic factors affecting the survival rate of RB patients (5,12,20). The model is unable to effectively analyze nonlinear relationship data and variables that do not meet the proportional hazard assumption. The RSF algorithm, first proposed in 2008, has now become an intuitive tool for predicting disease prognosis (21). The RSF model used in this study is capable of handling data with high dimensionality and complex features. Moreover, it is highly accurate and robust in predicting patients’ survival time and mortality risk (14).
Explanations of findings
It has been shown that about 60% of patients have unilateral RB and about 40% have bilateral RB. Unilateral cases are mostly non-hereditary and the median age of onset is 2 years old. Bilateral cases are predominantly heritable and the median age of onset is 1 year old (17). The incidence of RB does not differ significantly by sex, race, or region, but its survival rate varies significantly across countries and regions (22). The total study cohort consists of 52% females and 48% males, and the age distribution is highest among children aged 1–5 years old. Approximately 69% of tumors are unilateral, while about 31% are bilateral. The population distribution characteristics are broadly consistent with previous studies. Since the SEER database covers information on cancer patients in some regions of the U.S., most of RB patients in this study are white. Huang et al. have analyzed multiple previous studies and have found that age, sex, and treatment methods may be associated with the quality of life of RB patients (22). In contrast, Guo et al. have noted that tumor size, laterality, year of diagnosis, and place of residence are independent factors affecting the prognosis of children with RB (12). The results of the study show that distant tumor metastasis, T stage and M stage in RB patients all have an impact on patient survival. The survival rate of patients within situ cancer is higher than that of patients with regional metastasis. Furthermore, the survival rate of regional metastasis patients is higher than that of distant metastasis patients. The survival rates in T stage from high to low are ranked as T1, T2, TX, T3, and T4. Moreover, the survival rates in M stage from high to low are ranked as MX, M0, and M1.
The treatment of patients with RB has changed dramatically over the past decade. Intravitreal chemotherapy injection and ophthalmic artery chemotherapy surgery have changed the prognosis of patients compared to those who underwent previous systemic chemotherapy or external radiation therapy (23). Current treatment modalities for patients with RB include surgery, intravenous chemotherapy, intravitreal chemotherapy, radiation therapy, and consolidation therapy (cryotherapy and transpupillary thermotherapy). Due to the rarity of RB, it is difficult to conduct prospective randomized controlled trials to compare the different outcomes of each treatment modality (2,24). Among the 577 patients included in this study, a total of 17 patients died from RB, with a survival rate of over 95%. This survival rate is consistent with the survival rate of RB patients in developed countries in previous studies (1,4). At present, there is still significant heterogeneity in the treatment outcomes of RB in the Asian region (25). Metastatic RB is the leading cause of RB-related deaths in developing countries (26). The widespread application of future biomaterials and nanotechnology in clinical practice may further improve the prognosis of RB patients (27,28).
Limitations
However, there are still some limitations in this study. Since the SEER database itself has limitations on the types of variables, some of the variables we were interested in (such as family history, genetic history, and poor maternal habits during pregnancy) are not included in the study. In addition, the population included in the SEER database is mainly white people. Although we have validated the model in local populations, the generalization of the model to regions such as Africa or Southeast Asia may be somewhat influenced.
Conclusions
We have developed an RSF model with strong predictive performance based on a few simple variables. This may help evaluate the prognosis of high-risk RB patients. However, the performance of this model needs to be validated by larger population-based studies.
Acknowledgments
None.
Footnote
Reporting Checklist: The authors have completed the TRIPOD + AI reporting checklist. Available at https://tcr.amegroups.com/article/view/10.21037/tcr-24-1760/rc
Peer Review File: Available at https://tcr.amegroups.com/article/view/10.21037/tcr-24-1760/prf
Funding: None.
Conflicts of Interest: All authors have completed the ICMJE uniform disclosure form (available at https://tcr.amegroups.com/article/view/10.21037/tcr-24-1760/coif). The authors have no conflicts of interest to declare.
Ethical Statement: The authors are accountable for all aspects of the work in ensuring that questions related to the accuracy or integrity of any part of the work are appropriately investigated and resolved. The study was conducted in accordance with the Declaration of Helsinki (as revised in 2013).
Open Access Statement: This is an Open Access article distributed in accordance with the Creative Commons Attribution-NonCommercial-NoDerivs 4.0 International License (CC BY-NC-ND 4.0), which permits the non-commercial replication and distribution of the article with the strict proviso that no changes or edits are made and the original work is properly cited (including links to both the formal publication through the relevant DOI and the license). See: https://creativecommons.org/licenses/by-nc-nd/4.0/.
References
- Cruz-Gálvez CC, Ordaz-Favila JC, Villar-Calvo VM, et al. Retinoblastoma: Review and new insights. Front Oncol 2022;12:963780. [Crossref] [PubMed]
- Silvera VM, Guerin JB, Brinjikji W, et al. Retinoblastoma: What the Neuroradiologist Needs to Know. AJNR Am J Neuroradiol 2021;42:618-26. [Crossref] [PubMed]
- Byroju VV, Nadukkandy AS, Cordani M, et al. Retinoblastoma: present scenario and future challenges. Cell Commun Signal 2023;21:226. [Crossref] [PubMed]
- Abdelazeem B, Abbas KS, Shehata J, et al. Survival trends for patients with retinoblastoma between 2000 and 2018: What has changed? Cancer Med 2023;12:6318-24. [Crossref] [PubMed]
- Yin F, Guo Z, Sun W, et al. Comparing overall survival between pediatric and adult retinoblastoma with the construction of nomogram for adult retinoblastoma: A SEER population-based analysis. Asian J Surg 2024;47:2178-87. [Crossref] [PubMed]
- Balachandran VP, Gonen M, Smith JJ, et al. Nomograms in oncology: more than meets the eye. Lancet Oncol 2015;16:e173-80. [Crossref] [PubMed]
- Wang Q, Qiao W, Zhang H, et al. Nomogram established on account of Lasso-Cox regression for predicting recurrence in patients with early-stage hepatocellular carcinoma. Front Immunol 2022;13:1019638. [Crossref] [PubMed]
- Chen X, Xie Q, Zhang X, et al. Nomogram Prediction Model for Diabetic Retinopathy Development in Type 2 Diabetes Mellitus Patients: A Retrospective Cohort Study. J Diabetes Res 2021;2021:3825155. [Crossref] [PubMed]
- Lv J, Liu YY, Jia YT, et al. A nomogram model for predicting prognosis of obstructive colorectal cancer. World J Surg Oncol 2021;19:337. [Crossref] [PubMed]
- Xiong Y, Shi X, Hu Q, et al. A Nomogram for Predicting Survival in Patients With Breast Cancer Liver Metastasis: A Population-Based Study. Front Oncol 2021;11:600768. [Crossref] [PubMed]
- Schober P, Vetter TR. Survival Analysis and Interpretation of Time-to-Event Data: The Tortoise and the Hare. Anesth Analg 2018;127:792-8. [Crossref] [PubMed]
- Guo X, Wang L, Beeraka NM, et al. Incidence Trends, Clinicopathologic Characteristics, and Overall Survival Prediction in Retinoblastoma Children: SEER Prognostic Nomogram Analysis. Oncologist 2024;29:e275-81. [Crossref] [PubMed]
- Zhang IY, Hart GR, Qin B, et al. Long-term survival and second malignant tumor prediction in pediatric, adolescent, and young adult cancer survivors using Random Survival Forests: a SEER analysis. Sci Rep 2023;13:1911. [Crossref] [PubMed]
- Lin J, Yin M, Liu L, et al. The Development of a Prediction Model Based on Random Survival Forest for the Postoperative Prognosis of Pancreatic Cancer: A SEER-Based Study. Cancers (Basel) 2022;14:4667. [Crossref] [PubMed]
- Che WQ, Li YJ, Tsang CK, et al. How to use the Surveillance, Epidemiology, and End Results (SEER) data: research design and methodology. Mil Med Res 2023;10:50. [Crossref] [PubMed]
- Marković L, Bukovac A, Varošanec AM, et al. Genetics in ophthalmology: molecular blueprints of retinoblastoma. Hum Genomics 2023;17:82. [Crossref] [PubMed]
- Roy SR, Kaliki S. Retinoblastoma: A Major Review. Mymensingh Med J 2021;30:881-95. [PubMed]
- Park HS, Lloyd S, Decker RH, et al. Overview of the Surveillance, Epidemiology, and End Results database: evolution, data variables, and quality assurance. Curr Probl Cancer 2012;36:183-90. [Crossref] [PubMed]
- Fang Y, He W, Wang H, et al. Key considerations in the design of real-world studies. Contemp Clin Trials 2020;96:106091. [Crossref] [PubMed]
- Fernandes AG, Pollock BD, Rabito FA. Retinoblastoma in the United States: A 40-Year Incidence and Survival Analysis. J Pediatr Ophthalmol Strabismus 2018;55:182-8. [Crossref] [PubMed]
- Ishwaran H, Lu M. Random Survival Forests. Wiley StatsRef: Statistics Reference Online. doi:
10.1002/9781118445112.stat08188 .10.1002/9781118445112.stat08188 - Huang Y, Guo Y. Quality of life among people with eye cancer: a systematic review from 2012 to 2022. Health Qual Life Outcomes 2024;22:3. [Crossref] [PubMed]
- Schaiquevich P, Francis JH, Cancela MB, et al. Treatment of Retinoblastoma: What Is the Latest and What Is the Future. Front Oncol 2022;12:822330. [Crossref] [PubMed]
- Kaewkhaw R, Rojanaporn D. Retinoblastoma: Etiology, Modeling, and Treatment. Cancers (Basel) 2020;12:2304. [Crossref] [PubMed]
- Kaliki S, Vempuluru VS, Mohamed A, et al. Retinoblastoma in Asia: Clinical Presentation and Treatment Outcomes in 2112 Patients from 33 Countries. Ophthalmology 2024;131:468-77. [Crossref] [PubMed]
- Kakarala CL, Raval VR, Mallu A, et al. Metastatic retinoblastoma at presentation: Clinical presentation, treatment, and outcomes. Oman J Ophthalmol 2023;16:524-8. [Crossref] [PubMed]
- Farhat W, Yeung V, Ross A, et al. Advances in biomaterials for the treatment of retinoblastoma. Biomater Sci 2022;10:5391-429. [Crossref] [PubMed]
- Haase A, Miroschnikov N, Klein S, et al. New retinoblastoma (RB) drug delivery approaches: anti-tumor effect of atrial natriuretic peptide (ANP)-conjugated hyaluronic-acid-coated gold nanoparticles for intraocular treatment of chemoresistant RB. Mol Oncol 2024;18:832-49. [Crossref] [PubMed]