Efficacy of three lung cancer prediction models in diagnosing benign and malignant pulmonary nodules
Highlight box
Key findings
• This study assessed three models and found that all three had diagnostic value, with the lung cancer biomarker panel (LCBP) model emerging as the most promising for the Chinese population due to its higher specificity, positive predictive value, and concordance rate. However, there is still room for further optimization of these models to improve their diagnostic performance.
What is known and what is new?
• Several predictive models exist for assessing the malignancy risk of pulmonary nodules, including the Mayo model, Peking University People’s Hospital (PKUPH) model, and LCBP model. These models incorporate various clinical and imaging factors to predict the probability of malignancy in pulmonary nodules.
• This study evaluated and compared the diagnostic efficacy of the three models in a Chinese population using clinical data from 428 patients with pulmonary nodules. These models showed differences in the diagnostic performance, with the LCBP model demonstrating the highest diagnostic efficacy.
What is the implication, and what should change now?
• The LCBP model, developed using data from multiple regions in China, showed a wider suitability in the Chinese population compared with the Mayo and PKUPH models.
• The study highlights the need for predictive models to be validated and optimized for specific populations to ensure accurate and reliable diagnostic outcomes.
• Further validation of the LCBP model in larger, more diverse patient populations is required to confirm its generalizability and robustness.
• Ongoing research should focus on refining and optimizing existing predictive models to improve their sensitivity, specificity, and overall diagnostic accuracy.
Introduction
Lung cancer is the most prevalent and fatal malignant tumor in China, with a mortality rate higher than that of developed countries, posing a severe threat to the lives and health of the Chinese population (1-3). Notably, the incidence and mortality of lung cancer in this population has increased steadily over the past few decades, with adenocarcinoma being the most predominant subtype (4).
The prognosis for lung cancer in China is extremely poor, as early-stage lung cancer is often difficult to detect, causing patients to miss the optimal window for treatment. The 5-year survival rate for patients with advanced lung cancer is less than 7.0%, whereas for early-stage patients who undergo surgical treatment, the 5-year survival rate exceeds 90% (5-7). Pulmonary nodules, defined as focal shadows within the lungs with a diameter ≤30 mm, can be as either benign or malignant; the latter represent the most common manifestation of early lung cancer (8). Therefore, enhancing the early detection rate of malignant pulmonary nodules to reduce the incidence and mortality of lung cancer has become a focal point of ongoing research.
In clinical practice, the nature of pulmonary nodules is typically assessed through a combination of imaging and serum tumor marker tests. Carcinoembryonic antigen (CEA), squamous cell carcinoma antigen (SCC-Ag), cytokeratin 19 fragment (CYFRA21-1), and pro-gastrin-releasing peptide (ProGRP) offer easy sample collection, minimal invasiveness, and greater patient acceptance (9-15). However, the serum levels of individual tumor markers are insufficient for distinguishing lung cancer (16,17).
Medical research has identified key factors contributing to lung cancer, notably smoking, age, gender, genetic susceptibility, nodule location, and nodule characteristics (7,18-27). To better assist in the clinical diagnosis of lung cancer, researchers have incorporated these high-risk factors as variables in statistical analyses for malignant pulmonary nodules, resulting in the development of predictive models for malignant lung nodules (28,29). The Mayo model, one of the earliest and most widely used models for predicting malignant lung nodules, is based on the population of the American Midwest and may not be entirely applicable to the Chinese population (30,31). The Peking University People’s Hospital (PKUPH) model, developed based on the Chinese population, has demonstrated superior diagnostic efficacy in many studies in China compared with other models. However, it still lacks extensive validation from international data and is not yet widely applicable (32,33). The lung cancer biomarker panel (LCBP) model, developed by Yang et al. (34), is based on the Chinese lung cancer diagnostic biomarker spectrum and may be more suited to the Chinese population. However, as a relatively new model, it still requires extensive sample validation and further optimization for broader clinical use (35).
In order to identify a relatively safe, accurate, and rapid predictive method, we compared the diagnostic efficacy of the three aforementioned malignant pulmonary nodule prediction models. We present this article in accordance with the TRIPOD reporting checklist (available at https://tcr.amegroups.com/article/view/10.21037/tcr-2025-468/rc).
Methods
Study participants
The study includes 428 patients with pulmonary nodules from Meizhou People’s Hospital diagnosed by computed tomography (CT) of the thorax from March 2021 through January 2023. These patients were screened based on inclusion and exclusion criteria and were divided into two groups: the cancer group (210 cases) and the noncancer group (218 cases). Before inclusion in this study, informed consent was signed by each patient. The research protocol was approved by the Ethics Committee of Meizhou People’s Hospital (approval No. 2024-C-48). The study was conducted in accordance with the Declaration of Helsinki and its subsequent amendments.
Inclusion criteria
The inclusion criteria were as follows: (I) pulmonary nodules detected by chest CT, with a size measured between 2 and 45 mm; (II) availability of complete CT imaging and other clinical data; (III) clear pathological report of pulmonary nodules; (IV) complete clinical and imaging data; and (V) clinically confirmed benign pulmonary nodules with good treatment prognosis.
Exclusion criteria
The exclusion criteria were as follows: (I) a history of thoracic tumors; (II) a history of malignant tumors treated within the past 5 years; (III) previous pulmonary surgery; (IV) no without clear pathological results or clinical diagnosis; (V) metastatic cancer from other sites to the lungs; and (VI) incomplete clinical or imaging data.
Retrospective collection of clinical data
The collected data included gender, age (years), nodule diameter (cm/mm), smoking history, spiculated sign, nodule location, family history of cancer, personal cancer history, calcification sign, boundary clarity, clinical diagnosis of pulmonary nodules, results from pathological biopsy of the nodules, and the results from testing for the four tumor markers of lung cancer. Imaging features were based on pre-treatment 64-slice spiral CT scans, spanning from lung apex to costophrenic angles. Patients held their breath post-inhalation for a full scan (slice thickness ≤5 mm) in one breath-hold. The most recent pathological biopsy results were used for tissue diagnosis.
Experimental methods
Risk prediction model formulae for pulmonary nodules
- In the Mayo model, the formulae for estimating the probability of malignancy in pulmonary nodules were as follows: Y = ex/(1 + ex); X = −6.8272 + 0.0391 × age + 0.7917 × smoking history + 1.3388 × cancer history + 0.1274 × nodule diameter + 1.0407 × spiculation + 0.7838 × upper lobe location (8).
- For the PKUPH model, the formulae for estimating the probability of malignancy in pulmonary nodules were as follows: Y = ex/(1 + ex); X = −4.496 + 0.07 × age + 0.0676 × nodule diameter + 0.736 × spiculation + 1.267 × family history of cancer − 1.615 × calcification − 1.408 × clear boundary (10).
- For the LCBP model, the formulae for estimating the probability of malignancy in pulmonary nodules were as follows: Y = ex/(1 + ex); X = −5.6017 + 0.0264 × age + 8.8539 × smoking history + 0.1859 × nodule diameter + 3.1865 × spiculation − 8.7109 × gender − 0.00001 × ProGRP + 0.0057 × SCC-Ag + 0.1686 × CYFRA21-1 − 0.00311 × CEA (11).
In these formulae, e is the base of the natural logarithm, the nodule diameter is expressed in millimeters (mm), and age is expressed as a number. Moreover, female =0 and male =1; smoking history =1 and no smoking history =0; a history of no lung cancer in the past 5 years =1 and no history =0; spiculation =1 and no spiculation =0; nodule location in the upper lobe =1 and other locations =0; calcification =1 and no calcification =0; clear nodule boundary =1 and no clear nodule boundary =0; and a family history of cancer =1 and no family history of cancer =0. Tumor markers include CEA (ng/mL), SCC (ng/mL), CYFRA21-1 (ng/mL), and ProGRP (pg/mL) serum expression levels. According to the kit instructions, tumor markers were considered positive if the detected values exceeded the normal reference range.
Research methods and procedures
- The various indicators between the cancer group (experimental group) and the noncancer group (referent group) were compared, and whether significant differences existed in each indicator between the two groups was determined. Additionally, we examined whether these indicators were influencing factors for lung cancer prediction and assessment.
- Receiver operating characteristic (ROC) curves were drawn using SPSS 27.0 (IBM Corp., Armonk, NY, USA) for the three models (Mayo Clinic, PKUPH and LCBP). The area under the curve (AUC) was computed, and when the AUC exceeded 0.7, the model was considered to have high diagnostic value. A fourfold table was used to calculate the sensitivity and specificity for each model. The accuracy of Mayo model for the study data from the hospital also being evaluated. The concordance rate of the PKUPH and LCBP models in the hospital’s research data was assessed.
- The Youden’s index (correct index) was used to determine the optimal diagnostic cutoff for each of the three models. The diagnostic sensitivity, specificity, positive predictive value, negative predictive value, positive likelihood ratio, and negative likelihood ratio were compared when the Youden index was maximized for each model. Pairwise comparisons of the AUCs of the three models were conducted to determine whether there were statistical differences. A P value <0.05 was considered statistically significant. Finally, a comprehensive analysis of the three models was conducted to draw conclusions.
- Optimization of the three models:
- A comprehensive analysis was performed to identify the optimal model, and further optimization was carried out by incorporating additional lung cancer risk factors or adjusting the model coefficients to achieve the highest sensitivity while maintaining the highest concordance rate and specificity in the hospital.
- Based on the lung cancer influencing factors identified through univariate analysis of the three models, a new model was constructed using a binary logistic regression equation and then validated.
Statistical methods
Descriptive and comparative analyses were conducted on the indicators of the cancer and noncancer groups. Continuous variables are expressed as means ± standard deviations, while categorical variables are expressed as frequencies and percentages. The diagnostic indicators between the benign and malignant lung nodule groups were compared using univariable binary logistic regression (for both continuous and categorical variables). Based on the patients’ pathological diagnosis of benign or malignant lung nodules and the malignancy probabilities estimated by the three models, ROC curves for each model were plotted using SPSS 27.0. Sensitivity and specificity were evaluated using fourfold contingency tables, and the AUC of the three models were compared using ROC analysis.
Results
Comparison of variables between the cancer and noncancer groups
Univariable logistic regression analysis was performed to identify the risk factors for lung cancer (Table 1). Age, nodule diameter, spiculation, upper lobe location, history of cancer, calcification, CEA, and CYFRA21-1 all had P<0.05, indicating that there were significant differences between the cancer and noncancer groups and that these factors may be key influences on lung cancer risk. In contrast, gender, smoking history, SCC-Ag, ProGRP, and clear boundary did not show significant correlations with lung cancer, as their P values were >0.05. Owing to the small number of patients with a family history of cancer, a comparison analysis of this factor was not possible in this study.
Table 1
Basic characteristics | Noncancer group (n=218) | Cancer group (n=210) | Wald | P |
---|---|---|---|---|
Age (years) | 58.85±13.84 | 62.19±10.34 | 7.64 | 0.006 |
Nodule diameter (mm) | 15.31±12.04 | 21.61±13.30 | 23.365 | <0.001 |
Gender | 1.205 | 0.27 | ||
Male | 142 (65.14) | 126 (60.00) | ||
Female | 76 (34.86) | 84 (40.00) | ||
Smoking history | 0.491 | 0.48 | ||
Yes | 79 (36.24) | 83 (39.52) | ||
No | 139 (63.76) | 127 (60.48) | ||
Spiculation | 127.218 | <0.001 | ||
Yes | 57 (26.15) | 181 (86.19) | ||
No | 161 (73.85) | 29 (13.81) | ||
Upper lobe | 19.885 | <0.001 | ||
Yes | 70 (32.11) | 113 (53.81) | ||
No | 148 (67.89) | 97 (46.19) | ||
History of tumor | 9.521 | 0.002 | ||
Yes | 2 (0.92) | 18 (8.57) | ||
No | 216 (99.08) | 192 (91.43) | ||
Family history of cancer | 0 | <0.001 | ||
Yes | 0 (0.00) | 0 (0.00) | ||
No | 218 (100.00) | 210 (100.00) | ||
Calcification sign | 5.047 | 0.02 | ||
Yes | 34 (15.60) | 18 (8.57) | ||
No | 184 (84.40) | 192 (91.43) | ||
Clear boundaries | 1.237 | 0.26 | ||
Yes | 132 (60.55) | 116 (55.24) | ||
No | 86 (39.45) | 94 (44.76) | ||
CEA (ng/mL) | 3.44±6.86 | 43.74±190.46 | 10.959 | 0.001 |
SCC-Ag (ng/mL) | 3.02±8.73 | 2.61±7.41 | 3.24 | 0.07 |
CYFRA21-1 (ng/mL) | 1.67±1.30 | 6.62±12.31 | 27.809 | <0.001 |
ProGRP (pg/mL) | 35.36±19.49 | 67.36±256.52 | 2.37 | 0.12 |
Data are presented as mean ± standard deviation or n (%). CEA, carcinoembryonic antigen; CYFRA21-1, cytokeratin 19 fragment; ProGRP, pro-gastrin-releasing peptide; SCC-Ag, squamous cell carcinoma antigen.
Diagnostic performance of the three models in predicting malignant lung nodules
As shown in Figure 1, the AUC values for the Mayo model, PKUPH model, LCBP model were 0.783 [95% confidence interval (CI): 0.739–0.827], 0.726 (95% CI: 0.678–0.774), and 0.759 (95% CI: 0.712–0.805), respectively, and all P values <0.05, indicating that these three models are applicable to the patients with lung nodules at Meizhou People’s Hospital. According to Table 2, the Mayo model had a sensitivity of 48.57%, a specificity of 84.40%, and a concordance rate of 66.82%; the PKUPH model had a sensitivity of 74.76%, a specificity of 60.55%, and a concordance rate of 67.52%; and the LCBP model had a sensitivity of 77.62%, a specificity of 67.43%, and a concordance rate of 72.43%. These results suggest that the sensitivity, specificity, and concordance rates of all three models were relatively low and did not meet the study’s expectations (sensitivity ≥90%, specificity ≥80%, and concordance rate ≥90%). This indicates a high rate of false negatives and false positives, suggesting that the thresholds for each model should be re-evaluated and adjusted to improve sensitivity, specificity, and concordance.

Table 2
Group | Noncancer group, n (%) | Cancer group, n (%) | Total, n (%) | Sensitivity (%) | Specificity (%) |
---|---|---|---|---|---|
Mayo model | 48.57 | 84.40 | |||
Concordance rate | 184 (84.40) | 102 (48.57) | 286 (66.82) | ||
Nonconcordance rate | 34 (15.60) | 108 (51.43) | 142 (33.18) | ||
PKUPH model | 74.76 | 60.55 | |||
Concordance rate | 132 (60.55) | 157 (74.76) | 289 (67.52) | ||
Nonconcordance rate | 86 (39.45) | 53 (25.24) | 139 (32.48) | ||
LCBP model | 77.62 | 67.43 | |||
Concordance rate | 147 (67.43) | 163 (77.62) | 310 (72.43) | ||
Nonconcordance rate | 71 (32.57) | 47 (22.38) | 118 (27.57) | ||
Total | 218 (100.00) | 210 (100.00) | 428 (100.00) |
The concordance rate or nonconcordance rate of the noncancer group: (compliance or noncompliance)/218. The concordance rate or nonconcordance rate of the cancer group: (compliance or noncompliance)/210. The concordance rate or nonconcordance rate of the total: (compliance or noncompliance)/428. LCBP, lung cancer biomarker panel; PKUPH, Peking University People’s Hospital.
Comparison of diagnostic performance of the three prediction models
New threshold determined by the maximum Youden index
The sensitivity, specificity, predictive values, likelihood ratios, and concordance rates for the three models at the new threshold are summarized in Table 3. The comparison of the diagnostic performance of the three models revealed improvements in the concordance rates for all models. The Mayo model showed the highest sensitivity (85.71%) and negative predictive value (82.14%) but the lowest negative likelihood ratio (0.226). The LCBP model exhibited the highest specificity (77.52%), positive predictive value (75.62%), and positive likelihood ratio (3.220). These results together suggest that, after the threshold adjustment by setting the maximum Youden index, the LCBP model may have a better positive prediction on malignant lung nodules than the other two models.
Table 3
Group | Youden index | Concordance rate (%) | Sensitivity (%) | Specificity (%) | Predictive value (%) | Likelihood ratio | |||
---|---|---|---|---|---|---|---|---|---|
Positive | Negative | Positive | Negative | ||||||
Mayo model | 0.490 | 74.3 (69.96–78.21) | 85.71 (80.34–89.91) | 63.30 (56.73–69.42) | 69.23 (63.37–74.53) | 82.14 (75.65–87.20) | 2.335 (1.795–3.039) | 0.226 (0.152–0.335) | |
PKUPH model | 0.412 | 70.3 (65.83–74.46) | 84.29 (78.75–88.59) | 56.88 (50.24–63.28) | 65.31 (59.47–70.73) | 78.98 (71.95–84.62) | 1.955 (1.522–2.510) | 0.276 (0.188–0.406) | |
LCBP model | 0.494 | 75.0 (70.69–78.87) | 72.38 (65.97–77.99) | 77.52 (71.53–82.56) | 75.62 (69.24–81.04) | 74.45 (68.40–79.68) | 3.220 (2.334–4.443) | 0.356 (0.264–0.480) |
Data are shown as ratio or percentage (95% confidence interval). LCBP, lung cancer biomarker panel; PKUPH, Peking University People’s Hospital.
Evaluation of prediction capability of the three models
A box plot was generated to visualize the prediction ability of the three models based on their predicted probabilities. As shown in Figure 2, in the benign group, the Mayo model and LCBP model demonstrated higher predictive ability than did the PKUPH model. In contrast, for the malignant group, the LCBP model outperformed both the Mayo and PKUPH models. Overall, after adjustment, the LCBP model showed superior AUC, sensitivity, and specificity compared to the other two models, indicating that it possesses the highest diagnostic efficacy and is more suitable for assessing the probability of malignant lung nodules.
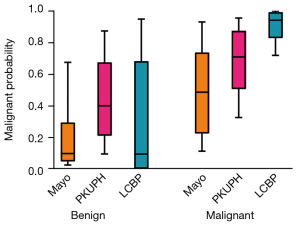
Discussion
Lung cancer risk prediction remains a focal point for researchers, with the study revealing that each of the three compared prediction models, especially the LCBP model after threshold adjustment, exhibits superior diagnostic performance based on a comprehensive consideration of various risk factors, with the LCBP model proving most suitable for assessing malignant lung nodules. These risk factors are as follows: (I) smoking, the most common high-risk factor for lung cancer (18,19); (II) age, with lung cancer being most prevalent in middle-aged and older adult individuals (20,21); (III) gender, with the incidence rate being higher in males than females (22); (IV) genetic susceptibility, with a family history of cancer in female relatives being associated with an increased risk of developing lung cancer (23,24); (V) nodule location, with the apex of the right upper lobe being the most common site for lung cancer in the Chinese population (25,26); and (VI) nodule characteristics, with larger, rough, irregularly lobulated, or spiculated nodules often indicative of a higher likelihood of malignancy (8). Nodule calcification typically suggests a benign nature but does not exclude the possibility of malignant lesions (27).
The development of predictive models, leveraging these risk factors, has emerged as a pivotal approach in aiding early lung cancer diagnosis and is gaining significant attention among researchers. Many guidelines, both within China and internationally, recommend using these models to predict lung cancer risk, thereby aiding clinicians in selecting optimal clinical decisions and reducing diagnostic and treatment risks for patients (36,37). The development of new risk prediction models aims to incorporate more valuable lung cancer-related factors and eliminate less significant ones, thus improving the accuracy of malignant pulmonary nodule prediction and guiding early lung cancer prevention (38).
Currently, the validated predictive models include the Mayo model, PKUPH model, and LCBP model. (I) The Mayo model, widely recognized and used internationally, is based on the Midwestern US population but has also proven valuable in Asian populations. In this study, the Mayo model’s AUC was 0.783, slightly lower than the original AUC of 0.833 (30). This discrepancy may be attributable to differences between the study populations in the Mayo model and Chinese cohorts; nonetheless, the model retains certain applicability in China. (II) The PKUPH model, China’s first model for predicting malignant pulmonary nodules, is specifically designed for Chinese patients (39). However, in this study, the PKUPH model’s AUC (0.726) fell short of the original AUC of 0.888, with a sensitivity and specificity below expectations. The literature (33) suggests that the PKUPH model may overemphasize the malignant tendency of spiculations and the benign tendencies of calcification and clear margins, leading to false positives in patients with spiculated but noncalcified nodules with unclear margins. Resolving this issue could significantly enhance the model’s sensitivity and specificity. (III) The LCBP model, developed using data from multiple regions in China, shows potential for national application (34). In this study, the AUC of the LCBP model (0.759) was lower than the initial AUC of 0.915. This discrepancy appears to arise from the model’s gender coefficient (−8.7109), which sharply reduces malignancy probability in nonsmoking male patients, resulting in false negatives. In this study, the optimal cut-off value for the probability of malignant pulmonary nodules in three risk assessment models for pulmonary nodules was determined by maximizing the Youden index. The data indicated that this adjustment strategy positively optimized all three models, with particularly significant improvements observed in the LCBP model, which exhibited a much higher positive predictive value for malignant pulmonary nodules compared to the Mayo model and the Peking University model. This adjustment not only made the LCBP model more suitable for clinical application needs but also significantly enhanced its accuracy in predicting malignant pulmonary nodules, providing strong support for clinicians in assessing the benignity or malignancy of pulmonary nodules and facilitating the promotion and application of this model in clinical practice.
It is worth noting that the effect of limited sample size on predictive accuracy could not been ignored for models development and validation. The Mayo model was developed using clinical data from 629 patients; the PKUPH model was created from a total of 371 cases; the LCBP model was based on a total of 715 participants. This study evaluated and compared the diagnostic efficacy of these three models using clinical data of 428 patients from a single tertiary center in Meizhou, a place gathering Hakka population with some unique eating and living habits. All three models exhibited different diagnostic efficacy in this population cohort. This discrepancy of these models between this study and other literatures may be attributable to the differences in the study populations and limited sample size. Undoubtly, our findings have limitation and need more validations. More populations and validations in different areas, larger sample size, and even subgroups should be used in the future studies, which will enhance the accuracy of these models in predicting malignant pulmonary nodules and make them more suitable for clinical application. A Chinese expert consensus on pulmonary nodules diagnosis and treatment (Year 2024 edition) recommended the LCBP model for domestic lung cancer risk assessment of pulmonary nodules, meanwhile the consensus pointed out that the use of the LCBP model guiding pulmonary nodules follow-up needs prospective studies (40). Altogether, prospective studies and more validations are important and necessary to promote the clinical application and continuous optimization of these predicative models.
Conclusions
In this study, all three malignant pulmonary nodule prediction models achieved an AUC >0.70, indicating their suitability for malignant nodule risk assessment with good diagnostic efficacy. Among them, the Mayo and LCBP models outperformed the PKUPH model in predicting benign cases, while the LCBP model showed markedly superior predictive ability in malignant cases. Overall, the LCBP model is superior to both the Mayo and PKUPH models in diagnostic performance, demonstrating higher diagnostic value for the Chinese population. However, there is still room for optimization in each model. Future models also may benefit from machine learning methods, along with including information on environmental and occupational exposures.
Acknowledgments
We thank Dr. Ramsy Abdelghani (Tulane University Medical Center, Tulane University School of Medicine, USA) for the critical comments and valuable advice on this study.
Footnote
Reporting Checklist: The authors have completed the TRIPOD reporting checklist. Available at https://tcr.amegroups.com/article/view/10.21037/tcr-2025-468/rc
Data Sharing Statement: Available at https://tcr.amegroups.com/article/view/10.21037/tcr-2025-468/dss
Peer Review File: Available at https://tcr.amegroups.com/article/view/10.21037/tcr-2025-468/prf
Funding: This study was supported by grants from
Conflicts of Interest: All authors have completed the ICMJE uniform disclosure form (available at https://tcr.amegroups.com/article/view/10.21037/tcr-2025-468/coif). J.T.E. serves as an unpaid editorial board member of Translational Cancer Research from August 2024 to July 2026. The other authors have no conflicts of interest to declare.
Ethical Statement: The authors are accountable for all aspects of the work in ensuring that questions related to the accuracy or integrity of any part of the work are appropriately investigated and resolved. The study was conducted in accordance with the Declaration of Helsinki and its subsequent amendments. The research protocol was approved by the Ethics Committee of Meizhou People’s Hospital (approval No. 2024-C-48). All patients provided written informed consent before taking part.
Open Access Statement: This is an Open Access article distributed in accordance with the Creative Commons Attribution-NonCommercial-NoDerivs 4.0 International License (CC BY-NC-ND 4.0), which permits the non-commercial replication and distribution of the article with the strict proviso that no changes or edits are made and the original work is properly cited (including links to both the formal publication through the relevant DOI and the license). See: https://creativecommons.org/licenses/by-nc-nd/4.0/.
References
- Cao W, Chen HD, Yu YW, et al. Changing profiles of cancer burden worldwide and in China: a secondary analysis of the global cancer statistics 2020. Chin Med J (Engl) 2021;134:783-91. [Crossref] [PubMed]
- Yang D, Liu Y, Bai C, et al. Epidemiology of lung cancer and lung cancer screening programs in China and the United States. Cancer Lett 2020;468:82-7. [Crossref] [PubMed]
- Cao M, Li H, Sun D, et al. Cancer burden of major cancers in China: A need for sustainable actions. Cancer Commun (Lond) 2020;40:205-10. [Crossref] [PubMed]
- Zhou C. Lung cancer molecular epidemiology in China: recent trends. Transl Lung Cancer Res 2014;3:270-9. [PubMed]
- Balata H, Quaife SL, Craig C, et al. Early Diagnosis and Lung Cancer Screening. Clin Oncol (R Coll Radiol) 2022;34:708-15. [Crossref] [PubMed]
- Wang N, Mengersen K, Tong S, et al. Lung Cancer Mortality in China: Spatial and Temporal Trends Among Subpopulations. Chest 2019;156:972-83. [Crossref] [PubMed]
- Sun X, Xu X, Wang Y, et al. Incidence and mortality rates of lung cancer in Qingdao, China (2013-2017). Asia Pac J Clin Oncol 2022;18:e73-8. [Crossref] [PubMed]
- Mazzone PJ, Lam L. Evaluating the Patient With a Pulmonary Nodule: A Review. JAMA 2022;327:264-73. [Crossref] [PubMed]
- Wu H, Wang Q, Liu Q, et al. The Serum Tumor Markers in Combination for Clinical Diagnosis of Lung Cancer. Clin Lab 2020;66: [Crossref] [PubMed]
- Vos D, Rao S, Pierce JD, et al. The Past, Present, and Future (Liquid Biopsy) of Serum Tumor Markers in Lung Cancer: A Primer for the Radiologist. J Comput Assist Tomogr 2021;45:950-8. [Crossref] [PubMed]
- Kuo YS, Zheng MY, Huang MF, et al. Association of Divergent Carcinoembryonic Antigen Patterns and Lung Cancer Progression. Sci Rep 2020;10:2066. [Crossref] [PubMed]
- Chen F, Zhang X. Predictive value of serum SCCA and CYFRA21-1 levels on radiotherapy efficacy and prognosis in patients with non-small cell lung cancer. Biotechnol Genet Eng Rev 2024;40:4205-14. [Crossref] [PubMed]
- Fu L, Wang R, Yin L, et al. CYFRA21-1 tests in the diagnosis of non-small cell lung cancer: A meta-analysis. Int J Biol Markers 2019;34:251-61. [Crossref] [PubMed]
- Yuan J, Sun Y, Wang K, et al. Development and validation of reassigned CEA, CYFRA21-1 and NSE-based models for lung cancer diagnosis and prognosis prediction. BMC Cancer 2022;22:686. [Crossref] [PubMed]
- He L, Wang J, Chang D, et al. Clinical value of Pro-GRP and T lymphocyte subpopulation for the assessment of immune functions of lung cancer patients after DC-CIK biological therapy. Exp Ther Med 2018;15:1580-5. [PubMed]
- Li J, Chen Y, Wang X, et al. The value of combined detection of CEA, CYFRA21-1, SCC-Ag, and pro-GRP in the differential diagnosis of lung cancer. Transl Cancer Res 2021;10:1900-6. [Crossref] [PubMed]
- Bi H, Yin L, Fang W, et al. Association of CEA, NSE, CYFRA 21-1, SCC-Ag, and ProGRP with Clinicopathological Characteristics and Chemotherapeutic Outcomes of Lung Cancer. Lab Med 2023;54:372-9. [Crossref] [PubMed]
- Nguyen RH, Vater LB, Timsina LR, et al. Impact of smoke-free ordinance strength on smoking prevalence and lung cancer incidence. PLoS One 2021;16:e0250285. [Crossref] [PubMed]
- Liu X, Yu Y, Wang M, et al. The mortality of lung cancer attributable to smoking among adults in China and the United States during 1990-2017. Cancer Commun (Lond) 2020;40:611-9. [Crossref] [PubMed]
- Pilleron S, Gower H, Janssen-Heijnen M, et al. Patterns of age disparities in colon and lung cancer survival: a systematic narrative literature review. BMJ Open 2021;11:e044239. [Crossref] [PubMed]
- Tamási L, Horváth K, Kiss Z, et al. Age and Gender Specific Lung Cancer Incidence and Mortality in Hungary: Trends from 2011 Through 2016. Pathol Oncol Res 2021;27:598862. [Crossref] [PubMed]
- Siegfried JM. Sex and Gender Differences in Lung Cancer and Chronic Obstructive Lung Disease. Endocrinology 2022;163:bqab254. [Crossref] [PubMed]
- Lan Q, Hsiung CA, Matsuo K, et al. Genome-wide association analysis identifies new lung cancer susceptibility loci in never-smoking women in Asia. Nat Genet 2012;44:1330-5. [Crossref] [PubMed]
- Corrales L, Rosell R, Cardona AF, et al. Lung cancer in never smokers: The role of different risk factors other than tobacco smoking. Crit Rev Oncol Hematol 2020;148:102895. [Crossref] [PubMed]
- Liu C, Li H, Xu K, et al. Multiple primary lung cancer versus intrapulmonary metastatic cancer: A case of multiple pulmonary nodules. Thorac Cancer 2019;10:352-8. [Crossref] [PubMed]
- Yang XN, Zhao ZR, Zhong WZ, et al. A lobe-specific lymphadenectomy protocol for solitary pulmonary nodules in non-small cell lung cancer. Chin J Cancer Res 2015;27:538-44. [PubMed]
- Zhang XC, Lv FJ, Fu BJ, et al. Significance of marginal vessels in differentiating peripheral small-cell lung cancer and benign lung tumor. Acta Radiol 2023;64:2526-34. [Crossref] [PubMed]
- Jacob M, Romano J, Ara Jo D, et al. Predicting lung nodules malignancy. Pulmonology 2022;28:454-60. [Crossref] [PubMed]
- Wu Z, Wang F, Cao W, et al. Lung cancer risk prediction models based on pulmonary nodules: A systematic review. Thorac Cancer 2022;13:664-77. [Crossref] [PubMed]
- Swensen SJ, Silverstein MD, Ilstrup DM, et al. The probability of malignancy in solitary pulmonary nodules. Application to small radiologically indeterminate nodules. Arch Intern Med 1997;157:849-55. [Crossref] [PubMed]
- Liu HY, Zhao XR, Chi M, et al. Risk assessment of malignancy in solitary pulmonary nodules in lung computed tomography: a multivariable predictive model study. Chin Med J (Engl) 2021;134:1687-94. [Crossref] [PubMed]
- Wu Z, Huang T, Zhang S, et al. A prediction model to evaluate the pretest risk of malignancy in solitary pulmonary nodules: evidence from a large Chinese southwestern population. J Cancer Res Clin Oncol 2021;147:275-85. [Crossref] [PubMed]
- Li Y, Chen KZ, Wang J. Development and validation of a clinical prediction model to estimate the probability of malignancy in solitary pulmonary nodules in Chinese people. Clin Lung Cancer 2011;12:313-9. [Crossref] [PubMed]
- Yang D, Zhang X, Powell CA, et al. Probability of cancer in high-risk patients predicted by the protein-based lung cancer biomarker panel in China: LCBP study. Cancer 2018;124:262-70. [Crossref] [PubMed]
- Ren XC, Wu H, Chen YF, et al. Clinical value of CT-guided percutaneous transthoracic needle biopsy and LCBP lung cancer prediction model in diagno-sing of benign and malignant pulmonary nodules. Journal of Medical Imaging 2022;32:952-6.
- Wang F, Tan F, Shen S, et al. Risk-stratified Approach for Never- and Ever-Smokers in Lung Cancer Screening: A Prospective Cohort Study in China. Am J Respir Crit Care Med 2023;207:77-88. [Crossref] [PubMed]
- Jia B, Zheng Q, Wang J, et al. A nomogram model to predict death rate among non-small cell lung cancer (NSCLC) patients with surgery in surveillance, epidemiology, and end results (SEER) database. BMC Cancer 2020;20:666. [Crossref] [PubMed]
- Liao Z, Zheng R, Shao G. A lung cancer risk prediction model for nonsmokers: A retrospective analysis of lung nodule cohorts in China. J Clin Lab Anal 2022;36:e24748. [Crossref] [PubMed]
- An Y, Li C, Zhao W. Validation of three lung cancer risk prediction models in patients with pulmonary nodules. Academic Journal of Chinese PLA Medical School 2020;41:1193-6, 1225.
- Chinese Thoracic Society, Chinese Medical Association. Chinese Alliance Against Lung Cancer Expert Group. Chinese expert consensus on diagnosis and treatment of pulmonary nodules(2024). Zhonghua Jie He He Hu Xi Za Zhi 2024;47:716-29.
(English Language Editor: J. Gray)