Artificial intelligence radiomics in the diagnosis, treatment, and prognosis of gynecological cancer: a literature review
Introduction
Gynecological cancer is a group of malignancies affecting the female reproductive system, with endometrial cancer, ovarian cancer, and cervical cancer being the most common. According to the GLOBOCAN estimates, there were an estimated 1,473,427 new cases of gynecological cancer and 680,372 deaths worldwide in 2022 (1). Globally, cervical cancer is the most common gynecological cancer, whereas ovarian cancer is the most lethal (2). Each gynecological cancer has a distinct pathogenesis and thus different clinical presentations. Pathogenesis and treatments of gynecological cancer, such as endometrial cancer, have evolved significantly during the last decade (3). Although significant therapeutic advancements have been made, effective treatment strategies are still lacking (4). Gynecological cancer can significantly affect women’s quality of life and cause a substantial burden to the healthcare system (5,6). Early diagnosis and individualized treatment remain the key in the management of gynecological cancer.
Radiomics is a rapidly developing field in oncology that applies advanced computational techniques to extract and mine high-dimensional quantitative features from medical images [e.g., magnetic resonance imaging (MRI), computed tomography (CT), positron emission tomography (PET), and ultrasonography (US)] (7). It has shown potential in the entire cancer patient journey: from early diagnosis to treatment decision support and prognosis prediction (8). Artificial intelligence (AI) refers to the ability of a computer to perform tasks that normally require human intelligence, such as image processing (9). The last decade has witnessed increasing development and implementation of AI-based methods in oncology imaging (10). As a combination of radiomics and AI techniques, AI radiomics has been increasingly developed to assist clinicians in clinical cancer care. The typical workflow of AI radiomics consists of imaging data collection and pre-processing, tumor segmentation, feature extraction and selection, and model construction and validation (Figure 1).
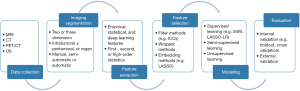
Substantial progress has been made with AI radiomics in clinical oncology such as lung and breast cancers (11,12). In gynecological cancer, Shrestha et al. conducted a systematic review reporting the use of AI in gynecologic imaging in 2022 (13). In 2024, Moro et al. summarized the applications of AI to ultrasound in gynecology cancer (14). Leo et al. reviewed AI and MRI-based radiomics in risk stratification and prognostic prediction of endometrial cancer (15). Meanwhile, Di Donato et al. assessed the role of MRI radiomics analysis for the prediction of molecular or clinicopathological prognostic factors, including tumor grading, deep myometrial invasion (MI), lymphovascular space invasion (LVSI), and nodal metastasis, in endometrial cancer (16). Egemen et al. summarized AI-based image analysis in cervical cancer screening (17). Sadeghi et al. reviewed deep learning (DL) and various imaging modalities in ovarian cancer diagnosis (18). However, most of the previous reviews have focused on the diagnosis, treatment, or prognosis of gynecological cancer or specific subtypes or using specific imaging data. Herein, we conducted a comprehensive review of the available literature on the application of AI radiomics in the management of gynecological cancer. This study provides an overview of AI radiomics use in gynecological cancer, including current landscape, challenges, and future directions. We present this article in accordance with the Narrative Review reporting checklist (available at https://tcr.amegroups.com/article/view/10.21037/tcr-2025-618/rc).
Methods
We searched the databases of PubMed, Embase, and Web of Science from January 2019 to September 2024 to explore the use of AI radiomics in gynecological cancer (Table 1). All identified studies were imported into EndNote software (Clarivate, Philadelphia, PA, USA) and screened according to the eligibility criteria. The inclusion criteria were studies involving AI radiomics in gynecological cancer (endometrial cancer, ovarian cancer, and cervical cancer) and including information related to tumor diagnosis (e.g., diagnosis and characterization), or treatment response or prognosis (recurrence, metastasis, or survival) prediction. Studies using AI radiomics for treatment plans of radiotherapy or using AI in field other than radiomics (such as colposcopy) were out of the scope of this review and therefore excluded. Other exclusion criteria were studies with article types other than original journal articles (e.g., case reports, letters, editorials, reviews), without full text (e.g., conference abstract only), or those not reported in English.
Table 1
Items | Specification |
---|---|
Dates of searches | 10 September 2024 |
Databases and other sources searched | PubMed, Embase, Web of Science |
Search terms used | “gynecological cancer”, “cervical cancer”, “endometrial cancer”, “ovarian cancer”, AND “artificial intelligence”, “AI”, AND “radiomics” |
Timeframe | From January 2019 to September 2024 |
Inclusion and exclusion criteria | Inclusion criteria: |
• Studies involving AI radiomics in gynecological cancer (endometrial cancer, ovarian cancer, and cervical cancer) and including information related to tumor diagnosis (e.g., diagnosis and characterization), or treatment response or prognosis (recurrence, metastasis, or survival) prediction | |
Exclusion criteria: | |
• Studies using AI radiomics for treatment plans of radiotherapy or using AI in field other than radiomics (such as colposcopy) | |
• Studies with article types other than original journal articles (e.g., case reports, letters, editorials, reviews), without full text (e.g., conference abstract only), or those not reported in English | |
Selection process | All authors participated in the literature screening. The discrepancy was resolved by consensus |
AI, artificial intelligence.
In total, 128 studies were included in the final analysis (Figure S1). The applications were grouped based on clinical scenarios in which they were used (Figure 2). Data were extracted for each purpose of use.
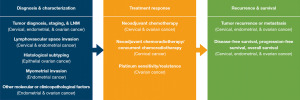
Tumor diagnosis and characterization
Tumor diagnosis
Accuracy in tumor diagnosis and characterization is undoubtedly a key issue in the management of gynecological cancer, which can sometimes be challenging when using conventional imaging techniques. Among 23 studies identified in tumor diagnosis, 15 (65.2%) were performed on ovarian cancer (Table 2).
Table 2
Author, year | Study | Patients [n] | Utility | Imaging | Methods | Validation [n] | Results |
---|---|---|---|---|---|---|---|
Urushibara et al. 2021 (19) | Single-center retrospective | Benign or malignant cervical lesions [418] | Diagnosis | MRI | CNN | NA | Sensitivity: 0.883; specificity: 0.933; accuracy: 0.908 |
Yang et al. 2024 (20) | Multi-center retrospective | Benign or malignant cervical lesions [261] | Diagnosis | US | Machine learning | Internal validation [63]; external validation [52] | AUC: 0.828 and 0.839 (internal and external validation, respectively) |
Li et al. 2021 (21) | Multi-center retrospective | Normal or malignant endometrial lesions [926] | Diagnosis | CT | Machine learning | Internal validation [83] and testing [83]; external validation [83] | AUC: 0.89 and 0.73 (internal and external test, respectively) |
Bi et al. 2022 (22) | Two-center retrospective | Benign or malignant endometrial lesions [371] | Diagnosis | MRI | Machine learning | Internal validation [82]; external validation [44] | AUC: 0.910 and 0.798 (internal and external validation, respectively) |
Urushibara et al. 2022 (23) | Single-center retrospective | Benign or malignant endometrial lesions [485] | Diagnosis | MRI | CNN | Internal validation [97] | Combined image sets: AUC: 0.87–0.93 |
Saida et al. 2022 (24) | Single-center retrospective | Carcinosarcoma or EC [331] | Differential diagnosis | MRI | CNN | Internal validation [66] | AUC: 0.80-0.94 (testing) |
Virarkar et al. 2024 (25) | Single-center retrospective | Malignant endometrial tumors [61] | Differential diagnosis | MRI | Machine learning | Five-fold cross-validation | AUC: 0.887 |
Capasso et al. 2024 (26) | Single-center retrospective | Benign or malignant endometrial lesions [302] | Diagnosis | US | Machine learning | Hold-out test | Interval validation: AUC: 0.90; hold-out test: AUC: 0.88 |
Wang et al. 2021 (27) | Single-center retrospective | Ovarian tumors [545] | Differential diagnosis | MRI | CNN | Internal validation [108] and testing [53] | Testing cohort: accuracy: 0.87; specificity: 0.92; sensitivity: 0.75 |
Hu et al. 2021 (28) | Single-center retrospective | Primary or secondary OC [110] | Differential diagnosis | CT | Logistic regression | Internal validation [34] | AUC: 0.854 and 0.751 (training and validation, respectively) |
Chiappa et al. 2021 (29) | Single-center retrospective | Benign or malignant ovarian masses [241] | Differential diagnosis | US | Machine learning | Nested K-fold cross validation | AUC: 0.87 (solid masses); 0.88 (cystic masses); and 0.89 (motley masses) |
Jian et al. 2021 (30) | Multi-center retrospective | Borderline or malignant ovarian lesions [501] | Differential diagnosis | MRI | Multiple instance CNN | Internal validation [119] | AUC: 0.878 |
Liu et al. 2022 (31) | Single-center retrospective | Borderline or malignant ovarian lesions [196] | Differential diagnosis | MRI | Machine learning | Internal validation [97] | 2D coronal model: AUC: 0.79; 2D sagittal model: AUC: 0.82; 3D coronal model: AUC: 0.84; 3D sagittal model: AUC: 1.0 |
Liu et al. 2022 (32) | Single-center retrospective | Ovarian tumors [135] | Diagnosis | CT | CNN | Internal validation [39] | AUC: 0.929 and 0.909 (training and test, respectively) |
Li et al. 2022 (33) | Single-center retrospective | Benign or malignant ovarian tumors [1,329] | Diagnosis | CECT | Machine learning | Internal validation [399] | AUC: 0.94–0.95 and 0.93–0.96 (training and validation, respectively) |
Saida et al. 2022 (34) | Single-center retrospective | Non-malignant or borderline or malignant ovarian lesions [465] | Diagnosis | MRI | CNN | Internal validation [110] | AUC: 0.83–0.89 |
Akazawa et al. 2023 (35) | Single-center retrospective | Benign, borderline or malignant ovarian lesions [185] | Differential diagnosis | MRI | CNN | Internal validation (split: 8:2) | Accuracy: 0.628; AUC: 0.529 |
Feng et al. 2024 (36) | Single-center retrospective | Ovarian lesions, and other lesions [754] | Diagnosis | MRI | BP neural network | Internal validation (split: 3:1) | AUC: 0.948; sensitivity: 91.9%; specificity: 86.9% (validation) |
Du et al. 2024 (37) | Single-center retrospective | Ovarian tumors [849] | Diagnosis | US | Deep learning | Internal validation [170] | AUC: 0.985 and 0.928; and 0.955 and 0.869 (training and testing, respectively) for malignant risk of overall and O-RADS 4/5 ovarian tumors |
Rong et al. 2024 (38) | Single-center retrospective | Benign and malignant ovarian tumors [135] | Diagnosis | CT | Deep learning | Five-fold cross-validation | AUC: 0.914 |
Zheng et al. 2024 (39) | Single-center retrospective | Ovarian thecoma-fibroma or OC [156] | Differential diagnosis | MRI | CNN | Internal validation and testing (split: 8:1:1) | Average AUC: 0.919 |
Chiappa et al. 2021 (40) | Single-center retrospective & prospective | Ovarian masses [274] | Malignant risk | US | Machine learning | Independent validation [35] | Accuracy: 91%; sensitivity: 100%; specificity 80% |
Liu et al. 2024 (41) | Two-center retrospective | Ovarian masses [1,080] | Malignant risk | US | Machine learning | Internal validation [397] | AUC: 0.930 (test) |
Zhao et al. 2022 (42) | Single-center retrospective | CC [57] | Staging | MRI | Machine learning | Internal validation [15] | AUC: 0.915 and 0.907 (training and testing, respectively) |
Cibi et al. 2023 (43) | NA | CC [54] | Staging | MRI | Deep capsule network | Five-fold cross validation | Accuracy: 0.903 |
Umutlu et al. 2024 (44) | Single-center prospective | CC [30] | N- and M-staging | PET/MRI | Machine learning | Five-fold cross-validation | AUC: 0.82 for N staging and 0.97 for M staging |
Aouadi et al. 2024 (45) | Single-center retrospective | CC [85] | Staging & grading | MRI | Deep CNN | Five-fold cross validation | AUC: 0.924 for grade prediction; AUC: 0.931 for stage prediction |
Cheng et al. 2024 (46) | Single-center retrospective | CC [119] | Staging | MRI | Deformable ConvLSTM | External validation [335] | AUC of 0.75 (BraTS 2019) |
Chang et al. 2024 (47) | Single-center retrospective | CC [90] | Staging | MRI | Deep residual network | Internal validation and testing | Precision: 93.6%; sensitivity: 91.2%; specificity: 92.2% |
Mao et al. 2022 (48) | Single-center retrospective | EC [117] | Staging | MRI | Deep learning | Internal validation [12] and test [35] | AUC: 0.85–0.94 |
Li et al. 2024 (49) | Single-center retrospective | EC [117] | Staging | Multi-modality | Latent relation shared learning | Ten-fold cross validation | AUC: 0.791–0.877 |
Sadeghi et al. 2023 (50) | Single-center retrospective | OC [37] | Classification & staging | PET/CT | 3D CNN | Internal validation [10] | AUC: 0.990 and 0.995 for diagnostic classification and staging |
Jian et al. 2021 (51) | Multi-center retrospective | EOC [294] | Histological types | MRI | Machine learning | Internal validation [75]; external validation [75] | AUC: 0.806 and 0.847 (internal and external validation, respectively) |
Song et al. 2021 (52) | Single-center retrospective | EOC [200] | Histological types | MRI | Machine learning | Five-fold cross-validation | AUC: 0.80–0.83 for five histological types; 0.91–0.93 for HGSOC versus non-HGSOC |
Li et al. 2023 (53) | Single-center retrospective | EOC [470] | Histological types | CECT | Machine learning | Internal validation [141] | Combined model: AUC: 0.900 and 0.934 (training and validation, respectively) |
Wei et al. 2024 (54) | Multi-center retrospective | EOC [437] | Histological types | MRI | Deep learning | Internal validation [68]; external validation [98] | AUC: 0.888 and 0.866 (internal and external validation, respectively) |
Du et al. 2024 (55) | Single-center retrospective | EOC [311] | Histological types | MRI | Deep learning | Internal validation [50]; external validation [63] | AUC: 0.916 (test) |
AI, artificial intelligence; AUC, area under the curve; CC, cervical cancer; CECT, contrast-enhanced CT; CNN, convolutional neural network; CT, computed tomography; EC, endometrial carcinoma; EOC, epithelial ovarian cancers; HGSOC, high-grade serous ovarian cancer; MRI, magnetic resonance imaging; NA, not applicable; OC, ovarian cancer; PET/CT, positron emission tomography/computed tomography; US, ultrasonography.
Cervical cancer
Urushibara et al. constructed an MRI-based model, Xception, that showed diagnostic performance equivalent to that of experienced radiologists in cervical cancer, with an area under the curve (AUC) of 0.931 (19). Furthermore, Yang et al. developed an interpretable model based on clinical and ultrasound-radiomics data for the diagnosis of stage I cervical cancer. The combined model showed good discriminative performance, with AUCs of 0.837, 0.828, and 0.839 in the training, internal validation, and external validation sets, respectively (20).
Endometrial cancer
Six studies were identified in endometrial cancer (21-26). For example, an MRI-based DL model developed by Urushibara et al. using convolutional neural networks (CNNs) demonstrated diagnostic performance not inferior to that of the radiologists (23). Bi et al. evaluated the performance of multiparametric MRI-based radiomics models in the differential diagnosis of stage IA endometrial cancer from benign lesions. Nine machine learning (ML) algorithms were used to determine the optimal radiomics model. The nomogram based on clinical and optimal radiomics models showed excellent and stable performance in the internal and external validation sets (AUCs of 0.917 and 0.802, respectively), suggesting that multiparametric MRI-based radiomics models could differentiate stage IA endometrial cancer in a non-invasive way (22). Moreover, Virarkar et al. analyzed MRI-based radiomics data from patients with malignant mixed Müllerian tumors and endometrial cancer to identify distinguishing features for differential diagnosis of these tumors (25). In addition to MRI, radiomics models based on CT or ultrasound were also developed for differential diagnosis of endometrial cancer (21,26).
Ovarian cancer
Several studies have attempted AI radiomics methods based on CT, MRI, or ultrasound for the differential diagnosis of cervical cancer (27-41). Almost all of these studies were single-center and retrospective. Hu et al. combined CT radiomics and clinical data to differentiate between primary and secondary ovarian cancers achieving an AUC of 0.854 in the training cohort and 0.71 in the internal validation cohort (28). In a single-center retrospective study, Li et al. included 1,329 patients with ovarian tumors, and six ML-based CT radiomics models and a clinical-radiomics mixed model were developed to distinguish benign and malignant tumors. The mixed model showed differentiation performance superior to that of radiomics models and senior radiologists (AUC: 0.93–0.96 vs. 0.88–0.91 vs. 0.78 in the validation cohorts) (33). A multiple-instance learning method based on multimodal MRI, MAC-Net, was used to differentiate between malignant and borderline ovarian lesions in a multicenter retrospective study by Jian et al. The MAC-Net outperformed several known multiple-instance learning approaches (AUC: 0.878 vs. 0.817–0.863) (30). Regarding ultrasound, Du et al. developed an ultrasound-based DL radiomics nomogram that showed a diagnostic capability comparable to that of the ovarian-adnexal reporting and data system (O-RADS) (AUC: 0.928 vs. 0.960 in the testing set). Moreover, the nomogram also exhibited superior performance in risk classification of malignancy in O-RADS 4 and 5 ovarian tumors (AUC: 0.869 in the testing set) (37). Liu et al. included 1,080 patients with ovarian masses from two hospitals to develop and validate an ultrasound-based clinical-radiomics nomogram in the risk classification of ovarian lesions. The nomogram achieved a higher performance when compared with the junior radiologists in the external test cohort (AUC: 0.930 vs. 0.802). With the assistance of the nomogram, the diagnostic performance of the junior radiologists was significantly improved (the average AUC: 0.929) (41).
Tumor staging
Nine studies were identified to use AI radiomics for tumor staging; most were performed on cervical cancer (n=6, 67.7%) and used MRI radiomics data (n=6, 67.7%) (Table 2).
Cervical cancer
Among the six studies on cervical cancer (42-47), only one study by Cheng et al. included more than 100 patients. To address the challenge of small sample size in their study, a sequence enhancement strategy was introduced to diversify samples and mitigate overfitting. They developed a deformable multi-sequence guidance model (DMGM) based on a novel deformable ConvLSTM module that enables the model to adapt to varying data. External validation using the multi-modal data from BraTS 2019 demonstrated the effectiveness of the DMGM model in tumor staging (46). Additionally, Umutlu et al. used multiparametric PET/MR imaging to develop an ML model for predicting node and metastasis staging. The developed model showed high performance for non-invasive tumor phenotyping (44).
Endometrial cancer
Two studies were included for endometrial cancer staging. Mao et al. developed an automatic DL-based prediction model for early endometrial cancer on MRI images using the area ratio of the tumor region to the uterine region as a reference (48). Interestingly, Li et al. proposed a latent relation shared learning method to overcome the incomplete multi-modality imaging data (e.g., MRI, ultrasound, and contrast-enhanced ultrasound). By jointly exploiting the consistent and complementary information from multi-modality data, the new method outperformed the current representative approaches. The method performed well under varying imaging missing rates (49).
Ovarian cancer
The only study by Sadeghi et al. created three-dimensional (3D) CNN systems (the OCDA-Net) based on whole-body PET/CT for the classification and staging of ovarian cancer. The OCDAc-Net achieved an AUC of 0.990 for diagnostic classification and the OCDAs-Net achieved an AUC of 0.995 for tumor staging (50).
Histological subtyping
Five studies were identified to use MRI/CT-based AI radiomics for histological subtyping; all were conducted on epithelial ovarian cancer (51-55) (Table 2). Jian et al. constructed an ML model based on MRI to differentiate between type I and type II epithelial ovarian cancers. The combined model based on four MRI sequences showed differential performance superior to each of the single-parametric radiomics models in both internal and external validation cohorts (51). ML models based on CT-based radiomics data were developed for histological subtyping of epithelial ovarian cancers (53). Again, DL methods were used to differentiate type Ι and type ΙΙ epithelial ovarian cancers using MRI radiomics (54,55).
Lymph node metastases
Among 15 studies using AI radiomics for lymph node metastasis (LNM) prediction, most were performed on cervical cancer (n=12, 80%) (56-67), followed by endometrial cancer (n=2, 13%) and ovarian cancer (n=1, 7%) (Table 3). All studies were retrospective except one.
Table 3
Author, year | Study | Patients [n] | Utility | Imaging | Methods | Validation [n] | Results |
---|---|---|---|---|---|---|---|
Dong et al. 2020 (56) | Two-center retrospective | CC [226] | LNM | CT | Deep neural network | Internal validation [50]; external validation [50] | AUC: 0.99 and 0.90 (internal and external validation, respectively) |
Wu et al. 2020 (57) | Multi-center retrospective | CC [479] | LNM | MRI | Deep learning | External validation [141] | AUC: 0.933 (external validation) |
Liu et al. 2021 (58) | Multi-center retrospective | CC [219]; LNs [273] | Normal-sized LNM | CT | Artificial neural network | Internal validation (LNs, 74); external validation (LNs, 51) | AUC: 0.859 and 0.800 (internal and external validation, respectively) |
Zhang et al. 2022 (59) | Single-center retrospective | CC [148] | PLNM, COX-2 | PET/CT | Machine learning | Internal validation [44] | AUC: 0.817 and 0.786 for PLNM; 0.814 and 0.748 for COX-2 (training and validation) |
Arezzo et al. 2023 (60) | Single-center retrospective | CC [92] | LNM | MRI | Machine learning | Ten-fold cross-validation | Accuracy: 89%; precision: 83%; recall: 78%; AUC: 0.79 |
Liu et al. 2023 (61) | Single-center retrospective | CC [180] | LNM | MRI | Machine learning | Internal validation [54] | AUC: 0.745; specificity: 0.900, and accuracy: 0.778 |
Lucia et al. 2023 (62) | Multi-center retrospective | CC [239] | PALNM | PET/CT, MRI | Multilayer perceptron network | Internal validation [76]; external validation [30; 31] | C-statistics: 0.88 to 0.96 (training and validation, respectively) |
Monthatip et al. 2024 (63) | Single-center retrospective | CC [832] | PLNM | CT | Machine learning | Repeated nested cross-validation | AUC: 0.869–0.910 |
Li et al. 2023 (64) | Two-center retrospective | CC [480] | LNM | CT | CNN | Internal validation [122]; external validation [62] | AUC: 0.925 (training), 0.771 (internal validation), and 0.790 (external validation) |
Qin et al. 2024 (65) | Multi-center retrospective | CC [392] | LNM | MRI | Deep multiple-instance learning | Internal validation [98]; external validation [69] | AUC: 0.838 (training), 0.764 (internal validation), and 0.835 (external validation) |
Yang et al. 2024 (66) | Single-center retrospective | CC [131] | LNM | US | Machine learning | Five-fold cross-validation | AUC: 0.898 (training) and 0.905(validation) |
Yang et al. 2024 (67) | Single-center retrospective | CC [106]; LNs [193] | LNM | PET/CT | Neural network | Internal validation [58] | AUC: 0.983 (training) and 0.860 (validation) |
Yang et al. 2021 (68) | Single-center prospective | EC [236] | LNM | MRI | Decision-tree | Internal validation [71] | AUC: 0.85 |
Liu et al. 2024 (69) | Multi-center retrospective | EC [186] | LNM | US | Machine learning | Internal validation (split: 7:3) | AUC: 0.919 and 0.884 (training and validation, respectively) |
Yao et al. 2022 (70) | Single-center retrospective | OC [224] | LNM | PET/CT | RNN and SVM | Internal validation [67] | AUC: 0.947 and 0.926 (training and validation, respectively) |
Huang et al. 2022 (71) | Single-center retrospective | CC [125] | LVSI | MRI | Logistic regression | Internal validation [25] | AUC: 0.940 (validation) |
Wang et al. 2023 (72) | Two-center retrospective | CC [300] | LVSI | MRI | SVM | External validation [102] | AUC: 0.805–0.873 (training) and 0.629–0.780 (external validation) |
Wu et al. 2023 (73) | Two-center retrospective | CC [168] | LVSI | MRI | Machine learning | External validation [29] | AUC of 0.883 (training) and 0.830 (external validation) |
Luo et al. 2020 (74) | Single-center retrospective | EC [144] | LVSI | MRI | Machine learning | Internal validation [43] | AUC: 0.820 (training) and 0.807 (validation) |
Yan et al. 2023 (75) | Single-center retrospective | EA [334] | LVSI | MRI | SVM | Internal validation [101] | AUC: 0.962 (training) and 0.965 (validation) |
Chen et al. 2020 (76) | Single-center retrospective | EC [530] | MI | MRI | Two-stage automatic deep learning | Internal validation [79] and test [138] | Accuracy: 86.2%; sensitivity: 77.8%; specificity: 87.5% |
Rodrsensitivity et al. 2021 (77) | Single-center retrospective | EC [143] | MI | MRI | Adaboost machine learning | Internal validation [36] | AUC: 0.871 (validation) |
Stanzione et al. 2021 (78) | Single-center retrospective | EC [54] | MI | MRI | Machine learning | Internal validation [11] | AUC: 0.92 (training) and 0.94 (validation) |
Wang et al. 2023 (79) | Single-center retrospective | EA [266] | MI | MRI | Machine learning | Internal validation [81] | AUC: 0.744, 0.869, and 0.883 |
Xiong et al. 2023 (80) | Single-center retrospective | EC [154] | MI | MRI | Multi-stage deep learning | Internal validation [46] | AUC: 0.89 vs. 0.81 radiologists |
Lefebvre et al. 2023 (81) | Two-center retrospective | EC [128] | MI & HG | MRI | Spherical harmonics; machine learning | External validation [53] | AUC: 0.94 for predicting deep MI; 0.81 for predicting high-grade tumor histology |
Xiao et al. 2024 (82) | Multi-center retrospective | CC [650] | Deep stromal invasion | MRI | Deep learning | External validations [62 & 52] | AUC: 0.933, 0.807, and 0.817 (primary and external validation cohorts, respectively) |
Hu et al. 2024 (83) | Single-center retrospective | CC [218] | Parametrial invasion | MRI | Machine learning | Internal validation [68] | AUC: 0.747–0.895 and 0.739–0.905 (training and validation cohorts, respectively) |
Chong et al. 2021 (84) | Single-center retrospective | CC [76] | Tumor budding | PET/CT | Machine learning | Internal validation [25] | AUC: 0.762 (validation cohort) |
Chong et al. 2021 (85) | Retrospective | CC [74] | Tumor budding | MRI | Machine learning | Internal validation [26] | AUC: 0.731–0.885 (validation cohort) |
Mainenti et al. 2022 (86) | Two-center retrospective | EC [133] | Risk classification (stage & grade) | MRI | SVM | External validation [29] | AUC: 0.78 (training); 0.71 (external validation) |
Moro et al. 2022 (87) | Multi-center retrospective | EC [498] | Risk classification (ESMO-ESGO-ESTRO 2016) | US | Machine learning | Internal validation [102] | AUC: 0.88/0.90 for high risk prediction; 0.85/0.88 for low risk prediction |
Yang et al. 2023 (88) | Single-center retrospective | EA [168] | Risk classification (ESMO-ESGO-ESTRO 2020) | MRI | Deep learning | Internal validation [73] | AUC: 0.923 (training); 0.842 (validation) |
Lefebvre et al. 2022 (89) | Two-center retrospective | EC [157] | MI, LVSI, HG, FIGO stage | MRI | Random forest | External validation [63] | AUC in test set: 0.81 for deep MI, 0.80 for LVSI, 0.74 for high grade, and 0.84 for FIGO stage |
Otani et al. 2022 (90) | Single-center retrospective | EC [200] | MI, LVI, HG, PLNM, PALNM | MRI | XGBoost | Internal validation [50] | AUC: 0.83 for MI, 0.81 for LVI, 0.77 for histological grade, 0.72 for PLNM, and 0.82 for PALNM |
Fang et al. 2024 (91) | Two-center retrospective | EC [198] | MI, CSI | MRI | SVM | External validation [60] | AUC: 0.928 and 0.869 for DMI and 0.913 and 0.937 for CSI (training and validation, respectively) |
Li et al. 2023 (92) | Multi-center retrospective | EC [495] | MI, clinical risk, histological type, LVSI | MRI | Machine learning | Independent validation [82] | AUCs: 0.79, 0.82, 0.91, and 0.85 for DMI, high-risk EC, histological type, and LVSI |
Zhang et al. 2024 (93) | Single-center retrospective | CC [180] | PI, LNM, DMI, LVSI, PT, DD, Ki-67 | IVIM-DWI | Machine learning | Internal validation [60] | AUC: 0.981 for PI; 0.848 for LNM; 0.896 for DMI; 0.840 for LVSI; 0.856 for PT; 0.810 for DD; 0.832 for Ki-67 |
Wang et al. 2024 (94) | Multi-center retrospective | CC [621] | LNM, LVSI | MRI | Multi-task deep learning | Internal validation [169]; external validation [54] | AUC: 0.895/0.848 (training), 0.806/0.795 (internal validation), and 0.804/0.728 (external validation) for LNM/LVSI positive |
AI, artificial intelligence; AUC, area under the curve; CC, cervical cancer; CECT, contrast-enhanced CT; CNN, convolutional neural network; CSI, cervical stromal invasion; CT, computed tomography; DD, differentiation degree; DMI, deep muscle invasion; EA, endometrial adenocarcinoma; EC, endometrial carcinoma; ESGO, European Society of Gynaecological Oncology; ESMO, European Society for Medical Oncology; EOC, epithelial ovarian cancers; ESTRO, European SocieTy for Radiotherapy & Oncology; FIGO, International Federation of Gynecology and Obstetrics; HG, histological grade; IVIM-DWI, intravoxel incoherent motion diffusion-weighted imaging; LNM, lymph node metastasis; LNs, lymph nodes; LVSI, lymphovascular space invasion; MI, myometrial invasion; MRI, magnetic resonance imaging; OC, ovarian cancer; PALNM, paraaortic lymph node metastasis; PET/CT, positron emission tomography/computed tomography; PI, parametrial infiltration; PLNM, pelvic lymph node metastasis; PT, pathological type; RNN, residual neural network; SVM, support vector machine; US, ultrasonography.
Cervical cancer
Eight studies used MRI or CT radiomics for model development in cervical cancer. Wu et al. developed an MRI-based DL model for LNM prediction in cervical cancer. The predictive performance of three MRI sequences and different regions (intratumoral and peritumoral) were explored to find the optimal model. The DL model based on contrast-enhanced T1-weighted imaging in both the intratumoral and peritumoral regions showed the best performance (AUCs of 0.894 and 0.844 in the training and validation cohorts, respectively). The predictive performance was further improved by combining the optimal DL model and MRI lymph node status in a hybrid model (AUCs of 0.963 and 0.933 in the training and validation cohorts, respectively) (57). Similarly, clinical and MRI radiomics data-integrated models were developed by Liu et al. (61) and Qin et al. (65) to predict LNM in cervical cancer. In addition, Liu et al. developed a CT-based radiomics model using an artificial neural network to predict normal-sized LNM in cervical cancer. The developed model exhibited better performance, with AUCs of 0.912, 0.859, and 0.800 in the training, internal validation, and external validation cohorts, respectively (58). Monthatip et al. showcased the performance of an ML model based on clinical and CT radiomics data to increase the accuracy in predicting pelvic LNM (63). In addition to MRI or CT radiomics, PET/CT radiomics was used by Zhang et al. to develop an ML model for predicting pelvic LNM (AUCs of 0.817 and 0.786 in the training and testing sets, respectively) (59). Notably, Lucia et al. combined clinical, PET/CT, and MRI radiomics data to develop an ML model (ComBat-combined model) for para-aortic LNM prediction (62).
Endometrial cancer
Two studies used AI radiomics for LNM prediction in endometrial cancer. In a prospective observational study, Yang et al. developed an ML model based on clinical and MRI-based radiomics, the RadSignature, that yielded an excellent predictive performance (AUC: 0.85) (68). Besides, the nomogram based on ultrasound radiomics and clinical features developed by Liu et al. provided good predictive performance (AUCs of 0.919 and 0.884 in the training and testing sets, respectively) (69).
Ovarian cancer
The only study by Yao et al. developed a residual neural network based on PET/CT to predict LNM in patients with primary ovarian cancer. The developed model could effectively predict LNM (AUCs of 0.947 and 0.926 in the training and testing sets, respectively) (70).
LVSI
We identified five studies using AI radiomics for LVSI prediction; all were retrospective and based on MRI radiomics data (71-75) (Table 3). Three studies were conducted on cervical cancer and two were on endometrial cancer.
Cervical cancer
Huang et al. developed a multi-parametric MRI-combined radiomics model achieving a significantly better performance than the clinical model or any single-layer model (AUC: 0.940 vs. 0.730 vs. 0.650–0.840) (71). Wu et al. developed a nomogram by integrating radiomics and clinical features, achieving high predictive performance (AUCs of 0.883 and 0.830 in the training and external test cohorts, respectively) (73). Additionally, Wang et al. developed a habitat-based MRI radiomics model for LVSI prediction (72).
Endometrial cancer
Luo et al. developed a predictive nomogram incorporating multiparametric MRI radiomics and clinical features. The nomogram achieved an AUC of 0.820 and 0.807 in the training and test cohorts, respectively (74). Yan et al. used multiparametric MRI radiomic features from intratumoral and peritumoral regions for LVSI prediction in endometrial adenocarcinoma, resulting in AUCs of 0.962 and 0.965 of the nomogram integrating clinical-tumor morphological features and radiomics in the training and validation cohorts, respectively (75).
MI
Six studies were identified to use AI radiomics for MI prediction in endometrial cancer; all used MRI-based radiomics data (76-81) (Table 3). Chen et al. conducted one of the largest studies on 530 patients. Using T2-weighted imaging-based MRI data, a DL model was developed and showed a competitive and time-efficient performance in determining deep MI compared to the radiologists (accuracy: 84.8% vs. 78.3%) (76). Lefebvre et al. introduced spherical harmonics (SPHARM) into an ML pipeline to develop an image descriptor based on multiparametric MRI for predicting deep MI and high-grade histology. The SPHARM descriptor yielded similar or even higher performance than radiomics (AUC: 0.94 vs. 0.92 for predicting deep MI; 0.81 vs. 0.72 for high-grade histology) (81).
Moreover, several studies have used AI radiomics for risk classification in other scenarios, such as cervical stromal invasion (82,83) and tumor budding (84,85) in cervical cancer. Finally, in addition to the studies focusing on specific risk factors, several reviewed studies used AI radiomics for risk classification of multiple factors (86-94) (Table 3). In particular, Wang et al. developed a multi-task DL model to predict LNM and LVSI based on MRI (94).
Other molecular or clinicopathological factors
AI radiomics has also been introduced to predict other molecular or clinicopathological factors. A total of 10 studies were identified in endometrial or ovarian cancer (95-104) (Table 4). Li et al. incorporated MRI-based radiomics and clinicopathological features to develop an ML model for predicting microsatellite instability (MSI) and programmed cell death ligand 1 (PD-L1) expression status. The combined model achieved AUCs of 0.848 and 0.764 for predicting MSI and PD-L1 status in the validation set, respectively (101). Jia et al. developed multiparametric MRI radiomics models using five ML methods [logistic regression (LR), k-nearest neighbors (KNN), Naive Bayes (NB), support vector machine (SVM), and random forest (RF)]. The SVM model outperformed the others and showed the best performance (AUC: 0.905, 0.875, and 0.862 in the training, internal, and external validation cohorts, respectively) (102). Wang et al. explored the efficacy of DL models based on diffusion-weighted imaging for MSI prediction. The AUCs of 0.989 and 0.885 were achieved in the optimal model (103). Other molecular phenotypes have been explored with AI radiomics, for example, hypoxia patterns and immunological characteristics (95), human papillomavirus status (97), tissue heterogeneity (98), breast cancer susceptibility gene (BRCA) or tumor protein 53 (TP53) mutations (96,104), and C-C motif chemokine receptor type 5 (CCR5) or lymphocyte cell-specific protein-tyrosine kinase (LCK) expression (99,100).
Table 4
Author, year | Study | Patients [n] | Utility | Imaging | Methods | Validation [n] | Results |
---|---|---|---|---|---|---|---|
Feng et al. 2022 (95) | NA | OC (TCGA-OV/GSE32062: 634) | Hypoxia patterns & immunological characteristics | CECT | Machine learning | External validation: (GSE32062, 260) | AUC: 0.900 and 0.703 (training and validation, respectively) |
Avesani et al. 2022 (96) | Multi-center retrospective | HGSOC [218] | BRCA mutation & early relapse | CT | CNN | Five-fold cross-validation; External validation [66] | AUC: 0.48 for BRCA and 0.50 for relapse (deep learning models); 0.74 for BRCA (clinical-radiomics model) |
İnce et al. 2023 (97) | Single-center retrospective | OC [41] | HPV | MRI | Machine learning | Five-fold cross-validation | AUC: 0.93 and 0.98 for LR and SVM-combined models |
Binas et al. 2023 (98) | Single-center retrospective | HGEOC [20] | Tissue heterogeneity | MRI | Machine learning | Ten-fold cross-validation | The average accuracy: 0.86 |
Wan et al. 2023 (99) | Database | OC (TCGA/TCIA: 343) | CCR5 expression | CT | Machine learning | Ten‑fold cross‑validation | AUC: 0.770 and 0.726 in the training and validation cohorts, respectively |
Zhan et al. 2023 (100) | Database | OC (TCGA: 343) | LCK expression | CT | Machine learning | Five-fold cross-validation | AUC: 0.879 and 0.834 (training and validation, respectively) |
Li et al. 2023 (101) | Two-center retrospective | EC [82] | MSI/PD-L1 status | MRI | Machine learning | External validation [22] | AUC: 0.595-0.848 for predicting MSI; and 0.660–0.764 for predicting PD-L1 expression |
Jia et al. 2024 (102) | Two-center retrospective | EC [225] | MSI status | MRI | Machine learning | Internal testing [67]; external validation [132] | AUC: 0.905, 0.875, and 0.862 (training, internal, and external validation, respectively) |
Wang et al. 2024 (103) | Single-center retrospective | EC [116] | MSI status | MRI | Deep learning | Internal validation [35] | AUC: 0.989 and 0.885 (training and validation, respectively) |
Ning et al. 2024 (104) | Single-center retrospective | EC [227] | TP53 mutation | MRI | Machine learning | Internal validation [65] | AUC: 0.845 (validation) |
AI, artificial intelligence; AUC, area under the curve; CECT, contrast-enhanced CT; CNN, convolutional neural network; CT, computed tomography; EC, endometrial cancer; HGEOC, high-grade epithelial ovarian cancer; HGSOC, high-grade serous ovarian cancer; HPV, human papilloma virus; MRI, magnetic resonance imaging; MSI, microsatellite instability; NA, not available; OC, ovarian cancer; PD-L1, programmed cell death ligand 1; TCGA, The Cancer Genome Atlas.
To sum up, numerous studies have focused on AI radiomics in the diagnosis and characterization of gynecological cancer. However, most of the studies are retrospective and involve only one or a few centers. Various AI radiomics-based models are available, with substantial heterogeneity in study methodology and results reporting. Prospective large-scale studies are required to validate the reliability and applicability of the methods reported using a rigorous evaluation system.
Treatment response
Chemotherapy response
Four studies used AI radiomics based on CT or MRI to predict response to neoadjuvant chemotherapy (neo-CT) in cervical or ovarian cancer (Table 5) (105-108).
Table 5
Author, year | Study | Patients [n] | Therapy | Utility | Imaging | Methods | Validation [n] | Results |
---|---|---|---|---|---|---|---|---|
Chiappa et al. 2023 (105) | Single-center retrospective | CC [72] | Neo-CT | Response | MRI | Machine learning | Nested six-fold cross validation | AUC: 0.823 |
Zhang et al. 2023 (106) | Two-center retrospective | CC [285] | Neo-CT | Response | MRI | Deep learning | Internal validation [67]; external validation [60] | AUC: 0.940 and 0.910 (internal and external validation, respectively) |
Crispin-Ortuzar et al. 2023 (107) | Multicenter retrospective* | HGSOC [134] | Neo-CT | Response | CT | Machine learning | Internal validation (hold-out, 20); external validation [42] | AUC: 0.78 (External validation) |
Yin et al. 2023 (108) | Multicenter retrospective & prospective | OC [757] | Neo-CT | Response | CECT | Deep learning | Prospective validation [67]; external validation [103] | AUC: 0.86 (prospective validation) and 0.79 (external validation) |
Yang et al. 2023 (109) | Single-center retrospective | CC [138] | Neo-CRT | Response | MRI | Deep learning | Five-fold cross-validation | AUC: 0.797 |
Cai et al. 2024 (110) | Single-center retrospective | CC [360] | Neo-CRT | Response | MRI | Deep learning | Internal validation [142] | AUC: 0.86 |
Jeong et al. 2024 (111) | Single-center retrospective | CC [252] | Neo-CRT | Response | MRI | Deep learning | Internal validation [85] | AUC: 0.782 |
Wu et al. 2023 (112) | Single-center retrospective | CC [157] | Neo-CRT | Response | MRI | Random forest | Internal validation [31] | AUC: 0.91 (training) and 0.89 (validation) |
Fang et al. 2020 (113) | Single-center retrospective | CC [120] | CCRT | Response | MRI | Deep learning | Multiple three-fold cross-validation | AUC: 0.820 (training) and 0.798 (validation) |
Veeraraghavan et al. 2020 (114) | Multi-center retrospective | HGSOC [75] | Platinum | Resistance | CT | Machine learning | K-fold cross validation | AUC: 0.78 |
Yi et al. 2021 (115) | Single-center retrospective | OC [102] | Platinum | Resistance | CT | Machine learning | Internal validation [31] | AUC: 0.993 (training) and 0.967 (validation) |
Lei et al. 2022 (116) | Single-center retrospective | EOC [93] | Platinum | Sensitivity | MRI | CNN | Internal validation [31] | AUC: 0.97 (training) and 0.98 (validation) |
Na et al. 2024 (117) | Single-center retrospective | HGSOC [96] | Platinum | Sensitivity | MRI | Machine learning | Five-fold cross-validation | AUC: 0.77 (validation) |
Bi et al. 2024 (118) | Multicenter retrospective | HGSOC [394] | Platinum | Resistance | MRI | Deep learning | External validation [87] | AUC: 0.932 (training) and 0.721 (validation) |
Zhuang et al. 2024 (119) | Single-center retrospective | HGSOC [289] | Platinum | Resistance | PET/CT | Deep learning | Five-fold cross-validation | AUC: 0.93 (validation) |
*, data from two prospective studies. AI, artificial intelligence; AUC, area under the curve; CC, cervical cancer; CECT, contrast-enhanced CT; CNN, convolutional neural network; CT, computed tomography; HGSOC, high grade serous ovarian cancer; MRI, magnetic resonance imaging; Neo-CT, neoadjuvant chemotherapy; Neo-CRT, neoadjuvant chemoradiotherapy; OC, ovarian cancer; PET/CT, positron emission tomography/computed tomography; RNN, residual neural network; SVM, support vector machine; US, ultrasonography.
Cervical cancer
Chiappa et al. used MRI radiomics and ML to predict response to neo-CT in locally advanced cervical cancer (LACC). The findings of their study support the adoption of an AI radiomic-based approach to predict the neo-CT response (105). Additionally, Zhang et al. constructed a multiparametric MRI-based DL radiomics nomogram in LACC. The nomogram was demonstrated to perform well in predicting the neo-CT response and could possibly aid in individualized treatment (106).
Ovarian cancer
Crispin-Ortuzar et al. integrated CT radiomics data to baseline clinical and blood data and showed a reduced response prediction error by 8% to neo-CT in patients with high-grade serous ovarian carcinoma (HGSOC) (107). Yin et al. developed a multitask DL model based on contrast-enhanced CT images and reported promising performances in Neo-CT response prediction and HGSOC classification (108).
Chemoradiotherapy response
Five studies used MRI radiomics to predict responses to neoadjuvant (neo) or concurrent chemoradiotherapy (CRT) in cervical cancer (109-113).
Cervical cancer
Fang et al. developed a radiomics model based on multiparametric MRI for predicting response to concurrent CRT in LACC. The multi-habitat model showed good predictive performance in the training and validation cohorts, with AUCs of 0.820 and 0.798, respectively, suggesting the ability of the radiomics model in treatment response prediction in LACC before concurrent CRT (113). Meanwhile, the studies by Yang et al. and Cai et al. developed and validated MRI-based DL models for predicting neo-CRT response in LACC (109,110). Furthermore, Jeong et al. compared DL and handcrafted radiomics models for predicting neo-CRT response in LACC. The results showed that both DL and handcrafted radiomics could predict neo-CRT responses (111).
Platinum resistance/sensitivity in ovarian cancer
Six studies used AI radiomics to predict platinum resistance/sensitivity in patients with ovarian cancer (114-119) (Table 5). Yi et al. developed an ML model by incorporating genomic data (SULF1 polymorphisms) with a CT radiomics model that could potentially predict platinum resistance in ovarian cancer (AUCs of 0.99 and 0.97 in the training and validation cohorts, respectively) (115). Lei et al. developed MRI-based DL models using the primary tumor or the whole abdomen as the volume of interest to predict platinum sensitivity in epithelial ovarian cancer. The whole-abdomen DL model showed satisfactory performance (AUCs of 0.97 and 0.98 in the training and validation cohorts, respectively) and might assist in optimal treatment-making (116). Na et al. established a radiomics framework by combining MRI radiomics and clinical data. The combined model showed the incremental benefits in predicting platinum sensitivity in advanced ovarian cancer (117). Additionally, Zhuang et al. developed a DL model based on multimodal PET/CT images to discriminate platinum resistance from platinum sensitivity, with an AUC of 0.93 using a five-fold cross-validation (119).
Available data showed the predictive value of AI radiomics in treatment response in patients with gynecological cancer. However, similar to the research on tumor diagnosis, the published studies are mainly exploratory, with a retrospective nature of study design and limited sample size without extensive external validation, as well as heterogeneous methodology. Besides, most of the studies did not include any potential underlying molecular mechanisms.
Recurrence and survival
Among 27 studies using AI radiomics for prognosis analysis, 15 (55.6%) were on cervical cancer, eight (29.6%) on ovarian cancer, and four (14.8%) on endometrial cancer (Table 6).
Table 6
Author, year | Study | Patients [n] | Treatment | Utility | Imaging | Methods | Validation [n] | Results |
---|---|---|---|---|---|---|---|---|
Shen et al. 2019 (120) | Single-center retrospective | CC [142] | CCRT | Local relapse & distant metastasis | PET/CT | Deep learning | K-fold cross-validation | The sensitivity, specificity, and accuracy: 71%, 93%, and 89% for local recurrence; and 77%, 90%, and 87% for distant metastasis, respectively |
Zhou et al. 2020 (121) | Single-center retrospective | CC [75] | Definitive CRT | locoregional & distant failure | PET | Multi-Objective SVM | Five-fold cross validation | The clinical-imaging model: AUC of 0.84 and 0.75 to predict locoregional and distant failure |
Takada et al. 2020 (122) | Single-center retrospective | CC [87] | Definitive RT | In-field tumor recurrence | MRI | Machine learning | Nested cross-validation | AUC: 0.82, 0.82, and 0.86 for the models (VOI+4 mm in T2WI and VOI+4 mm and VOI+8 mm in ADC) |
Jajodia et al. 2021 (123) | Single-center retrospective | CC [52] | Upfront CRT | Disease recurrence & metastasis | MRI | Machine Learning | Leave-out-one cross-validation | AUC: 0.80 for predicting recurrence; 0.84 for predicting metastasis |
Nakajo et al. 2022 (124) | Single-center retrospective | CC [50] | Surgery ± CRT/CT | Disease progression | PET/CT | Machine learning | Ten-fold cross-validation | Naive Bayes model: AUC of 0.872; an independent factor for PFS (HR, 6.98; 95% CI: 1.92–24.69; P=0.003) |
Kawahara et al. 2022 (125) | Single-center retrospective | ESCCC [89] | RT | Recurrence | MRI | machine learning | Internal validation [26] | AUC: 0.89, 0.69, and 0.94 in T1- and T2-weighted MRI models and their combined one |
Wang et al. 2023 (126) | Single-center retrospective | CC [104] | RT | Recurrence | CT, MRI | Deep learning | Internal validation [21] and testing [35] | AUC: 0.819 (testing) vs. 0.680–0.777 (four conventional radiomics methods) and 0.733/0.743 (two deep learning methods) |
Wu et al. 2024 (127) | Single-center retrospective | CC [211] | CRT | Distant metastasis | PET/CT | Deep learning | Internal validation [25] | AUC: 0.818 and 0.830 (training and validation) |
Ai et al. 2024 (128) | Multicenter retrospective | CC [212] | Surgery + AT | Recurrence | MRI | Machine learning | Internal validation [54]; external validation [35] | AUC: 0.806, 0.718 (internal and external validation) |
Zhang et al. 2024 (129) | Two-center retrospective | CC [225] | Surgery | Recurrence | MRI | Deep learning | Internal validation [70]; external validation [40] | AUC: 0.944 and 0.885 (internal and external validation) |
Lin et al. 2023 (130) | Multi-center retrospective | EC [421] | Surgery | Recurrence | MRI | Machine learning | Internal validation [102]; external validation [84] | AUC: 0.89, 0.87, and 0.85 (training, internal validation, and external validation) |
Wang et al. 2019 (131) | Multi-center retrospective | HGSOC [245] | Surgery | Recurrence | CT | Deep learning | Two independent validation [49 & 45] | C-index: 0.713 and 0.694 |
Li et al. 2021 (132) | Single-center retrospective | HGSOC [117] | Surgery | Recurrence | MRI | SVM | Leave-one-out cross-validation | AUC: 0.85 |
Liu et al. 2023 (133) | Single-center retrospective | HGSOC [185] | Surgery | Recurrence | MRI | deep learning | Two internal validation [56 & 37] | AUC: 0.986 and 0.961 (validation cohorts 1 or 2) |
Wei et al. 2024 (134) | Multi-center retrospective | EOC [478] | Cytoreductive surgery | Peritoneal Metastasis | T2-W MRI | Deep learning | Internal validation [75]; external validation [53] | AUC: 0.844, 0.859, and 0.872 in the validation and external validation sets. |
Yin et al. 2024 (135) | Multi-center retrospective | OC [515] | Surgery | Peritoneal recurrence & DFS | CECT | Multi-task CNN | Internal validation [127]; External validation [92] | AUC: 0.87, 0.88, and 0.82 for peritoneal recurrence; and 0.85, 0.83, and 0.85 for recurrence (training, internal and external validation) |
Ferreira et al. 2021 (136) | Multi-center retrospective | CC [158] | CRT | DFS | PET/CT | Machine learning | Internal validation (five-fold cross-validation); external validation [18] | The best model: AUC: 0.78 |
Xu et al. 2023 (137) | Single-center retrospective | CC [367] | CCRT | OS | CECT | Deep learning | Internal validation [104] | AUC: 0.833, 0.777, and 0.871 for 1-, 3-, and 5-year OS (training), and 0.811, 0.713, and 0.730 (validation) |
Liu et al. 2024 (138) | Multi-center retrospective | CC [190] | CCRT | PFS | PET/CT | Machine learning | Internal validation [50]; external validation [23] | AUC: 0.661, 0.711, and 0.767 for 5-year PFS (training, internal validation, and external validation) |
Xin et al. 2024 (139) | Multicenter retrospective | CC [700] | CCRT | DFS & OS | MRI | Machine learning | Internal validation [190]; external validation [130] | AUC: 0.829, 0.809, 0.841 for predicting 1-, 3-, and 5-year DFS (RSF-based model); and 0.904, 0.860, and 0.905 for OS (GBM model) |
Zhu et al. 2024 (140) | Single-center retrospective | CC [406] | Surgery | OS | MRI | Deep learning | Internal validation [81] and testing [82] | C-index: 0.732 |
Nakajo et al. 2021 (141) | Single-center retrospective | EC [53] | Surgery | PFS/OS | PET/CT | Machine learning | Ten-fold cross-validation | AUC: 0.890 of the kNN model; and 0.876 of the RF model |
Hoivik et al. 2021 (142) | Multi-center retrospective | EC [487] | Surgery | DFS | MRI | Machine learning | External validation [554] | Three clusters: 1, 2a, and 2b |
Coada et al. 2023 (143) | Single-center retrospective | EC [81] | Surgery | DFS/RFS | CECT | Machine learning | Internal validation [32] | AUC: 0.92 to 0.93 (training) and 0.86 to 0.90 (validation) |
Lu et al. 2019 (144) | Multicenter retrospective | EOC [364] | Surgery | OS & MP | CT | Machine learning | Internal validation [77]; external validation (TCGA, 70) | C-index: 0.658 to 0.739 (training); 0.659 to 0.679 (internal validation); 0.549 to 0.690 (external validation) |
Boehm et al. 2022 (145) | Two-center retrospective | HGSOC [444] | Surgery ± neo-CT | OS | CECT; H&E | Machine learning | Internal validation [40] | Median OS: 30 and 50 months in the high and low risk groups, respectively |
Zheng et al. 2022 (146) | Single-center retrospective | HGSOC [734] | Surgery | OS | CT | Deep learning | Internal validation [184] | AUC: 0.822 and 0.823 (training and validation, respectively) |
AI, artificial intelligence; AT, adjuvant therapy; AUC, area under the curve; CC, cervical cancer; CECT, contrast-enhanced CT; C-index, concordance index; CNN, convolutional neural network; CRT, chemoradiotherapy; CT, chemotherapy; CT, computed tomography; DFS, disease-free survival; EC, endometrial cancer; EOC, epithelial ovarian cancer; ESCCC, cervical squamous cell carcinoma cancer; HGSOC, high-grade serous ovarian cancer; MP, molecular phenotypes; MRI, magnetic resonance imaging; Neo-CT, neoadjuvant chemotherapy; Neo-CRT, neoadjuvant chemoradiotherapy; OC, ovarian cancer; OS, overall survival; PET/CT, positron emission tomography/computed tomography; PFS, progression-free survival; RNN, residual neural network; RT, radiotherapy; SVM, support vector machine; T2W-MRI, T2-weighted MRI; US, ultrasonography.
Local recurrence and/or distant metastasis
Cervical cancer
A total of 10 studies used AI radiomics to predict local recurrence or distant metastasis in cervical cancer (120-129). Zhou et al. used PET imaging and clinical features to construct a multi-objective SVM learning model for predicting locoregional and distant failure in patients receiving definitive chemoradiation. The clinical-imaging combined model achieved an AUC of 0.84 for locoregional failure and 0.75 for distant failure (121). Nakajo et al. compared six ML methods (RF, KNN, neural network, LR, NB, and SVM) in predicting disease progression following surgery and/or CRT or chemotherapy. The NB model showed the best performance with an AUC of 0.872. The 5-year progression-free survival (PFS) was 80.1% and 9.1% in patients with NB model-predicted non-progression and those with predicted progression, respectively (124). Similarly, Ai et al. used four ML methods [SVM, LR, the least absolute shrinkage and selection operator (LASSO), and extreme gradient boosting (XGboost)] to build MRI radiomics models for recurrence risk prediction. Postoperative adjuvant treatments were integrated in their study to improve the prediction performance in 212 patients who underwent surgery and adjuvant therapy (128). As for the DL approach, Shen et al. performed the first study using a DL model for assessing PET/CT in predicting local relapse and distant metastasis following definitive CRT in 2019. Their study showed the capability of the PET/CT-based DL model in treatment outcome prediction (120). Recently, Wu et al. developed a PET/CT-based DL model for early prediction of distant metastasis. The only PET/CT-based study included more than 100 patients (127).
In addition, MRI-based radiomics data were also used to address the problem of how to predict disease recurrence. Three studies used ML approaches based on MRI and showed potential for predicting disease recurrence (122,123,125). However, the number of patients included was small (<100) without external validation. A recent study by Zhang et al. included 225 patients from two centers to construct and validate a DL radiomics nomogram based on MRI and clinical features for recurrence prediction. The nomogram demonstrated satisfactory predictive performance in the internal and external validation cohorts (AUCs of 0.944 and 0.885, respectively) (129). Finally, Wang et al. investigated the capability of transformer networks based on CT and MRI in recurrence risk stratification (126). The multi-modality transformer network showed promising performance, outperforming that of the four conventional radiomics models (decision tree, NB, KNN, and SVM) and two DL networks (ResNet18 and MobileNetV1) in the testing cohort (0.819 vs. 0.680–0.777 vs. 0.733/0.743).
Endometrial cancer
The only study focused on recurrence risk prediction in endometrial cancer was a multicenter study performed by Lin et al. (130). The fusion model based on clinicopathological and MRI radiomics features showed better performance when compared with the clinicopathological or radiomics features-based models (AUC: 0.87 vs. 0.78 vs. 0.80 in the internal validation cohort; 0.85 vs. 0.75 vs. 0.78 in the external validation cohort). The predictive performance was found to decrease with the expansion of the peritumoral region, although higher AUCs were observed in all fusion models.
Ovarian cancer
Five studies investigated postoperative recurrence or metastasis using CT or MRI-based radiomics in ovarian cancer (131-135). Wang et al. proposed a CT-based DL method to extract prognostic biomarkers for recurrence prediction in patients with HGSOC (131). Yin et al. developed a multitask DL model for predicting peritoneal recurrence and disease-free survival (DFS) in advanced ovarian cancer using preoperative contrast-enhanced CT. The multitask CNN could predict peritoneal and overall recurrence, with AUCs of 0.88 and 0.83 in the internal validation cohort and 0.82 and 0.85 in the external validation cohort (135). Three studies used MRI-based radiomics for recurrence or metastasis prediction. For example, Wei et al. investigated the performance of a T2-weighted MRI radiomics model for predicting peritoneal metastasis using a DL method. An ensemble model combining DL, radiomics, and clinical models was constructed and outperformed their respective ones (AUC: 0.844 vs. 0.737 vs. 0.730 in the external validation set). The performance of the radiologists, especially those less experienced, was significantly improved with the assistance of the model (134).
Survival
Among the 11 studies on survival outcomes, five were conducted on cervical cancer (136-140), three were on endometrial cancer (141-143), and the remaining three were on ovarian cancer (144-146).
Cervical cancer
Two studies reported MRI-based radiomics for survival prediction in cervical cancer. Xin et al. compared six ML methods [LASSO, random survival forest (RSF), gradient boosting machine (GBM), XGboost, linear kernel SVM (SVM-lin), and polynomial kernel SVM (SVM-add)] for predicting DFS and overall survival (OS) after concurrent CRT. The RSF model combining tumor and peritumor radiomics showed the best performance for DFS prediction, whereas the GBM model performed best for OS prediction. The potential mechanisms of radiomics in progression and survival prediction were explored in The Cancer Genome Atlas (TCGA) and Gene Expression Omnibus (GEO) databases and suggested to be associated with Lys-Asp-Glu-Leu receptor 2 (KDELR2) and hexokinase 2 (HK2) (139). Two studies used ML methods based on PET/CT to predict survival. Ferreira et al. showed the added information of PET-CT-based ML models to the clinical data in terms of DFS, yet the data varied across the devices (136). By contrast, Liu et al. demonstrated the nomogram incorporating PET/CT radiomics and clinical features as a useful clinical tool for predicting PFS in LACC patients undergoing concurrent CRT (138). Additionally, Xu et al. established and validated a CT-based hybrid radiomics model to predict OS after concurrent CRT exhibiting favorable performance (AUC: 0.811, 0.713, and 0.730 for 1-, 3-, and 5-year OS in the test set, respectively) (137).
Endometrial cancer
All three studies used ML methods for postoperative progression or survival prediction in patients with endometrial cancer. As an example, Hoivik et al. integrated preoperative MRI with histologic-, transcriptomic-, and molecular biomarkers to develop a novel radiogenomics approach for prognostic profiling of endometrial cancer. An 11-gene high-risk signature was defined and showed prognostic role in orthologous validation cohorts (n=554), aligning with TCGA molecular class with poor survival (copy-number high/p53-altered) (142).
Ovarian cancer
Three studies used CT-based AI radiomics for postoperative survival prediction in ovarian cancer. Lu et al. extracted 657 mathematical descriptors from pretreatment CT images to discover a novel radiomics-based prognostic signature for prognostic and molecular phenotyping. By using ML, a novel non-invasive model based on four descriptors, radiomic prognostic vector (RPV), was obtained and showed reliable performance in identifying patients with a median OS of less than two years. The underlying mechanisms were explored by genetic, transcriptomic, and proteomic analyses using two independent datasets (144). Moreover, Boehm et al. integrated multi-modal data from hematoxylin and eosin staining and contrast-enhanced CT using ML to improve prognosis risk stratification of HGSOC. Histopathological and radiologic features were found to provide complementary prognostic information to one another and clinicogenomic features. By integrating these features, the authors demonstrated a promising path for risk stratification of cancer patients (145).
AI radiomics methods exhibited encouraging results in prognosis prediction of gynecological cancer. However, substantial heterogeneity in methodology and results existed and hampered the clinical application of models. Further validation in multicenter large datasets or randomized trials based on standardized protocol and transparent reporting guidelines is indispensable before translating AI radiomic analysis into clinical utility.
Discussion
Recent years have witnessed the rapid growth of AI radiomics use in gynecological cancer. Numerous studies have demonstrated the potential of AI radiomics in helping clinicians make precision diagnoses, support treatment decision-making, and evaluate the prognosis of patients. Despite the encouraging results reported, there are still many challenges to be solved.
Data scarcity and potential biases
Large high-quality data from across populations are essential for the development of AI-based methodology. However, obtaining such data can be difficult due to high costs, privacy concerns, and data-sharing regulations. Currently available research on AI radiomics in gynecological cancer is mainly exploratory and preliminary based on limited data from single- or few-center retrospective studies. Although external validation was reported in some studies, it was mainly performed on data from one or a few other centers. Although easier to collect, a small dataset may lead to potential biases, a high risk of overfitting, and therefore poor reliability and generalizability of such models. Further validations in large-scale datasets are warranted to evaluate the actual performance of models. This challenge calls for obtaining and sharing robust medical data according to the necessary rules governing medical data privacy [e.g., the Health Insurance Portability and Accountability Act (HIPAA)] (147).
Heterogeneity and reproducibility
Numerous AI radiomics-based models are available for given tasks in gynecological cancer. However, in addition to different data sources, different protocols in research conduction and heterogeneity in results reporting may also affect the reproducibility of results. Some studies still lack clear documentation on validation cohort selection and methodological development. The recently updated CLAIM checklist may offer guidance for improving transparent reporting of AI in medical imaging (148,149). Besides, guidelines such as SPIRIT-AI (150) and CONSORT-AI (151) now include AI-specific recommendations for clinical trial protocols and reporting. As the field evolves, following these guidelines is mandatory for future clinical trials.
Interpretability and explainability
One major hurdle for AI radiomics applications is the lack of trust by physicians and patients in a somewhat “black box” decision-making process. Most studies included in this review did not provide any interpretation or explanation of the models and predictions. Several breakthroughs have been made in visualizing the process and providing insights into underlying decision-making mechanisms. For instance, explainable AI (xAI) is an emerging field that helps physicians to gain mechanistic insights into the complex decision-making process (152). In addition, xAI enables us to enhance biological discovery, for example, revealing underlying molecular mechanisms.
Study limitations
This review has some methodological limitations that may have led to bias. Primarily, due to the considerable heterogeneity among the included studies, we did not compare model performance across studies. However, this is compensated by a narrative synthesis that illustrates common pitfalls and inconsistency of the studies. Some included studies developed multiple models based on multimodal data from different sources separately and together or multiple methods. Therefore, we could only present the summarized performance of a multi-modality fusion model or the one that had the best performance in the tables. In addition, some relevant studies published after the time frame of publication search were not included in the review.
Conclusions
In this review, we confirmed an increasing application of AI radiomics in the management of gynecological cancer. AI radiomics has shown potential value in assisting clinicians with tumor diagnosis and treatment decision-making. Despite the significant advances, however, the development of AI radiomics is still hindered by retrospective study design using data mainly from a single center, diverse methodologies, and opaque decision-making. More compelling evidence is required to develop a reliable and interpretable AI-radiomics tool for managing gynecological cancer.
Acknowledgments
None.
Footnote
Reporting Checklist: The authors have completed the Narrative Review reporting checklist. Available at https://tcr.amegroups.com/article/view/10.21037/tcr-2025-618/rc
Peer Review File: Available at https://tcr.amegroups.com/article/view/10.21037/tcr-2025-618/prf
Funding: None.
Conflicts of Interest: All authors have completed the ICMJE uniform disclosure form (available at https://tcr.amegroups.com/article/view/10.21037/tcr-2025-618/coif). S.H reports that he is an employee of Jiangsu Hengrui Pharmaceuticals Co., Ltd. The other authors have no conflicts of interest to declare.
Ethical Statement: The authors are accountable for all aspects of the work in ensuring that questions related to the accuracy or integrity of any part of the work are appropriately investigated and resolved.
Open Access Statement: This is an Open Access article distributed in accordance with the Creative Commons Attribution-NonCommercial-NoDerivs 4.0 International License (CC BY-NC-ND 4.0), which permits the non-commercial replication and distribution of the article with the strict proviso that no changes or edits are made and the original work is properly cited (including links to both the formal publication through the relevant DOI and the license). See: https://creativecommons.org/licenses/by-nc-nd/4.0/.
References
- Zhu B, Gu H, Mao Z, et al. Global burden of gynaecological cancers in 2022 and projections to 2050. J Glob Health 2024;14:04155. [Crossref] [PubMed]
- Bray F, Laversanne M, Sung H, et al. Global cancer statistics 2022: GLOBOCAN estimates of incidence and mortality worldwide for 36 cancers in 185 countries. CA Cancer J Clin 2024;74:229-63. [Crossref] [PubMed]
- Besharat AR, Giannini A, Caserta D. Pathogenesis and Treatments of Endometrial Carcinoma. CEOG. 2023;50(11).
- Zhang C, Sheng Y, Sun X, et al. New insights for gynecological cancer therapies: from molecular mechanisms and clinical evidence to future directions. Cancer Metastasis Rev 2023;42:891-925. [Crossref] [PubMed]
- Tan N, Wu Y, Li B, et al. Burden of cancers in six female organs in China and worldwide. Chin Med J (Engl) 2024;137:2190-201. [Crossref] [PubMed]
- Liang Y, Dai X, Chen J, et al. Global burden and trends in pre- and post-menopausal gynecological cancer from 1990 to 2019, with Projections to 2040: a cross-sectional study. Int J Surg 2025;111:891-903. [Crossref] [PubMed]
- Liu Z, Wang S, Dong D, et al. The Applications of Radiomics in Precision Diagnosis and Treatment of Oncology: Opportunities and Challenges. Theranostics 2019;9:1303-22. [Crossref] [PubMed]
- Horvat N, Papanikolaou N, Koh DM. Radiomics Beyond the Hype: A Critical Evaluation Toward Oncologic Clinical Use. Radiol Artif Intell 2024;6:e230437. [Crossref] [PubMed]
- Prelaj A, Miskovic V, Zanitti M, et al. Artificial intelligence for predictive biomarker discovery in immuno-oncology: a systematic review. Ann Oncol 2024;35:29-65. [Crossref] [PubMed]
- Lotter W, Hassett MJ, Schultz N, et al. Artificial Intelligence in Oncology: Current Landscape, Challenges, and Future Directions. Cancer Discov 2024;14:711-26. [Crossref] [PubMed]
- Chen M, Copley SJ, Viola P, et al. Radiomics and artificial intelligence for precision medicine in lung cancer treatment. Semin Cancer Biol 2023;93:97-113. [Crossref] [PubMed]
- Bera K, Braman N, Gupta A, et al. Predicting cancer outcomes with radiomics and artificial intelligence in radiology. Nat Rev Clin Oncol 2022;19:132-46. [Crossref] [PubMed]
- Shrestha P, Poudyal B, Yadollahi S, et al. A systematic review on the use of artificial intelligence in gynecologic imaging - Background, state of the art, and future directions. Gynecol Oncol 2022;166:596-605. [Crossref] [PubMed]
- Moro F, Ciancia M, Zace D, et al. Role of artificial intelligence applied to ultrasound in gynecology oncology: A systematic review. Int J Cancer 2024;155:1832-45. [Crossref] [PubMed]
- Leo E, Stanzione A, Miele M, et al. Artificial Intelligence and Radiomics for Endometrial Cancer MRI: Exploring the Whats, Whys and Hows. J Clin Med 2023;13:226. [Crossref] [PubMed]
- Di Donato V, Kontopantelis E, Cuccu I, et al. Magnetic resonance imaging-radiomics in endometrial cancer: a systematic review and meta-analysis. Int J Gynecol Cancer 2023;33:1070-6. [Crossref] [PubMed]
- Egemen D, Perkins RB, Cheung LC, et al. Artificial intelligence-based image analysis in clinical testing: lessons from cervical cancer screening. J Natl Cancer Inst 2024;116:26-33. [Crossref] [PubMed]
- Sadeghi MH, Sina S, Omidi H, et al. Deep learning in ovarian cancer diagnosis: a comprehensive review of various imaging modalities. Pol J Radiol 2024;89:e30-48. [Crossref] [PubMed]
- Urushibara A, Saida T, Mori K, et al. Diagnosing uterine cervical cancer on a single T2-weighted image: Comparison between deep learning versus radiologists. Eur J Radiol 2021;135:109471. [Crossref] [PubMed]
- Yang X, Gao C, Sun N, et al. An interpretable clinical ultrasound-radiomics combined model for diagnosis of stage I cervical cancer. Front Oncol 2024;14:1353780. [Crossref] [PubMed]
- Li D, Hu R, Li H, et al. Performance of automatic machine learning versus radiologists in the evaluation of endometrium on computed tomography. Abdom Radiol (NY) 2021;46:5316-24. [Crossref] [PubMed]
- Bi Q, Wang Y, Deng Y, et al. Different multiparametric MRI-based radiomics models for differentiating stage IA endometrial cancer from benign endometrial lesions: A multicenter study. Front Oncol 2022;12:939930. [Crossref] [PubMed]
- Urushibara A, Saida T, Mori K, et al. The efficacy of deep learning models in the diagnosis of endometrial cancer using MRI: a comparison with radiologists. BMC Med Imaging 2022;22:80. [Crossref] [PubMed]
- Saida T, Mori K, Hoshiai S, et al. Differentiation of carcinosarcoma from endometrial carcinoma on magnetic resonance imaging using deep learning. Pol J Radiol 2022;87:e521-9. [Crossref] [PubMed]
- Virarkar M, Daoud T, Sun J, et al. MRI Radiomics Data Analysis for Differentiation between Malignant Mixed Müllerian Tumors and Endometrial Carcinoma. Cancers (Basel) 2024;16:2647. [Crossref] [PubMed]
- Capasso I, Cucinella G, Wright DE, et al. Artificial intelligence model for enhancing the accuracy of transvaginal ultrasound in detecting endometrial cancer and endometrial atypical hyperplasia. Int J Gynecol Cancer 2024;34:1547-55. [Crossref] [PubMed]
- Wang R, Cai Y, Lee IK, et al. Evaluation of a convolutional neural network for ovarian tumor differentiation based on magnetic resonance imaging. Eur Radiol 2021;31:4960-71. [Crossref] [PubMed]
- Hu Y, Weng Q, Xia H, et al. A radiomic nomogram based on arterial phase of CT for differential diagnosis of ovarian cancer. Abdom Radiol (NY) 2021;46:2384-92. [Crossref] [PubMed]
- Chiappa V, Bogani G, Interlenghi M, et al. The Adoption of Radiomics and machine learning improves the diagnostic processes of women with Ovarian MAsses (the AROMA pilot study). J Ultrasound 2021;24:429-37. [Crossref] [PubMed]
- Jian J, Xia W, Zhang R, et al. Multiple instance convolutional neural network with modality-based attention and contextual multi-instance learning pooling layer for effective differentiation between borderline and malignant epithelial ovarian tumors. Artif Intell Med 2021;121:102194. [Crossref] [PubMed]
- Liu X, Wang T, Zhang G, et al. Two-dimensional and three-dimensional T2 weighted imaging-based radiomic signatures for the preoperative discrimination of ovarian borderline tumors and malignant tumors. J Ovarian Res 2022;15:22. [Crossref] [PubMed]
- Liu P, Liang X, Liao S, et al. Pattern Classification for Ovarian Tumors by Integration of Radiomics and Deep Learning Features. Curr Med Imaging 2022;18:1486-502. [Crossref] [PubMed]
- Li J, Zhang T, Ma J, et al. Machine-learning-based contrast-enhanced computed tomography radiomic analysis for categorization of ovarian tumors. Front Oncol 2022;12:934735. [Crossref] [PubMed]
- Saida T, Mori K, Hoshiai S, et al. Diagnosing Ovarian Cancer on MRI: A Preliminary Study Comparing Deep Learning and Radiologist Assessments. Cancers (Basel) 2022;14:987. [Crossref] [PubMed]
- Akazawa M, Hashimoto K. Preliminary Results of Deep Learning Approach for Preoperative Diagnosis of Ovarian Cancer Based on Pelvic MRI Scans. Anticancer Res 2023;43:3817-21. [Crossref] [PubMed]
- Feng Y. An integrated machine learning-based model for joint diagnosis of ovarian cancer with multiple test indicators. J Ovarian Res 2024;17:45. [Crossref] [PubMed]
- Du Y, Xiao Y, Guo W, et al. Development and validation of an ultrasound-based deep learning radiomics nomogram for predicting the malignant risk of ovarian tumours. Biomed Eng Online 2024;23:41. [Crossref] [PubMed]
- Rong Q, Wu W, Lu Z, et al. Decision-Level Fusion Classification of Ovarian CT Benign and Malignant Tumors Based on Radiomics and Deep Learning of Dual Views. IEEE Access 2024;12:102381-95.
- Zheng Y, Wang H, Weng T, et al. Application of convolutional neural network for differentiating ovarian thecoma-fibroma and solid ovarian cancer based on MRI. Acta Radiol 2024;65:860-8. [Crossref] [PubMed]
- Chiappa V, Interlenghi M, Bogani G, et al. A decision support system based on radiomics and machine learning to predict the risk of malignancy of ovarian masses from transvaginal ultrasonography and serum CA-125. Eur Radiol Exp 2021;5:28. [Crossref] [PubMed]
- Liu L, Cai W, Tian H, et al. Ultrasound image-based nomogram combining clinical, radiomics, and deep transfer learning features for automatic classification of ovarian masses according to O-RADS. Front Oncol 2024;14:1377489. [Crossref] [PubMed]
- Zhao X, Wang X, Zhang B, et al. Classifying early stages of cervical cancer with MRI-based radiomics. Magn Reson Imaging 2022;89:70-6. [Crossref] [PubMed]
- Cibi A, Rose RJ. Classification of stages in cervical cancer MRI by customized CNN and transfer learning. Cogn Neurodyn 2023;17:1261-9. [Crossref] [PubMed]
- Umutlu L, Nensa F, Demircioglu A, et al. Radiomics Analysis of Multiparametric PET/MRI for N- and M-Staging in Patients with Primary Cervical Cancer. Nuklearmedizin 2024;63:34-42. [Crossref] [PubMed]
- Aouadi S, Torfeh T, Bouhali O, et al. Prediction of cervix cancer stage and grade from diffusion weighted imaging using EfficientNet. Biomed Phys Eng Express 2024; [Crossref] [PubMed]
- Cheng J, Zhao B, Liu Z, et al. DMGM: deformable-mechanism based cervical cancer staging via MRI multi-sequence. Phys Med Biol 2024;69:
10.1088/1361-6560/ad4c50 . doi:10.1088/1361-6560/ad4c50 .10.1088/1361-6560/ad4c50 - Chang R, Li T, Ma X. Application value of artificial intelligence algorithm-based magnetic resonance multi-sequence imaging in staging diagnosis of cervical cancer. Open Life Sci 2024;19:20220733. [Crossref] [PubMed]
- Mao W, Chen C, Gao H, et al. A deep learning-based automatic staging method for early endometrial cancer on MRI images. Front Physiol 2022;13:974245. [Crossref] [PubMed]
- Li J, Liao L, Jia M, et al. Latent relation shared learning for endometrial cancer diagnosis with incomplete multi-modality medical images. iScience 2024;27:110509. [Crossref] [PubMed]
- Sadeghi MH, Sina S, Alavi M, et al. The OCDA-Net: a 3D convolutional neural network-based system for classification and staging of ovarian cancer patients using [18F]FDG PET/CT examinations. Ann Nucl Med 2023;37:645-54. [Crossref] [PubMed]
- Jian J, Li Y, Pickhardt PJ, et al. MR image-based radiomics to differentiate type I and type II epithelial ovarian cancers. Eur Radiol 2021;31:403-10. [Crossref] [PubMed]
- Song H, Bak S, Kim I, et al. An Application of Machine Learning That Uses the Magnetic Resonance Imaging Metric, Mean Apparent Diffusion Coefficient, to Differentiate between the Histological Types of Ovarian Cancer. J Clin Med 2021;11:229. [Crossref] [PubMed]
- Li J, Li X, Ma J, et al. Computed tomography-based radiomics machine learning classifiers to differentiate type I and type II epithelial ovarian cancers. Eur Radiol 2023;33:5193-204. [Crossref] [PubMed]
- Wei M, Feng G, Wang X, et al. Deep Learning Radiomics Nomogram Based on Magnetic Resonance Imaging for Differentiating Type I/II Epithelial Ovarian Cancer. Acad Radiol 2024;31:2391-401. [Crossref] [PubMed]
- Du Y, Wang T, Qu L, et al. Preoperative Molecular Subtype Classification Prediction of Ovarian Cancer Based on Multi-Parametric Magnetic Resonance Imaging Multi-Sequence Feature Fusion Network. Bioengineering (Basel) 2024;11:472. [Crossref] [PubMed]
- Dong T, Yang C, Cui B, et al. Development and Validation of a Deep Learning Radiomics Model Predicting Lymph Node Status in Operable Cervical Cancer. Front Oncol 2020;10:464. [Crossref] [PubMed]
- Wu Q, Wang S, Zhang S, et al. Development of a Deep Learning Model to Identify Lymph Node Metastasis on Magnetic Resonance Imaging in Patients With Cervical Cancer. JAMA Netw Open 2020;3:e2011625. [Crossref] [PubMed]
- Liu Y, Fan H, Dong D, et al. Computed tomography-based radiomic model at node level for the prediction of normal-sized lymph node metastasis in cervical cancer. Transl Oncol 2021;14:101113. [Crossref] [PubMed]
- Zhang Z, Li X, Sun H. Development of machine learning models integrating PET/CT radiomic and immunohistochemical pathomic features for treatment strategy choice of cervical cancer with negative pelvic lymph node by mediating COX-2 expression. Front Physiol 2022;13:994304. [Crossref] [PubMed]
- Arezzo F, Cormio G, Mongelli M, et al. Machine learning applied to MRI evaluation for the detection of lymph node metastasis in patients with locally advanced cervical cancer treated with neoadjuvant chemotherapy. Arch Gynecol Obstet 2023;307:1911-9. [Crossref] [PubMed]
- Liu S, Zhou Y, Wang C, et al. Prediction of lymph node status in patients with early-stage cervical cancer based on radiomic features of magnetic resonance imaging (MRI) images. BMC Med Imaging 2023;23:101. [Crossref] [PubMed]
- Lucia F, Bourbonne V, Pleyers C, et al. Multicentric development and evaluation of (18)F-FDG PET/CT and MRI radiomics models to predict para-aortic lymph node involvement in locally advanced cervical cancer. Eur J Nucl Med Mol Imaging 2023;50:2514-28. [Crossref] [PubMed]
- Monthatip K, Boonnag C, Muangmool T, et al. A machine learning-based prediction model of pelvic lymph node metastasis in women with early-stage cervical cancer. J Gynecol Oncol 2024;35:e17. [Crossref] [PubMed]
- Li P, Feng B, Liu Y, et al. Deep learning nomogram for predicting lymph node metastasis using computed tomography image in cervical cancer. Acta Radiol 2023;64:360-9. [Crossref] [PubMed]
- Qin F, Sun X, Tian M, et al. Prediction of lymph node metastasis in operable cervical cancer using clinical parameters and deep learning with MRI data: a multicentre study. Insights Imaging 2024;15:56. [Crossref] [PubMed]
- Yang X, Wang Y, Zhang J, et al. A Novel Ultrasound-Based Radiomics Model for the Preoperative Prediction of Lymph Node Metastasis in Cervical Cancer. Ultrasound Med Biol 2024;50:1793-9. [Crossref] [PubMed]
- Yang S, Zhang W, Liu C, et al. Predictive value and potential association of PET/CT radiomics on lymph node metastasis of cervical cancer. Ann Med Surg (Lond) 2024;86:805-10. [Crossref] [PubMed]
- Yang LY, Siow TY, Lin YC, et al. Computer-Aided Segmentation and Machine Learning of Integrated Clinical and Diffusion-Weighted Imaging Parameters for Predicting Lymph Node Metastasis in Endometrial Cancer. Cancers (Basel) 2021;13:1406. [Crossref] [PubMed]
- Liu X, Xiao W, Qiao J, et al. Prediction of Lymph Node Metastasis in Endometrial Cancer Based on Color Doppler Ultrasound Radiomics. Acad Radiol 2024;31:4499-508. [Crossref] [PubMed]
- Yao H, Zhang X. Prediction Model of Residual Neural Network for Pathological Confirmed Lymph Node Metastasis of Ovarian Cancer. Biomed Res Int 2022;2022:9646846. [Crossref] [PubMed]
- Huang G, Cui Y, Wang P, et al. Multi-Parametric Magnetic Resonance Imaging-Based Radiomics Analysis of Cervical Cancer for Preoperative Prediction of Lymphovascular Space Invasion. Front Oncol 2021;11:663370. [Crossref] [PubMed]
- Wang S, Liu X, Wu Y, et al. Habitat-based radiomics enhances the ability to predict lymphovascular space invasion in cervical cancer: a multi-center study. Front Oncol 2023;13:1252074. [Crossref] [PubMed]
- Wu Y, Wang S, Chen Y, et al. A Multicenter Study on Preoperative Assessment of Lymphovascular Space Invasion in Early-Stage Cervical Cancer Based on Multimodal MR Radiomics. J Magn Reson Imaging 2023;58:1638-48. [Crossref] [PubMed]
- Luo Y, Mei D, Gong J, et al. Multiparametric MRI-Based Radiomics Nomogram for Predicting Lymphovascular Space Invasion in Endometrial Carcinoma. J Magn Reson Imaging 2020;52:1257-62. [Crossref] [PubMed]
- Yan B, Jia Y, Li Z, et al. Preoperative prediction of lymphovascular space invasion in endometrioid adenocarcinoma: an MRI-based radiomics nomogram with consideration of the peritumoral region. Acta Radiol 2023;64:2636-45. [Crossref] [PubMed]
- Chen X, Wang Y, Shen M, et al. Deep learning for the determination of myometrial invasion depth and automatic lesion identification in endometrial cancer MR imaging: a preliminary study in a single institution. Eur Radiol 2020;30:4985-94. [Crossref] [PubMed]
- Rodríguez-Ortega A, Alegre A, Lago V, et al. Machine Learning-Based Integration of Prognostic Magnetic Resonance Imaging Biomarkers for Myometrial Invasion Stratification in Endometrial Cancer. J Magn Reson Imaging 2021;54:987-95. [Crossref] [PubMed]
- Stanzione A, Cuocolo R, Del Grosso R, et al. Deep Myometrial Infiltration of Endometrial Cancer on MRI: A Radiomics-Powered Machine Learning Pilot Study. Acad Radiol 2021;28:737-44. [Crossref] [PubMed]
- Wang Y, Bi Q, Deng Y, et al. Development and Validation of an MRI-based Radiomics Nomogram for Assessing Deep Myometrial Invasion in Early Stage Endometrial Adenocarcinoma. Acad Radiol 2023;30:668-79. [Crossref] [PubMed]
- Xiong L, Chen C, Lin Y, et al. A computer-aided determining method for the myometrial infiltration depth of early endometrial cancer on MRI images. Biomed Eng Online 2023;22:103. [Crossref] [PubMed]
- Lefebvre TL, Ciga O, Bhatnagar SR, et al. Predicting histopathology markers of endometrial carcinoma with a quantitative image analysis approach based on spherical harmonics in multiparametric MRI. Diagn Interv Imaging 2023;104:142-52. [Crossref] [PubMed]
- Xiao ML, Qian T, Fu L, et al. Deep Learning Nomogram for the Identification of Deep Stromal Invasion in Patients With Early-Stage Cervical Adenocarcinoma and Adenosquamous Carcinoma: A Multicenter Study. J Magn Reson Imaging 2024;59:1394-406. [Crossref] [PubMed]
- Hu Y, Ai J. Development and Validation of Radiomics-Based Models for Predicting the Parametrial Invasion in Stage IB1 to IIA2 Cervical Cancer. Int J Gen Med 2024;17:3813-24. [Crossref] [PubMed]
- Chong GO, Park SH, Jeong SY, et al. Prediction Model for Tumor Budding Status Using the Radiomic Features of F-18 Fluorodeoxyglucose Positron Emission Tomography/Computed Tomography in Cervical Cancer. Diagnostics (Basel) 2021;11:1517. [Crossref] [PubMed]
- Chong GO, Park SH, Park NJ, et al. Predicting Tumor Budding Status in Cervical Cancer Using MRI Radiomics: Linking Imaging Biomarkers to Histologic Characteristics. Cancers (Basel) 2021;13:5140. [Crossref] [PubMed]
- Mainenti PP, Stanzione A, Cuocolo R, et al. MRI radiomics: A machine learning approach for the risk stratification of endometrial cancer patients. Eur J Radiol 2022;149:110226. [Crossref] [PubMed]
- Moro F, Albanese M, Boldrini L, et al. Developing and validating ultrasound-based radiomics models for predicting high-risk endometrial cancer. Ultrasound Obstet Gynecol 2022;60:256-68. [Crossref] [PubMed]
- Yang J, Cao Y, Zhou F, et al. Combined deep-learning MRI-based radiomic models for preoperative risk classification of endometrial endometrioid adenocarcinoma. Front Oncol 2023;13:1231497. [Crossref] [PubMed]
- Lefebvre TL, Ueno Y, Dohan A, et al. Development and Validation of Multiparametric MRI-based Radiomics Models for Preoperative Risk Stratification of Endometrial Cancer. Radiology 2022;305:375-86. [Crossref] [PubMed]
- Otani S, Himoto Y, Nishio M, et al. Radiomic machine learning for pretreatment assessment of prognostic risk factors for endometrial cancer and its effects on radiologists' decisions of deep myometrial invasion. Magn Reson Imaging 2022;85:161-7. [Crossref] [PubMed]
- Fang R, Lin N, Weng S, et al. Multiparametric MRI radiomics improves preoperative diagnostic performance for local staging in patients with endometrial cancer. Abdom Radiol (NY) 2024;49:875-87. [Crossref] [PubMed]
- Li X, Dessi M, Marcus D, et al. Prediction of Deep Myometrial Infiltration, Clinical Risk Category, Histological Type, and Lymphovascular Space Invasion in Women with Endometrial Cancer Based on Clinical and T2-Weighted MRI Radiomic Features. Cancers (Basel) 2023;15:2209. [Crossref] [PubMed]
- Zhang Y, Qin Z, Li L, et al. Machine Learning-Based Models for Assessing Postoperative Risk Factors in Patients with Cervical Cancer. Acad Radiol 2024;31:1410-8. [Crossref] [PubMed]
- Wang Y, Liu W, Lu Y, et al. Fully Automated Identification of Lymph Node Metastases and Lymphovascular Invasion in Endometrial Cancer From Multi-Parametric MRI by Deep Learning. J Magn Reson Imaging 2024;60:2730-42. [Crossref] [PubMed]
- Feng S, Xia T, Ge Y, et al. Computed Tomography Imaging-Based Radiogenomics Analysis Reveals Hypoxia Patterns and Immunological Characteristics in Ovarian Cancer. Front Immunol 2022;13:868067. [Crossref] [PubMed]
- Avesani G, Tran HE, Cammarata G, et al. CT-Based Radiomics and Deep Learning for BRCA Mutation and Progression-Free Survival Prediction in Ovarian Cancer Using a Multicentric Dataset. Cancers (Basel) 2022;14:2739. [Crossref] [PubMed]
- İnce O, Uysal E, Durak G, et al. Prediction of carcinogenic human papillomavirus types in cervical cancer from multiparametric magnetic resonance images with machine learning-based radiomics models. Diagn Interv Radiol 2023;29:460-8. [Crossref] [PubMed]
- Binas DA, Tzanakakis P, Economopoulos TL, et al. A Novel Approach for Estimating Ovarian Cancer Tissue Heterogeneity through the Application of Image Processing Techniques and Artificial Intelligence. Cancers (Basel) 2023;15:1058. [Crossref] [PubMed]
- Wan S, Zhou T, Che R, et al. CT-based machine learning radiomics predicts CCR5 expression level and survival in ovarian cancer. J Ovarian Res 2023;16:1. [Crossref] [PubMed]
- Zhan F, He L, Yu Y, et al. A multimodal radiomic machine learning approach to predict the LCK expression and clinical prognosis in high-grade serous ovarian cancer. Sci Rep 2023;13:16397. [Crossref] [PubMed]
- Li Q, Huang Y, Xia Y, et al. Radiogenomics for predicting microsatellite instability status and PD-L1 expression with machine learning in endometrial cancers: A multicenter study. Heliyon 2023;9:e23166. [Crossref] [PubMed]
- Jia Y, Hou L, Zhao J, et al. Radiomics analysis of multiparametric MRI for preoperative prediction of microsatellite instability status in endometrial cancer: a dual-center study. Front Oncol 2024;14:1333020. [Crossref] [PubMed]
- Wang J, Song P, Zhang M, et al. A prediction model based on deep learning and radiomics features of DWI for the assessment of microsatellite instability in endometrial cancer. Cancer Med 2024;13:e70046. [Crossref] [PubMed]
- Ning Y, Liu W, Wang H, et al. Determination of p53abn endometrial cancer: a multitask analysis using radiological-clinical nomogram on MRI. Br J Radiol 2024;97:954-63. [Crossref] [PubMed]
- Chiappa V, Bogani G, Interlenghi M, et al. Using Radiomics and Machine Learning Applied to MRI to Predict Response to Neoadjuvant Chemotherapy in Locally Advanced Cervical Cancer. Diagnostics (Basel) 2023;13:3139. [Crossref] [PubMed]
- Zhang Y, Wu C, Xiao Z, et al. A Deep Learning Radiomics Nomogram to Predict Response to Neoadjuvant Chemotherapy for Locally Advanced Cervical Cancer: A Two-Center Study. Diagnostics (Basel) 2023;13:1073. [Crossref] [PubMed]
- Crispin-Ortuzar M, Woitek R, Reinius MAV, et al. Integrated radiogenomics models predict response to neoadjuvant chemotherapy in high grade serous ovarian cancer. Nat Commun 2023;14:6756. [Crossref] [PubMed]
- Yin R, Guo Y, Wang Y, et al. Predicting Neoadjuvant Chemotherapy Response and High-Grade Serous Ovarian Cancer From CT Images in Ovarian Cancer with Multitask Deep Learning: A Multicenter Study. Acad Radiol 2023;30:S192-201. [Crossref] [PubMed]
- Yang H, Xu Y, Dong M, et al. Automated Prediction of Neoadjuvant Chemoradiotherapy Response in Locally Advanced Cervical Cancer Using Hybrid Model-Based MRI Radiomics. Diagnostics (Basel) 2023;14:5. [Crossref] [PubMed]
- Cai Z, Li S, Xiong Z, et al. Multimodal MRI-based deep-radiomics model predicts response in cervical cancer treated with neoadjuvant chemoradiotherapy. Sci Rep 2024;14:19090. [Crossref] [PubMed]
- Jeong S, Yu H, Park SH, et al. Comparing deep learning and handcrafted radiomics to predict chemoradiotherapy response for locally advanced cervical cancer using pretreatment MRI. Sci Rep 2024;14:1180. [Crossref] [PubMed]
- Wu RR, Zhou YM, Xie XY, et al. Delta radiomics analysis for prediction of intermediary- and high-risk factors for patients with locally advanced cervical cancer receiving neoadjuvant therapy. Sci Rep 2023;13:19409. [Crossref] [PubMed]
- Fang M, Kan Y, Dong D, et al. Multi-Habitat Based Radiomics for the Prediction of Treatment Response to Concurrent Chemotherapy and Radiation Therapy in Locally Advanced Cervical Cancer. Front Oncol 2020;10:563. [Crossref] [PubMed]
- Veeraraghavan H, Vargas HA, Jimenez-Sanchez A, et al. Integrated Multi-Tumor Radio-Genomic Marker of Outcomes in Patients with High Serous Ovarian Carcinoma. Cancers (Basel) 2020;12:3403. [Crossref] [PubMed]
- Yi X, Liu Y, Zhou B, et al. Incorporating SULF1 polymorphisms in a pretreatment CT-based radiomic model for predicting platinum resistance in ovarian cancer treatment. Biomed Pharmacother 2021;133:111013. [Crossref] [PubMed]
- Lei R, Yu Y, Li Q, et al. Deep learning magnetic resonance imaging predicts platinum sensitivity in patients with epithelial ovarian cancer. Front Oncol 2022;12:895177. [Crossref] [PubMed]
- Na I, Noh JJ, Kim CK, et al. Combined radiomics-clinical model to predict platinum-sensitivity in advanced high-grade serous ovarian carcinoma using multimodal MRI. Front Oncol 2024;14:1341228. [Crossref] [PubMed]
- Bi Q, Miao K, Xu N, et al. Habitat Radiomics Based on MRI for Predicting Platinum Resistance in Patients with High-Grade Serous Ovarian Carcinoma: A Multicenter Study. Acad Radiol 2024;31:2367-80. [Crossref] [PubMed]
- Zhuang H, Li B, Ma J, et al. An Attention-Based Deep Learning Network for Predicting Platinum Resistance in Ovarian Cancer. IEEE Access 2024;12:41000-8.
- Shen WC, Chen SW, Wu KC, et al. Prediction of local relapse and distant metastasis in patients with definitive chemoradiotherapy-treated cervical cancer by deep learning from [18F]-fluorodeoxyglucose positron emission tomography/computed tomography. Eur Radiol 2019;29:6741-9. [Crossref] [PubMed]
- Zhou Z, Maquilan GM, Thomas K, et al. Quantitative PET Imaging and Clinical Parameters as Predictive Factors for Patients With Cervical Carcinoma: Implications of a Prediction Model Generated Using Multi-Objective Support Vector Machine Learning. Technol Cancer Res Treat 2020;19:1533033820983804. [Crossref] [PubMed]
- Takada A, Yokota H, Watanabe Nemoto M, et al. A multi-scanner study of MRI radiomics in uterine cervical cancer: prediction of in-field tumor control after definitive radiotherapy based on a machine learning method including peritumoral regions. Jpn J Radiol 2020;38:265-73. [Crossref] [PubMed]
- Jajodia A, Gupta A, Prosch H, et al. Combination of Radiomics and Machine Learning with Diffusion-Weighted MR Imaging for Clinical Outcome Prognostication in Cervical Cancer. Tomography 2021;7:344-57. [Crossref] [PubMed]
- Nakajo M, Jinguji M, Tani A, et al. Machine learning based evaluation of clinical and pretreatment (18)F-FDG-PET/CT radiomic features to predict prognosis of cervical cancer patients. Abdom Radiol (NY) 2022;47:838-47. [Crossref] [PubMed]
- Kawahara D, Nishibuchi I, Kawamura M, et al. Radiomic Analysis for Pretreatment Prediction of Recurrence Post-Radiotherapy in Cervical Squamous Cell Carcinoma Cancer. Diagnostics (Basel) 2022;12:2346. [Crossref] [PubMed]
- Wang J, Mao Y, Gao X, et al. Recurrence risk stratification for locally advanced cervical cancer using multi-modality transformer network. Front Oncol 2023;13:1100087. [Crossref] [PubMed]
- Wu KC, Chen SW, Hsieh TC, et al. Early prediction of distant metastasis in patients with uterine cervical cancer treated with definitive chemoradiotherapy by deep learning using pretreatment [18F]fluorodeoxyglucose positron emission tomography/computed tomography. Nucl Med Commun 2024;45:196-202. [Crossref] [PubMed]
- Ai Y, Zhu X, Zhang Y, et al. MRI radiomics nomogram integrating postoperative adjuvant treatments in recurrence risk prediction for patients with early-stage cervical cancer. Radiother Oncol 2024;197:110328. [Crossref] [PubMed]
- Zhang Y, Wu C, Du J, et al. Prediction of recurrence risk factors in patients with early-stage cervical cancers by nomogram based on MRI handcrafted radiomics features and deep learning features: a dual-center study. Abdom Radiol (NY) 2024;49:258-70. [Crossref] [PubMed]
- Lin Z, Wang T, Li Q, et al. Development and validation of MRI-based radiomics model to predict recurrence risk in patients with endometrial cancer: a multicenter study. Eur Radiol 2023;33:5814-24. [Crossref] [PubMed]
- Wang S, Liu Z, Rong Y, et al. Deep learning provides a new computed tomography-based prognostic biomarker for recurrence prediction in high-grade serous ovarian cancer. Radiother Oncol 2019;132:171-7. [Crossref] [PubMed]
- Li HM, Gong J, Li RM, et al. Development of MRI-Based Radiomics Model to Predict the Risk of Recurrence in Patients With Advanced High-Grade Serous Ovarian Carcinoma. AJR Am J Roentgenol 2021;217:664-75. [Crossref] [PubMed]
- Liu L, Wan H, Liu L, et al. Deep Learning Provides a New Magnetic Resonance Imaging-Based Prognostic Biomarker for Recurrence Prediction in High-Grade Serous Ovarian Cancer. Diagnostics (Basel) 2023;13:748. [Crossref] [PubMed]
- Wei M, Zhang Y, Ding C, et al. Associating Peritoneal Metastasis With T2-Weighted MRI Images in Epithelial Ovarian Cancer Using Deep Learning and Radiomics: A Multicenter Study. J Magn Reson Imaging 2024;59:122-31. [Crossref] [PubMed]
- Yin R, Dou Z, Wang Y, et al. Preoperative CECT-Based Multitask Model Predicts Peritoneal Recurrence and Disease-Free Survival in Advanced Ovarian Cancer: A Multicenter Study. Acad Radiol 2024;31:4488-98. [Crossref] [PubMed]
- Ferreira M, Lovinfosse P, Hermesse J, et al. [18F]FDG PET radiomics to predict disease-free survival in cervical cancer: a multi-scanner/center study with external validation. Eur J Nucl Med Mol Imaging 2021;48:3432-43. Erratum in: Eur J Nucl Med Mol Imaging 2021;48:3745-6. [Crossref] [PubMed]
- Xu C, Liu W, Zhao Q, et al. CT-based radiomics nomogram for overall survival prediction in patients with cervical cancer treated with concurrent chemoradiotherapy. Front Oncol 2023;13:1287121. [Crossref] [PubMed]
- Liu H, Cui Y, Chang C, et al. Development and validation of a (18)F-FDG PET/CT radiomics nomogram for predicting progression free survival in locally advanced cervical cancer: a retrospective multicenter study. BMC Cancer 2024;24:150. [Crossref] [PubMed]
- Xin W, Rixin S, Linrui L, et al. Machine learning-based radiomics for predicting outcomes in cervical cancer patients undergoing concurrent chemoradiotherapy. Comput Biol Med 2024;177:108593. [Crossref] [PubMed]
- Zhu S, Lin L, Liu Q, et al. Integrating a deep neural network and Transformer architecture for the automatic segmentation and survival prediction in cervical cancer. Quant Imaging Med Surg 2024;14:5408-19. [Crossref] [PubMed]
- Nakajo M, Jinguji M, Tani A, et al. Application of a Machine Learning Approach for the Analysis of Clinical and Radiomic Features of Pretreatment [18F]-FDG PET/CT to Predict Prognosis of Patients with Endometrial Cancer. Mol Imaging Biol 2021;23:756-65. [Crossref] [PubMed]
- Hoivik EA, Hodneland E, Dybvik JA, et al. A radiogenomics application for prognostic profiling of endometrial cancer. Commun Biol 2021;4:1363. [Crossref] [PubMed]
- Coada CA, Santoro M, Zybin V, et al. A Radiomic-Based Machine Learning Model Predicts Endometrial Cancer Recurrence Using Preoperative CT Radiomic Features: A Pilot Study. Cancers (Basel) 2023;15:4534. [Crossref] [PubMed]
- Lu H, Arshad M, Thornton A, et al. A mathematical-descriptor of tumor-mesoscopic-structure from computed-tomography images annotates prognostic- and molecular-phenotypes of epithelial ovarian cancer. Nat Commun 2019;10:764. [Crossref] [PubMed]
- Boehm KM, Aherne EA, Ellenson L, et al. Multimodal data integration using machine learning improves risk stratification of high-grade serous ovarian cancer. Nat Cancer 2022;3:723-33. [Crossref] [PubMed]
- Zheng Y, Wang F, Zhang W, et al. Preoperative CT-based deep learning model for predicting overall survival in patients with high-grade serous ovarian cancer. Front Oncol 2022;12:986089. [Crossref] [PubMed]
- Ness RBJoint Policy Committee, Societies of Epidemiology. Influence of the HIPAA Privacy Rule on health research. JAMA 2007;298:2164-70. [Crossref] [PubMed]
- Mongan J, Moy L, Kahn CE Jr. Checklist for Artificial Intelligence in Medical Imaging (CLAIM): A Guide for Authors and Reviewers. Radiol Artif Intell 2020;2:e200029. [Crossref] [PubMed]
- Tejani AS, Klontzas ME, Gatti AA, et al. Checklist for Artificial Intelligence in Medical Imaging (CLAIM): 2024 Update. Radiol Artif Intell 2024;6:e240300. [Crossref] [PubMed]
- Rivera SC, Liu X, Chan AW, et al. Guidelines for clinical trial protocols for interventions involving artificial intelligence: the SPIRIT-AI Extension. BMJ 2020;370:m3210. [Crossref] [PubMed]
- Liu X, Rivera SC, Moher D, et al. Reporting guidelines for clinical trial reports for interventions involving artificial intelligence: the CONSORT-AI Extension. BMJ 2020;370:m3164. [Crossref] [PubMed]
- Novakovsky G, Dexter N, Libbrecht MW, et al. Obtaining genetics insights from deep learning via explainable artificial intelligence. Nat Rev Genet 2023;24:125-37. [Crossref] [PubMed]
(English Language Editor: J. Jones)