A novel immune-related gene signature predicts survival in esophageal squamous cell carcinoma
Introduction
Esophageal cancer (EC) is the sixth leading cause of cancer death worldwide (1) and has two common subtypes: esophageal squamous cell carcinoma (ESCC) and esophageal adenocarcinoma (EAC). ESCC is the most frequent subtype of EC (2). Although the emergence of new chemotherapeutic drugs and regimens has improved the overall survival (OS) rate of ESCC patients, drug resistance has significantly worsened patients’ prognostic outcome. At present, the pathogenesis of ESCC is unclear. Therefore, there is a pressing need for effective biomarkers and targeted therapeutics to improve patients’ prognostic outcome.
Cancer immunotherapy has been a major driver of personalized medicine, with aggressive efforts to leverage the immune system to fight tumors (3,4). It has shown encouraging results as an emerging treatment for some cancers, including melanoma (5), hepatocellular carcinoma (6), and non-small cell lung cancer (7). Tumor cells in the tumor microenvironment (TME) can mimic immune cells’ functions to affect the composition and function of host immune cells and induce immunosuppression by overexpressing IRGs, thereby promoting the proliferation and spread of tumor cells (8). The acquisition of immune functions maintains tumor cells that can survive in the immunosuppressive TME. Therefore, patients with different immune infiltration states may have different prognoses. Meanwhile, reliable biomarkers or stratification systems that can be used for more accurate prediction are essential in the TME.
Several immune-related parameters or signatures that predict ESCC patients’ prognosis have been reported (9-11), further suggesting that distinct immune infiltration status has a profound influence on ESCC patient outcomes. Herein, we established a predictive risk model based on IRGs to estimate patient outcomes in ESCC. Importantly, we evaluated this model’s predictive ability in assessing OS in different immune infiltration patterns and screened out pathways closely related to these patterns. We performed a systematic investigation of the immune phenotype within the ESCC microenvironment to better understand the complex antitumor response and guide effective immunotherapies in ESCC. We present the following article in accordance with the Materials Design Analysis Reporting (MDAR) checklist (available at http://dx.doi.org/10.21037/tcr-20-2665).
Methods
Data from the UCSC Xena website
Log2(FPKM+1) format ribonucleic acid (RNA)-sequencing data used in this study were downloaded from the University of California, Santa Cruz (UCSC) Xena website (https://xenabrowser.net/), which provides comprehensive information retrieval services based on the Cancer Genome Atlas (TCGA) and Genotype-Tissue Expression (GTEx) project databases. We collected and processed transcription data from 79 ESCC samples from the TCGA database and the expression data of 269 normal esophageal mucosa samples from the GTEx project database. For comparative analysis between ESCC samples and many normal samples, we combined the TCGA dataset with the GTEx dataset (TCGA-GTEx dataset) for subsequent analysis. Moreover, the corresponding survival and clinical information files of 79 patients with ESCC were also downloaded from the UCSC. The study was conducted in accordance with the Declaration of Helsinki (as revised in 2013).
Screening immune-related differential genes
The limma R package was used to screen differentially expressed genes (DEGs) between normal and ESCC samples. |Log2FC| ≥2 and PFDR<0.05 were used as the interception criteria for screening DEGs. We also derived a list of 1,811 IRGs via the Immunology Database and Analysis Portal (ImmPort, https://immport.niaid.nih.gov) database. We then intersected DEGs with IRGs to obtain IRDEGs. Gene Ontology (GO) and Kyoto Encyclopedia of Genes and Genomes (KEGG) enrichment analyses were simultaneously conducted to explore potential molecular mechanisms of the IRDEGs, which were performed by the R bioconductor package clusterProfiler. PFDR<0.01 was set as the enrichment cut-off to screen meaningful enrichment results.
Construction of the immune-related prognostic signature
To provide clinicians with a quantitative tool to predict the individual probability of survival time, we developed an immune-related prognostic signature. A univariate Cox analysis was performed to select IRDEGs with a significant prognostic value (P<0.05) as candidates for the prognostic signature. Next, these selected IRDEGs were further refined with stepwise forward selection by employing the least absolute shrinkage and selection operator (LASSO) method. Finally, a multi-gene prognostic signature was constructed by multivariate Cox analysis, and an individual risk score was calculated based on a combination of coefficients and the expression status of these refined IRDEGs. The risk score was set as:
Ri, N, expression, and βgene i represented the patient’s risk score, the number of these refined IRDEGs, the gene expression level, and the coefficient value, respectively. The median value was used as the cutoff value for dividing high- and low-risk groups. Subsequently, Kaplan-Meier survival analysis, principal component analysis (PCA), and univariate and multivariate Cox analysis, were performed to evaluate the predictive value of this prognostic signature.
Gene set enrichment analysis (GSEA)
ESCC cases were divided into two risk groups according to the median value of the risk scores. GSEA was performed using Broad Institute GSEA software 4.1.0 (https://www.gsea-msigdb.org/gsea/downloads.jsp) in three immune clusters. The annotated gene set “h.all.v7.1.symbols.gmt” was selected and used for the enrichment analysis. Permutations were set to 1,000 to obtain a normalized enrichment score (NES). A normal P alue <0.05 and NES >1.5 were considered significantly enriched. We chose all hallmark gene sets with significant enrichment and displayed gene sets enrichment plots. The xenobiotic metabolism pathway was significantly enriched and up-regulated in both high-risk groups of the immunity-M and immunity-H clusters. Effective genes enriched by this pathway in two clusters were then intersected, and 34 significant correlation hub genes were imported to construct the protein-protein interaction (PPI) network.
Protein-protein interaction network (PPI)
STRING (https://string-db.org) was used for PPI network construction and hub gene screening. The PPI network was constructed by setting medium confidence at 0.400. After the irrelevant genes were excluded, 23 genes were used for constructing the PPI network.
Statistical analysis
All analyses were conducted using R software (version 3.5.3, https://www.r-project.org). PCA was also performed by using the ggplot2 package of R. Clinical correlation analyses were tested by the Student’s t-test or Wilcoxon test for two groups, and by one-way analysis of variance (ANOVA) or Kruskal-Wallis test for more than two groups. The Cox proportional hazards regression model was used for univariate and multivariate analyses. The performance of the multivariate Cox regression model was evaluated by the concordance index (C-index) using the survcompr software package. The area under the curve (AUC) of the multi-index receiver operating characteristic (multi-ROC) curve was calculated via the survivalROC R package to validate the prognostic signature’s performance. All tests were two sided, and P-values of less than 0.05 was considered statistically significant.
Results
Clinical characteristics
Seventy-nine patients diagnosed with ESCC were registered in the TCGA cohort, including 68 male (86.08%) and 11 female (13.92%) patients. Detailed clinical characteristics of all patients are presented in Table 1.
Table 1
Variables | Case, n (%) |
---|---|
Age at diagnosis (year) | |
≤55 | 34 (43.04) |
>55 | 45 (56.96) |
Gender | |
Male | 68 (86.08) |
Female | 11 (13.92) |
Histologic grade | |
G1 | 15 (18.99) |
G2 | 38 (48.10) |
G3 | 19 (24.05) |
NA | 7 (8.86) |
Alcohol history | |
No | 19 (24.05) |
Yes | 58 (73.42) |
NA | 2 (2.53) |
Pathologic-M | |
M0 | 70 (88.61) |
M1 | 3 (3.80) |
NA | 6 (7.59) |
Pathologic-N | |
N0 | 45 (56.96) |
N1 | 26 (32.91) |
N2 | 5 (6.33) |
N3 | 1 (1.27) |
NA | 2 (2.53) |
Pathologic-T | |
T1 | 7 (8.86) |
T2 | 27 (34.17) |
T3 | 41 (51.90) |
T4 | 3 (3.80) |
NA | 1 (1.27) |
Pathologic-stage | |
I | 6 (7.59) |
II | 47 (59.49) |
III | 22 (27.85) |
IV | 3 (3.80) |
NA | 1 (1.27) |
NA, not available.
Screening of immune-related genes
As shown in Figure 1A,B, we initially identified a total of 1,575 DEGs in ESCC tissues compared with normal tissues (P<0.05, |log2FC| >2). Next, we intersected 1,811 IRGs from ImmPort with 1,575 DEGs from the TCGA-GTEx dataset. As a result, a total of 124 IRDEGs, among which 67 genes were up-regulated while 57 genes were down-regulated, were obtained and displayed using a Venn diagram (Figure 1C).
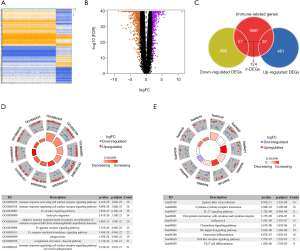
The 124 IRDEGs examined in this study played various roles in immune functions, and many of them were involved in the TME. Through Gene Ontology (GO) enrichment analysis, we found that the top 10 GO biological process (BP) terms were mainly related to receptor signaling pathways (Figure 1D). The most enriched GO BP term correlated with those genes was the “immune response-activating cell surface receptor signaling pathway”, which contained 36 IRDEGs. The top 10 KEGG enrichment terms are presented in Figure 1E. Epstein-Barr virus infection and cytokine-cytokine receptor interaction were the most frequently identified KEGG pathway. The results indicate that these IRGs directly or indirectly affect tumor cells in the TME by acting on different signaling pathways.
Construction and evaluation of the prognostic signature based on IRDEGs
In our univariate Cox regression analysis, seven genes (FCER1G, PSMD6, ISG20, IRF9, CD14, EGFR, and PLXNA1) were selected as candidates for developing the predictive model (Figure 2A). We then chose the appropriate candidates using the LASSO method (Figure 2B,C), and subsequently constructed an immune-related prognostic signature with a C-index of 0.73 [95% confidence interval (CI): 0.63–0.84, P=2.02E-05] to predict the outcome of ESCC patients by multivariate Cox regression analysis (Figure 2D). The risk score distribution and survival status were ranked by the risk scores in the ESCC set (shown in Figure 3A,B). The heatmap showing this signature’s expression profiles in the low- and high-risk groups is shown in Figure 3C. Through PCA analysis based on this immune signature, we found that high- and low-risk groups could be well distinguished (Figure 3D). Patients in the low-risk group had a longer OS compared to those in the high-risk group (Figure 3E). These results indicate that this prognostic signature exhibits good prognostic, predictive ability.
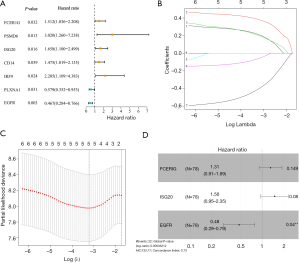
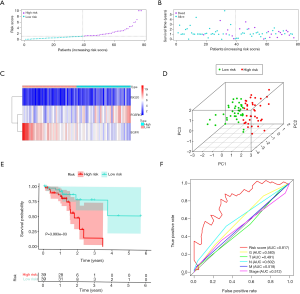
Following this, we evaluated the prognostic, predictive value of five clinicopathological characteristics, including T stage, lymph node metastasis (N), distant metastasis of tumor (M), histologic grade (G), and stage, and determined the risk scores by Cox regression analysis. The results of the univariate Cox regression analysis suggested that the risk score (P<0.001) and N (P=0.036) were associated with prognosis, whereas the other clinicopathological factors were not (Table 2). Multivariate Cox regression analysis suggested that only the risk score could become an independent predictor after the other parameters were adjusted (Table 2). Furthermore, through multi-index ROC analysis, we affirmed that the diagnostic efficiency of this immune signature (AUC =0.817) was better than the above five clinicopathological characteristics in predicting survival, including T (AUC =0.515), N (AUC =0.635), M (AUC =0.541), G (AUC =0.582), and stage (AUC =0.581) (Figure 3F). Put simply, this signature is an independent prognostic indicator and is superior to the other five clinicopathological characteristics.
Table 2
Variables | Univariate analysis | Multivariate analysis | |||
---|---|---|---|---|---|
Hazard ratio (95% CI) | P value | Hazard ratio (95% CI) | P value | ||
G | 1.00 (0.55–1.82) | 0.999 | 1.30 (0.59–2.86) | 0.518 | |
T | 1.05 (0.57–1.93) | 0.868 | 1.99 (0.70–5.68) | 0.200 | |
N (yes/no) | 2.67 (1.07–6.69) | 0.036* | 4.70 (0.98–22.59) | 0.053 | |
M (yes/no) | 3.20 (0.91–11.24) | 0.070 | 6.36 (0.68–59.89) | 0.106 | |
stage | 2.22 (0.96–5.15) | 0.063 | 0.59 (0.11–3.05) | 0.525 | |
RiskScore | 1.30 (1.13–1.49) | <0.001* | 1.32 (1.11–1.56) | 0.002* |
*, significant results.
We also explored the clinical significance of the included genes and the risk score of the prognostic signature. The results showed that the expression of FCER1G was significantly higher in advanced G stage cases (Figure 4A), the expression of ISG20 was significantly lower in advanced T stage cases (Figure 4B), and the risk score was significantly higher in advanced G stage cases and in males (Figure 4C,D) (all P<0.05).
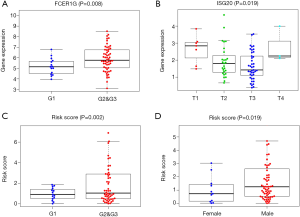
Evaluation of the prognostic, predictive ability of this prognostic signature in three immune clusters
The enrichment of immune cells and immune-related pathways were estimated using the single-sample GSEA (ssGSEA) algorithm. ESCC samples were clustered into low, intermediate, and high immune infiltration clusters (Immunity_L, Immunity_M, and Immunity_H) using the hierarchical clustering method (Figure 5A). The immunity_H cluster showed a higher risk score than both the immunity_M and immunity_L clusters (Figure 5B). Similarly, patients with a low risk score had a lower proportion of high immune infiltration than patients with a high risk score (Figure 5C). High immune infiltration in patients was linked to a high risk score and was associated with an unfavorable outcome (Figure 5D). The association between OS and the different clusters of ESCC was analyzed by log-rank test (Figure 5E,F,G). Due to the limited sample size, survival prediction was significant only in the immunity_M cluster. However, it can be seen from the results that the immunity_M and immunity_H clusters had an unfavorable survival probability compared with the immunity_L cluster.
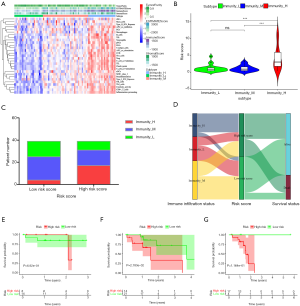
Functional annotation and screening hub genes
To identify the constructed immune signature’s underlying biological characteristics, GSEA analysis was performed based on the high- and low-risk score groups in the three immune clusters, respectively. No significant pathway was enriched in the immunity_L cluster. The GSEA results showed that only xenobiotic metabolism was significantly up-regulated in the high-risk score group of the immunity_M cluster, which is the same result observed in the high-risk score group of immunity_H cluster. In contrast, the mitotic spindle, glycolysis, phosphatidylinositol 3-hydroxy kinase/protein kinase B/mammalian target of rapamycin (PI3K/AKT/MTOR) signaling, G2M checkpoint, and unfolded protein response pathways were significantly down-regulated in the high-risk score group of the immunity_H cluster (Figure 6A,B,C,D,E,F,G).
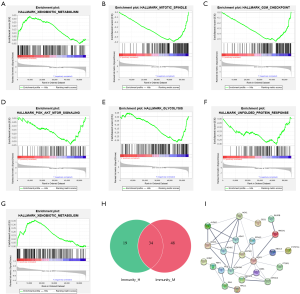
Next, effective genes enriched by the xenobiotic metabolism pathway in two clusters were intersected, and 34 significant correlation hub genes were imported to construct the PPI network (Figure 6H). Ultimately, the PPI network was constructed by 23 hub genes that were significantly related to this pathway (Figure 6I). We found that numerous genes, such as ADH7 (12), AKR1C2 (13), ALDH2 (14), NQO1 (15), ADH1C (12), EPHX1 (16), BLVRB (17), HMOX1 (18), ABCC3 (19), ALDH3A1 (20), and IDH1 (21,22), are related to ESCC tumor susceptibility, tumorigenesis, and progression.
Discussion
Cancer immunotherapy is a promising new avenue for ESCC treatment that acts primarily on the TME. Also, the heterogeneity of the TME is closely related to the efficiency of immunotherapy. In the present study, we attempted to identify an immune-related signature that contributes to the OS of ESCC. Using multiple indicators, we first evaluated its ability to predict prognosis in all samples. We discovered that this signature not only has good prognostic predictive ability but can also be used as an independent prognostic indicator. Importantly, we also assessed its ability to predict prognosis under different immune infiltration states, and used GSEA to determine which pathways were significantly enriched in the high- and low-risk groups. We found that this signature’s ability to predict prognosis was different under different infiltration patterns, and the pathways of significant enrichment in the high- and low-risk groups were also non-identical.
A multi-gene risk prediction model based on FCER1G, EGFR, and ISG20 was established in this study. FCER1G and ISG20 [also called cluster of differentiation (CD)25] are two risk genes, and EGFR is a protective gene (Figure 2D). Pauline et al. reported that FCER1G is essential for tumor development and squamous carcinogenesis by B cells, humoral immunity and activating FCER1G to establish chronic inflammatory programs (23). FCER1G is also engaged in many immune responses and plays a tumor-promoting role in numerous types of tumors, such as meningioma (24) and childhood leukemia (25). At present, there are no directly-related reports concerning the association between FCER1G and ESCC; however, it is generally conceded that chronic inflammation is closely related to oncogenesis. Therefore, we might assume that the overexpression of FCER1G regulates immune-related pathways of tumorigenesis. Furthermore, Guan et al. found that patients with ESCC had a significant increase in circulating anti-CD25 immunoglobulin G (IgG) antibodies compared to control subjects and that patients with early stage ESCC (stage I) had the highest expression of circulating IgG (26). In this study, we discovered that patients with T1 stage ESCC had the highest expression of ISG20 (Figure 4B). So, ISG20 may be considered as a potential biomarker for early diagnosis and prediction of ESCC recurrence or survival. EGFR expression was usually associated with a more advanced tumor stage and a reduced OS in patients with esophageal and esophagogastric junction adenocarcinomas (27). ESCC predominantly involves EGFR gene copy number alterations and protein overexpression (28-31), with little EGFR mutation (32-34). Furthermore, the literature regarding EGFR expression in ESCC contains conflicting data on the relationship between overexpression and survival (31,35,36). Although several clinical studies show the beneficial effects of EGFR inhibitors in the treatment of ESCC (37,38), evidence supporting the validity of EGFR-targeting therapies for esophageal cancer (39) is not robust. Yoshioka et al. discovered that one of the factors affecting the therapeutic effect of EGFR inhibitors in ESCC cells is cell phenotype. Mesenchymal-like ESCC cells are resistant to EGFR inhibitors because EGFR signaling is not blocked. On the contrary, EGFR signaling is affected by EGFR inhibitors, which exhibit antitumor effects on epithelial-like ESCC cells accompanied by the promotion of squamous cell differentiation. In the future, more potential mechanisms need to be studied to determine the effect of EGFR targeted therapy for ESCC (40).
We found that the OS of patients in the high-risk group was poor through model analysis in all samples compared with those in the low-risk group (Figure 3E). Patients in the high-risk group received more precise treatment options to improve their prognosis. We further evaluated the signature’s predictive ability for the OS of patients in different immune infiltration states. We discovered that the predictive ability and the predictive results of this signature could be different in different immune infiltration states (Figure 5E,F,G). Patients in the high-risk score group had a higher infiltration status and poorer survival than patients in the low-risk score group in the immunity_H and immunity_M clusters. However, further analysis of the prognostic evaluation for the OS of patients with low immune infiltration status in this paper is needed.
To understand which gene sets can be enriched in the high- and low-risk groups in different immune infiltration clusters, GSEA analysis was performed based on the high- and low-risk group in three immune clusters, respectively. Only xenobiotic metabolism was significantly up-regulated in the high-risk group of the immunity_M (Figure 6G) and immunity_H (Figure 6A) clusters. We found that some studies have been conducted on the association between xenobiotic metabolizing genes polymorphism and ESCC. Several studies have linked polymorphisms in genes encoding phase I and phase II xenobiotic metabolizing enzymes to the modulation of risk for ESCC (41-44). Based on the PPI network, we found numerous genes significantly related to the xenobiotic metabolism pathway, which are related to ESCC tumor susceptibility, tumorigenesis, and progression. For instance, Wang et al. have demonstrated that the ADH1B-ADH1C-ADH7 cluster single nucleotide polymorphisms (SNPs) confer susceptibility to ESCC in two case-control sets (12). Also, Ren et al. noted that ESCC tumors frequently expressed heme oxygenase 1 (HO-1), and knockdown of HO-1 promoted apoptosis through activation of a reactive oxygen species (ROS)-mediated caspase apoptosis pathway (18). Zhang et al. revealed that AKR1C2 could function as an oncogene (via activation of the PI3K/AKT pathway) and a novel prognostic biomarker and/or a potential therapeutic target in ESCC (13). In contrast, the mitotic spindle, glycolysis, PI3K/AKT/MTOR signaling, G2M checkpoint, and unfolded protein response pathways were significantly up-regulated in the low risk score group of the immunity_H cluster (Figure 6B,C,D,E,F). These pathways could be closely related to the good prognosis of the tumors. Shang et al. has confirmed that down-regulation of BIRC5 as a mitotic spindle checkpoint gene inhibits the migration and invasion of ESCC cells by interacting with the PI3K/AKT signaling pathway (45).
There are some limitations to our research that should be noted. This study is primarily based on database analyses, and lacks clinical practice confirmation. Thus, further verification through clinical trials is needed in the future.
Conclusions
In summary, this study established a stable multi-gene risk prediction and evaluated its predictive value for ESCC patients’ OS. We found several key pathways and hub genes closely related to ESCC patients’ prognosis under different immune infiltration states. Therefore, further verification with clinical trials in the future is needed.
Acknowledgments
Funding: This work was supported by
Footnote
Reporting Checklist: The authors have completed the MDAR checklist. Available at http://dx.doi.org/10.21037/tcr-20-2665
Conflicts of Interest: All authors have completed the ICMJE uniform disclosure form (available at http://dx.doi.org/10.21037/tcr-20-2665). The authors have no conflicts of interest to declare.
Ethical Statement: The authors are accountable for all aspects of the work in ensuring that questions related to the accuracy or integrity of any part of the work are appropriately investigated and resolved. The study was conducted in accordance with the Declaration of Helsinki (as revised in 2013).
Open Access Statement: This is an Open Access article distributed in accordance with the Creative Commons Attribution-NonCommercial-NoDerivs 4.0 International License (CC BY-NC-ND 4.0), which permits the non-commercial replication and distribution of the article with the strict proviso that no changes or edits are made and the original work is properly cited (including links to both the formal publication through the relevant DOI and the license). See: https://creativecommons.org/licenses/by-nc-nd/4.0/.
References
- Bray F, Ferlay J, Soerjomataram I, et al. Global cancer statistics 2018: GLOBOCAN estimates of incidence and mortality worldwide for 36 cancers in 185 countries. CA Cancer J Clin 2018;68:394-424. [Crossref] [PubMed]
- Rustgi AK, El-Serag HB. Esophageal carcinoma. N Engl J Med 2014;371:2499-509. [Crossref] [PubMed]
- Kobold S, Pantelyushin S, Rataj F, et al. Rationale for Combining Bispecific T Cell Activating Antibodies With Checkpoint Blockade for Cancer Therapy. Front Oncol 2018;8:285. [Crossref] [PubMed]
- Popovic A, Jaffee EM, Zaidi N. Emerging strategies for combination checkpoint modulators in cancer immunotherapy. J Clin Invest 2018;128:3209-18. [Crossref] [PubMed]
- Sullivan RJ, Flaherty KT. Immunotherapy: Anti-PD-1 therapies-a new first-line option in advanced melanoma. Nat Rev Clin Oncol 2015;12:625-6. [Crossref] [PubMed]
- Li S, Yang F, Ren X. Immunotherapy for hepatocellular carcinoma. Drug Discov Ther 2015;9:363-71. [Crossref] [PubMed]
- Carter BW, Halpenny DF, Ginsberg MS, et al. Immunotherapy in Non-Small Cell Lung Cancer Treatment: Current Status and the Role of Imaging. J Thorac Imaging 2017;32:300-12. [Crossref] [PubMed]
- Shi X, Li R, Dong X, et al. IRGS: an immune-related gene classifier for lung adenocarcinoma prognosis. J Transl Med 2020;18:55. [Crossref] [PubMed]
- Jiang D, Liu Y, Wang H, et al. Tumour infiltrating lymphocytes correlate with improved survival in patients with esophageal squamous cell carcinoma. Sci Rep 2017;7:44823. [Crossref] [PubMed]
- Nguyen N, Bellile E, Thomas D, et al. Tumor infiltrating lymphocytes and survival in patients with head and neck squamous cell carcinoma. Head Neck 2016;38:1074-84. [Crossref] [PubMed]
- Li Y, Lu Z, Che Y, et al. Immune signature profiling identified predictive and prognostic factors for esophageal squamous cell carcinoma. Oncoimmunology 2017;6:e1356147. [Crossref] [PubMed]
- Wang J, Wei J, Xu X, et al. Replication study of ESCC susceptibility genetic polymorphisms locating in the ADH1B-ADH1C-ADH7 cluster identified by GWAS. PLoS One 2014;9:e94096. [Crossref] [PubMed]
- Zhang ZF, Huang TJ, Zhang XK, et al. AKR1C2 acts as a targetable oncogene in esophageal squamous cell carcinoma via activating PI3K/AKT signaling pathway. J Cell Mol Med 2020;24:9999-10012. [Crossref] [PubMed]
- Liu P, Zhao HR, Li F, et al. Correlations of ALDH2 rs671 and C12orf30 rs4767364 polymorphisms with increased risk and prognosis of esophageal squamous cell carcinoma in the Kazak and Han populations in Xinjiang province. J Clin Lab Anal 2018;32:e22248. [Crossref] [PubMed]
- Mizumoto A, Ohashi S, Kamada M, et al. Combination treatment with highly bioavailable curcumin and NQO1 inhibitor exhibits potent antitumor effects on esophageal squamous cell carcinoma. J Gastroenterol 2019;54:687-98. [Crossref] [PubMed]
- Jain M, Tilak AR, Upadhyay R, et al. Microsomal epoxide hydrolase (EPHX1), slow (exon 3, 113His) and fast (exon 4, 139Arg) alleles confer susceptibility to squamous cell esophageal cancer. Toxicol Appl Pharmacol 2008;230:247-51. [Crossref] [PubMed]
- Zhang J, Wang K, Liu SS, et al. Using proteomic approach to identify tumor-associated proteins as biomarkers in human esophageal squamous cell carcinoma. J Proteome Res 2011;10:2863-72. [Crossref] [PubMed]
- Ren QG, Yang SL, Hu JL, et al. Evaluation of HO-1 expression, cellular ROS production, cellular proliferation and cellular apoptosis in human esophageal squamous cell carcinoma tumors and cell lines. Oncol Rep 2016;35:2270-6. [Crossref] [PubMed]
- Cheng Y, Xu J, Guo J, et al. Circulating autoantibody to ABCC3 may be a potential biomarker for esophageal squamous cell carcinoma. Clin Transl Oncol 2013;15:398-402. [Crossref] [PubMed]
- Jiang YY, Jiang Y, Li CQ, et al. TP63, SOX2, and KLF5 Establish a Core Regulatory Circuitry That Controls Epigenetic and Transcription Patterns in Esophageal Squamous Cell Carcinoma Cell Lines. Gastroenterology 2020;159:1311-27.e19. [Crossref] [PubMed]
- Miyake K, Baba Y, Ishimoto T, et al. Isocitrate dehydrogenase gene mutations and 2-hydroxyglutarate accumulation in esophageal squamous cell carcinoma. Med Oncol 2018;36:11. [Crossref] [PubMed]
- Chen X, Li Q, Wang C, et al. Prognostic and diagnostic potential of isocitrate dehydrogenase 1 in esophageal squamous cell carcinoma. Oncotarget 2016;7:86148-60. [Crossref] [PubMed]
- Andreu P, Johansson M, Affara NI, et al. FcRgamma activation regulates inflammation-associated squamous carcinogenesis. Cancer Cell 2010;17:121-34. [Crossref] [PubMed]
- Rajaraman P, Brenner AV, Neta G, et al. Risk of meningioma and common variation in genes related to innate immunity. Cancer Epidemiol Biomarkers Prev 2010;19:1356-61. [Crossref] [PubMed]
- Han S, Lan Q, Park AK, et al. Polymorphisms in innate immunity genes and risk of childhood leukemia. Hum Immunol 2010;71:727-30. [Crossref] [PubMed]
- Guan S, Liu B, Zhang C, et al. Circulating autoantibody to CD25 may be a potential biomarker for early diagnosis of esophageal squamous cell carcinoma. Clin Transl Oncol 2013;15:825-9. [Crossref] [PubMed]
- Wang KL, Wu TT, Choi IS, et al. Expression of epidermal growth factor receptor in esophageal and esophagogastric junction adenocarcinomas: association with poor outcome. Cancer 2007;109:658-67. [Crossref] [PubMed]
- Yang YL, Xu KL, Zhou Y, et al. Correlation of epidermal growth factor receptor overexpression with increased epidermal growth factor receptor gene copy number in esophageal squamous cell carcinomas. Chin Med J (Engl) 2012;125:450-4. [PubMed]
- Gonzaga IM, Soares-Lima SC, de Santos PT, et al. Alterations in epidermal growth factor receptors 1 and 2 in esophageal squamous cell carcinomas. BMC Cancer 2012;12:569. [Crossref] [PubMed]
- Fichter CD, Timme S, Braun JA, et al. EGFR, HER2 and HER3 dimerization patterns guide targeted inhibition in two histotypes of esophageal cancer. Int J Cancer 2014;135:1517-30. [Crossref] [PubMed]
- Hanawa M, Suzuki S, Dobashi Y, et al. EGFR protein overexpression and gene amplification in squamous cell carcinomas of the esophagus. Int J Cancer 2006;118:1173-80. [Crossref] [PubMed]
- Zhang J, Jiang D, Li X, et al. Establishment and characterization of esophageal squamous cell carcinoma patient-derived xenograft mouse models for preclinical drug discovery. Lab Invest 2014;94:917-26. [Crossref] [PubMed]
- Abedi-Ardekani B, Dar NA, Mir MM, et al. Epidermal growth factor receptor (EGFR) mutations and expression in squamous cell carcinoma of the esophagus in central Asia. BMC Cancer 2012;12:602. [Crossref] [PubMed]
- Kato H, Arao T, Matsumoto K, et al. Gene amplification of EGFR, HER2, FGFR2 and MET in esophageal squamous cell carcinoma. Int J Oncol 2013;42:1151-8. [Crossref] [PubMed]
- Itakura Y, Sasano H, Shiga C, et al. Epidermal growth factor receptor overexpression in esophageal carcinoma. An immunohistochemical study correlated with clinicopathologic findings and DNA amplification. Cancer 1994;74:795-804. [Crossref] [PubMed]
- Gibault L, Metges JP, Conan-Charlet V, et al. Diffuse EGFR staining is associated with reduced overall survival in locally advanced oesophageal squamous cell cancer. Br J Cancer 2005;93:107-15. [Crossref] [PubMed]
- Lu M, Wang X, Shen L, et al. Nimotuzumab plus paclitaxel and cisplatin as the first line treatment for advanced esophageal squamous cell cancer: A single centre prospective phase II trial. Cancer Sci 2016;107:486-90. [Crossref] [PubMed]
- Chen Y, Wu X, Bu S, et al. Promising outcomes of definitive chemoradiation and cetuximab for patients with esophageal squamous cell carcinoma. Cancer Sci 2012;103:1979-84. [Crossref] [PubMed]
- Mohamed A, El-Rayes B, Khuri FR, et al. Targeted therapies in metastatic esophageal cancer: advances over the past decade. Crit Rev Oncol Hematol 2014;91:186-96. [Crossref] [PubMed]
- Yoshioka M, Ohashi S, Ida T, et al. Distinct effects of EGFR inhibitors on epithelial- and mesenchymal-like esophageal squamous cell carcinoma cells. J Exp Clin Cancer Res 2017;36:101. [Crossref] [PubMed]
- Abbas A, Delvinquiere K, Lechevrel M, et al. GSTM1, GSTT1, GSTP1 and CYP1A1 genetic polymorphisms and susceptibility to esophageal cancer in a French population: different pattern of squamous cell carcinoma and adenocarcinoma. World J Gastroenterol 2004;10:3389-93. [Crossref] [PubMed]
- Shah IA, Bhat GA, Mehta P, et al. Genotypes of CYP1A1, SULT1A1 and SULT1A2 and risk of squamous cell carcinoma of esophagus: outcome of a case-control study from Kashmir, India. Dis Esophagus 2016;29:937-43. [Crossref] [PubMed]
- Bhat GA, Shah IA, Makhdoomi MA, et al. CYP1A1 and CYP2E1 genotypes and risk of esophageal squamous cell carcinoma in a high-incidence region, Kashmir. Tumour Biol 2014;35:5323-30. [Crossref] [PubMed]
- Malik MA, Upadhyay R, Mittal RD, et al. Association of xenobiotic metabolizing enzymes genetic polymorphisms with esophageal cancer in Kashmir Valley and influence of environmental factors. Nutr Cancer 2010;62:734-42. [Crossref] [PubMed]
- Shang X, Liu G, Zhang Y, et al. Downregulation of BIRC5 inhibits the migration and invasion of esophageal cancer cells by interacting with the PI3K/Akt signaling pathway. Oncol Lett 2018;16:3373-9. [Crossref] [PubMed]
(English Language Editors: A. Kassem and J. Chapnick)