PUM1 is upregulated by DNA methylation to suppress antitumor immunity and results in poor prognosis in pancreatic cancer
Introduction
Pancreatic cancer is the seventh leading cause of cancer-related death in the world, with a 5-year survival rate of only 9% (1). One of the significant reasons for the high mortality is that pancreatic cancer is difficult to diagnose in an early stage, and 80–90% of patients have missed the best opportunity for surgery when they were diagnosed (2). Patients with pancreatic cancer frequently present with nonspecific symptoms such as abdominal pain and weight loss, which can delay diagnosis (3). Pancreatic cancer currently lacks specific methods for early diagnosis.
There is no reliable screening test currently available to screen the general population and detect pancreatic cancer early (4,5). The main tumor markers for clinical diagnosis of pancreatic cancer are carbohydrate antigen 19-9 (CA19-9) and carbohydrate antigen 242 (CA242). However, their sensitivity and specificity are not very satisfying (6-11). CA19-9 is the most commonly used serum-based marker for diagnosis of pancreatic cancer (12,13). However, this biomarker has some limitations. The level of CA19-9 can be normal in patients with localized disease, so the effect of early screening pancreatic carcinoma (PAAD) was not obvious. High CA19-9 levels can also take place in benign diseases, including chronic pancreatitis and benign jaundice (12-14).
Therefore, to explore specific tumor markers for early diagnosis of pancreatic cancer is the key to improve the overall therapeutic effect of patients with pancreatic cancer.
Another important reason for the poor 5-year survival rate is that pancreatic cancer is resistant to current treatment modalities, including chemotherapy, radiotherapy and targeted therapies (15). Immunotherapy has been shown to be effective in a variety of tumors, but pancreatic cancer remains completely resistant to it (16). It has also been reported that the tumor microenvironment critically influence the gene expression of tumor tissues and the clinical outcomes (17-22). An important feature of the tumor immune microenvironment in pancreatic cancer is that there are abundant non-cancer cell components in pancreatic cancer tissue, which constitute the tumor matrix. The tumor matrix accounts for more than 50% of the total tumor mass. Current studies have shown that the pancreatic tumor microenvironment has a high degree of immunosuppression and inhibits the body’s spontaneous and treatment-induced antitumor immunity (23).
Pumilio homologous protein 1 (PUM1) is a sequence-specific RNA binding protein (24,25) that is involved in various physiological processes, such as the cell cycle, DNA repair, and cell renewal (26-29). It has been proven that PUM1 plays an oncogene role in ovarian cancer, non-small-cell lung carcinoma (NSCLC), lymphocyte leukemia and other tumors (30-33). In our previous studies, we also found that the expression of PUM1 is correlated with the stage and prognosis of pancreatic cancer (34).
In this study, we used a variety of bioinformatic tools and analyzed data from patients with pancreatic cancer in The Cancer Genome Atlas (TCGA). We attempt to explore the relationship between expression of PUM1 and prognosis of patients with PAAD, the potential of PUM1 as a diagnostic marker for PAAD and its possible regulatory mechanisms. We present the following article in accordance with the MDAR reporting checklist (available at http://dx.doi.org/10.21037/tcr-20-3295).
Methods
Ethics statement
The study was conducted in accordance with the Declaration of Helsinki (as revised in 2013). The study was approved by Research Ethics Committee of Southwest Hospital, Army Medical University (KY2020138). All the datasets were retrieved from published literature, and written informed consent was confirmed in all studies.
Data preparation
Data on the expression profile of PUM1 mRNA and the corresponding clinical information of the pancreatic cancer samples and normal samples, as well as the tumor subgroup of cancer samples, were obtained from the TCGA (https://www.cancer.gov/about-nci/organization/ccg/research/structural-genomics/tcga) database and Genotype-Tissue Expression (GTEx) (https://gtexportal.org/) database. The TCGA has sequencing and pathological data for 30 different cancers (35). TCGA contained 9,736 carcinoma tissues, and GTEx contained RNA-seq data of 8,587 adjacent non-tumor tissues. The data from TCGA and GTEx are publicly available and open-access. This study follows the TCGA data access policy and published guidelines.
Gene Expression Profiling Interactive Analysis (GEPIA) and Human Protein Atlas (HPA) analysis
The HPA database (https://proteinatlas.org/), Kaplan-Meier plotter survival analysis platform (http://kmplot.com/), and GEPIA database (http://gepia.cancer-pku.cn) were employed to examine PUM1 expression and survival in different cancers, including pancreatic cancer.
GEPIA is a newly developed interactive web server. It uses a standard processing pipeline to analyze the RNA-seq expression data of 9,736 tumors and 8,587 normal samples from the TCGA and GTEx projects. Customizable functions are provided by GEPIA, such as tumor/normal differential expression analysis, profiling according to cancer types or pathological stages, patient survival analysis, similar gene detection, correlation analysis and dimensionality reduction analysis (36). In this study, using GEPIA database, 179 pancreatic cancer samples and 171 normal samples were collected to analyze the expression levels of PUM1 in pancreatic cancer.
BBCancer analysis
The difference in PUM1 mRNA levels of extracellular vesicles in the blood between patients with pancreatic cancer and healthy individuals was analyzed using the BBCancer (http://bbcancer.renlab.org/) database (37). The BBCancer database is derived from the Gene Expression Omnibus (GEO) (https://www.ncbi.nlm.nih.gov/geo/), miRBase V22 (http://www.mirbase.org/), piRNABank (http://pirnabank.ibab.ac.in/), and Minebase V2.0 (https://cm.jefferson.edu/MINTbase/) databases.
cBioPortal and MethSurv analysis
The analyses of PUM1 expression and methylation used cBioPortal (https://www.cbioportal.org/) (38). The genomic profiles involved mutations from GISTIC, Z-scores for mRNA expression (RNA-seq V2 RSEM) and Z-scores for protein expression (from reverse-phase protein array, RPPA). Co-expression and network were calculated on the basis of the online instructions of cBioPortal.
The relationship between the DNA methylation of PUM1 and survival was analyzed using the MethSurv database (https://biit.cs.ut.ee/methsurv/) (39). The MethSurv database is a web-based tool for multivariate survival analysis using DNA methylation data derived from the methylation group data of TCGA and developed using a Cox proportional hazard model, CpG, which allows survival analysis of CpGs located in or adjacent to a query gene and includes 7,358 methylation sites in 25 different cancer types.
The Cancer Imaging Archive (TCIA) and xCell analysis
Using the RNA-seq data of TCIA and xCell gene expression, the CIBERSORT algorithm was used to estimate the proportion of 22 infiltrating immune cells.
The TCIA (https://tcia.at/) includes tumors from 20 solid cancers and uses gene enrichment analysis and deconvolution to calculate the contents of individual immune cells (40). Gene expression of specific immune-related gene sets, composition of tumor-infiltrating immune cells (TIICs), new tumor antigens and cancer lineage antigens, HLA types and tumor heterogeneity can be found. The database is based on the features of infiltrating immune cells that respond to tumor genotypes that determine immunophenoscores (IPSs) and immune escape mechanisms.
xCell (https://xcell.ucsf.edu/) is a network tool that can perform cell type enrichment analysis on gene expression data of 64 immune cells and interstitial cells (41). xCell is a method based on genetic markers, with thousands of pure cell types. The results of xCell analysis were verified through extensive computer simulations and cellular immunophenotyping.
Tumor Immune Estimation Resource (TIMER) database analysis
TIMER is a comprehensive resource which can systematically analyze the immune infiltrates in various cancer types (https://cistrome.shinyapps.io/timer/) (42). A previously published statistical deconvolution method (43) is used by TIMER to infer the abundance of TIICs from gene expression profiles. The TIMER database covers 10,897 samples across 32 cancer types from the TCGA for estimating the abundance of immune infiltrates. We analyzed the expression of PUM1 in different types of cancer and the correlation between PUM1 expression and the abundance of immune infiltrates, including B cells, CD4+ T cells, CD8+ T cells, neutrophils, macrophages, and dendritic cells, via gene modules. The correlation module generated scatter plots of expression between a pair of user-defined genes in a given cancer type, and the Spearman’s correlation and estimated statistical significance of the estimates. LAYN was used for the X-axis with gene symbols, and associated marker genes are represented as gene symbols on the Y-axis. The log2 RSEM is used to show gene expression levels.
Diagnostic prediction
According to the best cut-off value obtained from the receiver operating characteristic (ROC) curve, patients with pancreatic cancer were divided into high- and low-mRNA-expression groups. In addition, the area under the curve (AUC) of the ROC curve was computed to evaluate the predictive power of PUM1 in pancreatic cancer diagnosis. In the case of AUC >0.5, the closer the AUC is to 1, the better the diagnosis.
Statistical methods
All results are presented as the mean ± standard deviation. Excel, GraphPad 7.0. and SPSS 25.0 (IBM, Chicago, IL, USA) were used to analyze the data. Comparisons between two groups were conducted using Student’s t-test. Comparisons among three or more groups were conducted using one-way analysis of variance (ANOVA). Independent-samples t-test was used to statistically analyze the measurement data. The survival data were analyzed using the Kaplan-Meier method. Pearson’s χ2 method was used to determine the correlations between PUM1 expression and clinical parameters. P<0.05 was considered to be statistically significant.
Results
PUM1 mRNA expression correlates with the prognosis of pancreatic cancer patients
We obtained data from the GEPIA database and evaluated the transcription level of PUM1. The data revealed that the mRNA expression of PUM1 in diffuse large B-cell lymphoma, esophageal cancer, pancreatic cancer, gastric cancer and thymoma was significantly higher than that in the corresponding normal tissues. The expression of PUM1 mRNA in pancreatic cancer was 24.64 transcripts per million (TPM) and that in normal pancreas was 8.09 TPM (P<0.01). The fold difference was approximately three (Figure 1A). Figure 1B provides a scatter diagram of the difference in PUM1 mRNA expression between pancreatic tumors and normal tissues. Thus, PUM1 expression may serve as a potential diagnostic indicator in PAAD.
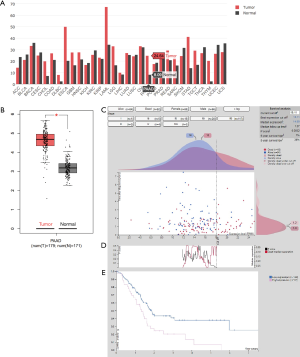
In our previous research, we verified that PUM1 expression in pancreatic cancer is correlated with the prognosis of patients (34). We then explored the prognostic significance of the PUM1 expression level in pancreatic cancer using the HPA database. Figure 1C shows the distribution of the PUM1 expression profiles in the high-expression (7%, n=37) and low-expression (38%, n=139) subgroups in pancreatic cancer. We calculated the best expression cut-off value (18.11%) according to the P score and median expression value to separate the patients into high-expression and low-expression subgroups (Figure 1D). Figure 1E shows that the survival probability of the high-expression group was significantly lower than that of the low-expression group {hazard ratio (HR) [95% confidence interval (CI)] =1.894 (1.131–3.170), log-rank test P value =0.0032). High PUM1 expression was associated with unfavorable prognosis in pancreatic cancer.
The PUM1 mRNA expression level correlates with the incidence of pancreatic cancer
The results of ROC curves showed that PUM1 mRNA expression levels correlated with pancreatic cancer incidence. Detailed ROC results are provided in Figure 2A (AUC value =0.9734, P<0.0001). Our results suggest that PUM1 mRNA levels in tissues can be exploited as useful biomarkers to diagnose pancreatic cancer.
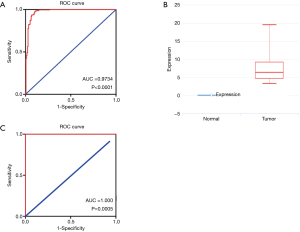
We further analyzed the PUM1 mRNA of extracellular vesicles in the blood. The expression in the patients with pancreatic cancer (n=14) was more than 5 TPM, while that in normal people (n=6) was almost undetectable [log fold change (FC) =6.3264, P<0.0001, Figure 2B]. ROC curve analysis showed that the PUM1 mRNA level of extracellular vesicles in the blood is an excellent diagnostic marker for pancreatic cancer (AUC =1, P=0.0005, Figure 2C).
Association of the DNA methylation level of PUM1 with the prognosis of pancreatic cancer patients
What affects the expression level of PUM1 in pancreatic cancer? Here, we analyzed the association between gene expression and methyltransferase (DNMT) expression in diverse tumors using TCGA expression profile data. As shown in Figure 3A, PUM1 expression in PAAD was positively correlated with the four methyltransferases (DNMT1: red, r=0.52, P<0.0001; DNMT2: blue, r=0.6, P<0.0001; DNMT3A: green, r=0.46, P<0.0001; DNMT3B: purple, r=0.18, P=0.016). We further explored the association between the mRNA expression and methylation status of PUM1 to elucidate potential mechanisms of abnormal upregulation in pancreatic cancer tissues. Analysis of the data from TCGA in the cBioPortal database showed that PUM1 mRNA expression in pancreatic cancer was negatively correlated with methylation of PUM1 (r=–0.29, P<0.0001, Figure 3B). We then investigated the association between PUM1 methylation and patient prognosis using the MethSurv database. The analysis showed that the frequencies of detected sites with low, moderate and high methylation levels were 52.17% (12/23), 8.70% (2/23) and 47.83% (9/23), respectively (Figure 3C). We performed Kaplan-Meier plotter analysis based on the methylation level profiles for the 23 specific sites and the prognosis information. Of a total of 23 methylation sites, 5 (cg04976330, cg23281075, cg04078732, cg0891023, cg08849613) were associated with survival outcomes, as shown in Figure 3D. The other 18 methylation sites are shown in the Figure S1. Hypomethylation was common among with high-risk patients, while hypermethylation was common among with low-risk patients.
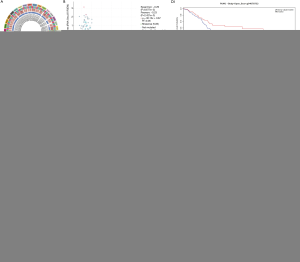
Association of PUM1 expression and the immune microenvironment in pancreatic cancer
How does PUM1 play a role in pancreatic cancer? Analysis of data from the TCGA pancreatic cancer dataset with the xCell web tool showed that increased expression of PUM1 reduced the proportions of common lymphoid progenitors (CLP, P<0.001), natural killer T cells (NKT cells, P<0.0001), type 1 helper T cells (Th1 cells, P<0.0001), and progenitor B cells (pro-B cells, P<0.0001), which play positive roles in immune activation. However, this treatment increased the proportion of regulatory T cells (Tregs, P<0.01), which are inhibitory, and reduced the proportion of epithelial cells, which represents high differentiation and low malignancy (P<0.05, Figure 4A).
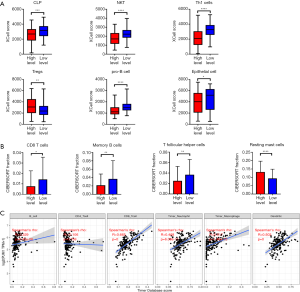
In addition, we use the CIBERSORT algorithm to analyze the data from TCIA. The results showed that the expression of PUM1 reduced the proportion of CD8+ T cells (P<0.05), memory B cells (P<0.01) and follicular helper T cells (Tfh cells) (P<0.01), which play a positive role in immune activation, and increased the proportion of resting mast cells, which are inhibitory immune cells (P<0.0001, Figure 4B).
We validated the results in the TIMER database and found that PUM1 expression was significantly positively correlated with the infiltration of memory B cells (r=0.329, P<0.0001), CD8+ T cells (r=0.665, P<0.0001), neutrophils (r=0.468, P<0.0001), macrophages (r=0.517, P<0.0001) and dendritic cells (r=0.503, P<0.0001) but not CD4+ T cells (r=0.106, P=0.159, Figure 4C).
PUM1 was associated with the IPS, DNA mismatch repair (MMR) and tumor mutation burden (TMB) of pancreatic cancer
What effect does PUM1 have on the immunotherapy of pancreatic cancer? In this study, we evaluated the relationship between the status of 5 MMR genes and PUM1 expression using TCGA expression profile data. As shown in Figure 5A, PUM1 expression was significantly correlated with MLH1, MSH2, MSH6, PMS2, and EPCAM mutations (P<0.001). TCGA data also showed that patients with low PUM1 expression had a higher TMB than those with high expression (P=0.002, Figure 5B). Furthermore, we thoroughly explored the association between IPS and PUM1 mRNA expression levels in pancreatic cancer patients. In order to estimate the potential of PUM1, the IPS, IPS-PD1/PD2/PD-L1, IPS-CTLA4+ PD1/PD2/PD-L1, and IPS-CTLA4 scores were designed as a biomarker for patients. The following scores were significantly increased in the PUM1 low-expression group compared with the high-expression group (Figure 5C): IPS, P<0.001; IPS-CTLA4, P<0.0001; and IPS-CTLA4+ PD1/PD2/PD-L1, P<0.05. However, there was no significant difference in the IPS of patients treated with PD1/PD2/PD-L1 antibody alone between the high- and low-expression groups (P>0.05, Figure 5C).
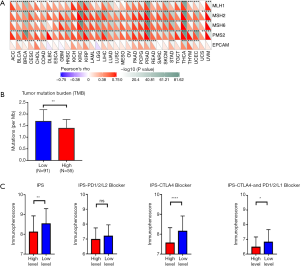
These results indicate that the PUM1 low-expression group has few MMR gene mutations, which means that it is more susceptible to somatic mutations and a high TMB. This group also displayed a higher IPS than the high-expression group, which appeared to reflect a more immunogenic phenotype. All the results above indicate that patients with low PUM1 expression benefit more from immunotherapy than those with high PUM1 expression.
Discussion
Pancreatic cancer is notorious for its poor prognosis and high mortality. On the one hand, patients with pancreatic cancer usually present with nonspecific symptoms, which makes it difficult to detect early. On the other hand, there are no effective systemic treatments for PAAD, including chemotherapies and molecularly targeted therapies (44,45). This fact has motivated us to carry out further research to explore the pathogenesis of PAAD and to mine new diagnostic and prognostic indicators. Only in these ways can the prognosis of patients with pancreatic cancer be rapidly improved.
PUM1 is a sequence-specific RNA binding protein (24,25) that is involved in various physiological processes, such as the cell cycle, DNA repair, and cell renewal (26-29). In our previous work, we verified that the expression of PUM1 is correlated with the stage and prognosis of pancreatic cancer (34). In this study, we further explored the potential of PUM1 as a diagnostic and therapeutic target and investigated its underlying mechanisms in pancreatic cancer.
The main tumor markers in the diagnosis of pancreatic cancer at present are CA242 and CA19-9. However, their diagnostic potential is limited because of their restricted sensitivity and specificity (7-11,46-48). In our study, we found that the AUC was 0.9734 when the PUM1 mRNA expression level in tissues was used as a diagnostic marker in pancreatic cancer, and the AUC was close to 1 when of the PUM1 expression level in extracellular vesicles in the blood was used as a diagnostic marker. By contrast, the AUCs of CA19-9 and CA242 were between 0.7 and 0.85 (7). Therefore, PUM1 could serve as a promising diagnostic marker of pancreatic cancer. However, the sample size is small in our analysis; these findings need further validation in a larger database. In addition, our analysis is based on the data of PUM1 mRNA expression and needs to be verified at the protein level.
We also explored the potential regulatory mechanisms of PUM1 expression. Increased methylation of tumor suppressor genes or decreased methylation of oncogenes can promote tumorigenesis (49-55). Large-scale DNA hypomethylation in the genome affects chromosome stability and genome integrity, resulting in an increased chance of cell cancer (50,51,54,56). DNA methylation is a biological process accomplished by DNMTs, which catalyze the covalent addition of a methyl group to the 5-position of cytosine within the CpG island (43). Here, we analyzed the association between PUM1 and DNMT expression levels in a total of 33 tumors. In PAAD, PUM1 expression was significantly positively correlated with the expression of the four methyltransferases (DNMT1, DNMT2, DNMT3A, DNMT3B). This result is consistent with the negative correlation between the PUM1 methylation level and its mRNA expression level according to analysis of the cBioPortal database. In addition, we analyzed 23 methylation sites of PUM1 in pancreatic cancer, and five (cg04976330, cg23281075, cg04078732, cg0891023, cg08849613) were associated with survival. The methylation level of these five sites can be used as a predictor of prognosis in patients with pancreatic cancer.
How does PUM1 play a role in pancreatic cancer? We found that PUM1 was closely related to the immune microenvironment of PAAD. In addition to the oncogenic role of PUM1, the enriched tumor matrix of pancreatic cancer is also a reason for treatment failure. There are abundant non-cancer cell components in pancreatic cancer tissue, which constitute the tumor matrix. The matrix accounts for more than 50% of the total tumor mass. Current studies have shown that the pancreatic tumor microenvironment has a high degree of immunosuppression and inhibits the body's spontaneous and treatment-induced antitumor immunity (23). The components of the matrix barrier include cellular and chemical components, including fibroblasts, myeloid-derived suppressor cells (MDSCs), tumor-associated macrophages, Tregs and so on. Our study analyzed data from the TCIA and xCell databases and found that Tregs and resting mast cells were more prevalent in the pancreatic cancer tissues of patients with high expression of PUM1 than in those of patients with low expression. These cells are thought to have immunosuppressive effects. Moreover, the number of CD8+ T cells, NKT cells, Tfh cells and memory B cells, which can exert antitumor effects, was small. The suppressive cellular components in the immune microenvironment of pancreatic cancer, on the one hand, form a barrier by producing extracellular matrix, making pancreatic cancer tissue dense and blocking the entry of effector cells and drugs that clear the tumor. On the other hand, the secretion of suppressive cytokines inactivates and depletes killer cells infiltrating the tumor microenvironment so that they cannot kill tumor cells. The infiltration of immune cells in the microenvironment of cancer is an important predictor of prognosis (57,58). Therefore, PUM1 affects the prognosis of patients by suppressing immune cells in the microenvironment.
In addition to the immune microenvironment, PUM1 also has an impact on the efficacy of immunotherapy. Cancer is a genetic disease, which is associated with the accumulation of mutations. Accurate DNA replication and the repair of DNA damage are significant aspects for genome maintenance. DNA MMR was one of the first DNA repair pathways related to cancer predisposition (59). MMR-deficient tumors have the highest mutation rates among all types of cancer types (60). The mutation of DNA MMR genes is associated with a high TMB (61-63). Current studies have shown that a high TMB results in more antigen site exposure, which is more conducive to T cell recognition and tumor cell killing. The higher the TMB is, the better the response to immunocheckpoint therapies, such as those targeting PD-1/PD-L1, and the greater the overall benefit from immunotherapy (64,65). Using TCGA expression profile data, we found that PUM1 expression was significantly positively correlated with the status of five MMR genes (MLH1, MSH2, MSH6, PMS2, EPCAM mutations). Consequently, patients with high expression of PUM1 lack MMR genes, which leads to a high TMB. Hence, the IPS, IPS-CTLA4, and IPS-PD1/PD2/PD-L1+ CTLA4 scores were markedly decreased in these patients compared to patients with low expression of PUM1, indicating a worse prognosis. These results suggest that one of the reasons for poor prognosis in patients with high expression of PUM1 is that PUM1 enhances the inhibition of the tumor immune microenvironment in pancreatic cancer.
Overall, in this study, we verified that PUM1 is an oncogene that can inhibit the immune microenvironment and lead to poor prognosis in pancreatic cancer. We provided a novel perspective that PUM1 could be utilized as a diagnostic marker for pancreatic cancer and showed that PUM1 expression was affected by DNA methylation. Online tools, which were on the basis of the most popular bioinformatics theories, were used by us to perform target gene analyses of tumor data from public databases. Compared with traditional chip screening, the advantages of this method include a large sample size, low cost, and simple methods. However, this study still has some limitations. First, all the data were retrieved from public databases, and our findings are required to be verified by external validation. Second, the PAAD samples in the TCGA database are mainly from Caucasians. The etiology and genetic background of PAAD can vary significantly among different ethnic groups. Third, most PAAD samples included in the study were predominantly from early-stage patients who can be treated with surgery. However, in the clinical reality, most PAAD patients are first diagnosed with a disease that is advanced and has an extremely poor prognosis. Data on the level of PUM1 expression in advanced-stage pancreatic cancer are limited. These questions should be addressed in multicenter, large-sample studies, in which clinical samples covering different ethnic groups and PAAD stages should be included.
Acknowledgments
We thank the hospital leaders for their support of our work. We thank Jennifer P. and Courey A. of AJE for English language editing.
Funding: This study was financially supported by
Footnote
Reporting Checklist: The authors have completed the MDAR reporting checklist. Available at http://dx.doi.org/10.21037/tcr-20-3295
Conflicts of Interest: All authors have completed the ICMJE uniform disclosure form (available at http://dx.doi.org/10.21037/tcr-20-3295). The authors have no conflicts of interest to declare.
Ethical Statement: The authors are accountable for all aspects of the work in ensuring that questions related to the accuracy or integrity of any part of the work are appropriately investigated and resolved. The study was conducted in accordance with the Declaration of Helsinki (as revised in 2013). The study was approved by Research Ethics Committee of Southwest Hospital, Army Medical University (KY2020138). All the datasets were retrieved from published literature, and written informed consent was confirmed in all studies.
Open Access Statement: This is an Open Access article distributed in accordance with the Creative Commons Attribution-NonCommercial-NoDerivs 4.0 International License (CC BY-NC-ND 4.0), which permits the non-commercial replication and distribution of the article with the strict proviso that no changes or edits are made and the original work is properly cited (including links to both the formal publication through the relevant DOI and the license). See: https://creativecommons.org/licenses/by-nc-nd/4.0/.
References
- Bray F, Ferlay J, Soerjomataram I, et al. Global cancer statistics 2018: GLOBOCAN estimates of incidence and mortality worldwide for 36 cancers in 185 countries. CA Cancer J Clin 2018;68:394-424. [Crossref] [PubMed]
- Rawla P, Sunkara T, Gaduputi V. Epidemiology of pancreatic cancer: global trends, etiology and risk factors. World J Oncol 2019;10:10-27. [Crossref] [PubMed]
- Chu LC, Goggins MG, Fishman EK. Diagnosis and detection of pancreatic cancer. Cancer J 2017;23:333-42. [Crossref] [PubMed]
- Greenhalf W, Grocock C, Harcus M, et al. Screening of high-risk families for pancreatic cancer. Pancreatology 2009;9:215-22. [Crossref] [PubMed]
- Shin EJ, Canto MI. Pancreatic cancer screening. Gastroenterol Clin North Am 2012;41:143-57. [Crossref] [PubMed]
- Duraker N, Hot S, Polat Y, et al. CEA, CA 19-9, and CA 125 in the differential diagnosis of benign and malignant pancreatic diseases with or without jaundice. J Surg Oncol 2007;95:142-7. [Crossref] [PubMed]
- Gui JC, Yan WL, Liu XD. CA19-9 and CA242 as tumor markers for the diagnosis of pancreatic cancer: a meta-analysis. Clin Exp Med 2014;14:225-33. [Crossref] [PubMed]
- Kawa S, Tokoo M, Hasebe O, et al. Comparative study of CA242 and CA19-9 for the diagnosis of pancreatic cancer. Br J Cancer 1994;70:481-6. [Crossref] [PubMed]
- Su SB, Qin SY, Chen W, et al. Carbohydrate antigen 19-9 for differential diagnosis of pancreatic carcinoma and chronic pancreatitis. World J Gastroenterol 2015;21:4323-33. [Crossref] [PubMed]
- Dou H, Sun G, Zhang L. CA242 as a biomarker for pancreatic cancer and other diseases. Prog Mol Biol Transl Sci 2019;162:229-39. [Crossref] [PubMed]
- Bauer TM, El-Rayes BF, Li X, et al. Carbohydrate antigen 19-9 is a prognostic and predictive biomarker in patients with advanced pancreatic cancer who receive gemcitabine-containing chemotherapy: a pooled analysis of 6 prospective trials. Cancer 2013;119:285-92. [Crossref] [PubMed]
- Zhang S, Wang YM, Sun CD, et al. Clinical value of serum CA19-9 levels in evaluating resectability of pancreatic carcinoma. World J Gastroenterol 2008;14:3750-3. [Crossref] [PubMed]
- Goonetilleke KS, Siriwardena AK. Systematic review of carbohydrate antigen (CA 19-9) as a biochemical marker in the diagnosis of pancreatic cancer. Eur J Surg Oncol 2007;33:266-70. [Crossref] [PubMed]
- Sawabu N, Watanabe H, Yamaguchi Y, et al. Serum tumor markers and molecular biological diagnosis in pancreatic cancer. Pancreas 2004;28:263-7. [Crossref] [PubMed]
- Neoptolemos JP, Kleeff J, Michl P, et al. Therapeutic developments in pancreatic cancer: current and future perspectives. Nat Rev Gastroenterol Hepatol 2018;15:333-48. [Crossref] [PubMed]
- Balachandran VP, Beatty GL, Dougan SK. Broadening the impact of immunotherapy to pancreatic cancer: challenges and opportunities. Gastroenterology 2019;156:2056-72. [Crossref] [PubMed]
- Cooper LAD, Gutman DA, Chisolm C, et al. The tumor microenvironment strongly impacts master transcriptional regulators and gene expression class of glioblastoma. Am J Pathol 2012;180:2108-19. [Crossref] [PubMed]
- Curry JM, Sprandio J, Cognetti D, et al. Tumor microenvironment in head and neck squamous cell carcinoma. Semin Oncol 2014;41:217-34. [Crossref] [PubMed]
- Galon J, Pagès F, Marincola FM, et al. The immune score as a new possible approach for the classification of cancer. J Transl Med 2012;10:1. [Crossref] [PubMed]
- Yoshihara K, Shahmoradgoli M, Martínez E, et al. Inferring tumour purity and stromal and immune cell admixture from expression data. Nat Commun 2013;4:2612. [Crossref] [PubMed]
- Şenbabaoğlu Y, Gejman RS, Winer AG, et al. Tumor immune microenvironment characterization in clear cell renal cell carcinoma identifies prognostic and immunotherapeutically relevant messenger RNA signatures. Genome Biol 2016;17:231. Erratum in: Genome Biol 2017;18:46. [Crossref] [PubMed]
- Winslow S, Lindquist KE, Edsjö A, et al. The expression pattern of matrix-producing tumor stroma is of prognostic importance in breast cancer. BMC Cancer 2016;16:841. [Crossref] [PubMed]
- Fan JQ, Wang MF, Chen HL, et al. Current advances and outlooks in immunotherapy for pancreatic ductal adenocarcinoma. Mol Cancer 2020;19:32. [Crossref] [PubMed]
- Spassov DS, Jurecic R. Cloning and comparative sequence analysis of PUM1 and PUM2 genes, human members of the Pumilio family of RNA-binding proteins. Gene 2002;299:195-204. [Crossref] [PubMed]
- Spassov DS, Jurecic R. The PUF family of RNA-binding proteins: does evolutionarily conserved structure equal conserved function? IUBMB Life 2003;55:359-66. [Crossref] [PubMed]
- Treiber T, Treiber N, Plessmann U, et al. A compendium of RNA-binding proteins that regulate microRNA biogenesis. Mol Cell 2017;66:270-84.e13. [Crossref] [PubMed]
- Gennarino VA, Singh RK, White JJ, et al. Pumilio1 haploinsufficiency leads to SCA1-like neurodegeneration by increasing wild-type Ataxin1 levels. Cell 2015;160:1087-98. [Crossref] [PubMed]
- Lee S, Kopp F, Chang TC, et al. Noncoding RNA NORAD regulates genomic stability by sequestering PUMILIO proteins. Cell 2016;164:69-80. [Crossref] [PubMed]
- Kedde M, van Kouwenhove M, Zwart W, et al. A Pumilio-induced RNA structure switch in p27-3’ UTR controls miR-221 and miR-222 accessibility. Nat Cell Biol 2010;12:1014-20. [Crossref] [PubMed]
- Guan X, Chen S, Liu Y, et al. PUM1 promotes ovarian cancer proliferation, migration and invasion. Biochem Biophys Res Commun 2018;497:313-8. [Crossref] [PubMed]
- Guan X, Zong ZH, Liu Y, et al. circPUM1 promotes tumorigenesis and progression of ovarian cancer by sponging miR-615-5p and miR-6753-5p. Mol Ther Nucleic Acids 2019;18:882-92. [Crossref] [PubMed]
- Xia LH, Yan QH, Sun QD, et al. MiR-411-5p acts as a tumor suppressor in non-small cell lung cancer through targeting PUM1. Eur Rev Med Pharmacol Sci 2018;22:5546-53. [PubMed]
- Naudin C, Hattabi A, Michelet F, et al. PUMILIO/FOXP1 signaling drives expansion of hematopoietic stem/progenitor and leukemia cells. Blood 2017;129:2493-506. [Crossref] [PubMed]
- Dai H, Shen K, Yang Y, et al. PUM1 knockdown prevents tumor progression by activating the PERK/eIF2/ATF4 signaling pathway in pancreatic adenocarcinoma cells. Cell Death Dis 2019;10:595. [Crossref] [PubMed]
- Cancer Genome Atlas Network. Comprehensive molecular portraits of human breast tumours. Nature 2012;490:61-70. [Crossref] [PubMed]
- Tang Z, Li C, Kang B, et al. GEPIA: a web server for cancer and normal gene expression profiling and interactive analyses. Nucleic Acids Res 2017;45:W98-102. [Crossref] [PubMed]
- Zuo Z, Hu H, Xu Q, et al. BBCancer: an expression atlas of blood-based biomarkers in the early diagnosis of cancers. Nucleic Acids Res 2020;48:D789-96. [PubMed]
- Cerami E, Gao J, Dogrusoz U, et al. The cBio cancer genomics portal: an open platform for exploring multidimensional cancer genomics data. Cancer Discov 2012;2:401-4. [Crossref] [PubMed]
- Modhukur V, Iljasenko T, Metsalu T, et al. MethSurv: a web tool to perform multivariable survival analysis using DNA methylation data. Epigenomics 2018;10:277-88. [Crossref] [PubMed]
- Charoentong P, Finotello F, Angelova M, et al. Pan-cancer immunogenomic analyses reveal genotype-immunophenotype relationships and predictors of response to checkpoint blockade. Cell Rep 2017;18:248-62. [Crossref] [PubMed]
- Aran D, Hu Z, Butte AJ. xCell: digitally portraying the tissue cellular heterogeneity landscape. Genome Biol 2017;18:220. [Crossref] [PubMed]
- Li T, Fan J, Wang B, et al. TIMER: A web server for comprehensive analysis of tumor-infiltrating immune cells. Cancer Res 2017;77:e108-10. [Crossref] [PubMed]
- Li B, Severson E, Pignon J-C, et al. Comprehensive analyses of tumor immunity: implications for cancer immunotherapy. Genome Biol 2016;17:174. [Crossref] [PubMed]
- Garrido-Laguna I, Hidalgo M. Pancreatic cancer: from state-of-the-art treatments to promising novel therapies. Nat Rev Clin Oncol 2015;12:319-34. [Crossref] [PubMed]
- Hidalgo M. Pancreatic cancer. N Engl J Med 2010;362:1605-17. [Crossref] [PubMed]
- Haglund C, Lindgren J, Roberts PJ, et al. Tissue expression of the tumour associated antigen CA242 in benign and malignant pancreatic lesions. A comparison with CA 50 and CA 19-9. Br J Cancer 1989;60:845-51. [Crossref] [PubMed]
- Yang XQ, Chen C, Peng CW, et al. Carbohydrate antigen 242 highly consists with carbohydrate antigen 19-9 in diagnosis and prognosis of colorectal cancer: study on 185 cases. Med Oncol 2012;29:1030-6. [Crossref] [PubMed]
- Li X, Guo X, Li H, et al. Serum carbohydrate antigen 242 expression exerts crucial function in the diagnosis of pancreatic cancer. Tumour Biol 2014;35:5281-6. [Crossref] [PubMed]
- Goelz SE, Vogelstein B, Hamilton SR, et al. Hypomethylation of DNA from benign and malignant human colon neoplasms. Science 1985;228:187-90. [Crossref] [PubMed]
- Hernandez-Blazquez FJ, Habib M, Dumollard JM, et al. Evaluation of global DNA hypomethylation in human colon cancer tissues by immunohistochemistry and image analysis. Gut 2000;47:689-93. [Crossref] [PubMed]
- Novik KL, Nimmrich I, Genc B, et al. Epigenomics: genome-wide study of methylation phenomena. Curr Issues Mol Biol 2002;4:111-28. [PubMed]
- Bariol C, Suter C, Cheong K, et al. The relationship between hypomethylation and CpG island methylation in colorectal neoplasia. Am J Pathol 2003;162:1361-71. [Crossref] [PubMed]
- Florl AR, Steinhoff C, Müller M, et al. Coordinate hypermethylation at specific genes in prostate carcinoma precedes LINE-1 hypomethylation. Br J Cancer 2004;91:985-94. [Crossref] [PubMed]
- Brothman AR, Swanson G, Maxwell TM, et al. Global hypomethylation is common in prostate cancer cells: a quantitative predictor for clinical outcome? Cancer Genet Cytogenet 2005;156:31-6. [Crossref] [PubMed]
- Suzuki MM, Bird A. DNA methylation landscapes: provocative insights from epigenomics. Nat Rev Genet 2008;9:465-76. [Crossref] [PubMed]
- Lim DHK, Maher ER. Genomic imprinting syndromes and cancer. Adv Genet 2010;70:145-75. [Crossref] [PubMed]
- Yang S, Liu T, Cheng Y, et al. Immune cell infiltration as a biomarker for the diagnosis and prognosis of digestive system cancer. Cancer Sci 2019;110:3639-49. [Crossref] [PubMed]
- Mlecnik B, Bindea G, Angell HK, et al. Integrative analyses of colorectal cancer show immunoscore is a stronger predictor of patient survival than microsatellite instability. Immunity 2016;44:698-711. [Crossref] [PubMed]
- Baretti M, Le DT. DNA mismatch repair in cancer. Pharmacol Ther 2018;189:45-62. [Crossref] [PubMed]
- Meier B, Volkova NV, Hong Y, et al. Mutational signatures of DNA mismatch repair deficiency in C. elegans and human cancers. Genome Res 2018;28:666-75. [Crossref] [PubMed]
- Shlien A, Campbell BB, de Borja R, et al. Combined hereditary and somatic mutations of replication error repair genes result in rapid onset of ultra-hypermutated cancers. Nat Genet 2015;47:257-62. [Crossref] [PubMed]
- Tetzlaff MT, Singh RR, Seviour EG, et al. Next-generation sequencing identifies high frequency of mutations in potentially clinically actionable genes in sebaceous carcinoma. J Pathol 2016;240:84-95. [Crossref] [PubMed]
- Stadler ZK, Battaglin F, Middha S, et al. Reliable detection of mismatch repair deficiency in colorectal cancers using mutational load in next-generation sequencing panels. J Clin Oncol 2016;34:2141-7. [Crossref] [PubMed]
- Robert C, Long G V, Brady B, et al. Nivolumab in previously untreated melanoma without BRAF mutation. N Engl J Med 2015;372:320-30. [Crossref] [PubMed]
- Yarchoan M, Hopkins A, Jaffee EM. Tumor mutational burden and response rate to PD-1 inhibition. N Engl J Med 2017;377:2500-1. [Crossref] [PubMed]