Construction of five microRNAs prognostic markers and a prognostic model for clear cell renal cell carcinoma
Introduction
In western countries, renal cell carcinoma (RCC) has become the seventh-largest tumor in men and the third most common malignant tumor of the genitourinary system; its incidence is increasing at 2–4% (1,2). RCC includes a variety of histological types. CcRCC is the most aggressive, carries the poorest outcome, and is the most common, accounting for nearly 90% of all malignant renal tumors (1,3). About 30% of RCC patients had advanced disease at the initial diagnosis; the 5-year survival rate was only 11% (4). Chemotherapy or radiotherapy does not affect CcRCC, and the overall clinical effect is not good (5,6). There are surgical and targeted therapies for the treatment of RCC that improve the overall survival rate of patients (7). However, most patients eventually died of the disease because of the ineffective treatment. Therefore, there is an urgent need to use diagnostic and prognostic biomarkers in ccRCC. Nevertheless, to the best of our knowledge, ccRCC has no biomarkers used in routine clinical practice.
MicroRNAs (miRNAs) are single-stranded non-coding RNAs, small endogenous RNAs that regulate gene expression (8-10). Several lines of evidence showed that miRNA is an essential regulator of gene expression. Nevertheless, their function in this type of tumorigenesis remains elusive. With the development of gene sequencing, next-generation sequencing (NGS), and other related technologies, the abnormal expression of miRNA in ccRCC has been widely studied. Systematic and comprehensive analysis of multiple microRNA expression data sets might reveal the potential mechanism of microRNA involved in the pathogenesis and outcome of ccRCC (11-13).
MiRNA is an essential part of the non-coding RNA family, consisting of about 18–25 nucleotides. Because miRNA is a crucial regulator of gene expression and a promising candidate for the development of biomarkers, research on the progression and outcome of tumor patients is expanding. With the further study of genes, many significant miRNAs have been found. Previous studies have shown that miRNAs regulate the progression of a variety of tumors by affecting oncogenes or tumor suppressor genes, including cell proliferation, angiogenesis, differentiation, and apoptosis (14). A single miRNA can target hundreds of mRNA and affect the expression of many genes involved in functional interaction pathways. Abnormal miRNA expression changes are associated with human malignant tumors (15,16). miRNA mimics and inhibitors in clinical development have shown hope as therapeutic agents. Several miRNAs have shown the potential to target tumor outcome pathways and develop into new therapeutic targets (17-20). The miRNA prognostic model of ccRCC has been constructed by using TCGA database (21). Nevertheless, the independence and verification evaluation of the miRNAs model needs to be further improved. We present the following article in accordance with the STROBE reporting checklist (available at http://dx.doi.org/10.21037/tcr-21-37).
Methods
Raw data
This study’s original data came from the cancer genome map TCGA (https://tcga-data.nci.nih.gov/tcga/), which did not violate relevant ethical norms. The Strawberry Perl (Version 5.30.0.1) software package was used to organize these text records and clinical data, and the collated text records and clinical data were obtained. The expression of miRNA in 72 normal samples and 539 tumor samples (ccRCC) and miRNA expression in 71 normal samples and 545 tumor samples were included. The clinical data are found in Table 1, including information on 537 patients. The study data are from the National Cancer Institute (NCI) and the National Human Genome Institute (NHGRI), without the need for an ethical statement. The study was conducted in accordance with the Declaration of Helsinki (as revised in 2013).
Table 1
Factors | Cases | Percentage (%) |
---|---|---|
Gender | ||
Female | 191 | 0.356 |
Male | 346 | 0.644 |
Age(years) | ||
≥60 | 290 | 0.540 |
<60 | 247 | 0.460 |
Clinical stage | ||
Stage I | 269 | 0.500 |
Stage II | 57 | 0.106 |
Stage III | 125 | 0.233 |
Stage IV | 83 | 0.155 |
Unknow | 3 | 0.006 |
T stage | ||
T1(T1+T1a+T1b) | 275 | 0.512 |
T2(T2+T2a+T2b) | 69 | 0.128 |
T3(T3+T3a+T3b+T3c) | 182 | 0.339 |
T4 | 11 | 0.021 |
Lymph node status | ||
N0 | 240 | 0.447 |
N1 | 17 | 0.032 |
Nx | 280 | 0.521 |
Metastatic | ||
M0 | 426 | 0.793 |
M1 | 79 | 0.147 |
Mx | 30 | 0.056 |
Unknow | 2 | 0.004 |
mRNA/miRNAs difference analysis and combination of miRNAs and survival data
The differential expression of miRNAs (normal: 72, tumor: 539) and mRNAs (normal: 71, tumor: 545) was analyzed using R Language 3.6.1, Edge R, and P heatmap software packages. We screened out the differential genes through relevant filtering conditions (FDR <0.05 and |log2FC| >1). The differential genes were shown using heat maps and volcano maps (Figure 1). The differentially expressed miRNAs and mRNA were combined with clinical data (survival time ≥30 days).
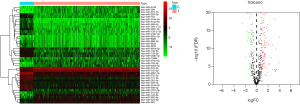
Construction of prediction model
Using R Language, 3.6.1 version “survival, caret, glmnet, survminer, survivalROC” software packages, the survival data samples (including survival time and survival status) differentially expressed miRNA were randomly divided into a training group and experimental group with a 1:1 ratio. miRNA expression was extracted from training and experimental groups, and univariate Cox was used to analyzing the training and survival data. miRNA related to the outcome was screened (P<0.001). The prognostic model was established using miRNA multivariate Cox analysis. The risk value of each patient in the train group and the test group was calculated according to the constructed model function, and the risk value of each patient was calculated as follows: (Risk Score = hsa-miR-155-5p×0.1821+hsa-miR-149-5p×0.2452+hsa-miR-365b-3p×0.5473+hsa-miR-144-5p×−0.2628+hsa-miR-130b-3p×0.2786). This process used the packages “survival”, “caret”, “glmnet”, “survminer”, and “survivalROC”.
A series of analyses verify the accuracy of the model
We used R Language version 3.6.1 to verify the prognostic model and determined whether the model effectively predicted survival. We verified it by drawing survival curves, ROC curves, and independent outcome analyses. The process involved the R software packages “survival” and “survivalROC”.
Prediction, functional enrichment analysis, and potential function of five miRNA target genes
After we obtained five model-related miRNA genes, we predicted the target genes of these miRNAs. The miRNA forecast database was downloaded from the miRTarBase, miRDB, and targetScan websites. We defined at least two positive genes on the website because of the target gene and then constructed the miRNA regulatory network. To further study the functional annotations and signal pathways related to the target genes, we analyzed the functional enrichment of GO and KEGG. We used the STRING database (http://www.string-db.org/) to build a protein interaction (PPI) data network. The process involved the R packages “colorspace”, “stringi” and “ggplot2”.
Statistical software
We used Perl language (10.0.18362.592), R language version 3.6.1, and its accompanying software packages. The differential genes were screened by Edge R and P Heatmap software packages. Use the “Survival”, “Insert”, “glmnet”, “Survminer”, “Survminer”, “SurvivalROC” package to filter out the miRNA of related to the results (P<0.001). Use R package “Colorspace”, “stri” and “ggplot2” to enrich the functions of GO and KEGG.
Results
MiRNA variance analysis.
The differential expression of miRNA was analyzed using R Language 3.6.1, EdgeR, and P heatmap software packages. We obtained 211 differentially expressed miRNAs, of which 115 genes were upregulated and 96 genes were downregulated in the tumor group (Figure 1). For details of the 211 differentially expressed miRNA (5p and 3p), see Table S1.
Construction of prognostic model using differentially expressed miRNA
According to the median of miRNAs expression, patients were divided into an experimental group and a training group at 1:1, circulation analysis was carried out, and the respective expression levels of the two groups were extracted simultaneously. Univariate Cox regression analysis was carried out in the training group. The relationships between each miRNA, survival time, survival state were compared in turn, and 11 miRNAs with prognostic value were selected (P<0.05). To further screen the miRNA, in the model to eliminate the inherent correlation between each miRNA, we then carried out a multi-factor Cox regression analysis, and finally identified five miRNAs (P<0.05): miR-130b-3p (Figure 2A), miR-365b-3p (Figure 2B), miR-149-5p (Figure 2C), miR-155-5p (Figure 2D), and miR-144-5p (Figure 2E). We used these five miRNAs to construct a prognostic model: Risk score = miR-130b-3p*0.278+ miR-365b-3p*0.547 + miR-149-5p*0.245 + miR-155-5p*0.182 + miR-144-5p*-0.262 (Table 2). The Kaplan-Meier survival analysis of the five differential miRNAs is shown in Figure 2. The results showed a significant difference in the high- and low-expression of the five prognostic miRNAs in ccRCC; the survival rate in the high-expression group was lower than that in the low-expression group, suggesting that these five miRNAs are risk factors for the occurrence of ccRCC.
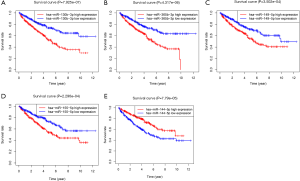
Table 2
ID | Coef | HR | HR.95L | HR.95H | P value |
---|---|---|---|---|---|
hsa-miR-155-5p | 0.1821 | 1.19978 | 1.01175 | 1.42275 | 0.03623 |
hsa-miR-149-5p | 0.2452 | 1.27788 | 1.06306 | 1.53613 | 0.00902 |
hsa-miR-365b-3p | 0.5473 | 1.72875 | 1.30848 | 2.28399 | 0.00011 |
hsa-miR-144-5p | −0.2628 | 0.76882 | 0.66296 | 0.89158 | 0.00050 |
hsa-miR-130b-3p | 0.2786 | 1.32131 | 1.00985 | 1.72883 | 0.04221 |
A series of analysis and verification models carried out for the training group, the test group, and the whole group
We based the risk model on the calculation formula obtained using the training group. However, the training group and the verification group were still grouped 1:1, and each patient in the two groups obtained the corresponding risk value through the calculation formula, and was divided into high-risk and low-risk groups according to the median risk value. The survival curve of risk value was obtained by comparing the survival difference between the high-risk and the low-risk groups. Whether it was the training group, the verification group, or the whole group, the risk value of the patients was calculated according to the model constructed by the training group. In Figure 3A,B,C of the training group (P=1.893E-07), test group (P=8.046E-07), and the whole group (P=4.814E-13), there was a significant difference in survival rate between the high-risk and low-risk groups. The overall survival rate of the high-risk group was much lower than that of the low-risk group. Then we wanted to verify the accuracy of our model prediction and draw the ROC curve. The area under the curve (AUC) values under the curve in the training group, the experimental group, and the whole group were 0.781, 0.728, and 0.757, respectively (Figure 3D,E,F). This shows that the model has high accuracy in predicting the survival of patients with ccRCC. Also, by drawing the survival status chart, we can see the number and proportion of dying patients in the high-risk and low-risk groups. The high-risk group's mortality rate was significantly higher than that of the low-risk group (Figure 3G,H,I).
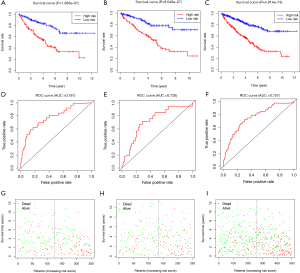
Independence of prognostic models compared with other clinical traits
The Survival software package of R Language 3.6.1 was used to analyze the independence of the risk value of the model compared with the clinical features, draw the ROC curve, and evaluate the accuracy of the prognostic model prediction according to the AUC value. From the curve, the AUC of the risk score was 0.724, which is much higher than that of other clinical indicators, suggesting that the risk score value of the prognostic model was more accurate than other clinical indicators for predicting outcome. The feasibility of the model was further verified from the side (Figure 4A). Then we carried out univariate Cox regression analysis to compare the correlation between clinical factors, risk value, survival time, and survival status. All patients except gender had a significant correlation with the outcome (P<0.05). The risk value was as follows (HR =1.13947, 95% CI: 1.096–1.185, P=6.9662e-11, Figure 4B). Then multivariate Cox regression analysis was conducted to analyze the correlation between clinical factors, risk value, and survival. The results showed that risk value and age were significantly related to outcome (risk value HR =1.077, 95% CI: 1.028–1.128, P=0.002, Figure 4C). From this, we determined that risk value and age were independent prognostic factors. ROC curves, univariate and multivariate prognostic analyses showed that the risk value of the model was significantly correlated with outcome (P<0.05), demonstrating that the model can be used as an independent prognostic tool.
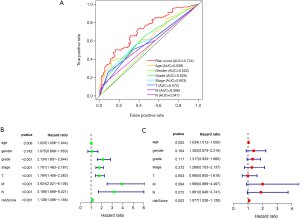
Prediction and functional enrichment analysis of five miRNA target genes
To study the potential biological functions of these five miRNAs, we used three target gene prediction software packages (miRTarBase, miRDB, and targetScan) to predict their target genes. If there were two or more target gene prediction software that supported the gene as the target gene, we assumed that the gene might be the target gene of miRNA. Using these three prediction software packages, a total of 3,015 target genes of five miRNAs were predicted. A Venn diagram was used to draw the relationship between miRNA and these target genes. MiR-130b-3p (Figure 5A), miR-155-5p (Figure 5B), miR-365b-3p (Figure 5C), miR-149-5p (Figure 5D), and miR-144-5p (Figure 5E) had 207, 232, 21, 41 and two overlapping genes, respectively. Then, the target genes were intersected with the differential genes of TCGA, and an miRNA-mRNA regulatory network was constructed using Cytoscape 3.7.2 (Figure 6). To further study the functional annotation and signal pathway related to the target gene, it was necessary to transform the name of the gene into the ID of the gene and then to carry on the functional enrichment analysis of GO and KEGG to understand the main functions and pathways of the genes in the network. The results of functional enrichment of GO were as follows: biological process (BP), cellular component (CC), and molecular functional (MF) are shown by bubble diagram (Figure 7A,B,C). The results of the KEGG pathway enrichment analysis (Figure 7D,E,F) of target genes showed that target genes were involved in a series of pathways related to clear renal cells (Table 3) (P<0.05).
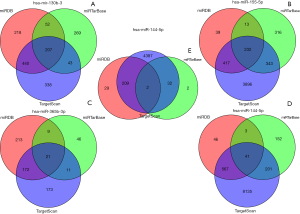
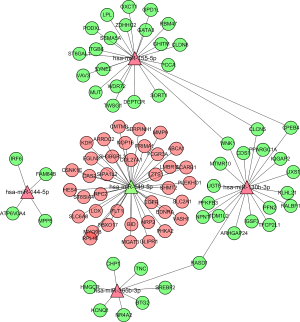
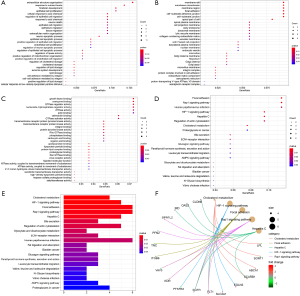
Table 3
ID | Description | BgRatio | P value | Gene ID | Count |
---|---|---|---|---|---|
hsa04979 | Cholesterol metabolism | 50/8011 | 0.0002 | LPL/SORT1/ABCA1/SCAR1 | 4 |
hsa04066 | HIF-1 signaling pathway | 109/8011 | 0.000 | EGLN3/SLC2A1/FLT1/EGFR/PFKFB3 | 5 |
hsa04510 | Focal adhesion | 199/8011 | 0.001 | KDR/VAV3/ITGB6/FLT1/TNC/EGFR | 6 |
hsa04015 | Rap1 signaling pathway | 210/8011 | 0.002 | PFN2/KDR/VAV3/FLT1/SIPA1L2/EGFR | 6 |
hsa05160 | Hepatitis C | 155/8011 | 0.003 | BID/OAS2/CLDN8/EGFR/SCARB1 | 5 |
hsa04976 | Bile secretion | 72/8011 | 0.011 | SLC2A1/HMGCR/SCARB1 | 3 |
hsa04810 | Regulation of actin cytoskeleton | 213/8011 | 0.011 | IQGAP2/PFN2/VAV3/ITGB6/EGFR | 5 |
hsa00630 | Glyoxylate and dicarboxylate metabolism | 30/8011 | 0.016 | SHMT2/PCCA | 2 |
hsa04512 | ECM-receptor interaction | 88/8011 | 0.019 | ITGB6/NPNT/TNC | 3 |
hsa05165 | Human papillomavirus infection | 330/8011 | 0.019 | ITGB6/TNC/HES4/ATP6V0A4/MPP5/EGFR | 6 |
hsa04975 | Fat digestion and absorption | 41/8011 | 0.028 | ABCA1/SCARB1 | 2 |
hsa05219 | Bladder cancer | 41/8011 | 0.028 | MMP9/EGFR 2 | 2 |
hsa04922 | Glucagon signaling pathway | 106/8011 | 0.031 | PPARGC1A/SLC2A1/PHKA2 | 3 |
hsa04928 | Parathyroid hormone synthesis | 106/8011 | 0.031 | NR4A2/GATA3/EGFR | 3 |
hsa04670 | Leukocyte transendothelial migration | 112/8011 | 0.035 | VAV3/MMP9/CLDN8 | 3 |
hsa00280 | Valine, leucine and isoleucine degradation | 48/8011 | 0.038 | OXCT1/PCCA | 2 |
hsa00510 | N-Glycan biosynthesis | 50/8011 | 0.041 | ST6GAL1/MGAT3 | 2 |
hsa05110 | Vibrio cholerae infection | 50/8011 | 0.041 | KCNQ1/ATP6V0A4 | 2 |
hsa04152 | AMPK signaling pathway | 120/8011 | 0.042 | PPARGC1A/HMGCR/PFKFB3 | 3 |
hsa05205 | Proteoglycans in cancer | 204/8011 | 0.042 | KDR/VAV3/MMP9/EGFR | 4 |
Construction of PPI Network and batch survival analysis of target genes
mRNA (expression quantity of all target genes) was combined with survival time and survival status data. According to the median of mRNA expression, these were divided into high-risk and low-risk groups. The relationships between target gene expression and outcome were compared. To make the relationship between the target gene and outcome most significant, we set P<0.00001. Finally, we obtained 12 target genes most closely related to outcome (ARHGAP24, CLCN5, IQGAP2, IRF6, MUT, MPP5, PCCA, PODXL, SEMA5A, SH3BGRL3, VAV3, and WDR72), as shown in Figure 8. The corresponding miRNA of these 12 target genes are shown in Table 4. Among them, the outcome of target gene SH3BGRL3 was better in the low-expression group; the five-year survival rate was significantly higher than that of the high-expression group, the outcome of the other 11 target genes was better in the high-expression group, and the five-year survival rate was significantly higher than that of the low-expression group. Then the protein-protein interaction network (Figure 9A) was constructed using Cytoscape 3.6.1, and then the PPI network diagram (Figure 9B) was obtained using the plug-in cytoHubba. According to a degree, the target genes with the largest number of ten nodes [epidermal growth factor receptor (EGFR), SLC2A1, KDR, ABCA1, LPL, FLT1, LOX, MMP9, SCARB1, and PPARGC1A] were screened (Table 5).
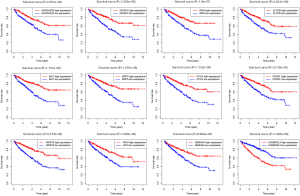
Table 4
Target gene | MiRNA | miRDB | miRTarBase | TargetScan | Sum |
---|---|---|---|---|---|
ARHGAP24 | has-miR-130b-3p | 1 | 0 | 1 | 2 |
CLCN5 | has-miR-155-5p | 1 | 0 | 1 | 2 |
has-miR-130b-3p | 1 | 0 | 1 | 2 | |
has-miR-149-5p | 1 | 0 | 1 | 2 | |
IQGAP2 | has-miR-130b-3p | 1 | 0 | 1 | 2 |
IRF6 | has-miR-144-5p | 1 | 0 | 1 | 2 |
MUT | has-miR-155-5p | 0 | 1 | 1 | 2 |
MPP5 | has-miR-155-5p | 1 | 1 | 1 | 3 |
has-miR-144-5p | 1 | 0 | 1 | 2 | |
PCCA | has-miR-155-5p | 0 | 1 | 1 | 2 |
PODXL | has-miR-155-5p | 0 | 1 | 1 | 2 |
SEMA5A | has-miR-155-5p | 1 | 0 | 1 | 2 |
SH3BGRL3 | has-miR-149-5p | 0 | 1 | 1 | 2 |
VAV3 | has-miR-155-5p | 1 | 0 | 1 | 2 |
WDR72 | has-miR-155-5p | 1 | 0 | 1 | 2 |
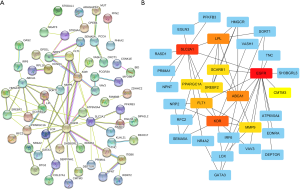
Table 5
ID | MCC | DMNC | MNC | Degree | EPC | Bottle Neck | EcCentricity | Close ness | Radiality | Betweenness | Stress | Clustering Coefficient |
---|---|---|---|---|---|---|---|---|---|---|---|---|
EGFR | 30 | 0.33 | 7 | 15 | 18.40 | 22 | 0.15 | 27.3 | 5.4 | 855.3 | 1482 | 0.08 |
SLC2A1 | 13 | 0.46 | 3 | 10 | 17.66 | 24 | 0.15 | 24.6 | 5.3 | 683.2 | 1214 | 0.11 |
KDR | 27 | 0.32 | 8 | 9 | 17.86 | 18 | 0.19 | 23.9 | 5.3 | 468.8 | 976 | 0.30 |
ABCA1 | 28 | 0.45 | 5 | 7 | 16.31 | 5 | 0.13 | 19.5 | 4.8 | 203.1 | 454 | 0.33 |
LPL | 30 | 0.33 | 7 | 7 | 16.67 | 2 | 0.13 | 20.5 | 4.9 | 123.8 | 244 | 0.42 |
FLT1 | 18 | 0.38 | 6 | 6 | 16.84 | 3 | 0.19 | 21.9 | 5.2 | 210.3 | 564 | 0.53 |
LOX | 9 | 0.38 | 4 | 5 | 14.97 | 3 | 0.15 | 20.3 | 5.0 | 191.4 | 326 | 0.4 |
MMP9 | 13 | 0.47 | 4 | 5 | 16.39 | 6 | 0.15 | 20.7 | 5.1 | 127.9 | 314 | 0.5 |
SCARB1 | 25 | 0.57 | 4 | 5 | 15.55 | 1 | 0.13 | 19.9 | 4.9 | 94.9 | 238 | 0.6 |
PPARGC1A | 6 | 0.31 | 3 | 5 | 14.11 | 5 | 0.13 | 19.4 | 4.8 | 179.4 | 350 | 0.2 |
Discussion
CcRCC is a malignant RCC and is the most common renal cell subtype, characterized by the poor outcome, complex molecular and cellular heterogeneity, and high mortality and morbidity (22,23). A great deal of evidence shows that miRNAs regulate the expression of many genes and play critical roles in many biological processes of human malignant tumors (24). Previous studies showed that miRNAs participate in the occurrence and development of RCC (25-27). miRNA participates as an oncogene or tumor suppressor gene; therefore, the survival of tumor patients can be predicted at the miRNA level. The outcome can be improved by regulating the expression of these miRNA. Several miRNAs can be used as potential prognostic indicators of ccRCC (28-30). Nevertheless, the relationship between miRNA expression and the outcome of patients with ccRCC remains to be further clarified. At present, there are many prognostic markers related to the tumor based on multi-miRNAs signals (31-33), especially ccRCC (34). Unlike in the past, our model includes both miRNA-5p and 3p analysis, improvement of research methods, larger sample size, and most importantly, we verified the model using a series of analyses to make our model more accurate and reliable than the others. The target genes and related potential biological functions of miRNAs were also explored.
We downloaded ccRCC miRNA expression data spectrum and corresponding clinical data from TCGA. Using R language to analyze the difference of miRNA, a total of 211 differentially expressed miRNA were obtained in ccRCC and paracancerous tissues. They were randomly divided into training and test groups. Univariate and multivariate Cox regression methods were used to establish a miRNA prognostic model for training, and five miRNAs with prognostic value were obtained (miR-130b-3p, miR-155-5p, miR-365b-3p, miR-149-5p, miR-144-5p). Five miRNAs were verified in the testing group and the whole group. They were divided into high- and low-risk groups according to the median value of risk. The survival curve showed that the high-risk group’s overall survival rate was significantly lower than that of the low-risk group. The ROC curve shows that the model has an excellent ability to predict the survival of the three groups. The rates of training and test groups were 0.781 and 0.728, respectively. After considering clinical factors such as sex, age, grade, and stage, the ROC survival analysis showed that the AUC value was 0.724. The univariate and multivariate prognostic analysis showed that the risk value of the model was significantly correlated with the outcome (P<0.05). To sum up, the risk value of the model can be used as an independent predictor of outcome (Figure 4).
Sun et al. demonstrated that the upregulation of miR-130b-3p activates the PI3K/AKT/ F- kappa B pathway and promotes cell proliferation and cell cycle by down-regulating PTEN (35). Qu et al. demonstrated the function of miR-365b-3p in coronary atherosclerosis (36). Tao et al. demonstrated miR-155-5p/3p in ccRCC cells and the increased expression of MIR155HG in RCC, which interfered with the inhibition of renal cell function. At the same time, overexpression of miR-155-3p and miR-155-5p reversed this effect. MiR155HG interference inhibited the expression of miR-155-5p and miR-155-3p in ccRCC.
In contrast, inhibition of miR-155-5p and miR-155-3p inhibited the proliferation, invasion, and migration of ccRCC, providing a new method for early diagnosis and proper treatment of ccRCC (37). Yamada et al. reported that miR-144-5p and carcinogenic SDC3 axis were closely related to the pathogenesis of RCC and played antitumor roles (38). However, the interaction mechanism of these five miRNAs in ccRCC has not been clearly defined. To determine the role of these five- miRNAs in ccRCC, three target gene prediction libraries were used to predict target genes, and a total of 3015 target genes were predicted. Then, the target genes were intersected with the differential genes of TCGA, and the functional enrichment analysis of these overlapping genes was carried out. The signal pathways of target genes were concentrated in the Rap1 signal pathway, focal adhesion, human papillomavirus infection, and others. Si-tong et al. shoed that sharpin promotes migration and invasion of melanoma by regulating Ras-associated protein-1 (Rap1) and its downstream pathways, including p38 and JNK/c-jun. RAP1 activators (8-PCPT-2'-O-Me-cAMP) and inhibitors (ESI-09 and farnesyl thiosalicylamide) partially inhibit the invasion and migration of tumor cells. Studies have shown that sharpin promotes melanoma development through the p38 and JNK/c-jun pathways by upregulating the expression of Rap1 (38). Therefore, we can speculate that upregulated Rap1 promotes the progression and poor outcome of ccRCC. In this way, we can use RAP1 inhibitors to inhibit progression and improve outcomes, although this needs to be further confirmed by experiments. It is well known that infection by human papillomavirus (HPV) is associated with cervical cancer. These signal pathways show their role in tumorigenesis and progression in varying degrees; however, the signal pathways involved in these target genes are only the tip of the iceberg, suggesting that the miRNA prognostic model we constructed may be involved in the regulation of signal pathways in the progression and outcome of ccRCC. Ten essential genes of regulatory model miRNA were screened using Cytoscape (Table 4). Survival analysis showed 12 genes (ARHGAP24, CLCN5, IQGAP2, IRF6, MPP5, MUT, MUT, PODXL, SEMA5A, SH3BGRL3, VAV3, and WDR72) was related to survival and outcome. The expression of SH3BGRL3 was negatively correlated with the outcome; that is to say, higher expression of SH3BGRL3 correlated with worse outcome. The other 11 genes were positively correlated with the outcome; higher expression correlated with a better outcome. These findings suggest that we can regulate the expression of these 12 essential genes to affect the outcome. According to related studies, circRNAs are involved in tumorigenesis, becoming a new tumor biomarker and therapeutic target of lung adenocarcinoma (LUAD), playing an essential role in various biological processes, and related to chemotherapeutic drug resistance. We found that the expression of CDR1-AS was upregulated in LUAD tissues and cell lines, and CDR1-AS was closely related to the EGFR/PI3K signal pathway in A549/CR cells. CDR1-AS participates in chemotherapy resistance of PTX and CDDP in LUAD through the EGFR/PI3K signal pathway.
To sum up, CDR1-AS is highly expressed in LUAD and is an independent biomarker of outcome in patients with LUAD. EGFR-tyrosine kinase inhibitor treats squamous cell carcinoma; however, the transformation details are not clear (39). Therefore, the five miRNAs models may affect the survival, outcome, and progression in patients with ccRCC by regulating the expression of EGFR target genes.
We screened these five miRNAs target genes significantly enriched in many tumor-related pathways, including the Rap1 signal pathway, the focal adhesion signal pathway, the human papillomavirus infection signal pathway, and others. These signaling pathways are involved in the occurrence, development, and outcome of many human cancers, including LUAD, RCC, cervical cancer, and melanoma (40). Therefore, we have good reason to believe that signal pathway regulation may play a vital role in the occurrence, development, and outcome of ccRCC. Further molecular research may provide new therapeutic targets for ccRCC and significantly improve outcomes.
Acknowledgments
Funding: This work was supported by
Footnote
Reporting Checklist: The authors have completed the STROBE reporting checklist. Available at http://dx.doi.org/10.21037/tcr-21-37
Peer Review File: Available at http://dx.doi.org/10.21037/tcr-21-37
Conflicts of Interest: All authors have completed the ICMJE uniform disclosure form (available at http://dx.doi.org/10.21037/tcr-21-37). The authors have no conflicts of interest to declare.
Ethical Statement: The authors are accountable for all aspects of the work in ensuring that questions related to the accuracy or integrity of any part of the work are appropriately investigated and resolved. The study was conducted in accordance with the Declaration of Helsinki (as revised in 2013). The study data are from the National Cancer Institute (NCI) and the National Human Genome Institute (NHGRI), without the need for an ethical statement.
Open Access Statement: This is an Open Access article distributed in accordance with the Creative Commons Attribution-NonCommercial-NoDerivs 4.0 International License (CC BY-NC-ND 4.0), which permits the non-commercial replication and distribution of the article with the strict proviso that no changes or edits are made and the original work is properly cited (including links to both the formal publication through the relevant DOI and the license). See: https://creativecommons.org/licenses/by-nc-nd/4.0/.
References
- Cairns P. Renal cell carcinoma. Cancer Biomark 2010;9:461-73. [Crossref] [PubMed]
- Torre LA, Bray F, Siegel RL, et al. Global cancer statistics, 2012. CA Cancer J Clin 2015;65:87-108. [Crossref] [PubMed]
- Ljungberg B, Albiges L, Abu-Ghanem Y, et al. European Association of Urology Guidelines on Renal Cell Carcinoma: The 2019 Update. Eur Urol 2019;75:799-810. [Crossref] [PubMed]
- Fisher R, Gore M, Larkin J. Current and future systemic treatments for renal cell carcinoma. Semin Cancer Biol 2013;23:38-45. [Crossref] [PubMed]
- Chen J, Zhang D, Zhang W, et al. Clear cell renal cell carcinoma associated microRNA expression signatures identified by an integrated bioinformatics analysis. J Transl Med 2013;11:169. [Crossref] [PubMed]
- Escudier B. Advanced renal cell carcinoma: current and emerging management strategies. Drugs 2007;67:1257-64. [Crossref] [PubMed]
- Vera-Badillo FE, Templeton AJ, Duran I, et al. Systemic therapy for non-clear cell renal cell carcinomas: a systematic review and meta-analysis. Eur Urol 2015;67:740-9. [Crossref] [PubMed]
- Allison DB, Cui X, Page GP, et al. Microarray data analysis: from disarray to consolidation and consensus. Nat Rev Genet 2006;7:55-65. [Crossref] [PubMed]
- Lossos IS, Czerwinski DK, Alizadeh AA, et al. Prediction of survival in diffuse large-B-cell lymphoma based on the expression of six genes. N Engl J Med 2004;350:1828-37. [Crossref] [PubMed]
- Lu TX, Rothenberg ME. MicroRNA. J Allergy Clin Immunol 2018;141:1202-7. [Crossref] [PubMed]
- Chekouo T, Stingo FC, Doecke JD, et al. miRNA-target gene regulatory networks: A Bayesian integrative approach to biomarker selection with application to kidney cancer. Biometrics 2015;71:428-38. [Crossref] [PubMed]
- Dondeti VR, Wubbenhorst B, Lal P, et al. Integrative genomic analyses of sporadic clear cell renal cell carcinoma define disease subtypes and potential new therapeutic targets. Cancer Res 2012;72:112-21. [Crossref] [PubMed]
- Sato Y, Yoshizato T, Shiraishi Y, et al. Integrated molecular analysis of clear-cell renal cell carcinoma. Nat Genet 2013;45:860-7. [Crossref] [PubMed]
- Bartel DP. MicroRNAs: genomics, biogenesis, mechanism, and function. Cell 2004;116:281-97. [Crossref] [PubMed]
- Quévillon Huberdeau M, Simard MJ. A guide to microRNA-mediated gene silencing. Febs j 2019;286:642-52. [Crossref] [PubMed]
- Wang RH, He LY, Zhou SH. The role of gene sculptor microRNAs in human precancerous lesions. Onco Targets Ther 2018;11:5667-75. [Crossref] [PubMed]
- Duan Z, Gao Y, Shen J, et al. miR-15b modulates multidrug resistance in human osteosarcoma in vitro and in vivo. Mol Oncol 2017;11:151-66. [Crossref] [PubMed]
- Giannelli G, Santoro A, Kelley RK, et al. Biomarkers and overall survival in patients with advanced hepatocellular carcinoma treated with TGF-βRI inhibitor galunisertib. PLoS One 2020;15:e0222259. [Crossref] [PubMed]
- Murphy DA, Rini BI, Escudier B, et al. Angiogenic and immunomodulatory biomarkers in axitinib-treated patients with advanced renal cell carcinoma. Future Oncol 2020;16:1199-210. [Crossref] [PubMed]
- Wang X, You Z, Zhao G, et al. MicroRNA-194-5p Levels Decrease during Deep Hypothermic Circulatory Arrest. Sci Rep 2018;8:14044. [Crossref] [PubMed]
- Xie M, Lv Y, Liu Z, et al. Identification and validation of a four-miRNA (miRNA-21-5p, miRNA-9-5p, miR-149-5p, and miRNA-30b-5p) prognosis signature in clear cell renal cell carcinoma. Cancer Manag Res 2018;10:5759-66. [Crossref] [PubMed]
- Patard JJ, Leray E, Rioux-Leclercq N, et al. Prognostic value of histologic subtypes in renal cell carcinoma: a multicenter experience. J Clin Oncol 2005;23:2763-71. [Crossref] [PubMed]
- Siegel RL, Miller KD, Jemal A. Cancer statistics, 2018. CA Cancer J Clin 2018;68:7-30. [Crossref] [PubMed]
- Bertoli G, Cava C, Castiglioni I. MicroRNAs: New Biomarkers for Diagnosis, Prognosis, Therapy Prediction and Therapeutic Tools for Breast Cancer. Theranostics 2015;5:1122-43. [Crossref] [PubMed]
- Gao C, Peng FH, Peng LK. MiR-200c sensitizes clear-cell renal cell carcinoma cells to sorafenib and imatinib by targeting heme oxygenase-1. Neoplasma 2014;61:680-9. [Crossref] [PubMed]
- Szabó Z, Szegedi K, Gombos K, et al. Expression of miRNA-21 and miRNA-221 in clear cell renal cell carcinoma (ccRCC) and their possible role in the development of ccRCC. Urol Oncol 2016;34:533.e21-533.e27. [Crossref] [PubMed]
- Zhao JJ, Chen PJ, Duan RQ, et al. miR-630 functions as a tumor oncogene in renal cell carcinoma. Arch Med Sci 2016;12:473-8. [Crossref] [PubMed]
- Carrasco-Rozas A, Fernández-Simón E, Lleixà MC, et al. Identification of serum microRNAs as potential biomarkers in Pompe disease. Ann Clin Transl Neurol 2019;6:1214-24. [Crossref] [PubMed]
- Roser AE, Caldi Gomes L, Schünemann J, et al. Circulating miRNAs as Diagnostic Biomarkers for Parkinson's Disease. Front Neurosci 2018;12:625. [Crossref] [PubMed]
- Yin Q, Feng W, Shen X, et al. Regulatory effects of lncRNAs and miRNAs on autophagy in malignant tumorigenesis. Biosci Rep 2018;38:BSR20180516. [Crossref] [PubMed]
- Shi XH, Li X, Zhang H, et al. A Five-microRNA Signature for Survival Prognosis in Pancreatic Adenocarcinoma based on TCGA Data. Sci Rep 2018;8:7638. [Crossref] [PubMed]
- Xu J, Zhao J, Zhang R. Four microRNAs Signature for Survival Prognosis in Colon Cancer using TCGA Data. Sci Rep 2016;6:38306. [Crossref] [PubMed]
- Zhang C, Zhang CD, Ma MH, et al. Three-microRNA signature identified by bioinformatics analysis predicts prognosis of gastric cancer patients. World J Gastroenterol 2018;24:1206-15. [Crossref] [PubMed]
- Liang B, Zhao J, Wang X. A three-microRNA signature as a diagnostic and prognostic marker in clear cell renal cancer: An In Silico analysis. PLoS One 2017;12:e0180660. [Crossref] [PubMed]
- Sun L, Liu J, Yuan Q, et al. Association between PTEN Gene IVS4 polymorphism and risk of cancer: a meta-analysis. PLoS One 2014;9:e98851. [Crossref] [PubMed]
- Qu Y, Zhang N. miR-365b-3p inhibits the cell proliferation and migration of human coronary artery smooth muscle cells by directly targeting ADAMTS1 in coronary atherosclerosis. Exp Ther Med 2018;16:4239-45. [Crossref] [PubMed]
- Tao M, Zhou Y, Jin Y, et al. Blocking lncRNA MIR155HG/miR-155-5p/-3p inhibits proliferation, invasion and migration of clear cell renal cell carcinoma. Pathol Res Pract 2020;216:152803. [Crossref] [PubMed]
- Yamada Y, Arai T, Kojima S, et al. Regulation of antitumor miR-144-5p targets oncogenes: Direct regulation of syndecan-3 and its clinical significance. Cancer Sci 2018;109:2919-36. [Crossref] [PubMed]
- Uruga H, Fujii T, Nakamura N, et al. Squamous cell transformation as a mechanism of acquired resistance to tyrosine kinase inhibitor in EGFR-mutated lung adenocarcinoma: a report of two cases. Respirol Case Rep 2020;8:e00521. [Crossref] [PubMed]
- Mao Y, Xu R. Circular RNA CDR1-AS contributes to pemetrexed and cisplatin chemoresistance through EGFR/PI3K signaling pathway in lung adenocarcinoma. Biomed Pharmacother 2020;123:109771. [Crossref] [PubMed]