A six-gene signature related with tumor mutation burden for predicting lymph node metastasis in breast cancer
Introduction
Breast cancer (BC) is one of the leading malignancies among females worldwide, with 279,100 estimated new cases and 42,690 estimated deaths in 2020 (1). In spite of the development of medical technologies, large amounts of BC patients were still diagnosed with lymph node metastasis (2). Lymph node status was identified as an important prognostic factor in BC and patients with lymph node metastasis had a worse survival outcome than those without metastatic status (3,4). Moreover, BC patients with lymph node metastasis often suffer from sentinel lymph node biopsy or axillary dissection, taking a risk of many serious complications, such as lymphedema, chyle leak, seroma formation and so on (5-7). Therefore, it is urgent for clinicians to search for potential biomarkers to predict the lymph node metastasis in BC.
Tumor mutation burden (TMB) refers to the total quantity of non-synonymous point mutations per coding region in the tumor gene (8). Recently, TMB has been regarded as an effective biomarker for predicting response to immune checkpoint inhibitors in multiple cancer types, such as melanoma, non-small cell lung cancer and advanced urothelial cancer (9-11). Besides, an increasing number of researchers begin to explore the relationship between TMB and BC. It was discovered that TMB was closely related with immune-mediated survival in BC (12). According to Park, high TMB was associated with good overall survival and functioned as an independent prognostic factor in HER2-positive metastatic BC (13). Barroso-Sousa suggested that BC patients with high TMB were more likely to benefit from PD-1 inhibitors (14).
Current researches mostly focused on the role of TMB in predicting clinical outcomes of BC, the correlation between TMB and tumor biological characteristics, such as lymph node metastasis, remained unclear. It was reported that tumor progression, such as lymphatic metastasis was regulated by both tumor escape mechanism and dysfunction of immune system, which highlighted the significant role of immune system in tumor biological behaviors (15,16). Moreover, tumor infiltrating lymphocytes (TILs) were observed to participate in the regulation of lymph node metastasis, including CD8(+) T cells and Foxp3(+) Tregs (17). Meanwhile, as to TMB, many researchers considered TMB as an effective biomarker for predicting immune response owing to its potential function of increasing neoantigens and inducing TILs infiltration (18,19). According to Mei, high TMB level in BC patients was positively related with the amount of TILs (20). However, whether TMB can influence the immune cell infiltration and further regulate lymph node metastasis in BC is still lack of evidence, which deserves further investigation.
With the development of sequencing and chip technologies, an increasing number of public databases are emerging, such as The Cancer Genome Atlas (TCGA) database and Gene Expression Omnibus (GEO) database. Researchers around the world upload their research data to these public databases, promoting information sharing and accelerating medical development. In the present study, we obtained mutation data, transcriptome data and clinical data from the TCGA database. The TMB value of each BC sample was calculated and the correlation between TMB and lymph node metastasis was investigated. We identified TMB-related and lymph node-related differentially expressed genes (DEGs) respectively to extract common DEGs. Functional enrichment analysis and protein-protein interaction (PPI) network were performed to explore the biological roles of common DEGs. A TMB-related signature including six genes was constructed for predicting the lymph node metastasis in BC and external verification was further conducted. After a comprehensive analysis, we believed that the TMB-related signature had potential in predicting the lymph node metastasis in BC. We present the following article in accordance with the STROBE reporting checklist (available at http://dx.doi.org/10.21037/tcr-20-3471).
Methods
Data acquisition
We obtained the BC mutation data, transcriptome data and clinical data from TCGA database (https://portal.gdc.cancer.gov/), comprising 460 lymph node negative samples and 489 lymph node positive samples, which served as the training dataset. We employed “maftools” R package to analyze the Masked Somatic Mutation data, which were processed via VarScan software. Transcriptome data were acquired from HTseq-FPKM platform while clinical data contained age, gender, pathological stage and AJCC-TNM stage. We also obtained a gene expression profile (GSE102484) from the Gene Expression Omnibus (GEO) database (https://www.ncbi.nlm.nih.gov/geo/), comprising 300 lymph node negative samples and 383 lymph node positive samples, which functioned as the validation dataset. The platform for GSE102484 was the GPL570 [HG-U133_Plus_2] Affymetrix Human Genome U133 Plus 2.0 Array. All data were publicly available and patients with data deficiency were excluded. The clinical characteristics of BC patients in training and validation datasets are summarized in Table 1. The study was conducted in accordance with the Declaration of Helsinki (as revised in 2013).
Table 1
Variable | Number, n (%) | |
---|---|---|
TCGA database | GSE102484 database | |
Age | 0 patient missing | 0 patient missing |
≤58 years | 481 (50.7) | 541 (79.2) |
>58 years | 468 (49.3) | 142 (20.8) |
Gender | 0 patient missing | 0 patient missing |
Female | 938 (98.8) | 683 (100.0) |
Male | 11 (1.2) | 0 (0) |
Stage | 13 (1.3) patients missing | 0 patient missing |
I | 162 (17.1) | 175 (25.6) |
II | 550 (58.0) | 328 (48.0) |
III | 208 (21.9) | 174 (25.5) |
IV | 16 (1.7) | 6 (0.9) |
T | 1 (0.1) patient missing | 0 patient missing |
T1 | 248 (26.1) | 276 (40.4) |
T2 | 557 (58.7) | 377 (55.2) |
T3 | 109 (11.5) | 24 (3.5) |
T4 | 34 (3.6) | 6 (0.9) |
N | 0 patient missing | 0 patient missing |
N0 | 460 (48.5) | 300 (43.9) |
N1 | 317 (33.4) | 214 (31.3) |
N2 | 112 (11.8) | 87 (12.7) |
N3 | 60 (6.3) | 82 (12.1) |
M | 129 (13.6) patients missing | 0 patient missing |
M0 | 802 (84.5) | 582 (85.2) |
M1 | 18 (1.9) | 101 (14.8) |
TMB value calculation and correlation with lymph node metastasis
TMB was calculated by the ratio of total mutation amounts to human exon length (38 Mb) and was regarded as the frequency of tumor gene mutations, consisting of insertions or deletions, coding errors and base substitutions. We conducted correlation analysis between TMB and different lymph node status. Wilcoxon rank-sum test and Kruskal-Wallis test were respectively used for two or more groups.
Differential analysis, functional enrichment analysis and PPI network construction
We divided BC samples into lymph node positive and negative groups to identify lymph node-related DEGs. Furthermore, based on the median TMB value, TMB-related DEGs between high and low TMB groups were respectively acquired in lymph node positive and negative groups. Differential analysis above was performed through “limma” package with the criterion of P value <0.05 and false discovery rate (FDR) <0.05, which was further visualized by volcano plots. Subsequently, the intersection between lymph node-related and TMB-related DEGs was extracted as common DEGs through “VennDiagram” package and further visualized via “UpSetR” package. Besides, in order to explore biological functions of common DEGs, Gene Ontology (GO) and Kyoto Encyclopedia of Genes and Genomes (KEGG) analysis were applied via “org.Hs.eg.db”, “clusterProfiler”, “ggplot2” and “enrichplot” packages. Moreover, the PPI network of common DEGs was conducted by STRING database (https://string-db.org/) and presented via Cytoscape software.
TMB-related signature construction and external verification
We firstly conducted univariate Logistic regression analysis to screen for common DEGs associated with lymph node metastasis with P value <0.001. Then, we brought selected DEGs into multivariate Logistic regression analysis to calculate regression coefficient(β) and establish a TMB-related signature through the formula: risk score = Σ(β * the expression of gene). We applied the formula above to calculate risk score of each sample and divided all samples into high and low risk groups according to the median risk score. The correlation between risk score and clinical variables was explored via Chi-square test and the expression of six hub genes between high and low risk groups was displayed via “pheatmap” package. Meanwhile, the receiver operating characteristic (ROC) curve was applied to assess the predictive value via “pROC” package and the evaluation of independent predictive factors was also performed through Logistic regression analysis. Furthermore, we brought all independent predictive factors into a nomogram through “rms” package and assessed its predictive accuracy via the calibration plot. A validation dataset (GSE102484) was further utilized for external verification.
Expression level and relapse-free survival (RFS) analysis of hub genes between patients with different lymph node status
We investigated comparative analysis between six hub genes and different lymph node status via Wilcoxon rank-sum test for two groups and Kruskal-Wallis test for more groups. Moreover, we performed RFS analysis of six hub genes respectively in lymph node positive and negative groups via the Kaplan-Meier Plotter mRNA BC database (http://kmplot.com/analysis/index.php?p=service). We divided patients into high and low expression groups based on the best cutoff and calculated relevant log-rank P values respectively in lymph node positive and negative groups. P value <0.05 was identified as statistically significant.
Correlation of hub genes with TMB and cBioPortal analysis
We performed correlation analysis between six hub genes and TMB via Spearman correlation analysis and visualized the results via the “ggplot2”, “ggpubr” and “ggExtra” packages. Furthermore, we used cBioPortal database (http://www.cbioportal.org/) to acquire gene alteration frequencies and types of six hub genes in different BC studies.
Statistical analysis
We utilized R software (Version 3.6.3) and SPSS software (Version 24.0) to perform data analysis. The construction of TMB-related signature was conducted by univariate and multivariate Logistic regression analysis. Survival analysis was performed by Kaplan-Meier method and log-rank test. We applied differential analysis via “limma” package and conducted correlation analysis through Spearman correlation analysis. We investigated comparative analysis of continuous variables through Wilcoxon rank-sum test for two groups and Kruskal-Wallis test for more groups. Meanwhile, comparative analysis of categorical variables was conducted by Chi-square test. A P value <0.05 was identified as statistically significant.
Results
Visualization of BC mutation profiles
We utilized waterfall plot to exhibit high-frequency mutated genes in BC, such as TP53 (34%), PIK3CA (33%), TTN (16%), CDH1 (13%) and GATA3 (12%) (Figure 1A). Besides, missense mutation ranked first in variant classification, single nucleotide polymorphism (SNP) appeared more frequently than insertion or deletion and C>T was considered as the most common single nucleotide variant (SNV). Meanwhile, the median variation was estimated to be 29 and variant types were also shown via box plot (Figure 1B). Furthermore, the co-occurance and mutual exclusion among top 25 mutated genes were also displayed (Figure 1C).
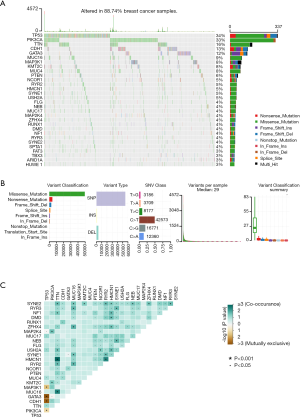
TMB correlated with lymph node metastasis
Clinical information was acquired and merged with TMB value to further explore the correlation between TMB and different lymph node status of BC. We discovered that TMB was negatively correlated with lymph node metastasis in BC (P=0.003) (Figure 2A). Moreover, N1 group had lower TMB level than N0 group (P<0.001) and N2 group (P=0.018) (Figure 2B).
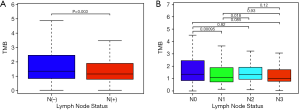
Identification of DEGs
We performed differential analysis to screen for lymph node-related and TMB-related DEGs between lymph node positive and negative groups. According to TMB-related DEGs, 8,751 DEGs (2,522 up-regulated and 6,229 down-regulated) and 9,354 DEGs (3,636 up-regulated and 5,718 down-regulated) were respectively identified in lymph node positive and negative groups, while 763 lymph node-related DEGs (455 up-regulated and 308 down-regulated) was confirmed. Differential expression was displayed through volcano plots (Figure 3) and 125 common DEGs were extracted as the intersection of three datasets above via Venn diagram (Figure 4A).
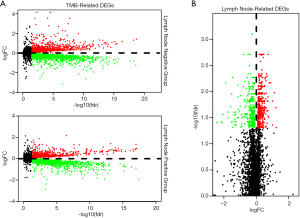
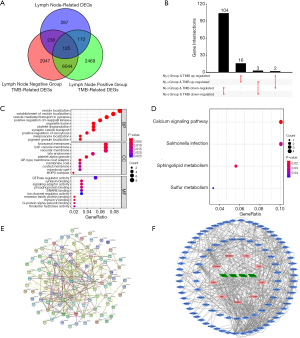
Functional enrichment analysis and PPI network of common DEGs
After acquiring 125 common DEGs, we utilized the Upset diagram for further detailed analysis. Based on median TMB value, 16 simultaneously up-regulated and 104 simultaneously down-regulated common DEGs were identified in lymph node positive and negative groups, while 5 other common DEGs were regulated reversely (Figure 4B). We conducted functional enrichment analysis to further investigate significant pathways of 125 common DEGs. GO analysis indicated that common DEGs were enriched in vesicle localization, the membrane of lysosome, vacuole and lytic vacuole, GTPase regulator activity among biological process (BP), cellular component (CC) and molecular function (MF) categories respectively (Figure 4C). KEGG analysis demonstrated that calcium signaling pathway and salmonella infection were mainly enriched (Figure 4D). Subsequently, we searched 125 common DEGs in STRING database with the interaction score of 0.150 and constructed a PPI network with disconnected nodes hided, which was further visualized via Cytoscape software (Figure 4E). The PPI network of common DEGs contained 101 nodes and 498 edges, with simultaneously up-regulated, simultaneously down-regulated and reversely regulated common DEGs marked in pink, blue and green respectively (Figure 4F).
Construction and verification of the TMB-related signature
We firstly performed univariate Logistic regression analysis to select 29 genes associated with lymph node metastasis among 125 common DEGs, with P value <0.001. We further brought selected 29 genes into multivariate Logistic regression analysis and utilized “Forward: LR” to establish a TMB-related signature of six genes for predicting lymph node metastasis (Table 2). The risk score of TMB-related signature was calculated via regression coefficient(β) and expression level of six hub genes, which was identified as follows: risk score= expBAHD1*(0.054)+ expPPM1A*(0.069)+ expPQLC3*(0.024)+ expSMPD3*(0.104)+ expEEF1A1*(-0.001)+ expS100B*(-0.010) (Figure 5). Subsequently, we calculated risk score of each sample and divided all samples into high and low risk groups. As shown in the heat map, risk score was significantly associated with age (P<0.05), stage (P<0.001) and lymph node status (P<0.001) in TCGA database. Meanwhile, the expression of BAHD1, PPM1A, PQLC3 and SMPD3 was higher in high risk group while EEF1A1 and S100B presented opposite trends (Figure 6A,B). Furthermore, the ROC curve indicated reliable predictivity of six-gene signature with the area under curve (AUC) of 0.656 in TCGA database and 0.561 in GSE102484 database (Figure 6C,D). Univariate and multivariate Logistic regression analysis proved the TMB-related signature as an independent predictive factor for lymph node metastasis in BC (Table 3, Table 4). Besides, a nomogram containing independent predictive factors was constructed and the calibration plot confirmed its effective predictivity in TCGA database and GSE102484 database (Figure 6E,F,G).
Table 2
Gene | Univariate analysis | Multivariate analysis | ||||
---|---|---|---|---|---|---|
OR (95% CI) | P value | OR (95% CI) | P value | Coefficient | ||
BAHD1 | 1.088 (1.041–1.137) | <0.001 | 1.055 (1.007–1.105) | 0.023 | 0.054 | |
PPM1A | 1.106 (1.059–1.155) | <0.001 | 1.072 (1.024–1.122) | 0.003 | 0.069 | |
PQLC3 | 1.028 (1.014–1.042) | <0.001 | 1.024 (1.010–1.038) | 0.001 | 0.024 | |
SMPD3 | 1.160 (1.077–1.249) | <0.001 | 1.110 (1.032–1.193) | 0.005 | 0.104 | |
EEF1A1 | 0.999 (0.999–1.000) | <0.001 | 0.999 (0.999–1.000) | 0.009 | -0.001 | |
S100B | 0.980 (0.968–0.991) | <0.001 | 0.990 (0.980–0.999) | 0.039 | -0.010 |
OR, odds ratio; CI, confidence interval.
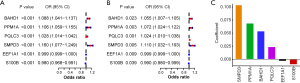
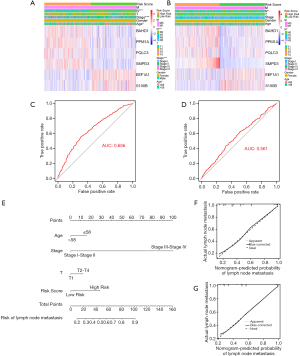
Table 3
Variable | Univariate analysis | Multivariate analysis | |||
---|---|---|---|---|---|
OR (95% CI) | P value | OR (95% CI) | P value | ||
Risk Score | 2.638 (2.068–3.364) | <0.001* | 2.664 (2.013–3.526) | <0.001* | |
Age (≤58 years) | 1.442 (1.093–1.901) | 0.010* | 1.947 (1.376–2.753) | <0.001* | |
Gender (female) | 0.426 (0.109–1.661) | 0.219 | – | – | |
Stage (Stage I-II) | 0.018 (0.008–0.042) | <0.001* | 0.019 (0.008–0.047) | <0.001* | |
T (T1) | 0.416 (0.301–0.576) | <0.001* | 0.619 (0.424–0.903) | 0.013* | |
M (M0) | 0.065 (0.009–0.492) | 0.008* | 1.943 (0.208–18.151) | 0.560 |
Age was classified as ≤58 years and >58 years; Gender was classified as female and male; Stage was classified as Stage I-II and Stage III-IV; T was classified as T1 and T2-T4; M was classified as M0 and M1; OR, odds ratio; CI, confidence interval; *, P value <0.05 has statistical significance.
Table 4
Variable | Univariate analysis | Multivariate analysis | |||
---|---|---|---|---|---|
OR (95% CI) | P value | OR (95% CI) | P value | ||
Risk score | 35.579 (2.865–441.816) | 0.005* | 21.034 (1.167–379.142) | 0.039* | |
Age (≤58 years) | 1.268 (0.876–1.837) | 0.208 | – | – | |
Stage (Stage I-II) | 0.012 (0.004–0.037) | <0.001* | 0.015 (0.005–0.048) | <0.001* | |
T (T1) | 0.242 (0.175–0.335) | <0.001* | 0.372 (0.258–0.538) | <0.001* | |
M (M0) | 0.285 (0.172–0.473) | <0.001* | 0.934 (0.478–1.825) | 0.842 |
Age was classified as ≤58 years and >58 years; Stage was classified as Stage I-II and Stage III-IV; T was classified as T1 and T2-T4; M was classified as M0 and M1; OR, odds ratio; CI, confidence interval; *, P value <0.05 has statistical significance.
Differential expression of six hub genes between patients with different lymph node status
We performed comparative analysis between six hub genes and different lymph node status in BC. The results demonstrated that four hub genes were positively correlated with lymph node metastasis, including BAHD1 (P<0.001), PPM1A (P<0.001), PQLC3 (P<0.001) and SMPD3 (P<0.001), while EEF1A1 (P<0.001) and S100B (P=0.001) exhibited negative correlation (Figure 7A). Furthermore, more detailed comparative analysis was conducted. The expression levels of BAHD1 (P<0.001), PPM1A (P<0.001), PQLC3 (P<0.001) and SMPD3 (P<0.001) were found lower in N0 group than in N1 group, while EEF1A1 (P=0.008) and S100B (P=0.006) presented the opposite expression. Meanwhile, PPM1A (P<0.001), PQLC3 (P<0.001) and SMPD3 (P=0.002) had lower expression in N0 group than in N2 group, while EEF1A1 (P=0.005) and S100B (P=0.002) presented the opposite expression. Besides, BAHD1 (P=0.002) had lower mRNA expression in N0 group than in N3 group (Figure 7B).
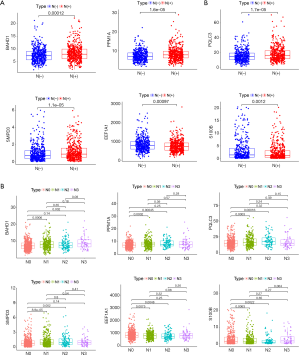
RFS analysis of six hub genes between patients with different lymph node status
Based on the Kaplan-Meier Plotter, we divided patients into high and low expression groups via the best cutoff and conducted RFS analysis of six hub genes respectively in lymph node positive and negative BC. The results indicated that higher expression levels of BAHD1 (P=0.020), PPM1A (P=0.049), PQLC3 (P=0.009), SMPD3 (P=0.037) and EEF1A1 (P=0.034) were associated with better RFS in lymph node negative group while S100B (P=0.260) showed no association with RFS (Figure 8A). Meanwhile, higher expression levels of BAHD1 (P=0.009), PPM1A (P<0.001), PQLC3 (P=0.043), SMPD3 (P=0.021) and EEF1A1 (P=0.015) were associated with better RFS in lymph node positive group while higher S100B (P=0.033) expression had worse prognosis (Figure 8B).
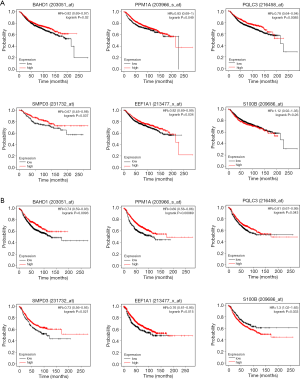
Correlation of six hub genes with TMB and cBioPortal analysis
We investigated the correlation between six hub genes and TMB. The results demonstrated that the expression levels of BAHD1 (P<0.001), PPM1A (P<0.001), PQLC3 (P<0.001), SMPD3 (P<0.001) and EEF1A1 (P<0.001) were negatively correlated with TMB while S100B (P=0.18) showed no significant correlation with TMB (Figure 9A). Besides, we utilized cBioPortal database to acquire gene alteration status of six hub genes in different BC studies. The results suggested that gene alteration frequency of PQLC3 and SMPD3 was less than 2% while PPM1A and S100B had less than 4.5% gene alterations. Meanwhile, BAHD1 had less than 3% gene alterations while the gene alteration frequency of EEF1A1 was less than 5%. Moreover, deep deletion was the most common alteration type in BAHD1, SMPD3 and EEF1A1, while amplification ranked first in PPM1A, PQLC3 and S100B (Figure 9B).
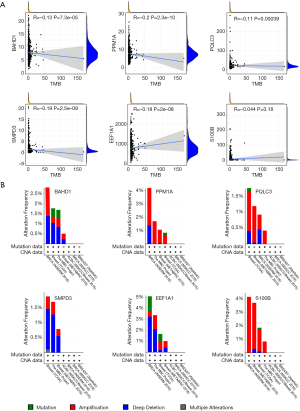
Discussion
With the rapid development of sequencing technology, an increasing number of researchers begin to investigate the potential role of TMB in the occurrence and development of BC. Voutsadakis reported that the TMB expression was higher in HER2-positive subtype than in luminal and triple-negative subtypes (21). Similar to Voutsadakis, Xu suggested that elevated TMB expression was identified in HR-negative or HER2-positive BC (22). Besides, many sequencing researches were performed in triple-negative breast cancer (TNBC) and discovered the association between high TMB expression and better prognosis. Karn indicated that early TNBC patients with high TMB were more likely to achieve pathological complete response (pCR) and TMB was regarded as an independent predictive factor for pCR (23). According to Barroso-Sousa, higher TMB expression was associated with better progress free survival in metastatic TNBC patients treated with anti-PD-1/L1 (24).
However, the majority of current studies paid attention to the predictive function of TMB for clinical outcomes in BC, the correlation between TMB and lymph node metastasis is still ambiguous. Among BC patients, those with lymph node metastasis were under a threat of wider surgical margin, more serious complications and higher chemotherapy toxicity, suffering from a worse prognosis (25-27). Therefore, it is of great significance to further investigate the relationship between TMB and lymph node metastasis in BC.
It was demonstrated that tumor-mediated immune dysfunction played an important role in accelerating tumor progression, including lymphatic metastasis (28-30). According to Zuckerman, the down-regulation of immune-related genes and up-regulation of tumor-promoting genes were observed in BC patients with lymph node metastasis (31). Besides, Kohrt demonstrated that CD1a dendritic cells were lower in tumor-involved axillary nodes than tumor-free axillary nodes in BC and dendritic cells in axillary nodes were closely related with disease-free survival (32). As to TMB, it has been known as a potential predictor for tumor-related immunological response due to the emerging neoantigens from gene alterations and increased infiltration of immune cells (33,34). Based on above researches, we hypothesize that TMB may take part in the regulation of lymph node metastasis in BC through influencing immune cell infiltration and more detailed researches should be performed for further verification.
In our study, we downloaded mutation, transcriptome and clinical data from TCGA database and calculated TMB value of each patient. We merged TMB value and clinical data and discovered that TMB was negatively associated with lymph node metastasis in BC. Subsequently, TMB-related and lymph node-related DEGs were identified respectively and we extracted common DEGs from them. We further conducted functional enrichment analysis and constructed a PPI network of common DEGs. The membrane of lysosome and vacuole, vesicle localization and GTPase regulator activity were mainly enriched in GO analysis, while calcium signaling pathway and salmonella infection were mainly enriched in KEGG analysis. The roles of vesicle localization, calcium signaling and salmonella infection in regulating BC metastasis have been explored in some researches (35-37). Furthermore, we established the TMB-related signature of six genes, including BAHD1, PPM1A, PQLC3, SMPD3, EEF1A1 and S100B, via univariate and multivariate Logistic regression analysis. The predictive accuracy of this signature was assessed reliable in both TCGA database and GSE102484 database.
The function of six hub genes in BC was previously investigated in many researches. Singh verified that SMPD3 participated in the encoding of neutral sphingomyelinase 2 (nSMase2) enzyme and nSMase2 regulated BC invasion via enhancing the exosome-mediated secretion of miR-10b (38). Lin discovered that EEF1A1 had gene alterations in 27% BC patients and could protect tumor cells from proteotoxic injuries via enhancing heat shock responses (39). Li identified that the promoter regulatory element of EEF1A1 was regulated by MALAT1 and over-expressed MALAT1 played an important role in the metastasis of BC (40). In addition, the decreased EEF1A1 expression via curcumol attributed to the suppression of BC metastasis (41). The function of S100B for inhibiting tumor migration was found in ER-negative BC and high expression of S100B was associated with better distant metastases-free survival in BC (42). Over-expressed PPM1A was demonstrated to restrain the progression of triple negative BC through suppressing cell cycle and reducing the phosphorylation of CDK and Rb (43). The researches of BAHD1 in BC remained rare while Goryca identified that the mutation of BAHD1 took part in promoting the metastasis of colorectal cancer (44). The role of PQLC3 in tumorigenesis and progression was still unclear and deserved further investigation.
In this study, we discovered that TMB was negatively correlated with lymph node metastasis and constructed a TMB-related signature based on six genes for predicting lymph node metastasis in BC, which might provide novel sights for clinicians. With the further investigation of TMB, we would like to explore other potential roles of TMB-related signature in BC, such as predicting immune response, survival status and so on. However, there are still some limitations in our study. On one hand, the samples involved in our study is limited and clinical trials of large samples should be further conducted to evaluate the predictivity of the TMB-related signature. On the other hand, our study was lack of experiments in vitro or in vivo, which should be performed to verify the biological functions of six hub genes.
Conclusions
In summary, our study suggested that TMB was negatively correlated with lymph node metastasis in BC. A TMB-related signature including six genes was further constructed and validated for predicting lymph node metastasis in BC, which may provide guidance for clinicians.
Acknowledgments
Funding: This study was supported by
Footnote
Reporting Checklist: The authors have completed the STROBE reporting checklist. Available at http://dx.doi.org/10.21037/tcr-20-3471
Conflicts of Interest: All authors have completed the ICMJE uniform disclosure form (available at http://dx.doi.org/10.21037/tcr-20-3471). The authors have no conflicts of interest to declare.
Ethical Statement: The authors are accountable for all aspects of the work in ensuring that questions related to the accuracy or integrity of any part of the work are appropriately investigated and resolved. The study was conducted in accordance with the Declaration of Helsinki (as revised in 2013).
Open Access Statement: This is an Open Access article distributed in accordance with the Creative Commons Attribution-NonCommercial-NoDerivs 4.0 International License (CC BY-NC-ND 4.0), which permits the non-commercial replication and distribution of the article with the strict proviso that no changes or edits are made and the original work is properly cited (including links to both the formal publication through the relevant DOI and the license). See: https://creativecommons.org/licenses/by-nc-nd/4.0/.
References
- Siegel RL, Miller KD, Jemal A. Cancer statistics, 2020. CA Cancer J Clin 2020;70:7-30. [Crossref] [PubMed]
- Ahmad A. Breast Cancer Statistics: Recent Trends. Adv Exp Med Biol 2019;1152:1-7. [Crossref] [PubMed]
- Liu D, Chen Y, Deng M, et al. Lymph node ratio and breast cancer prognosis: a meta-analysis. Breast Cancer 2014;21:1-9. [Crossref] [PubMed]
- Michaelson JS, Silverstein M, Sgroi D, et al. The effect of tumor size and lymph node status on breast carcinoma lethality. Cancer 2003;98:2133-43. [Crossref] [PubMed]
- Sidapra M, Fuller M, Masannat YA. Diagnosis and management of chyle leak following axillary dissection. Surgeon 2020;18:360-4. [Crossref] [PubMed]
- De Luca A, Tripodi D, Frusone F, et al. Retrospective Evaluation of the Effectiveness of a Synthetic Glue and a Fibrin-Based Sealant for the Prevention of Seroma Following Axillary Dissection in Breast Cancer Patients. Front Oncol 2020;10:1061. [Crossref] [PubMed]
- Armer JM, Ballman KV, McCall L, et al. Lymphedema symptoms and limb measurement changes in breast cancer survivors treated with neoadjuvant chemotherapy and axillary dissection: results of American College of Surgeons Oncology Group (ACOSOG) Z1071 (Alliance) substudy. Support Care Cancer 2019;27:495-503. [Crossref] [PubMed]
- Meléndez B, Van Campenhout C, Rorive S, et al. Methods of measurement for tumor mutational burden in tumor tissue. Transl Lung Cancer Res 2018;7:661-7. [Crossref] [PubMed]
- Hugo W, Zaretsky JM, Sun L, et al. Genomic and Transcriptomic Features of Response to Anti-PD-1 Therapy in Metastatic Melanoma. Cell 2016;165:35-44. [Crossref] [PubMed]
- Rizvi NA, Hellmann MD, Snyder A, et al. Cancer immunology. Mutational landscape determines sensitivity to PD-1 blockade in non-small cell lung cancer. Science 2015;348:124-8. [Crossref] [PubMed]
- Rosenberg JE, Hoffman-Censits J, Powles T, et al. Atezolizumab in patients with locally advanced and metastatic urothelial carcinoma who have progressed following treatment with platinum-based chemotherapy: a single-arm, multicentre, phase 2 trial. Lancet 2016;387:1909-20. [Crossref] [PubMed]
- Thomas A, Routh ED, Pullikuth A, et al. Tumor mutational burden is a determinant of immune-mediated survival in breast cancer. Oncoimmunology 2018;7:e1490854. [Crossref] [PubMed]
- Park SE, Park K, Lee E, et al. Clinical implication of tumor mutational burden in patients with HER2-positive refractory metastatic breast cancer. Oncoimmunology 2018;7:e1466768. [Crossref] [PubMed]
- Barroso-Sousa R, Jain E, Cohen O, et al. Prevalence and mutational determinants of high tumor mutation burden in breast cancer. Ann Oncol 2020;31:387-94. [Crossref] [PubMed]
- DeNardo DG, Coussens LM. Inflammation and breast cancer. Balancing immune response: crosstalk between adaptive and innate immune cells during breast cancer progression. Breast Cancer Res 2007;9:212. [Crossref] [PubMed]
- Dunn GP, Bruce AT, Ikeda H, et al. Cancer immunoediting: from immunosurveillance to tumor escape. Nat Immunol 2002;3:991-8. [Crossref] [PubMed]
- Kim ST, Jeong H, Woo OH, et al. Tumor-infiltrating lymphocytes, tumor characteristics, and recurrence in patients with early breast cancer. Am J Clin Oncol 2013;36:224-31. [Crossref] [PubMed]
- Efremova M, Finotello F, Rieder D, et al. Neoantigens Generated by Individual Mutations and Their Role in Cancer Immunity and Immunotherapy. Front Immunol 2017;8:1679. [Crossref] [PubMed]
- Chan TA, Yarchoan M, Jaffee E, et al. Development of tumor mutation burden as an immunotherapy biomarker: utility for the oncology clinic. Ann Oncol 2019;30:44-56. [Crossref] [PubMed]
- Mei P, Freitag CE, Wei L, et al. High tumor mutation burden is associated with DNA damage repair gene mutation in breast carcinomas. Diagn Pathol 2020;15:50. [Crossref] [PubMed]
- Voutsadakis IA. High Tumor Mutation Burden and Other Immunotherapy Response Predictors in Breast Cancers: Associations and Therapeutic Opportunities. Target Oncol 2020;15:127-38. [Crossref] [PubMed]
- Xu J, Bao H, Wu X, et al. Elevated tumor mutation burden and immunogenic activity in patients with hormone receptor-negative or human epidermal growth factor receptor 2-positive breast cancer. Oncol Lett 2019;18:449-55. [Crossref] [PubMed]
- Karn T, Denkert C, Weber KE, et al. Tumor mutational burden and immune infiltration as independent predictors of response to neoadjuvant immune checkpoint inhibition in early TNBC in GeparNuevo. Ann Oncol 2020;31:1216-22. [Crossref] [PubMed]
- Barroso-Sousa R, Keenan TE, Pernas S. Tumor Mutational Burden and PTEN Alterations as Molecular Correlates of Response to PD-1/L1 Blockade in Metastatic Triple-Negative Breast Cancer. Clin Cancer Res 2020;26:2565-72. [Crossref] [PubMed]
- Lyu Z, Wang J, Kang L, et al. Lymph node metastasis and prognostic analysis of 354 cases of T1 breast cancer. Zhonghua Zhong Liu Za Zhi 2014;36:382-5. [PubMed]
- Montagna E, Viale G, Rotmensz N, et al. Minimal axillary lymph node involvement in breast cancer has different prognostic implications according to the staging procedure. Breast Cancer Res Treat 2009;118:385-94. [Crossref] [PubMed]
- Sakorafas GH, Geraghty J, Pavlakis G. The clinical significance of axillary lymph node micrometastases in breast cancer. Eur J Surg Oncol 2004;30:807-16. [Crossref] [PubMed]
- Galon J, Angell HK, Bedognetti D, et al. The continuum of cancer immunosurveillance: prognostic, predictive, and mechanistic signatures. Immunity 2013;39:11-26. [Crossref] [PubMed]
- Mlecnik B, Bindea G, Pagès F, et al. Tumor immunosurveillance in human cancers. Cancer Metastasis Rev 2011;30:5-12. [Crossref] [PubMed]
- Fridman WH, Zitvogel L, Sautès-Fridman C, et al. The immune contexture in cancer prognosis and treatment. Nat Rev Clin Oncol 2017;14:717-34. [Crossref] [PubMed]
- Zuckerman NS, Yu H, Simons DL, et al. Altered local and systemic immune profiles underlie lymph node metastasis in breast cancer patients. Int J Cancer 2013;132:2537-47. [Crossref] [PubMed]
- Kohrt HE, Nouri N, Nowels K, et al. Profile of immune cells in axillary lymph nodes predicts disease-free survival in breast cancer. PLoS Med 2005;2:e284. [Crossref] [PubMed]
- Galuppini F, Dal Pozzo CA, Deckert J, et al. Tumor mutation burden: from comprehensive mutational screening to the clinic. Cancer Cell Int 2019;19:209. [Crossref] [PubMed]
- De Mattos-Arruda L, Blanco-Heredia J. New emerging targets in cancer immunotherapy: the role of neoantigens. ESMO Open 2020;4:e000684. [Crossref] [PubMed]
- Duan S, Nordmeier S, Byrnes AE, et al. Extracellular Vesicle-Mediated Purinergic Signaling Contributes to Host Microenvironment Plasticity and Metastasis in Triple Negative Breast Cancer. Int J Mol Sci 2021;22:597. [Crossref] [PubMed]
- Kanwar N, Carmine-Simmen K, Nair R, et al. Amplification of a calcium channel subunit CACNG4 increases breast cancer metastasis. EBioMedicine 2020;52:102646. [Crossref] [PubMed]
- Miwa S, Yano S, Zhang Y, et al. Tumor-targeting Salmonella typhimurium A1-R prevents experimental human breast cancer bone metastasis in nude mice. Oncotarget 2014;5:7119-25. [Crossref] [PubMed]
- Singh R, Pochampally R, Watabe K, et al. Exosome-mediated transfer of miR-10b promotes cell invasion in breast cancer. Mol Cancer 2014;13:256. [Crossref] [PubMed]
- Lin CY, Beattie A, Baradaran B, et al. Contradictory mRNA and protein misexpression of EEF1A1 in ductal breast carcinoma due to cell cycle regulation and cellular stress. Sci Rep 2018;8:13904. [Crossref] [PubMed]
- Li X, Chen N, Zhou L, et al. Genome-wide target interactome profiling reveals a novel EEF1A1 epigenetic pathway for oncogenic lncRNA MALAT1 in breast cancer. Am J Cancer Res 2019;9:714-29. [PubMed]
- Qi H, Ning L, Yu Z, et al. Proteomic Identification of eEF1A1 as a Molecular Target of Curcumol for Suppressing Metastasis of MDA-MB-231 Cells. J Agric Food Chem 2017;65:3074-82. [Crossref] [PubMed]
- Yen MC, Huang YC, Kan JY, et al. S100B expression in breast cancer as a predictive marker for cancer metastasis. Int J Oncol 2018;52:433-40. [PubMed]
- Mazumdar A, Tahaney WM. The phosphatase PPM1A inhibits triple negative breast cancer growth by blocking cell cycle progression. NPJ Breast Cancer 2019;5:22. [Crossref] [PubMed]
- Goryca K, Kulecka M, Paziewska A, et al. Exome scale map of genetic alterations promoting metastasis in colorectal cancer. BMC Genet 2018;19:85. [Crossref] [PubMed]