Identification of prognostically relevant gene signatures in lung squamous cell carcinoma by Lung Cancer Explorer
Introduction
Immunotherapy or combination therapy based on PD1/PD-L1 checkpoint blockers has facilitated a relatively long-term survival stage (1,2) for non-small-cell lung cancer patients. However, due to the limited overall response rates of such immune checkpoint inhibitors (ICIs) for lung squamous cell carcinoma (LUSC) patients regardless of PD-L1 expression status (3), as well as the economic inaccessibility of those drugs in many countries (4,5), most patients with LUSC still must receive first-line chemotherapy due to a lack of precise targeting therapies that have been widely used for lung adenocarcinoma (6). Hence, it is imperative and urgent to identify key genes for the prognosis and treatment of LUSC.
The Cancer Genomics Atlas (TCGA) project and Gene Expression Omnibus (GEO) have emerged as promising tools for detection of genetic aberrations in tumorigenesis and have enabled researchers to seek potential targets for multiple cancer types, including LUSC. However, the limited sample numbers collected in each project, the significant heterogenicity among samples in each dataset and the large variations among projects due to the inconsistent platforms and probes used have remarkably restricted the credibility of the obtained results (7). Indeed, several researchers attempted to find key genes by looking at common differentially expressed genes (DEGs) in different datasets (8,9). However, that approach might somehow decrease the number of identified DEGs due to different experimental methods or conditions as well as limited sample numbers and gene numbers in one or several projects. Additionally, most papers exploring the transcriptional landscape have only focused on genes with increased expression in tumour tissues and poor outcomes in LUSC patients, giving a restricted view of the whole landscape.
Lung Cancer Explorer (LCE) is a web tool designed by UT Southwestern’s Quantitative Biomedical Research Center (QBRC) (10) that facilitates meta-analysis of datasets from TCGA, GEO and the individual literature with gene expression data. This makes the results more reliable and likely to be repeated due to its careful process, quality control, clinical information standardization and rigorous data curation. Thereafter, we explored the functional and molecular features of LUSC by looking into the systematic meta-analysis results of tumour-normal differential expression data and survival association with the gene expression data provided by the LCE web tool. DEGs and prognostically relevant genes (PRGs) were determined, and common genes, as both DEGs and PRGs, were subsequently categorized. Gene Ontology (GO) and Kyoto Encyclopedia of Genes and Genomes (KEGG) enrichment analyses were performed to explore the functional and pathway variations. Finally, the hub genes in each category were identified. We further tested the results for their survival correlation and expression differentiation via univariate and multivariate Cox regression analysis using the KM Plotter website (11) and quantitative real-time polymerase chain reaction (PCR) comparison of LUSC and adjacent tissue samples, respectively. In addition, the associations of those genes with recurrence-free survival (RFS) were also examined in LUSC. We present the following article in accordance with the MDAR checklist (available at http://dx.doi.org/10.21037/tcr-21-222).
Methods
Data collection
We downloaded the tumour-normal gene expression difference profile and survival association with the gene expression profile of LUSC from the LCE web tool, which collected a total of 56 datasets including TCGA and various GEO datasets focusing on lung cancer patients, and separately performed meta-analyses for lung adenocarcinoma (LUAD) and LUSC. In brief, only studies with at least 10 samples in each group were included in the gene expression difference meta-analysis, and only studies with at least 10 samples with survival data were included in the survival association with gene expression meta-analysis. Both types of meta-analysis were conducted only for genes with data available from at least 3 qualifying studies. Detailed information can be found on the website lce.biohpc.swmed.edu/lungcancer/. For survival association meta-analysis, the R package ‘meta’ (12) was applied to calculate the summary hazard ratio (HR) from the HRs of individual datasets. For tumour vs. normal gene expression meta-analysis, the summary standardized mean difference (tumour-normal) was calculated with the R package ‘metafor’ (13) using Hedges’ G as an effect size metric.
Identification of DEGs and PRGs
DEGs between LUSC and normal samples were selected from the tumour-normal gene expression profile with the cut-off criteria defined as P value <0.05 and the absolute value of standard mean difference (SMD) >1. PRGs were screened from the survival association with the gene expression profile using the cut-off criteria set as |HR – 1| >0.1 and P value <0.05. Common genes found in both DEGs and PRGs using the Venn diagram were further allocated into 4 groups according to their SMD and HR values: low-pro (SMD <−1 and HR <0.9), high-pro (SMD >1 and HR <0.9), low-worsen (SMD <−1 and HR >1.1), and high-worsen (SMD >1 and HR >1.1). Detailed raw data and filtered data were listed in https://cdn.amegroups.cn/static/public/tcr-21-222-1.xlsx.
Functional and pathway analysis
GO biological process (BP) functional enrichment analysis and KEGG pathway enrichment analysis of the four identified gene sets were performed using the ‘ClusterProfiler’ R package (14). The top 8 ontology or pathway terms enriched in each gene set that we identified were visualized using bubble plots. An adjusted P value <0.05 was considered statistically significant.
PPI network construction and hub gene selection
The protein-protein interaction (PPI) network of each identified gene signature was visualized using the Search Tool for the Retrieval of Interacting Genes (STRING, https://string-db.org/), and interactions with a combined score >0.15 were extracted and analysed using Cytoscape software (Version 3.71). CytoHubba, a Cytoscape plugin, was used to rank the genes in the network by the ‘degree’ method. The top 10 genes were visualized and recognized as hub genes, and the top 3 genes were selected for further analysis.
Survival analysis and clinical comparison of hub genes
The KM plotter, another web tool that predicts the survival association of cancer-related genes in various cancer types according to their mRNA levels via the integration of multiple gene chip datasets, was employed to test our selected hub genes. The analysis was restricted to lung squamous cell carcinoma, and patients were divided into two groups according to the best cut-off values of hub genes and median value respectively. Univariate Cox regression analysis of each finally selected hub gene was performed, the corresponding hazard ratio with 95% confidence interval (CI) and log-rank P value was calculated, and multivariate Cox regression analysis was further applied to test the independence of each gene when mixed with clinical characteristics, including stage, gender and smoking history. In total, 524 LUSC patients were enrolled for overall survival (OS) analysis, and 141 LUSC patients were included for time to first progression (FP) analysis. P<0.05 was considered statistically significant.
Quantitative real-time PCR
The study was conducted in accordance with the Declaration of Helsinki (as revised in 2013). Surgical specimens from 5 LUSC patients were collected from the Specimen Bank of Hangzhou First People’s Hospital with approval by the Institutional Review Board of Hangzhou First People’s Hospital {[2015](043)-01}. Informed consent was waived since this study was observational and presents no more than minimal risk of harm to subjects and involves no procedures for which written consent is normally required outside the research context. Total RNA was extracted from 30 mg tissue with the AxyPrep Multisource RNA Miniprep Kit according to the manufacturer’s protocol (Axygen, Tewksbury, MA, USA). cDNA from 1 µg RNA was prepared using the PrimeScriptTM RT Reagent Kit (Takara, Japan). Quantitative real-time PCR (RT-qPCR) was performed using TB Green Premix Ex Taq (Takara, Japan) and a 7500 System (Applied Biosystems, Singapore). All RT-qPCR assays were carried out in 3 biological replicates, and the average cycle threshold (CT) was used. Target genes were normalized using the RPLP0 gene, and the relative expression of the target genes was calculated as follows: 2−ΔCT, where ΔCT = Avg. CT (Target gene) − Avg. CT (RPLP0). The primers used for quantitative real-time PCR are listed in Table 1.
Table 1
Genes | Forward primer | Reverse primer |
---|---|---|
KARS | GTGGCAAAAGCTGTTGAATG | CCAGGAACTCCCCAACAAG |
PKM | ATAGTGAAGCCGGGACTGC | TGATGGGTGGTGAATCAATG |
LDHA | TTTTCCCAGTGAGTCACATCC | TTGGAAGAATTATGCACAAGACA |
CDC20 | ATGTGTGGCCTAGTGCTCCT | ATTGGACTGCCAGGGACA |
ENO1 | TCCCAACATCCTGGAGAATAA | ATGCCGATGACCACCTTATC |
MYO9A | TGTCTGTTGAGAGAGTACAGGACA | CTCTGCCGGTGCAACTACT |
RAB11A | AAATGAACAAAGATTGTGAAAGGA | CTGTTCCGCTAACCCATTAAA |
NTRK2 | AGTGCCTCTCGGATCTGGT | TTTCTGGTTTGCGATGAAAAT |
CD52 | GTCTCAGCCTTAGCCCTGTG | GAGGGGATTCTCTTGCGAGT |
Statistical analysis
The optimal statistical method of each comparison of data in the databases was selected according to the database itself. Survival risks were reported as hazard ratios [95% confidential intervals]. A paired t-test was used to compare the RT-qPCR results, and P<0.05 indicated that the difference was statistically significant.
Results
Identification of DEGs and PRGs in LUSC
The LCE website systematically provided a meta-analysis of tumour-normal standardized gene expression differences and a meta-analysis of survival associations with gene expression. According to the criteria of P<0.05 and |SMD| >1, a total of 6,102 DEGs were differentially expressed between tumour and normal tissues, including 3,072 upregulated genes and 3,030 downregulated genes (Figure 1A). The top 5 upregulated genes were ARNTL2, PKP3, MTHFD2, ORC5 and BZW2, and the top 5 downregulated genes were LHFP, GJA4, FAM162B, BTNL9 and VEGFD. After applying the criteria of P<0.05 and |HR-1| >0.1 to select genes highly associated with survival in LUSC, we found a total of 1738 PRGs containing 703 pro-survival genes and 1,035 worse-survival genes (Figure 1B). The top 5 worse-survival PRGs were ART3, CTTN, SHANK2, KRT7 and AZGP1. Subsequently, DRGs and PRGs were analysed with the Venn diagram tool, and a total of 506 genes were identified as both DRGs and PRGs (Figure 1C). When we looked closely at those genes, most genes (group low-pro, 36.96%, 187/506) had lower expression levels in tumours than in normal tissues, whereas increasing gene expression levels indicated worse survival in LUSC patients. The second-largest proportional gene sets (group high-pro, 27.87%, 141/506) were relatively abundant in tumour tissues compared with normal tissues, and higher expression of those genes predicted a longer survival. Seventy (13.83%) genes (group low-worsen) were decreased in tumours compared to normal lungs, and their expression was correlated with good survival; however, 108 (21.34%) genes (group high-worsen) were highly expressed in tumours but not in normal lungs, and accordingly, their expression was positively associated with short survival times (Figure 1D).
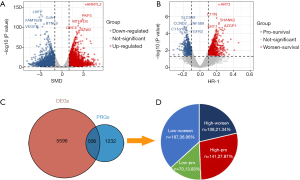
Functional and pathway analysis of identified gene signatures
GO BP and KEGG enrichment analyses of the 4 distinct gene sets were carried out to explore the intrinsic biological functions and critical pathways participating in tumorigenesis and aggressive growth or invasion. Low-pro genes were mainly enriched in ‘regulation of immune system process’, ‘defence response’, ‘regulation of immune response’ and ‘leukocyte activation’ in the BP category enrichment (adjusted P<0.05, Figure 2A). High-pro genes were mainly involved in ‘epithelium development’, ‘epithelial cell differentiation’, ‘skin development’, and ‘epidermis development’ processes (adjusted P<0.05, Figure 2B). However, for low-worsen genes, ‘small GTPase mediated signal transduction’ and ‘actin filament organization’ were predominant (adjusted P<0.05, Figure 2C), and for high-worsen genes, ‘Adenosine diphosphate (ADP) metabolic process’ and ‘nucleoside diphosphate metabolic process’, specifically ‘purine ribonucleoside diphosphate metabolic process’, were the top associated BP terms (adjusted P<0.05, Figure 2D). Unfortunately, the results of KEGG pathway analysis exhibited no significant findings for low-pro, high-pro and low-worsen genes, although most genes in those gene sets were categorized in the ‘malaria’ and ‘natural killer mediated cytotoxicity’, ‘regulation of actin cytoskeleton’ and ‘focal adhesion’ pathways, respectively (Figure 2E,F,G). High-pro genes were associated with metabolic pathways and significantly enriched with ‘glycolysis/gluconeogenesis’, ‘HIF-1 signalling pathway’, ‘carbon metabolism’ and ‘purine metabolism’ (adjusted P<0.05, Figure 2H).
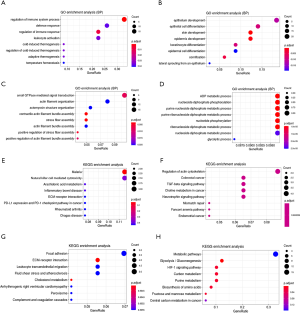
Hub gene selection
PPI analysis of these 4 gene signatures was performed using the STRING V11.0 tool and subsequently constructed with Cytoscape software. The CytoHubba plugin was employed to rank those network nodes by the ‘Degree’ method, and the top 10 nodes were selected as hub genes for further exploration (network shown in Figure 3A,B,C,D). To screen out genes with robust survival association, we further performed univariate Cox regression analysis on those genes in KM Plotter, another web tool that provided pooled analysis of multiple GEO datasets. As listed in Table 2, ITGAL, CD52, ARHGAP9, ITK and EVI2B in the low-pro group, as well as SOX2, NTRK2, LEF1 and TP63 in the high-pro group, were shown to favour survival. RAB11A, SHANK2, RERGL and MYO9A in the low-worsen group and ATIC, CDC20, ENO1, IPO4, KARS, LDHA, PFKP and PKM were consolidated as risks of survival. When we included each of these genes and clinical characteristics, including stage, gender, and smoking history for multivariate Cox regression analysis, only CD52 [HR (95% CI): 0.51 (0.32–0.82)] in the low-pro group and NTRK2 [HR (95% CI): 0.6 (0.39–0.93)] in the high-pro group could be independent favourable prognostic factors in LUSC. RAB11A [HR (95% CI): 2 (1.29–3.08)] and MYO9A [HR (95% CI): 1.76 (1.12–2.76)] in the low-worsen group, as well as CDC20 [HR (95% CI): 1.9 (1.21–2.97)], ENO1 [HR (95% CI): 1.63 (1.04–2.56)], KARS [HR (95% CI): 1.73 (1.06–2.84)], LDHA [HR (95% CI): 1.83 (1.16–2.86)], and PKM [HR (95% CI): 1.9 (1.25–2.89)] in the high-worsen group could be independent unfavourable prognostic factors for LUSC patients. Thereafter, a total of 9 genes were finally screened out that could predict survival independently as both DEGs and PRGs, representing different biological processes during cancer formation and proliferation that cause limited survival of LUSC patients.
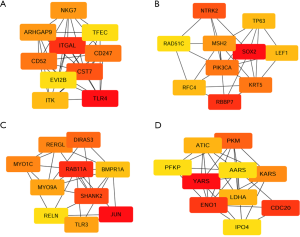
Table 2
Group | Probe | Symbol | Univariate | Multivariate (stage, gender, and smoking history included) | |||||||
---|---|---|---|---|---|---|---|---|---|---|---|
HR | Lower CI | Upper CI | P | HR | Lower CI | Upper CI | P | ||||
Lower pro group | 221060_s_at | TLR4 | 0.8 | 0.61 | 1.05 | 0.106 | 1.57 | 1 | 2.47 | 0.0523 | |
213475_s_at | ITGAL | 0.74 | 0.58 | 0.96 | 0.0226 | 0.8 | 0.52 | 1.24 | 0.3131 | ||
210140_at | CST7 | 0.75 | 0.56 | 1.01 | 0.0566 | 0.73 | 0.44 | 1.23 | 0.2373 | ||
210031_at | CD247 | 0.79 | 0.59 | 1.07 | 0.1231 | 0.81 | 0.5 | 1.31 | 0.3945 | ||
34210_at | CD52 | 0.67 | 0.52 | 0.88 | 0.0035 | 0.51 | 0.32 | 0.82 | 0.0054 | ||
213915_at | NKG7 | 0.8 | 0.62 | 1.04 | 0.1023 | 0.64 | 0.39 | 1.04 | 0.0731 | ||
224451_x_at | ARHGAP9 | 0.65 | 0.47 | 0.9 | 0.0091 | 0.47 | 0.17 | 1.34 | 0.1587 | ||
211339_s_at | ITK | 0.66 | 0.49 | 0.89 | 0.0069 | 0.63 | 0.39 | 1.02 | 0.0625 | ||
206715_at | TFEC | 0.84 | 0.64 | 1.1 | 0.21 | 0.77 | 0.45 | 1.3 | 0.3256 | ||
211742_s_at | EVI2B | 0.74 | 0.59 | 0.94 | 0.0144 | 0.68 | 0.44 | 1.06 | 0.09 | ||
Higher pro group | 213721_at | SOX2 | 0.71 | 0.56 | 0.89 | 0.0038 | 1.25 | 0.75 | 2.09 | 0.387 | |
201092_at | RBBP7 | 0.82 | 0.65 | 1.05 | 0.1202 | 0.81 | 0.5 | 1.33 | 0.409 | ||
221796_at | NTRK2 | 0.74 | 0.58 | 0.94 | 0.0123 | 0.6 | 0.39 | 0.93 | 0.0211 | ||
204369_at | PIK3CA | 0.81 | 0.64 | 1.02 | 0.075 | 1.65 | 1.02 | 2.68 | 0.0434 | ||
201820_at | KRT5 | 0.89 | 0.69 | 1.14 | 0.3458 | 0.6 | 0.38 | 0.93 | 0.0216 | ||
209421_at | MSH2 | 0.78 | 0.6 | 1 | 0.0535 | 1.74 | 1.12 | 2.71 | 0.0142 | ||
221558_s_at | LEF1 | 0.57 | 0.44 | 0.73 | 7.4E-06 | 0.66 | 0.42 | 1.04 | 0.0755 | ||
204023_at | RFC4 | 1.22 | 0.94 | 1.58 | 0.1355 | 1.37 | 0.88 | 2.12 | 0.1623 | ||
211834_s_at | TP63 | 0.71 | 0.55 | 0.91 | 0.0069 | 1.4 | 0.86 | 2.28 | 0.1727 | ||
209849_s_at | RAD51C | 0.85 | 0.66 | 1.1 | 0.2199 | 1.3 | 0.82 | 2.05 | 0.264 | ||
Lower worsen group | 201466_s_at | JUN | 1.22 | 0.95 | 1.58 | 0.1206 | 0.96 | 0.63 | 1.46 | 0.8455 | |
200863_s_at | RAB11A | 1.5 | 1.14 | 1.97 | 0.0038 | 2 | 1.29 | 3.08 | 0.0018 | ||
213307_at | SHANK2 | 1.47 | 1.12 | 1.93 | 0.0062 | 1.55 | 0.97 | 2.48 | 0.0692 | ||
215506_s_at | DIRAS3 | 1.18 | 0.93 | 1.49 | 0.1779 | 1.34 | 0.85 | 2.1 | 0.2112 | ||
220276_at | RERGL | 1.32 | 1.03 | 1.69 | 0.0295 | 1.48 | 0.92 | 2.36 | 0.1025 | ||
32811_at | MYO1C | 1.31 | 0.99 | 1.73 | 0.0552 | 1.83 | 1.2 | 2.79 | 0.0052 | ||
219027_s_at | MYO9A | 1.29 | 1.01 | 1.65 | 0.0445 | 1.76 | 1.12 | 2.76 | 0.0139 | ||
206271_at | TLR3 | 1.14 | 0.89 | 1.47 | 0.2928 | 1.52 | 0.96 | 2.41 | 0.0722 | ||
213578_at | BMPR1A | 1.2 | 0.94 | 1.52 | 0.1416 | 1.19 | 0.71 | 1.98 | 0.5125 | ||
205923_at | RELN | 1.18 | 0.91 | 1.52 | 0.2187 | 1.32 | 0.78 | 2.21 | 0.3023 | ||
Higher worsen group | 201000_at | AARS | 1.25 | 0.95 | 1.66 | 0.1124 | 1.6 | 1 | 2.57 | 0.0491 | |
208758_at | ATIC | 1.49 | 1.15 | 1.93 | 0.0025 | 1.53 | 0.99 | 2.36 | 0.0578 | ||
202870_s_at | CDC20 | 1.6 | 1.2 | 2.14 | 0.0014 | 1.9 | 1.21 | 2.97 | 0.0054 | ||
217294_s_at | ENO1 | 1.31 | 1.03 | 1.68 | 0.0291 | 1.63 | 1.04 | 2.56 | 0.0323 | ||
218305_at | IPO4 | 1.44 | 1.11 | 1.86 | 0.0056 | 1.41 | 0.9 | 2.22 | 0.1354 | ||
200840_at | KARS | 1.57 | 1.24 | 1.99 | 0.0002 | 1.73 | 1.06 | 2.84 | 0.0281 | ||
200650_s_at | LDHA | 1.34 | 1.05 | 1.71 | 0.0171 | 1.83 | 1.16 | 2.86 | 0.0088 | ||
201037_at | PFKP | 1.34 | 1.05 | 1.71 | 0.0204 | 1.42 | 0.91 | 2.21 | 0.1238 | ||
201251_at | PKM | 1.54 | 1.18 | 2 | 0.0013 | 1.9 | 1.25 | 2.89 | 0.0029 | ||
212048_s_at | YARS | 1.16 | 0.9 | 1.49 | 0.2628 | 0.72 | 0.45 | 1.13 | 0.1494 |
Association of identified genes with RFS
The independent correlations of these 9 genes with overall survival were already tested above, with the survival plots shown in Figure 4. We also wondered whether those genes were associated with RFS. As shown in Figure 5, CD52 indicated delayed recurrence [HR (95% CI): 0.48 (0.28–0.82)], and NTRK2 also tended to be correlated with worse RFS, although statistical significance was not achieved [HR (95% CI): 0.64 (0.38–1.08)]. RAB11A but not MYO9A was more obviously in favour of accelerated recurrence or progression [HR (95% CI): 1.86 (1.11–3.13), 1.52 (0.88, 2.62), respectively]. As high-worsen genes, PKM and KARS also suggested poor RFS [HR (95% CI): 1.9 (1.13–3.17), 2.38 (1.35–4.19)]. Surprisingly, we found that the expression of ENO1, as a key enzyme in aerobic glycolysis, implied a worse OS but an improved RFS for LUSC patients [HR (95% CI): 0.52 (0.31–0.89)].
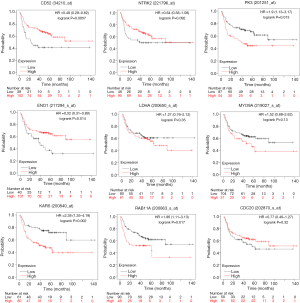
Hub gene expression validation
The mRNA expression level changes of these hub genes were further verified using paired cancer and adjacent tissues from 5 LUSC patients at our site. Consistent with the mRNA comparison results obtained from LCE, the mRNA level of NTRK2 (Figure 6A) was higher in cancer tissues than in adjacent tissues, and RAB11A (Figure 6B) and CD52 (Figure 6C) were significantly lower in cancer tissues than in adjacent tissues. However, no significant variations were found for other genes (Figure 6D,E,F,G,H,I).
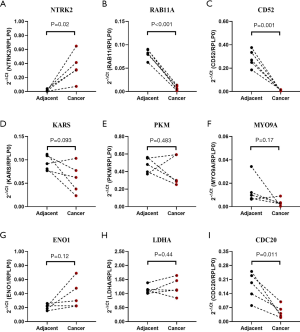
Discussion
In this study, we creatively examined the relationship between DEGs and PRGs and identified 4 distinct gene signatures showing varied functions and positive or negative correlations with survival in patients with LUSC through bioinformatics analysis.
Low-pro genes indicated that resected tumours tend to have attenuated immune defence responses and leukocyte activation, which expectedly contribute to the immune escape of lung squamous carcinoma cells. CD52, a glycosylphosphatidylinositol-anchored antigen found on the cell surfaces of lymphocytes (15), is rarely expressed on cancer cells, and its role in solid tumours remains largely unknown. Recently, CD52 expression in breast cancer tissues was found to be positively correlated with infiltrating immune cells (16). Our study identified that the mRNA level of CD52 was frequently decreased in LUSC tissues compared to normal tissues, which further indicated the poor immune microenvironment in lung squamous carcinoma.
High-pro genes were associated with epithelial development, which is critical for bronchial tissue regeneration and thereby highly risky to lose differentiation control and acquire oncogenic mutations in LUSC tissues. In particular, SOX2 and TP63, which are stem markers and lineage drivers in squamous carcinoma, were demonstrated to be oncogenic drivers and were commonly used to identify the pathological subtype between adenocarcinoma and squamous cell carcinoma (17,18). Similarly, NTRK2 was found to be a highly specific marker of squamous lung carcinoma compared to other pathological subtypes, including adenocarcinoma (19). These genes were found to be in favour of the overall survival of lung squamous cell carcinoma patients, which was demonstrated in earlier articles (19-21) and confirmed in our study. However, the underlying mechanisms remain unclear. Previously, RhoA activities were shown to inhibit cell proliferation and were commonly found to be suppressed by ΔNp63α, a dominant p63 isoform in LUSC (22), which might explain the oncogenic role of TP63 but not the favourable survival association. Thus, further molecular studies are suggested. Clinical administration of licenced NTRK inhibitors (23,24) in LUSC should be used with caution because positive IHC staining of NTRK2 might not represent oncogenic fusion (24,25); therefore, inhibition of NTRK2 in a specific population might not improve survival.
Low-worsen genes represent small GTPase-mediated signal transduction in LUSC tumours. The Ras-homologue (Rho) subfamily and Ras-related in the brain (Rab) subfamily belong to small GTPases, which are small molecular weight GTP-hydrolysing enzymes that actively bind and hydrolyse GTP to regulate key cellular processes that are also crucial for cancer cells, such as cell differentiation, proliferation and motility (26). The RhoA substrate JUN was reported to repress Lkb1 deficiency-induced lung squamous cell carcinoma progression (27). The relationship of MYO9A and LUSC has not been reported except for its family member MYO9B, which was identified as a key player in SLIT/ROBO-mediated lung tumour suppression (28). Both genes could negatively regulate the RhoA signalling pathway (28,29). Additionally, myosin members, including MYO9A, could mediate membrane trafficking of TGF-β, causing immune escape and metastasis (30), which might explain its negative correlation with patient outcomes, as found in our study. Overexpression of the Rab family member RAB11A was associated with advanced TNM stage and poor patient prognosis through regulation of YAP and inhibition of Hippo signalling (31). However, stemness pathways, including the Hippo signalling cascade, are critical for SCC tumour development (32).
High-worsen genes were frequently reported in scientific articles representing the highest potential to promote tumorigenesis and metastasis. Our study showed that high-worsen genes were mostly enriched in the ADP metabolic process, which includes many sub-terms, including the glycolysis process identified by KEGG pathway analysis. Aerobic glycolysis is characterized as a well-known hallmark of cancers and plays an essential role in cancer cell proliferation and metastasis (33-35), which also provides sufficient macromolecules for nucleotide anabolism through the pentose phosphate pathway (36). LUSC especially shows a critical reliance on glycolysis, which makes itself vulnerable to glycolytic inhibition, and LUAD exhibits significant glucose independence (37), implicating significant potential for the development of therapeutic strategies targeting glycolysis for LUSC because the existing options are clinically insufficient. PKM, PFKP, ENO1, and LDHA are key enzymes in the glycolysis process that generate instant ATP for cell proliferation. The ENO1 gene, which encodes enolase, was frequently amplified in LUSC. Zhang et al. showed that 87.5% (14/16) of patients with lung squamous cell carcinoma had higher ENO1 protein levels in tumour tissues than in paired lung tissues (38). The quantitative PCR results in our study only showed a tendency of upregulation, which might be due to the limited sample size. For PKM and LDHA, no obvious variation was found. However, many articles have demonstrated their role in lung cancer through regulation of metabolism (39,40).
In the LCE webtool, manually curated datasets were used for meta-analyses of gene expression differences or survival associations to avoid heterogenicity among datasets, which makes each gene comparison result more convincing and reproductive, as demonstrated by the team in another paper (41). Therefore, we selected the P value as a filter but did not adjust the P value to avoid false negative results. Survival analysis with the median value used as the dichotomization cut-off value for continuous gene expression is common; however, the gene expression distribution pattern is often unbalanced as a result of heterogeneous oncogenotypes (41). Thus, we chose the best cut-off value for each gene survival comparison in the KM Plotter analysis, and the P value was used. We admit that using the P value may increase the false positive rate when multiple comparisons are conducted. Thereby, gene survival association analysis in the KM plotter was also conducted with median value as the cut-off value, which also showed that CD52 and NTRK2 tended to promote survival while RAB11A, CDC20, and LDHA significantly worsen the survival (Table S1). However, the aim of this study was to explore the functional and molecular features of LUSC, and the genes identified in this work by bioinformatics analysis must be validated in large amount clinical samples.
By analysing the DEGs and PRGs screened from systematic results of meta-analysis of transcriptomics data of LUSC provided by the LCE database, we unmasked 4 gene signatures that were related with multifaceted biological features of lung squamous cell carcinoma and identified 9 hub genes consistently associated with overall survival in both pooled analysis by KM Plotter and meta-analysis by LCE web-tool, including NTRK2, RAB11A, and CD52. This information may provide visionary guides for finding diagnostic and therapeutic strategies to eliminate lung squamous cancer in human bodies.
Acknowledgments
Funding: This work was supported by
Footnote
Reporting Checklist: The authors have completed the MDAR checklist. Available at http://dx.doi.org/10.21037/tcr-21-222
Data Sharing Statement: Available at http://dx.doi.org/10.21037/tcr-21-222
Conflicts of Interest: All authors have completed the ICMJE uniform disclosure form (available at http://dx.doi.org/10.21037/tcr-21-222). The authors have no conflicts of interest to declare.
Ethical Statement: The authors are accountable for all aspects of the work in ensuring that questions related to the accuracy or integrity of any part of the work are appropriately investigated and resolved. The study was conducted in accordance with the Declaration of Helsinki (as revised in 2013). The study was approved by the Institutional Review Board of Hangzhou First People’s Hospital ([2015](043)-01). Informed consent was waived since this study was observational and presents no more than minimal risk of harm to subjects and involves no procedures for which written consent is normally required outside the research context.
Open Access Statement: This is an Open Access article distributed in accordance with the Creative Commons Attribution-NonCommercial-NoDerivs 4.0 International License (CC BY-NC-ND 4.0), which permits the non-commercial replication and distribution of the article with the strict proviso that no changes or edits are made and the original work is properly cited (including links to both the formal publication through the relevant DOI and the license). See: https://creativecommons.org/licenses/by-nc-nd/4.0/.
References
- de Silva M, Itchins M, Pavlakis N. Breakthrough 5-year survival with pembrolizumab in Keynote-001 study: horizon shifting in advanced non-small cell lung cancer with immune check point inhibition. Ann Transl Med 2020;8:555. [Crossref] [PubMed]
- Chen RL, Zhou JX, Cao Y, et al. The efficacy of PD-1/PD-L1 inhibitors in advanced squamous-cell lung cancer: a meta-analysis of 3112 patients. Immunotherapy 2019;11:1481-90. [Crossref] [PubMed]
- Pacheco JM. KEYNOTE-407: changing the way we treat stage IV squamous non-small cell lung cancer. Transl Lung Cancer Res 2020;9:148-53. [Crossref] [PubMed]
- Nesline MK, Knight T, Colman S, et al. Economic Burden of Checkpoint Inhibitor Immunotherapy for the Treatment of Non–Small Cell Lung Cancer in US Clinical Practice. Clin Ther 2020;42:1682-98.e7. [Crossref] [PubMed]
- Vorobiof DA, Hasid L, Litvin A, et al. 1612P Real-world evidence data (RWED) of financial toxicity (FT) in cancer patients (pts) receiving immunotherapy drugs (IOT). Ann Oncol 2020;31:S969. [Crossref]
- Yuan M, Huang LL, Chen JH, et al. The emerging treatment landscape of targeted therapy in non-small-cell lung cancer. Signal Transduct Target Ther 2019;4:61. [Crossref] [PubMed]
- Kulasingam V, Diamandis EP. Strategies for discovering novel cancer biomarkers through utilization of emerging technologies. Nat Clin Pract Oncol 2008;5:588-99. [Crossref] [PubMed]
- Li Y, Gu J, Xu F, et al. Transcriptomic and functional network features of lung squamous cell carcinoma through integrative analysis of GEO and TCGA data. Sci Rep 2018;8:15834. [Crossref] [PubMed]
- Man J, Zhang X, Dong H, et al. Screening and identification of key biomarkers in lung squamous cell carcinoma by bioinformatics analysis. Oncol Lett 2019;18:5185-96. [Crossref] [PubMed]
- Cai L, Lin S, Girard L, et al. LCE: an open web portal to explore gene expression and clinical associations in lung cancer. Oncogene 2019;38:2551-64. [Crossref] [PubMed]
- Győrffy B, Surowiak P, Budczies J, et al. Online survival analysis software to assess the prognostic value of biomarkers using transcriptomic data in non-small-cell lung cancer. PLoS One 2013;8:e82241. [Crossref] [PubMed]
- Guido S. Meta: An R package for meta-analysis. R News 2007;7:40-5.
- Viechtbauer W. Conducting meta-analyses in R with the metafor package. J Stat Softw 2010;36:1-48. [Crossref]
- Yu G, Wang LG, Han Y, et al. clusterProfiler: an R package for comparing biological themes among gene clusters. OMICS 2012;16:284-7. [Crossref] [PubMed]
- Saito Y, Nakahata S, Yamakawa N, et al. CD52 as a molecular target for immunotherapy to treat acute myeloid leukemia with high EVI1 expression. Leukemia 2011;25:921-31. [Crossref] [PubMed]
- Wang J, Zhang G, Sui Y, et al. CD52 Is a Prognostic Biomarker and Associated With Tumor Microenvironment in Breast Cancer. Front Genet 2020;11:578002. [Crossref] [PubMed]
- Guan Y, Wang G, Fails D, et al. Unraveling cancer lineage drivers in squamous cell carcinomas. Pharmacol Ther 2020;206:107448. [Crossref] [PubMed]
- Moses MA, George AL, Sakakibara N, et al. Molecular Mechanisms of p63-Mediated Squamous Cancer Pathogenesis. Int J Mol Sci 2019;20:3590. [Crossref] [PubMed]
- Terry J, De Luca A, Leung S, et al. Immunohistochemical expression of neurotrophic tyrosine kinase receptors 1 and 2 in lung carcinoma: potential discriminators between squamous and nonsquamous subtypes. Arch Pathol Lab Med 2011;135:433-9. [Crossref] [PubMed]
- Ma Y, Fan M, Dai L, et al. Expression of p63 and CK5/6 in early-stage lung squamous cell carcinoma is not only an early diagnostic indicator but also correlates with a good prognosis. Thorac Cancer 2015;6:288-95. [Crossref] [PubMed]
- Wilbertz T, Wagner P, Petersen K, et al. SOX2 gene amplification and protein overexpression are associated with better outcome in squamous cell lung cancer. Mod Pathol 2011;24:944-53. [Crossref] [PubMed]
- Abraham CG, Ludwig MP, Andrysik Z, et al. ΔNp63α Suppresses TGFB2 Expression and RHOA Activity to Drive Cell Proliferation in Squamous Cell Carcinomas. Cell Rep 2018;24:3224-36. [Crossref] [PubMed]
- Lassen U. Entrectinib for ROS1 fusion-positive NSCLC and NTRK fusion-positive solid tumours. Lancet Oncol 2020;21:193-4. [Crossref] [PubMed]
- Haratake N, Seto T. NTRK fusion-positive non-small-cell lung cancer -The diagnosis and targeted therapy. Clin Lung Cancer 2021;22:1-5. [Crossref] [PubMed]
- Gautschi O, Bubendorf L, Leyvraz S, et al. Challenges in the Diagnosis of NTRK Fusion-Positive Cancers. J Thorac Oncol 2020;15:e108-10. [Crossref] [PubMed]
- Citi S, Spadaro D, Schneider Y, et al. Regulation of small GTPases at epithelial cell-cell junctions. Mol Membr Biol 2011;28:427-44. [Crossref] [PubMed]
- Liu J, Wang T, Creighton CJ, et al. JNK1/2 represses Lkb1-deficiency-induced lung squamous cell carcinoma progression. Nat Commun 2019;10:2148. [Crossref] [PubMed]
- Kong R, Yi F, Wen P, et al. Myo9b is a key player in SLIT/ROBO-mediated lung tumor suppression. J Clin Invest 2015;125:4407-20. [Crossref] [PubMed]
- Omelchenko T, Hall A. Myosin-IXA regulates collective epithelial cell migration by targeting RhoGAP activity to cell-cell junctions. Curr Biol 2012;22:278-88. [Crossref] [PubMed]
- Chung CL, Tai SB, Hu TH, et al. Roles of Myosin-Mediated Membrane Trafficking in TGF-β Signaling. Int J Mol Sci 2019;20:3913. [Crossref] [PubMed]
- Dong Q, Fu L, Zhao Y, et al. Rab11a promotes proliferation and invasion through regulation of YAP in non-small cell lung cancer. Oncotarget 2017;8:27800-11. [Crossref] [PubMed]
- Maehama T, Nishio M, Otani J, et al. The Role of Hippo-YAP Signaling in Squamous Cell Carcinomas. Cancer Sci 2021;112:51-60. [Crossref] [PubMed]
- DeBerardinis RJ, Chandel NS. Fundamentals of cancer metabolism. Sci Adv 2016;2:e1600200. [Crossref] [PubMed]
- Pavlova NN, Thompson CB. The emerging hallmarks of cancer metabolism. Cell Metab 2016;23:27-47. [Crossref] [PubMed]
- Lunt SY, Vander Heiden MG. Aerobic glycolysis: meeting the metabolic requirements of cell proliferation. Annu Rev Cell Dev Biol 2011;27:441-64. [Crossref] [PubMed]
- Patra KC, Hay N. The pentose phosphate pathway and cancer. Trends Biochem Sci 2014;39:347-54. [Crossref] [PubMed]
- Goodwin J, Neugent ML, Lee SY, et al. The distinct metabolic phenotype of lung squamous cell carcinoma defines selective vulnerability to glycolytic inhibition. Nat Commun 2017;8:15503. [Crossref] [PubMed]
- Zhang Y, Li M, Liu Y, et al. ENO1 protein levels in the tumor tissues and circulating plasma samples of non-small cell lung cancer patients. Zhongguo Fei Ai Za Zhi 2010;13:1089-93. [PubMed]
- Lv J, Zhou Z, Wang J, et al. Prognostic Value of Lactate Dehydrogenase Expression in Different Cancers: A Meta-Analysis. Am J Med Sci 2019;358:412-21. [Crossref] [PubMed]
- Wang C, Zhang S, Liu J, et al. Secreted Pyruvate Kinase M2 Promotes Lung Cancer Metastasis through Activating the Integrin Beta1/FAK Signaling Pathway. Cell Rep 2020;30:1780-97.e6. [Crossref] [PubMed]
- Cai L, Luo D, Yao B, et al. Systematic Analysis of Gene Expression in Lung Adenocarcinoma and Squamous Cell Carcinoma with a Case Study of FAM83A and FAM83B. Cancers 2019;11:886. [Crossref] [PubMed]