Construction of a prognostic risk model of colorectal adenocarcinoma through integrated analysis of RNA-binding proteins
Introduction
Colorectal cancer (CRC) is the third most frequently diagnosed malignancy and one of the leading causes of cancer-related mortality worldwide (1). Colon adenocarcinoma (COAD) is a common type of CRC (2). Even with the significant progress that has been attained in recent COAD research, the data show that the morbidity and mortality rates of COAD are increasing (3). Findings have demonstrated that COAD can be successfully treated when identified at an early stage (4). In the pathogenesis of COAD, accumulation of genetic and epigenetic alterations transforms normal colonic epithelial cells to adenocarcinoma cells (5). Therefore, it is required to systematically study the genetic and epigenetic alterations in COAD to identify potential diagnostic markers and therapeutic targets.
RNA-binding protein (RBPs) regulates gene expression at the post-transcriptional level mainly through interaction with target RNA (6-8). A large number of studies have shown that RBPs participate in RNA metabolism and play an important role in the regulation of RNA stability, modification, localization, translation and alternative splicing (9,10). Moreover, a recent study found that RBPs can interact directly with chromatin to regulate gene expression at the epigenetic level (11).
Considering the extensive and important role of RBP in post-transcriptional regulation, changes in RBP expression will inevitably lead to the occurrence of various diseases. A total of 1,542 RBPs have been identified by high throughput screening in human cells, however, the role of these RBPs in the occurrence and development of COAD has not been examined (12).
In this study, we obtained COAD expression profile data through TCGA database. Through preprocessing and differential analysis of the data, we obtained RBPs that were differentially expressed in COAD patients and normal samples. Then, the Cox analysis was performed to determine the RBPs that are significantly related to COAD survival, and a COAD risk model was then constructed. Gene ontology (GO) and Kyoto Encyclopedia of Genes and Genomes (KEGG) pathway enrichment analyses of the risk model genes were then carried out to reveal the potential functional mechanism of RBPs in COAD. Finally, the expression level of the risk model genes was identified, and it was determined that some genes were appropriate for use as potential prognostic biomarkers. We present the following article in accordance with the TRIPOD reporting checklist (available at http://dx.doi.org/10.21037/tcr-21-40).
Methods
The study was conducted in accordance with the Declaration of Helsinki (as revised in 2013).
Patient and public involvement
The data of all cancer samples and normal samples in this study are from The Cancer Genome Atlas (TCGA), and all the data in this database is publicly available for anyone in the research community to use.
Dissemination policy
The results of the trial will be widely disseminated to health professionals, commissioners, policy-makers, colorectal adenocarcinoma (COAD) patient and the general public. The study results will be disseminated to a wide clinical audience through publication in a high-impact international scientific journal.
Identification of differentially expressed RBPs
The transcriptome data and corresponding clinical information for 473 COAD samples and 41 normal samples were downloaded from TCGA. The transcriptome data were preprocessed using the Bioconductor-limma R package (13). False discovery rate <0.05 and |log2 fold change (FC)| ≥1 were used as the criteria for differential RPBs (DE-RPBs) screening. The average count value of all DE-RPBs was greater than 1.
GO and KEGG functional enrichment analyses
The biological functions of these DE-RPBs including molecular functions, biological processes and cellular components, were systematically studied by GO enrichment (14). The potential biological pathways of DE-RPBs were detected using KEGG database (15). All GO and KEGG pathway enrichment analyses were carried out using the Bioconductor-cluster Profiler and enrichplot packages with a P value less than 0.05 (16).
PPI network construction and module screening
START (https://string-db.org/) was used to detect the protein-protein interaction (PPIs) among all DE-RPBs, and the network was constructed using Cytoscape 3.8.0 software (17). Then the subnetwork was selected from PPI network by using MCODE (Molecular Complex Detection) plugin and Cytoscape. P value <0.05 was considered significant.
Prognostic model construction and survival analysis
Univariate Cox regression analysis was used to evaluate the prognostic value of DE-RPBs. DE-RPBs related to overall survival time (P<0.05) was selected DE-RPBs related to overall survival time (P<0.05) was selected for subsequent analysis. A risk signature was formulated according to the multivariate Cox proportional hazards regression analyses. The risk-score for each COAD patient was calculated as follows:
Risk-score = beta-gene1 × exp-gene1 + beta-gene2 × exp-gene2 + beta-gene × exp-gene
The samples were randomly divided into the train group and the test group, and the train group and the test group were divided into the high-risk group and the low-risk group according to the middle risk value. Beta-gene represents the regression coefficient that derived from the multivariate Cox regression analysis. Exp-gene represents the expression of genes.
Independent prognostic analysis
The patient’s age, gender, cancer stage, and other information was preprocessed and then integrated with the risk value. Then, single-factor and multi-factor independent prognostic analyses of the train group and test group were performed by utilizing the “survival” package in R (version 3.6.3).
Nomogram construction
A nomogram for individualized prediction of overall survival was generated based on the results of the multivariate analysis (Cox model) to predict 1-, 2-, and 3-year overall survival. The nomogram plots were generated using the “rms” package of R software (version 3.6.3). The total points were calculated, and the 1-, 2-, and 3-year patient survival rates were predicted based on the total points.
Prognostic model gene expression validation
The expression levels of prognostic model genes in COAD and normal samples were verified by the Human Protein Atlas (HPA) database. This is a web-based database that provides protein expression in normal samples, cancer samples, cells, and blood.
Statistical analysis
Most of the statistical analyses were performed using the bioinformatic tools mentioned above. When we conducted differential expression analysis, only RBPs with |log2 FC| ≥1 and P<0.05 were considered as statistically significant. The univariate and multivariate Cox regression analyses were performed utilizing the “survival” package in R (version 3.6.3). Cox P<0.05 was regarded as statistically significant for survival analysis. Boxplot generation were conducted using the R packages “ggplot2”, “ggpubr,” and “ggsignif”. A survival curve created using the R packages “survival” and “survminer” was utilized to estimate the differences in the overall survival between high-risk and low-risk COAD.
Results
Identification of differentially expressed RBPs
The transcriptome data and corresponding clinical information for 473 COAD samples and 41 normal samples were downloaded from TCGA. After preprocessing and difference analysis of the raw data of the 1,542 RBPs (12), we found that there were 123 upregulated and 54 downregulated RBPs (Figure 1A,B).
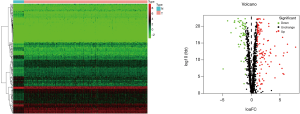
GO and KEGG enrichment analysis of differentially expressed RBPs
In order to explore the function and molecular mechanism of these differentially expressed RBPs, we performed GO and KEGG enrichment analysis on upregulated and downregulated RBPs, respectively. Upregulated differentially expressed RBPs (UP-DE-RBPs) were significantly enriched in biological processes associated with ncRNA processing, RNA phosphodiester bond hydrolysis, and ribosome biogenesis. In the cellular component enrichment analysis, UP-DE-RBPs were significantly enriched in cytoplasmic ribonucleoprotein granule, ribonucleoprotein granule, and nucleolar part. The molecular function analysis showed that UP-DE-RBPs were significantly enriched in catalytic activity, acting on RNA, ribonuclease activity, and translation regulator activity (Figure 2A,B). Downregulated differentially expressed RBPs (DOWN-DE-RBPs) were significantly enriched in biological processes associated with defense response to virus, regulation of cytoplasmic translation, and regulation of translation. In cellular component enrichment analysis, DOWN-DE-RBPs were significantly enriched in endolysosome membrane, apical dendrite, and mitochondrial matrix. The molecular function analysis showed that DOWN-DE-RBPs were significantly enriched in mRNA 3'-UTR AU-rich region binding, AU-rich element binding and mRNA 3'-UTR binding and (Figure 2C,D). In regard to the KEGG enrichment analysis, the UP-DE-RBPs were mainly enriched in ribosome biogenesis in eukaryotes, mRNA surveillance pathway, and RNA transport (Figure 2E,F). The DOWN-DE-RBPs were mainly enriched in progesterone-mediated oocyte maturation, oocyte meiosis, and hepatitis C (Figure 2G,H).
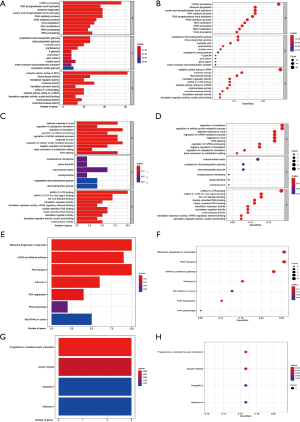
PPI network construction
In order to more clearly understand the mechanism of these DE-RBPs, we used the STRING database and Cytoscape software to construct a PPI network (Figure 3A). This PPI network contained a total of 145 nodes and 596 edges. Next, we used the plug-in MODE in Cytoscape to analyze the PPI network to identify the potential key subnetwork. Finally, we determined the first three important subnetworks. Subnetwork 1 included 21 nodes and 195 edges, subnetwork 2 included 13 nodes and 38 edges, and subnetwork 3 included 5 nodes and 10 edges. An overall analysis was conducted by integrating these three key subnetworks into a network (Figure 3B). The GO and KEGG analyses showed that the subnetwork 1 genes were mainly enriched in ncRNA processing, preribosome, snoRNA binding, and ribosome biogenesis in eukaryotes. The subnetwork 2 genes were significantly enriched in DNA alkylation, cytoplasmic ribonucleoprotein granule, and catalytic activity acting on RNA. The subnetwork 3 genes were significantly enriched in RNA splicing via transesterification reactions with bulged adenosine as nucleophile, perikaryon, and the mRNA 3'-UTR AU-rich region binding.
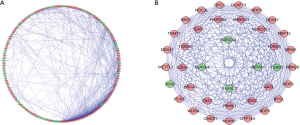
Prognosis-related RBP screening
We conducted a univariate Cox regression analysis of 177 differentially expressed RBPs and found that TDRD6, POP1, TDRD7, LUZP4, PPARGC1A, LIN28B, PABPC1L, LRRFIP2, ZC3H12C, RBM47, CELF4, and PNLDC1 were significantly related to survival (Figure 4A).
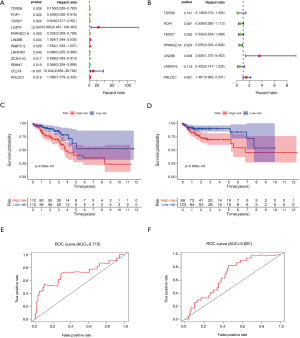
Prognosis-related genetic risk score model construction and validation
To further determine the RBPs with the greatest potential prognosis ability, multivariate Cox regression analyses were used to identify seven RBPs: TDRD6, POP1, TDRD7, PPARGC1A, LIN28B, LRRFIP2, and PNLDC1. The seven RBPs were analyzed and used to construct a predictive model (Figure 4B). The formula for calculating the risk-score of COAD patient is as follows:
Risk-score = (-1.9076×ExpTDRD6) + (-0.5828×ExpPOP1) + (-0.6433×ExpTDRD7) + (-0.5888×ExpPPARGC1A) + (1.2823×ExpLIN28B) + (-0.8568×ExpLRRFIP2) + (0.3925×ExpPNLDC1)
In order to evaluate the predictive ability of the model, we randomly divided 446 samples of COAD patients with detailed survival information into two groups, namely the train group and the test group. At the same time, we divided the train group and the test group into high-risk and low-risk groups according to the median risk score for survival analysis. In the train and test groups, patients in the high-risk group exhibited a significantly lower overall survival rate than low-risk group (Figure 4C,D). Then, we performed a time-dependent receiver operating characteristic (ROC) analysis to further evaluate the prognostic accuracy of this model. The AUC of the ROC curve for overall survival in the train and test groups was 0.715 and 0.681, respectively (Figure 4E,F). The risk-score, survival status, and expression heatmap of patients in the train and test groups are shown in Figure 5.
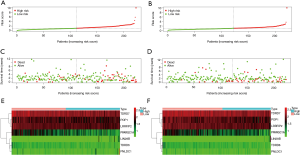
Independent prognostic analysis
In order to further explore the independent prognostic factors of COAD, we integrated the patient’s age, gender, cancer stage, risk value, and other information, and conducted single-factor and multi-factor independent prognostic analyses on the train group and the test group, respectively. The results are shown in Figure 6A,B. In the single-factor independent prognostic analysis of the train group and the test group, the P value of age and cancer stage was less than 0.05, indicating that these two factors are significantly related to the survival of COAD patients. In the multi-factor independent prognostic analysis, the P value of age and cancer stage was less than 0.05, indicating that these two factors can be used as independent prognostic factors for COAD patients.
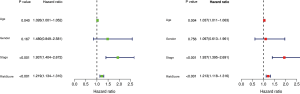
Nomogram construction
In order to more accurately predict the survival rate of COAD patients, we constructed a nomogram using the expression levels and points of model genes (Figure 7). By corresponding to the expression level and score of the Cox model genes, the total points of the patient can be calculated. Based on the total points, the patient’s predicted 1-, 2-, and 3-year survival rates are intuitively displayed.
Prognostic model gene expression validation
In order to further confirm the expression of key genes in COAD patients in the risk model, we used the Human Protein Atlas database to search for the expression of these seven genes. It was found that TDRD6, TDRD7, LIN28B, LRRFIP2, and PNLDC1 were significantly increased in COAD patients (Figure 8). At present, the database does not contain data related to POP1 or PPARGC1A protein expression.
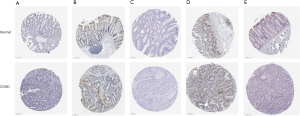
Discussion
COAD is a noncutaneous carcinoma that is common worldwide with high morbidity and mortality. Effective prevention methods are clearly underutilized. Both diagnostic and prognostic models that can precisely and accurately predict the status and survival time of COAD are urgently needed. In the field of COAD, there have been limited studies on molecular biomarkers that can predict disease status and prognosis (18). Through Cox analysis of TCGA COAD expression profile data, we constructed a risk model for seven genes, conducted a series of bioinformatics analyses on the basis of the risk model, and finally identified new diagnostic and prognostic markers for COAD.
The GO and KEGG enrichment analyses of these DE-RBPs showed that the UP-DE-RBPs were significantly enriched in RNA phosphodiester bond hydrolysis, RNA catabolic process, RNA stabilization, and translation regulator activity. The DOWN-DE-RBPs were significantly enriched for regulation of mRNA processing, regulation of mRNA metabolic process, RNA splicing, and mRNA 3'-UTR binding. Previous experiments have shown that RNA splicing, processing, localization, transport, and stability are directly related to the occurrence of COAD (19,20). Circular RNAs (circRNAs) and micro RNAs (miRNAs) are the most common products of RNA splicing, and a large number of studies have also shown that they play an important role in the occurrence of COAD (21-24). Previous experiments have shown that circRNA can specifically bind to RBP, which in turn affects the occurrence of disease (25,26). Therefore, we predict that these differentially expressed RBPs may intentionally bind with circRNA, thereby affecting the occurrence of COAD. The interaction between risk model genes and circRNA and its mechanism of action in COAD will be studied in follow-up work.
Subsequently, we used univariate Cox regression analysis to identify twelve RBPs that were significantly associated with COAD survival. Then, these were gradually reduced to seven using multivariate Cox regression analysis, and were subsequently used to construct a COAD risk model. The P values of the survival curves of the train group and the test group are both less than 0.01, indicating that there is a significant survival difference between the high-risk group and the low-risk group. This indicates that our risk model can be used to predict the prognosis of COAD. The ROC curve of the risk model shows that our model has better prediction accuracy (train group AUC =0.715 and test group AUC =0.681).
The COAD nomogram we constructed can predict 1-, 2-, and 3-year overall survival of COAD patients. According to the results of the model, the higher the score mean the lower overall survival, which provides important warning information for the treatment of patients. The genes in the nomogram can be used as the best target for our further study of the pathogenesis of COAD.
Finally, we conducted an immunohistochemical prediction of the expression levels of seven genes in the risk model and found that TDRD6, TDRD7, LIN28B, LRRFIP2, and PNLDC1 were significantly increased in COAD patients compared with normal colon tissue. Because the database has not yet included the immunohistochemical data of POP1 and PPARGC1A, we cannot ascertain the difference in expression between the two in patients and normal samples. The heat maps of the expression of these seven genes in the high-risk and low-risk groups of the training and test groups showed that high expression of TDRD6, TDRD7, LRRFIP2, PNLDC1, POP1, and PPARGC1A was associated with a good prognosis in patients with COAD, whereas that of LIN28B was related to poor prognosis.
LIN28B is highly expressed in primary tumors and various cancer cell lines, and numerous experiments have shown that LIN28B plays an important role in the occurrence of cancer (27-31). Studies have shown that high expression of LIN28B can promote the occurrence of COAD, cancer cell migration, and drug resistance (32,33). TDRD6, TDRD7, LRRFIP2, PNLDC1, POP1, and PPARGC1A are involved in RNA splicing and stability, but no previous studies have proved their relationship with COAD. Studies have shown that RBP can participate in the regulation of mRNA alternative splicing, regulation of mRNA stability and regulation of mRNA translation, thereby promoting the occurrence of cancer (34-36). Other studies have shown that RBP can play an anti-tumor effect by reducing the stability of the mRNA of anti-apoptotic proteins and inflammatory factors (37,38). These pathways cause different RBPs to play an antagonistic role in the occurrence of cancer. Our research provides a risk prediction model for COAD and also provides a new direction for in-depth study of the pathogenesis of COAD.
Although we performed detailed bioinformatics analyses on the expression data of TCGA COAD patients and normal samples, provided new prognostic indicators, and generated a COAD-RBPs-risk model, there are still deficiencies associated with the current study. First, the data are all from the TCGA database, and the sample data still requires further expansion. Second, we only analyzed the expression data, and after synthesizing other types of data, different situations may occur. Finally, we have not yet carried out experimental verification of the research results, which will be the focus of our follow-up research.
Conclusions
In conclusion, our comprehensive bioinformatics analysis examined the key RBP modules specifically associated with the overall survival of COAD patients. The constructed prognostic model and nomogram exhibited good predictive accuracy with regard to survival of COAD and these RBP genes could be used in clinical adjuvant treatments.
Acknowledgments
Funding: This research was funded by
Footnote
Reporting Checklist: The authors have completed the TRIPOD reporting checklist. Available at http://dx.doi.org/10.21037/tcr-21-40
Conflicts of Interest: All authors have completed the ICMJE uniform disclosure form (available at http://dx.doi.org/10.21037/tcr-21-40). The authors have no conflicts of interest to declare.
Ethical Statement: The authors are accountable for all aspects of the work in ensuring that questions related to the accuracy or integrity of any part of the work are appropriately investigated and resolved. The study was conducted in accordance with the Declaration of Helsinki (as revised in 2013).
Open Access Statement: This is an Open Access article distributed in accordance with the Creative Commons Attribution-NonCommercial-NoDerivs 4.0 International License (CC BY-NC-ND 4.0), which permits the non-commercial replication and distribution of the article with the strict proviso that no changes or edits are made and the original work is properly cited (including links to both the formal publication through the relevant DOI and the license). See: https://creativecommons.org/licenses/by-nc-nd/4.0/.
References
- Siegel RL, Miller KD, Jemal A. Cancer statistics, 2019. CA Cancer J Clin 2019;69:7-34. [Crossref] [PubMed]
- Barret M, Chaussade S, Coriat R. Adenoma detection rate and risk of colorectal cancer and death. N Engl J Med 2014;370:1298-306. [Crossref] [PubMed]
- Cai L, Bennedsen ALB, Qvortrup C, et al. Increasing incidence of colorectal cancer in young patients. Ugeskr Laeger 2019;30;182:V09190524.
- Roncucci L, Mariani F. Prevention of colorectal cancer: How many tools do we have in our basket? Eur J Intern Med 2015;26:752-6. [Crossref] [PubMed]
- Khare S, Verma M. Epigenetics of colon cancer. Methods Mol Biol 2012;863:177-85. [Crossref] [PubMed]
- Calabretta S, Richard S. Emerging roles of disordered sequences in RNA-binding proteins. Trends Biochem Sci 2015;40:662-72. [Crossref] [PubMed]
- Velasco MX, Kosti A, Penalva LOF, et al. The diverse roles of RNA-binding proteins in glioma development. Adv Exp Med Biol 2019;1157:29-39. [Crossref] [PubMed]
- Cooke A, Schwarzl T, Huppertz I, et al. The RNA-binding protein YBX3 controls amino acid levels by regulating SLC mRNA abundance. Cell Rep 2019;27:3097-106. [Crossref] [PubMed]
- Frisone P, Pradella D, Di Matteo A, et al. SAM68: Signal transduction and RNA metabolism in human cancer. Biomed Res Int 2015;2015:528954. [Crossref] [PubMed]
- Perron G, Jandaghi P, Solanki S, et al. A General Framework for Interrogation of mRNA Stability Programs Identifies RNA-Binding Proteins that Govern Cancer Transcriptomes. Cell Rep 2018;23:1639-50. [Crossref] [PubMed]
- Xiao R, Chen JY, Liang Z, et al. Pervasive Chromatin-RNA Binding Protein Interactions Enable RNA-Based Regulation of Transcription. Cell 2019;178:107-21. [Crossref] [PubMed]
- Gerstberger S, Hafner M, Tuschl T. A census of human RNA-binding proteins. Nat Rev Genet 2014;15:829-45. [Crossref] [PubMed]
- Ritchie ME, Phipson B, Wu D, et al. Limma powers differential expression analyses for RNA-sequencing and microarray studies. Nucleic Acids Res 2015;43:e47. [Crossref] [PubMed]
- Ashburner M, Ball CA, Blake JA, et al. Gene ontology: tool for the unification of biology. Nat Genet 2000;25:25-9. [Crossref] [PubMed]
- Kanehisa M, Goto S, Kawashima S, et al. The KEGG resource for deciphering the genome. Nucleic Acids Res 2004;32:D277-80. [Crossref] [PubMed]
- Yu G, Wang LG, Han Y, et al. ClusterProfiler: an R package for comparing biological themes among gene clusters. OMICS 2012;16:284-7. [Crossref] [PubMed]
- Von Mering C, Jensen LJ, Snel B, et al. STRING: known and predicted protein-protein associations, integrated and transferred across organisms. Nucleic Acids Res 2005;33:D433-7. [Crossref] [PubMed]
- Liu Z, Bai Y, Xie F, et al. Comprehensive analysis for identifying diagnostic and prognostic biomarkers in colon adenocarcinoma. DNA Cell Biol 2020;39:599-614. [Crossref] [PubMed]
- Hollander D, Donyo M, Atias N, et al. A network-based analysis of colon cancer splicing changes reveals a tumorigenesis-favoring regulatory pathway emanating from ELK1. Genome Res 2016;26:541-53. [Crossref] [PubMed]
- Amirkhah R, Naderi-Meshkin H, Shah JS, et al. The Intricate Interplay between Epigenetic Events, Alternative Splicing and Noncoding RNA Deregulation in Colorectal Cancer. Cells 2019;8:929. [Crossref] [PubMed]
- Ruan J. A robust circular RNA-based prognostic signature for postoperative recurrence in stage II/III colon cancer. AIMS Genet 2019;6:67-9. [Crossref] [PubMed]
- He JH, Han ZP, Luo JG, et al. Hsa-Circ-0007843 Acts as a mIR-518c-5p Sponge to Regulate the Migration and Invasion of Colon Cancer SW480 Cells. Front Genet 2020;25;11:9.
- Huang G, Ma J, Zhang L. Integrin subunit Alpha 5 (ITGA5) gene circular RNA sponges microRNA-107 in colorectal carcinoma cells and tissues and regulates the expression of the forkhead box J3 (FOXJ3) gene. Med Sci Monit 2020;26:e920623. [Crossref] [PubMed]
- He JH, Li YG, Han ZP, et al. The circRNA-ACAP2/hsa-miR-21-5p/tiam1 regulatory feedback circuit affects the proliferation, migration, and invasion of colon cancer SW480 Cells. Cell Physiol Biochem 2018;49:1539-50. [Crossref] [PubMed]
- Zang J, Lu D, Xu A. The interaction of circRNAs and RNA binding proteins: An important part of circRNA maintenance and function. J Neurosci Res 2020;98:87-97. [Crossref] [PubMed]
- Zhu YJ, Zheng B, Luo GJ, et al. Circular RNAs negatively regulate cancer stem cells by physically binding FMRP against CCAR1 complex in hepatocellular carcinoma. Theranostics 2019;9:3526-40. [Crossref] [PubMed]
- Kugel S, Sebastián C, Fitamant J, et al. SIRT6 suppresses pancreatic cancer through control of Lin28b. Cell 2016;165:1401-15. [Crossref] [PubMed]
- Ma L, Zhao Q, Chen W, et al. Oncogene Lin28B increases chemosensitivity of colon cancer cells in a let-7-independent manner. Oncol Lett 2018;15:6975-81. [Crossref] [PubMed]
- Ottaviani S, Stebbing J, Frampton AE, et al. TGF-β induces miR-100 and miR-125b but blocks let-7a through LIN28B controlling PDAC progression. Nat Commun 2018;9:1845. [Crossref] [PubMed]
- Qu TT, Chen F, Wang J, et al. PCAF-mediated acetylation of Lin28B increases let-7 biogenesis in lung adenocarcinoma H1299 cells. BMC Cancer 2018;18:27. [Crossref] [PubMed]
- Ji W, Diao YL, Qiu YR, et al. LINC00665 promotes breast cancer progression through regulation of the miR-379-5p/LIN28B axis. Cell Death Dis 2020;11:16. [Crossref] [PubMed]
- Madison BB, Liu Q, Zhong X, et al. LIN28B promotes growth and tumorigenesis of the intestinal epithelium via Let-7. Genes Dev 2013;27:2233-45. [Crossref] [PubMed]
- King CE, Cuatrecasas M, Castells A, et al. LIN28B promotes colon cancer progression and metastasis. Cancer Res 2011;71:4260-8. [Crossref] [PubMed]
- Pereira B, Billaud M, Almeida R. RNA-binding proteins in cancer: old players and new actors. Trends Cancer 2017;3:506-28. [Crossref] [PubMed]
- Gouble A, Grazide S, Meggetto F, et al. A new player in oncogenesis: AUF1/hnRNPD overexpression leads to tumorigenesis in transgenic mice. Cancer Res 2002;62:1489-95. [PubMed]
- Miles WO, Lembo A, Volorio A, et al. Alternative polyadenylation in triple-negative breast tumors allows NRAS and c-JUN to bypass PUMILIO posttranscriptional regulation. Cancer Res 2016;76:7231-41. [Crossref] [PubMed]
- Moore AE, Chenette DM, Larkin LC, et al. Physiological networks and disease functions of RNA-binding protein AUF1. Wiley Interdiscip Rev RNA 2014;5:549-64. [Crossref] [PubMed]
- Lal A, Mazan-Mamczarz K, Kawai T, et al. Concurrent versus individual binding of HuR and AUF1 to common labile target mRNAs. EMBO J 2004;23:3092-102. [Crossref] [PubMed]