Prognostic and immunological role of alpha-L-fucosidase 2 (FUCA2) in hepatocellular carcinoma
Highlight box
Key findings
• Increased alpha-L-fucosidase 2 (FUCA2) expression is associated with a poor prognosis and immune infiltration of hepatocellular carcinoma (HCC).
What is known and what is new?
• HCC is one of the leading causes of cancer related death worldwide. Research into HCC immune-associated genes and the immunological microenvironment enhances our knowledge of the mechanisms of carcinogenesis.
• We evaluated the significance of FUCA2 in the genesis and prognosis of HCC by analyzing gene expression, survival status, and immune infiltration correlations across many databases.
What is the implication, and what should change now?
• The implication of this study is that FUCA2 is a good prognostic biomarker and the likely molecular processes that influence prognosis in HCC. Based on the theoretical foundation this study provides, what should change is the upcoming cellular and animal investigations, which will advance HCC diagnosis and therapy.
Introduction
Hepatocellular carcinoma (HCC), whose incidence is increasing, is one of the top 5 deadliest illnesses, the seventh most prevalent type of illness in the United States, and the leading cause of cancer-related death worldwide (1,2). Annually, there are around 841,000 new cases of HCC and 782,000 deaths worldwide, with survival reported to be 6–20 months without treatment globally (3). HCC is the predominant (>90%) form of primary liver cancer, and is very prone to recurrence and dissemination, posing a significant threat to health (4,5). Recently, there has been considerable progress in the field of tumor immunity research. Immunotherapy provides an unparalleled opportunity to effectively treat cancer by stimulating the immune system to fight tumor development and progression (6,7). Research into HCC immune-associated genes and the immunological microenvironment enhances our knowledge of the mechanisms of carcinogenesis and may serve as a guide for the use of drugs or the development of new treatments (8).
Alpha-L-fucosidase 2 (FUCA2) is a member of the glycosyl hydrolase 29 family and has fucosidase activity; nevertheless, very few studies have been conducted so far to determine its function (9). FUCA2 is responsible for the removal of alpha-1,6-fucose attached to the N-acetylglucosamine residue of glycoproteins. FUCA2 can be used to identify and treat stomach cancer linked to Helicobacter pylori (10). In recent years, it has become apparent that serum FUCA2 may be a possible biomarker for the early detection of HCC (11). However, the connection between FUCA2 and HCC prognosis remains unclear. Further research is required to identify the role of FUCA2 in the genesis and progression of HCC.
Using integrated bioinformatics analysis, it is now possible to evaluate hundreds of relevant genes in several databases simultaneously (12). To the best of our knowledge, a bioinformatics study has not yet been conducted to determine the unique characteristics of FUCA2 in HCC. Moreover, it is yet to be determined how FUCA2 influences the immune microenvironment in HCC. In the present study, we evaluated the significance of FUCA2 in the genesis and prognosis of HCC by analyzing gene expression, survival status, and immune infiltration correlations across many databases. Using The Cancer Genome Atlas (TCGA) database, we found a high correlation between FUCA2 expression levels and the clinical features of patients with HCC. Therefore, we examined the signaling pathways associated with FUCA2 and correlated them with the immune infiltration of FUCA2 in patients with HCC. The aim of this study was to determine whether FUCA2 is a unique prognostic biomarker and the likely molecular processes that influence prognosis in liver cancer and thus to provide a theoretical foundation for our upcoming cellular and animal investigations, which we anticipate will advance HCC diagnosis and therapy. We present the following article in accordance with the TRIPOD reporting checklist (available at https://tcr.amegroups.com/article/view/10.21037/tcr-22-1850/rc).
Methods
Data collection
Data were collected from TCGA (https://portal.gdc.cancer.gov/), which includes RNA sequencing (RNA-seq) data on the expression of FUCA2 and clinical information from 370 HCC and 50 adjacent nontumor tissues. The relationship between gene expression and survival was evaluated using the R “survival” package (The R Foundation for Statistical Computing; https://www.rdocumentation.org/packages/survival/versions/3.4-0), whereas the “rms” package (https://cran.r-project.org/web/packages/rms/) was used to predict 1-, 3-, and 5-year survival rates by analyzing different variables. The study was conducted in accordance with the Declaration of Helsinki (as revised in 2013). The study was approved by the Institutional Ethics Board of Renmin Hospital of Wuhan University (No. WDRY2020-K223), and individual consent for this retrospective analysis was waived.
Gene Expression Profiling Interactive Analysis 2 (GEPIA2), Human Protein Atlas (HPA), and the University of Alabama Cancer (UALCAN) database
GEPIA2 integrates TCGA and genotype tissue expression data using shared pipelines. GEPIA2 was used in the present study to evaluate pan-cancer FUCA2 expression, gene association, and overall survival (OS). The HPA contains data on the transcriptomes of over 8,000 patients (13). In the present study, using the HPA, we undertook a proteome analysis using 26,941 antibodies to analyze 17,165 different proteins. The UALCAN database is a popular tool for evaluating genomics data in cancer (14). Using the UALCAN database, we evaluated the association between FUCA2 messenger RNA (mRNA) and protein levels and clinicopathological markers.
Enrichment analysis
A heat map and volcano plot were created using the pheatmap tool in R. The “ggplot2” R package (https://cran.r-project.org/web/packages/ggplot2/index.html) in R 4.1.2 was used to study Gene Ontology (GO), which comprises cellular components, molecular functions, biological processes, and Kyoto Encyclopedia of Genes and Genomes (KEGG) pathway enrichment. The Search Tool for the Retrieval of Interacting Genes (STRING) was used to construct the FUCA2 protein-protein interaction (PPI) network. The cytoHubba and molecular complex detection (MCODE) Cytoscape plugins were used to identify hub genes in PPI networks (15). Gene set enrichment analysis (GSEA) was used to determine whether changes in gene expression between 2 biological situations (high and low FUCA2 expressions) were statistically significant (16).
Tumor-infiltrating immune cell analysis
Immune infiltration [B cells, dendritic cells, T cells, macrophages, and natural killer (NK) cells] was investigated using Cell-type Identification by Estimating Relative Subsets of RNA Transcripts (CIBERSORT) (17). Only samples with P<0.05 in CIBERSORT were evaluated. Spearman correlation coefficient was used to compare immune cell types in groups with high and low levels of FUCA2. The Tumor Immune Estimation Resource (TIMER) 2.0 website was used to assess pan-cancer gene expression and immune infiltration (18). We further investigated the relationship between FUCA2 and the infiltration of CD4+ T cells, B cells, CD8+ T cells, dendritic cells, neutrophils, and macrophages. The Tumor and Immune System Interaction Database (TISIDB) was used to investigate the impact of cancer on the immune system (19).
Statistical analysis
All statistical analyses were conducted using R 4.1.2. Variables were compared between groups using either Fisher exact test or the chi-squared test. Survival was analyzed using Kaplan-Meier curves and was compared between groups using the log-rank test. Univariate and multivariate Cox regression analyses were used to investigate the effect of distinct variables on HCC patient survival. Spearman rank correlation test was used to determine the relationship between 2 variables. Statistical significance was set at a two-sided P value <0.05.
Results
FUCA2 transcript levels in patients with HCC
FUCA2 pan-cancer expression was analyzed using GEPIA2 (Figure 1A) and TIMER 2.0 (Figure 1B). Cancers of the breast, esophagus, lungs, stomach, liver, colon, and pancreas expressed FUCA2 to a greater extent than did other cancers. These results indicate that FUCA2 is prevalent in HCC. With the cutoff value of 50% (TCGA database; the cutoff value of FUCA2 expression used to divide groups was 4.791648) as the dividing threshold, the patients were divided into a high-FUCA2 expression group (n=167) and a low-FUCA2 expression group (n=168), and several clinical parameters were evaluated according to FUCA2 mRNA expression (Table 1). This analysis revealed that FUCA2 expression is associated with the age at which HCC is diagnosed.
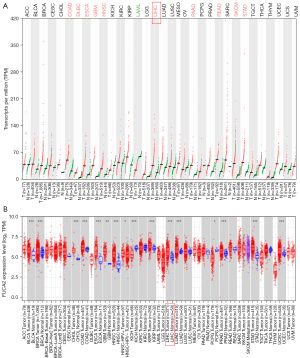
Table 1
Variables | FUCA2 expression | P value | |
---|---|---|---|
High (n=167) | Low (n=168) | ||
Sex | 0.534 | ||
Female | 57 (34.1) | 51 (30.4) | |
Male | 110 (65.9) | 117 (69.6) | |
Age (years) | 0.0149 | ||
<40 | 23 (13.8) | 9 (5.4) | |
≥40 | 144 (86.2) | 159 (94.6) | |
Stage | 0.836 | ||
I | 80 (47.9) | 87 (51.8) | |
II | 43 (25.7) | 41 (24.4) | |
III | 42 (25.1) | 37 (22.0) | |
IV | 2 (1.2) | 3 (1.8) | |
T classification | 0.766 | ||
T1 | 82 (49.1) | 87 (51.8) | |
T2 | 43 (25.7) | 43 (25.6) | |
T3 | 39 (23.4) | 33 (19.6) | |
T4 | 3 (1.8) | 5 (3.0) | |
M classification | 0.384 | ||
M0 | 133 (79.6) | 125 (74.4) | |
M1 | 1 (0.6) | 3 (1.8) | |
MX | 33 (19.8) | 40 (23.8) | |
N classification | 0.52 | ||
N0 | 124 (74.3) | 122 (72.6) | |
N1 | 3 (1.8) | 1 (0.6) | |
NX | 40 (24.0) | 45 (26.8) | |
Residual tumor | 0.523 | ||
R0 | 151 (90.4) | 151 (89.9) | |
R1 | 4 (2.4) | 7 (4.2) | |
R2 | 0 (0) | 1 (0.6) | |
RX | 12 (7.2) | 9 (5.4) | |
Overall survival | 0.058 | ||
Yes | 64 (38.3) | 47 (28.0) | |
No | 103 (61.7) | 121 (72.0) |
Unless indicated otherwise, data are presented as n (%). With the cutoff value of 50% as the dividing threshold, the patients were divided into a high-FUCA2 expression group (n=167) and a low-FUCA2 expression group (n=168). FUCA2, alpha-L-fucosidase 2.
Prognostic value of FUCA2
FUCA2 expression was found to be considerably higher in HCC than in adjacent tissues (Figure 2A,2B). As shown in Figure 2C, the median OS of patients with HCC was much longer for those with low FUCA2 expression than for those with high FUCA2 expression (P<0.001). These findings suggest that overexpression of FUCA2 protein decreases the survival rate of individuals with HCC. Certain treatments, such as antibody or short interference RNA, that reduce the amount of FUCA2 protein in the body may help patients with HCC live longer.
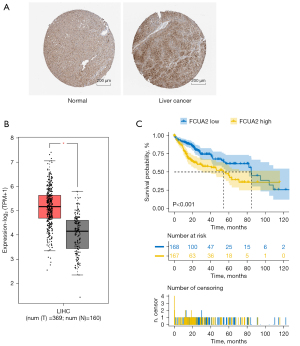
Using univariate and multivariate Cox proportional hazards regression analysis, we reviewed and assessed the clinical parameters that could be possible risk factors. According to univariate Cox regression analysis of the data, the following factors could contribute to predicting patient survival: age, sex, race, stage, FUCA2 expression, T, N, and M classification, and residual tumor. The forest plot in Figure 3A shows the hazard ratios (HRs) for the clinical characteristics, with “Coef” >0 indicating that these parameters are factors affecting survival in HCC. The overall P value was 3.9026×10–8, and the model had a concordance index (C-index) of 0.7 (Figure 3A).
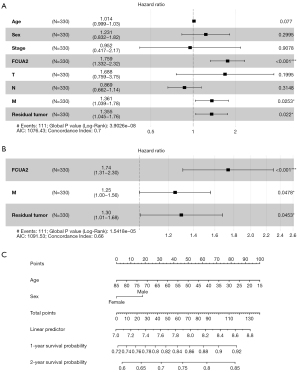
The outcomes of multivariate studies using stepwise models that included the significant risk identified in univariate analyses showed that FUCA2, M classification, and residual tumor were independent predictors of HCC survival (Figure 3B). The overall P value was 1.5418×10–5, and the model had a C-index of 0.66 (Figure 3B). In the multivariate Cox regression analysis, the HRs for FUCA2, M classification, and residual tumor were 1.74 [95% confidence interval (CI): 1.31–2.30; P<0.001], 1.25 (95% CI: 1.00–1.56; P=0.0478), and 1.30 (95% CI: 1.01–1.68; P=0.0453), respectively (Figure 3B).
We created a nomogram that uses age and sex to predict the chances of survival at 1 and 2 years for patients with HCC, with each component allocated proportional points according to its influence on survival (Figure 3C).
Correlations between FUCA2 expression and clinical features
Using the UALCAN database, the relationships between FUCA2 expression and clinical characteristics in patients with HCC were investigated. First, FUCA2 expression was higher in HCC than in normal tissues (Figure 4A; P=1.62×10–12). In addition, compared with normal tissues, FUCA2 expression was higher in HCC stages 1, 2, and 3 (P=1.62×10–12, P=2.24×10–10, and P=2.75×10–11, respectively; Figure 4B). FUCA2 expression in HCC stage 4 did not differ significantly from that in other HCC stages or normal tissues (Figure 4B). FUCA2 expression was higher in the Caucasian, African American, and Asian groups than in the normal group (P=1.62×10–12, P=3.77×10–4, and P<1×10–12, respectively; Figure 4C). There was no significant difference in FUCA2 expression in HCC samples between males and females (P>0.05; Figure 4D). Compared with normal tissues, FUCA2 expression was significantly higher in samples from patients with HCC aged 21–40, 41–60, and 61–80 years (P=1.79×10–4, P=1.62×10–12, and P=1.62×10–12), but not in those aged 81–100 years (Figure 4E). In addition, compared with normal tissues, FUCA2 expression was significantly higher in all weight categories (normal, extreme, obese, and extremely obese (P<1×10–12, P=1.16×10–8, P=1.06×10–7, and P=3.12×10–2, respectively; Figure 4F) and all tumor grades (1, 2, 3, and 4; P=6.04×10–5, P=3.33×10–15, P<1×10–12, P=4.93×10–2, respectively; Figure 4G). FUCA2 expression did not differ significantly between the N0 and N1 group (P>0.05; Figure 4H). FUCA2 expression was significantly higher in both the tumor protein p53 (TP53) mutant and nonmutant groups than in the normal group (both P<1×10–12) and higher in the TP53 mutant group than in the nonmutant group (P=4.91×10–8; Figure 4I). Finally, FUCA2 expression was significantly higher in samples of HCC, fibrolamellar carcinoma, and hepatocholangiocarcinoma (mixed) than in normal tissues (P<1×10–12, P=2.22×10–2, P=1.42×10–7; Figure 4J).
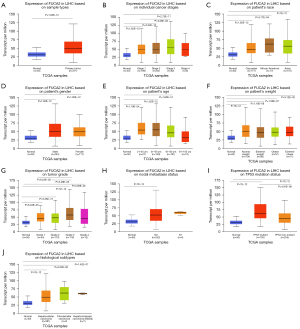
Analysis of the enrichment of FUCA2-related pathways
To explore the potential mechanism by which FUCA2 causes tumor progression, we analyzed differentially expressed genes (DEGs) between the high- and low-FUCA2 expression groups using a heat map and volcano plots (Figure 5A,5B). A clustering heat map was constructed of genes whose expression levels varied across samples, with the horizontal and vertical axes, respectively, reflecting sample and protein clustering (Figure 5A). The heat map was separated into 2 categories of tumor tissue and adjacent normal tissue, with red indicating the upregulation of gene expression and blue indicating the downregulation. The volcano plots provide an integral overview of differentially expressed genes (Figure 5B).
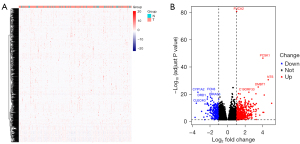
The DEGs identified were analyzed for enrichment of GO terms and KEGG pathways. The following biological processes were markedly affected by the level of FUCA2 expression: signal release, response to xenobiotic stimulus, and organic anion transport (Figure 6A). The most enriched cellular component terms were collagen-containing extracellular matrix, apical part of cell, and apical plasma membrane (Figure 6B). In terms of molecular function, signaling receptor activator activity, receptor ligand activity, and serine hydrolase activity were the most enriched phrases (Figure 6C). For KEGG terms, neuroactive ligand–receptor interaction, cyclic adenosine monophosphate (cAMP) signal pathway, and chemical carcinogenesis-receptor activation were the most enriched pathways (Figure 6D).
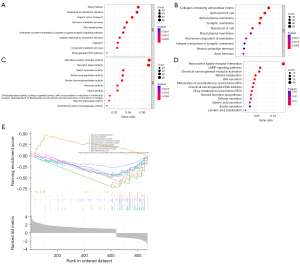
According to the median value of FUCA2 expression, data were separated into high and low expression sets, and signaling pathways were evaluated using GSEA. Enriched signaling pathways were chosen on the basis of the normalized enrichment score, the false discovery rate (FDR) Q value, and nominal P value (Figure 6E). There were 10 enriched and cancer-related functions: fatty acid metabolic process, gamete generation process, icosanoid metabolic process, long chain fatty acid metabolic process, monocarboxylic acid metabolic process, organic acid metabolic process, small molecule biosynthetic process, molecule metabolic process, oxidoreductase activity, and oxidoreductase activity acting on paired donors with incorporation or reduction of molecular oxygen (Figure 6E).
Protein interaction network
To further study the interactions between the chosen DEGs, we submitted them to the STRING database. There were 71 nodes and 450 edges in the network (Figure 7A). Based on the Cytoscape cytoHubba plug-in, the 10 most significant hub genes were determined to be galactosidase beta 1 (GLB1), hexokinase 1 (HK1), hexokinase 2 (HK2), hexokinase 3 (HK3), glucokinase (GCK), lactase (LCT), aldo-keto reductase family 1 member B (AKR1B1), glucose-6-phosphatase catalytic subunit (G6PC), hexokinase domain containing 1 (HKDC1), and glucose-6-phosphatase catalytic subunit 2 (G6PC2) (Figure 7B). Figure 7C-7F shows the Cytoscape plugin MCODE module analysis of the PPI networks. HKDC1, LCT, HK3, G6PC, GLB1, G6PC2, GCK, aldo-keto reductase family 1 member B10 (AKR1B10), maltase-glucoamylase (MGAM), galactose mutarotase (GALM), HK2, AKR1B1, glucose-6-phosphatase catalytic subunit 3 (G6PC3), HK1, and sucrase-isomaltase (SI) were the hub nodes with the highest score (13.875) in module 1 (15 nodes, 97 edges; Figure 7C), followed by module 2 (8 nodes, 18 edges, score 5.143), module 3 (14 nodes, 32 edges, score 4.923), and module 4 (11 nodes, 20 edges, score 4; Figure 7D-7F).
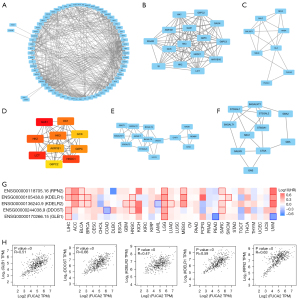
Immune infiltration and correlations with FUCA2 expression
The heat maps also revealed positive associations between FUCA2 and the top 5 genes in every kind of cancer (Figure 7G). The top 100 FUCA2-associated genes were identified with the GEPIA2 database. The associated heat map demonstrated a favorable connection between FUCA2 and the top 5 genes across a number of cancer types (Figure 7G). In addition, FUCA2 interacted with GLB1, dolichyl-diphosphooligosaccharide--protein glycosyltransferase noncatalytic subunit (DDOST), KDEL endoplasmic reticulum protein retention receptor 2 (KDELR2), KDEL endoplasmic reticulum protein retention receptor 1 (KDELR1), and ribophorin II (RPN2) in this mode (Figure 7H).
Using CIBERSORT, we next assessed the fractions of tumor-infiltrating immune cells to confirm the link between FUCA2 expression and the immunological tumor microenvironment. The percentage of each of the 22 types of immune cells in HCC tissues was determined (Figure 8A). The connection between immune infiltration and FUCA2 expression was computed using TIMER 2.0. As shown in Figure 8B, FUCA2 expression was correlated with CD4+ T cells, B cells, CD8+ T cells, dendritic cells, neutrophils, and macrophage infiltration. The TISIDB database analyzed the connection between FUCA2 and 28 tumor-infiltrating lymphocytes (TILs), revealing that FUCA2 was associated with TILs in most malignancies (Figure 9A). Figure 9B shows the correlation between FUCA2 expression and 15 of the 28 TILs in HCC.
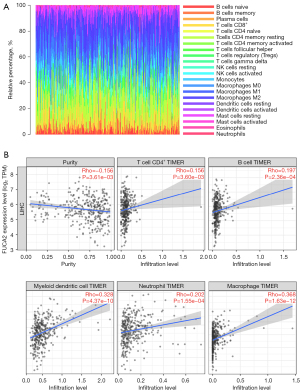
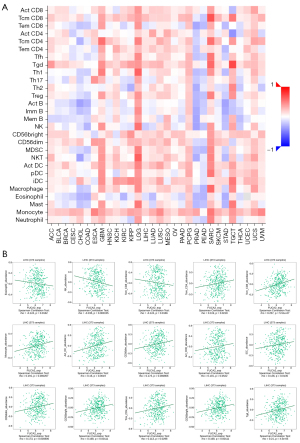
Discussion
HCC is one of the most difficult-to-treat and fatal cancers (20). Identifying potential biomarkers may contribute to precise prognostic evaluation and guide systemic therapy in patients with HCC. Various studies have sought prognostic biomarkers (21,22). According to previous studies, esophageal squamous cell carcinoma and gastric cancer are among the malignancies in which FUCA2 mRNA is expressed at elevated levels (10,23). In accordance with these results, we observed higher FUCA2 mRNA expression in HCC. Because of this, it is now possible that FUCA2 is a target molecule in HCC. Furthermore, through analyzing FUCA2 mRNA expression according to different clinical characteristics, it was found that FUCA2 expression (TCGA database) is associated with the age at which the patients were diagnosed with HCC. Moreover, higher FUCA2 mRNA expression predicted a poor prognosis and was an independent factor influencing OS.
The poor prognosis of HCC is a concern globally, and the recurrence and spread of the tumor are significant prognostic variables. There is a strong relationship between cancer stem cells (CSCs) and both tumor recurrence and metastasis. CSCs can both self-replicate to create extra stem cells and differentiate into cancerous cells that are distinct from themselves (24). These CSCs that are able to withstand therapy will initiate the process of tumor growth (25). It is essential to have complete knowledge of human malignancies to identify particular targets or characteristics for more accurate and individualized therapy (26).
The heat map in Figure 7D shows the coexpression and correlation of the 5 genes most positively associated with FUCA2: GLB1, DDOST, KDELR2, KDELR1, and RPN2. In the TIMER 2.0 database, these genes had a positive connection with FUCA2 across most cancer types. Overall, the FUCA2 gene and the GLB1, DDOST, KDELR2, KDELR1, and RPN2 genes may serve as indicators for the prognosis of HCC. According to the PPI networks, GLB1, HK1, GCK, G6PC, HKDC1, G6PC2, LCT, HK2, HK3, and AKR1B1 are the top 10 hub genes associated with the expression of FUCA2 in HCC (Figure 7B). According to the findings of the GO analysis, most of these genes are involved in signal release and the creation of collagen-containing extracellular matrix. KEGG analysis determined that the functional activities of FUCA2 include the interaction of neuroactive ligands and receptors, as well as the cAMP signaling pathway.
Previous studies have established that disruption of the tumor immune microenvironment is a leading driver of cancer development (27). Immune cells that infiltrate the tumor affect the microenvironment and behavior of the tumor. By changing immune cell proportions, FUCA2 may affect the tumor microenvironment, hence promoting tumor growth and spread. Our study demonstrated a connection between macrophages and FUCA2 expression. Tumor-associated macrophages have several roles in cancer etiology (28). The control of the tumor microenvironment is complex, and CD4+ T cells, B cells, CD8+ T cells, dendritic cells, neutrophils, and macrophages may influence the survival of tumor cells. Future studies are needed to determine how FUCA2 expression affects these cells.
Finally, we identified FUCA2-binding proteins and associated genes in cancer. We found that FUCA2 is overexpressed in HCC, suggesting that FUCA2 can predict and be used to evaluate the prognosis of HCC patients independently. The results of this study show that FUCA2 interacts with invading immune cells and linked genes in HCC, which contributes to its association with a poor prognosis in patients with HCC. However, this study focused on clinical relevance and did not investigate the molecular process. Future research will be undertaken in vivo and in vitro to investigate the FUCA2-related pathogenic mechanism of HCC. The findings suggest that FUCA2 may be a potential target of cancer treatment.
Conclusions
In summary, FUCA2 may exert a vital regulatory part in tumor immune cell infiltration, which is also a significant prognostic biomarker for hepatocellular carcinoma, and may become a promising novel and therapeutic target in hepatocellular carcinoma. Besides, our study requires more exploratory research in bioinformatics, and more basic research is needed to verify these outcomes in the future.
Acknowledgments
We would like to express our gratitude to the UCSC, TCGA, and TIMER databases for their contributions to the Cancer Genomics project.
Funding: This research was funded by the National Natural Science Foundation of China (No. 81773444).
Footnote
Reporting Checklist: The authors have completed the TRIPOD reporting checklist. Available at https://tcr.amegroups.com/article/view/10.21037/tcr-22-1850/rc
Conflicts of Interest: All authors have completed the ICMJE uniform disclosure form (available at https://tcr.amegroups.com/article/view/10.21037/tcr-22-1850/coif). The authors have no conflicts of interest to declare.
Ethical Statement: The authors are accountable for all aspects of the work in ensuring that questions related to the accuracy or integrity of any part of the work are appropriately investigated and resolved. The study was conducted in accordance with the Declaration of Helsinki (as revised in 2013). The study was approved by the Institutional Ethics Board of Renmin Hospital of Wuhan University (No. WDRY2020-K223), and individual consent for this retrospective analysis was waived.
Open Access Statement: This is an Open Access article distributed in accordance with the Creative Commons Attribution-NonCommercial-NoDerivs 4.0 International License (CC BY-NC-ND 4.0), which permits the non-commercial replication and distribution of the article with the strict proviso that no changes or edits are made and the original work is properly cited (including links to both the formal publication through the relevant DOI and the license). See: https://creativecommons.org/licenses/by-nc-nd/4.0/.
References
- Siegel RL, Miller KD, Fuchs HE, et al. Cancer statistics, 2022. CA Cancer J Clin 2022;72:7-33. [Crossref] [PubMed]
- Xia C, Dong X, Li H, et al. Cancer statistics in China and United States, 2022: profiles, trends, and determinants. Chin Med J (Engl) 2022;135:584-90. [Crossref] [PubMed]
- Shuai Y, Fan E, Zhong Q, et al. CDCA8 as an independent predictor for a poor prognosis in liver cancer. Cancer Cell Int 2021;21:159. [Crossref] [PubMed]
- Arvanitakis K, Koletsa T, Mitroulis I, et al. Tumor-Associated Macrophages in Hepatocellular Carcinoma Pathogenesis, Prognosis and Therapy. Cancers (Basel) 2022;14:226. [Crossref] [PubMed]
- Foerster F, Gairing SJ, Ilyas SI, et al. Emerging immunotherapy for HCC: A guide for hepatologists. Hepatology 2022;75:1604-26. [Crossref] [PubMed]
- Fridman WH, Zitvogel L, Sautès-Fridman C, et al. The immune contexture in cancer prognosis and treatment. Nat Rev Clin Oncol 2017;14:717-34. [Crossref] [PubMed]
- Mai J, Hua X, Bian ZH, et al. Identification of prognostic immune cells and potential immune-related markers in hepatocellular carcinoma. Transl Cancer Res 2022;11:2262-74. [Crossref] [PubMed]
- Abdel Ghafar MT, Morad MA, El-Zamarany EA, et al. Autologous dendritic cells pulsed with lysate from an allogeneic hepatic cancer cell line as a treatment for patients with advanced hepatocellular carcinoma: A pilot study. Int Immunopharmacol 2020; Epub ahead of print. [Crossref] [PubMed]
- Grootaert H, Van Landuyt L, Hulpiau P, et al. Functional exploration of the GH29 fucosidase family. Glycobiology 2020;30:735-45. [Crossref] [PubMed]
- Liu TW, Ho CW, Huang HH, et al. Role for alpha-L-fucosidase in the control of Helicobacter pylori-infected gastric cancer cells. Proc Natl Acad Sci U S A 2009;106:14581-6. [Crossref] [PubMed]
- Waidely E, Al-Youbi AO, Bashammakh AS, et al. Alpha-l-Fucosidase Immunoassay for Early Detection of Hepatocellular Carcinoma. Anal Chem 2017;89:9459-66. [Crossref] [PubMed]
- Liu S, Wang W, Zhao Y, et al. Identification of Potential Key Genes for Pathogenesis and Prognosis in Prostate Cancer by Integrated Analysis of Gene Expression Profiles and the Cancer Genome Atlas. Front Oncol 2020;10:809. [Crossref] [PubMed]
- Uhlen M, Zhang C, Lee S, et al. A pathology atlas of the human cancer transcriptome. Science 2017;357:eaan2507. [Crossref] [PubMed]
- Chandrashekar DS, Bashel B, Balasubramanya SAH, et al. UALCAN: A Portal for Facilitating Tumor Subgroup Gene Expression and Survival Analyses. Neoplasia 2017;19:649-58. [Crossref] [PubMed]
- Bader GD, Hogue CW. An automated method for finding molecular complexes in large protein interaction networks. BMC Bioinformatics 2003;4:2. [Crossref] [PubMed]
- Subramanian A, Tamayo P, Mootha VK, et al. Gene set enrichment analysis: a knowledge-based approach for interpreting genome-wide expression profiles. Proc Natl Acad Sci U S A 2005;102:15545-50. [Crossref] [PubMed]
- Newman AM, Liu CL, Green MR, et al. Robust enumeration of cell subsets from tissue expression profiles. Nat Methods 2015;12:453-7. [Crossref] [PubMed]
- Li T, Fu J, Zeng Z, et al. TIMER2.0 for analysis of tumor-infiltrating immune cells. Nucleic Acids Res 2020;48:W509-14. [Crossref] [PubMed]
- Ru B, Wong CN, Tong Y, et al. TISIDB: an integrated repository portal for tumor-immune system interactions. Bioinformatics 2019;35:4200-2. [Crossref] [PubMed]
- Zhang Y, Zhang X, Kuang M, et al. Emerging Insights on Immunotherapy in Liver Cancer. Antioxid Redox Signal 2022;37:1325-38. [Crossref] [PubMed]
- Fang C, Liu S, Feng K, et al. Ferroptosis-related lncRNA signature predicts the prognosis and immune microenvironment of hepatocellular carcinoma. Sci Rep 2022;12:6642. [Crossref] [PubMed]
- Li YR, Chen JD, Zhu YY, et al. Evaluation of nuclear PGAM2 value in hepatocellular carcinoma prognosis. Anticancer Drugs 2022;33:e500-6. [Crossref] [PubMed]
- Yu X, Zhang R, Yang T, et al. Alpha-l-fucosidase: a novel serum biomarker to predict prognosis in early stage esophageal squamous cell carcinoma. J Thorac Dis 2019;11:3980-90. [Crossref] [PubMed]
- Yang F, Cui P, Lu Y, et al. Requirement of the transcription factor YB-1 for maintaining the stemness of cancer stem cells and reverting differentiated cancer cells into cancer stem cells. Stem Cell Res Ther 2019;10:233. [Crossref] [PubMed]
- Lee TK, Guan XY, Ma S. Cancer stem cells in hepatocellular carcinoma - from origin to clinical implications. Nat Rev Gastroenterol Hepatol 2022;19:26-44. [Crossref] [PubMed]
- Andre F, Mardis E, Salm M, et al. Prioritizing targets for precision cancer medicine. Ann Oncol 2014;25:2295-303. [Crossref] [PubMed]
- Hao X, Sun G, Zhang Y, et al. Targeting Immune Cells in the Tumor Microenvironment of HCC: New Opportunities and Challenges. Front Cell Dev Biol 2021;9:775462. [Crossref] [PubMed]
- Gibson EM, Nagaraja S, Ocampo A, et al. Methotrexate Chemotherapy Induces Persistent Tri-glial Dysregulation that Underlies Chemotherapy-Related Cognitive Impairment. Cell 2019;176:43-55.e13. [Crossref] [PubMed]
(English Language Editors: N. Korszniak and J. Gray)