Construction of a predictive model for breast cancer metastasis based on lncRNAs
Highlight box
Key findings
• Zinc finger protein 582-antisense RNA 1 (ZNF582-AS1), metastasis-associated lung adenocarcinoma transcript 1 (MALAT1), and actin filament associated protein 1-antisense RNA 1 (AFAP1-AS1) were the key lncRNA in lymph node metastasis of breast cancer. The risk score model based on ZNF582-AS1, MALAT1 and AFAP1-AS1 can accurately predict the risk of breast cancer lymph node metastasis.
What is known and what is new?
• At present, there is a lack of biological markers to determine the risk of lymph node metastasis in breast cancer. A single lncRNA cannot accurately explain the heterogeneity of tumors.
• A weighted correlation network analysis and logistic regression analysis were conducted to screen the hub lncRNAs associated with lymph node metastasis traits.
What is the implication, and what should change now?
• Our analysis suggests that ZNF582-AS1, MALAT1, and AFAP1-AS1 may be involved in the regulation of the breast cancer immune microenvironment.
Introduction
Breast cancer is a malignant tumor, is the most common cancer in women worldwide, and is known for its high mortality, recurrence, and metastasis rates (1). With the popularization of breast cancer screening, some types of breast cancer can be diagnosed and treated at an early stage. However, some breast cancer patients still have lymph node metastasis at the time of first diagnosis (2,3). In these patients, the cancer cells may have spread to other parts of the body and into the lymphatic system. In such patients, both the primary tumor and metastatic tumor require treatment (4). Thus, patients with lymph node metastasis need complex treatment strategies and face a higher risk of recurrence and death. The cancer cells of breast cancer generally metastasize along lymphatic vessels. The first station of lymph node metastasis in breast cancer is supraclavicular lymph nodes. Breast cancer patients with lymph node metastasis are generally considered to be in the middle and late stages of the disease, indicating a poor prognosis. The mechanisms of lymph node metastasis of cancer cells are diverse. The infiltration of immune cells in most cases indicates a good prognosis, but a study has pointed out that macrophages and cancer cells form chimeras to assist cancer cells to achieve lymph node metastasis (5). In terms of treatment, patients with tumor metastasis are more likely to develop drug resistance. At present, there are no clinical biological markers for determining the risk of breast cancer lymph node metastasis.
Long non-coding RNA (lncRNA) refers to RNA molecules that encode proteins. The abnormal expression of lncRNA is involved in the occurrence and development of breast cancer, and is closely related to the subtype and prognosis of breast cancer patients. For example, the overexpression of lncRNA HOX transcript antisense RNA can activate the estrogen receptor signaling pathway and lead to tamoxifen drug resistance in patients (6). The high expression of lncRNA regulators of reprogramming (ROR) can promote the process of epithelial-mesenchymal transition and induce the invasion and metastasis of breast cancer cells (7). High expression of lncRNA MAPT-AS1 promotes the proliferation of breast cancer cells (8). The expression level of lncRNA is highly heterogeneous among breast cancer patients. At present, most studies have only examined the effect of a single lncRNA on the biological behavior of breast cancer. However, a single lncRNA cannot accurately describe the heterogeneity of tumors. Thus, more precise algorithms need to be developed to screen key pathogenic lncRNAs, and quantitative models need to be developed to describe the heterogeneity of breast cancer. One research has built models based on the expression of lncRNAs to predict the prognosis of breast cancer patients, but there are few models to predict the metastasis of breast cancer (9). In this study, a weighted correlation network analysis (WGCNA) was conducted to screen the hub lncRNAs related to the lymph node metastasis traits, and a logistic regression analysis was conducted to construct a risk score model to show the correlation between the risk score and immune cell subtype infiltration content. We present the following article in accordance with the TRIPOD reporting checklist (available at https://tcr.amegroups.com/article/view/10.21037/tcr-23-129/rc).
Methods
Sample collection and download
The RNA-sequencing data and associated clinical information of patients with breast cancer (n=1,091) were downloaded from The Cancer Genome Atlas (TCGA) database (https://portal.gdc.cancer.gov/). There data set comprised 561 breast cancer tissue samples with lymph node metastasis, 516 breast cancer tissue samples with non-lymph node metastasis, and 14 samples with missing information. The data set was logarithmically transformed using the following transformation formula: relative gene expression=log2 [Fragments Per Kilobase of exon model per Million mapped fragments (FPKM)+1]. The study was conducted in accordance with the Declaration of Helsinki (as revised in 2013).
Screening of key genes
The WGCNA was conducted using R software (Lucent Technologies, USA) and the WGCNA package. Based on this method, the co-expressed genes of metastatic breast cancer and non-metastatic breast cancer were screened. To obtain scale-free network, data are clustered to detect outliers and appropriate soft thresholds are set. Gene modules were identified by hierarchical clustering tree and detected by hierarchical clustering based on topological overlap matrix. The correlation coefficient was calculated to determine the correlation between each module and clinical features. The shear height is set to 0.25 and the minimum number of module genes is set to 30. Gene modules with a |correlation| >0.5 and a P value <0.01 were retained. The module significance (MS) and the average gene significance (GS) within each module was calculated. The lncRNAs were considered key if they had a MS value >0.8 and a GS value >0.5.
Calculation of the infiltration content of the immune cell subtypes
The infiltration content of the immune cell subtypes in the breast cancer tissue samples was calculated using a single sample gene set enrichment analysis.
Evaluation of diagnostic efficacy
The diagnostic efficacy of the key lncRNAs for metastatic breast cancer was evaluated using receiver operating characteristic (ROC) curves. The larger the area under the curve (AUC), the better the performance of the risk model.
Statistical analysis
R software (V3.5.1, Lucent Technologies, USA) was used for the statistical analysis. Differences between groups were compared, and a correlation analysis was performed; a two-sided P value <0.05 indicated statistical significance.
Results
Acquisition of differentially expressed lncRNAs
The differentially expressed lncRNAs between the breast cancer samples with lymph node metastasis and breast cancer samples without lymph node metastasis were compared using the Wilcoxon test (|log2 fold change| >1, false discovery rate <0.05). In total, 36 lncRNAs were identified, of which 10 were downregulated and 26 were upregulated (Figure 1).
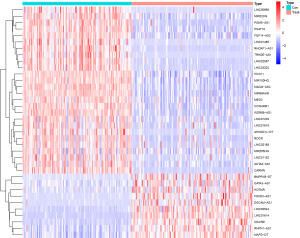
WGCNA
Each module contained at least 30 lncRNAs and the height was set at 0.25. We finally obtained 10 modules (Figure 2). The tan module (r=0.67, P=3e−23) and the magenta module (r=0.53, P=7e−14) were positively associated with non-metastatic breast cancer. The pink module was positively correlated with breast cancer lymph node metastasis traits (r=0.54, P=5e−14; Figure 3). The brown, magenta, and pink modules contained 9, 14, and 9 core lncRNAs (hub lncRNAs), respectively (Figures 4-6).
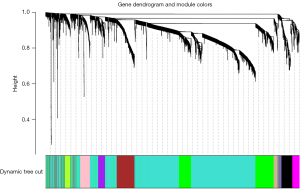
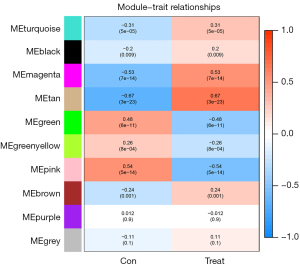
Building a predictive model
The differentially expressed lncRNA set and the hub lncRNA set had 3 common lncRNAs; that is, ZNF582-AS1, MALAT1, and AFAP1-AS1 (Figure 7). ZNF582-ASI was highly expressed in the non-metastatic samples, while MALAT1 and AFAP1-AS1 were lowly expressed in the metastatic breast cancer samples (Figures 8-10). A risk score formula was developed based on the logistic regression analysis. The following formula was used to calculate the risk score: risk score =1.31 + 0.51 * ZNF582-AS1 – 0.66 * MALAT1 – 0.50 * AFAP1-AS1.
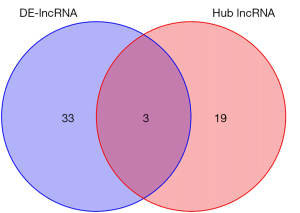
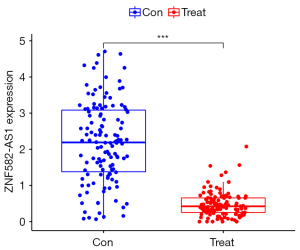

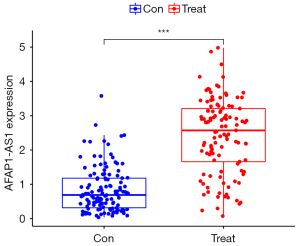
Risk score and diagnostic efficacy evaluation of the key lncRNAs
The ROC curves (Figures 11-14) showed that the AUCs of the risk score, ZNF582-AS1, MALAT1, and AFAP1-AS1 were 0.975, 0.793, 0.685, and 0.764, respectively (P<0.05).
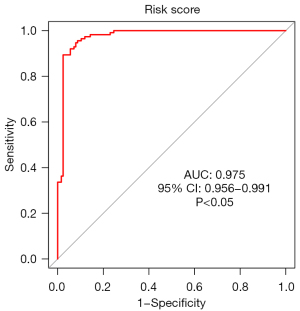
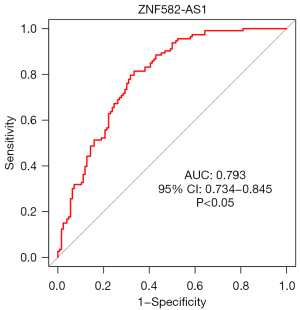
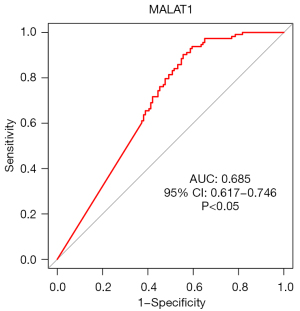
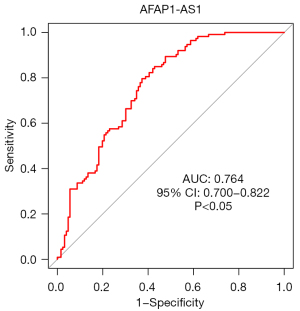
Correlation between the risk score and immune cell subtypes
In the breast cancer metastatic and non-metastatic tissue samples, there were statistical differences in the infiltration content of various immune cell subtypes (P<0.05; Figure 15). The risk score was positively correlated with the infiltration content of the immune cell subtypes (P<0.05; Figure 16).
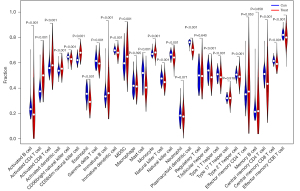
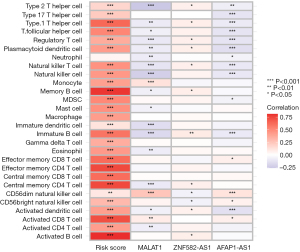
Discussion
New molecular targets are of great significance in improving the clinical treatment strategies for and the outcomes of patients with tumors. There is emerging evidence that lncRNAs are involved in the biological regulation of tumors (10-12), and some lncRNAs have been reported to be extremely valuable tumor prognostic markers (13-15). Whole-genome sequencing has revealed that most of the human genome is transcribed; however, only <2% of the genomes may encode proteins (16,17). Non-coding RNAs account for >90% of the entire human gene transcripts, participate in almost all epigenetic regulation, and play an important role in the complex life activities of advanced eukaryotes (16,17). Abnormal expressions of lncRNAs are involved in the process, metastasis, and prognosis of breast cancer (18-20). LncRNAs may play a role in promoting or inhibiting tumorigenesis and progression. This regulatory mechanism is relatively complex and diverse. The lncRNA Nkila can act on T cells, promoting immune escape (18). LncRNA DANCR promotes breast cancer cell proliferation and metastasis by upregulating VAPB (19). LncRNA152 acts as an angiogenesis inhibitor in triple-negative breast cancer that impedes cancer cell metastasis (20).
In the present study, we identified 3 key lncRNAs associated with breast cancer lymph node metastasis; that is, ZNF582-AS1, MALAT1, and AFAP1-AS1. MALAT1 is considered a potential oncogene. it’s the transcription product of MALAT1 can regulate RNA alternative splicing by combining multiple splicing factors, can affect the localization of various proteins in the nucleus through scaffolding, and can also affect the signaling pathways, chromosomal rearrangement, histone modification, and small RNA construction, and other important physiological processes (21). Additionally, MALAT1 can regulate biological behaviors, such as tumor cell proliferation, invasion and metastasis, and affect the occurrence and development of tumors (22). MALAT1 is closely related to the formation of breast cancer and various biological behaviors. MALAT1 is downregulated in breast cancer cells and tissues and promotes the epithelial-mesenchymal differentiation and metastasis of breast cancer cells (23-25). Meseure et al. (26) showed that MALAT1 was upregulated in 14.13% of breast cancer patients. Jadaliha et al. (27) showed that MALAT1 was differentially upregulated in triple-negative breast cancer. Our research suggested that MALAT1 was related to the lymph node metastasis of breast cancer, but its mechanism still requires further exploration.
One study pointed out that the abnormal expression of AFAP1-AS1 is closely related to the clinical stage and lymph node metastasis of breast cancer (28). AFAP1-AS1 may be involved in the occurrence and development of breast cancer, and thus may have potential as a marker for the clinical diagnosis and prognosis of breast cancer (28). Dianatpour et al. (29) found that the expression of AFAP1-AS1 was upregulated in various cancers, including breast cancer. AFAP1-AS1 has also been reported to be more upregulated in breast cancer samples than adjacent tissues, and thus may be related to the onset and progression of breast cancer (29). AFAP1-AS1 has also been shown to be significantly downregulated in Ki-67-negative tumor samples (29). However, this study (29) did not observe any correlation between AFAP1-AS1 and clinical features. This differs to the results of the current study; however, this difference in results may be related to the small sample size of the study (29). Zhang et al. (30) concluded that AFAP1-AS1 promotes the proliferation and metastasis of cancer cells through microRNA (miR)-145 in triple-negative breast cancer.
Our study found that ZNF582-AS1 was highly expressed in the non-lymph node metastatic samples and was positively correlated with the infiltration content of various immune cell subtypes. This is consistent with the findings of previous research. Notably, Wang et al. (31) showed that the expression of ZNF582-AS1 was downregulated in breast cancer tissues. Patients with high expressions of ZNF582-AS1 have a good prognosis and a low recurrence rate. The study (31) also indicated ZNF582-AS1 is a protective factor and indicates a good prognosis in breast cancer patients.
We developed a risk score formula for breast cancer lymph node metastasis based on ZNF582-AS1, MALAT1, and AFAP1-AS1. The ROC curves showed that the model had good predictive performance. We also found that the risk score was positively correlated with the infiltration content of multiple immune cell subtypes. According to our analysis, ZNF582-AS1, MALAT1, and AFAP1-AS1 may be involved in the regulation of the breast cancer immune microenvironment.
There are some limitations in this study. First, the accuracy of the model was not verified by external data. Second, there is a lack of in vivo and in vitro experiments to validate the results of our analysis without further insight into the specific roles and functions of key lncRNAs in breast cancer.
In summary, ZNF582-AS1, MALAT1, and AFAP1-AS1 are key lncRNAs for breast cancer lymph node metastasis and are potential biomarkers.
Conclusions
ZNF582-AS1, MALAT1 and AFAP1-AS1 are key lncRNAs for lymph node metastasis of breast cancer. The risk scoring model based on ZNF582-AS1, MALAT1 and AFAP1-AS1 can accurately predict the risk of lymph node metastasis of breast cancer. ZNF582-AS1, MALAT1 and AFAP1-AS1 are potential biomarkers of lymph node metastasis of breast cancer.
Acknowledgments
Funding: This study was supported by the 2019 Jiaxing Key Medical Discipline Clinical Laboratory Diagnostics (Innovative Discipline) Program (No. 2019-cx-03) and the Jiaxing Science and Technology Plan Project (No. 2021AD30142).
Footnote
Reporting Checklist: The authors have completed the TRIPOD reporting checklist. Available at https://tcr.amegroups.com/article/view/10.21037/tcr-23-129/rc
Conflicts of Interest: Both authors have completed the ICMJE uniform disclosure form (available at https://tcr.amegroups.com/article/view/10.21037/tcr-23-129/coif). The authors have no conflicts of interest to declare.
Ethical Statement: The authors are accountable for all aspects of the work in ensuring that questions related to the accuracy or integrity of any part of the work are appropriately investigated and resolved. The study was conducted in accordance with the Declaration of Helsinki (as revised in 2013).
Open Access Statement: This is an Open Access article distributed in accordance with the Creative Commons Attribution-NonCommercial-NoDerivs 4.0 International License (CC BY-NC-ND 4.0), which permits the non-commercial replication and distribution of the article with the strict proviso that no changes or edits are made and the original work is properly cited (including links to both the formal publication through the relevant DOI and the license). See: https://creativecommons.org/licenses/by-nc-nd/4.0/.
References
- Loibl S, Poortmans P, Morrow M, et al. Breast cancer. Lancet 2021;397:1750-69. [Crossref] [PubMed]
- Kim MY. Breast Cancer Metastasis. Adv Exp Med Biol 2021;1187:183-204. [Crossref] [PubMed]
- Waks AG, Winer EP. Breast Cancer Treatment. JAMA 2019;321:316. [Crossref] [PubMed]
- Peart O. Metastatic Breast Cancer. Radiol Technol 2017;88:519M-39M. [PubMed]
- Gast CE, Silk AD, Zarour L, et al. Cell fusion potentiates tumor heterogeneity and reveals circulating hybrid cells that correlate with stage and survival. Sci Adv 2018;4:eaat7828. [Crossref] [PubMed]
- Wu D, Zhu J, Fu Y, et al. LncRNA HOTAIR promotes breast cancer progression through regulating the miR-129-5p/FZD7 axis. Cancer Biomark 2021;30:203-12. [Crossref] [PubMed]
- Ma J, Yang Y, Huo D, et al. LincRNA-RoR/miR-145 promote invasion and metastasis in triple-negative breast cancer via targeting MUC1. Biochem Biophys Res Commun 2018;500:614-20. [Crossref] [PubMed]
- Xu M, Chen Z, Lin B, et al. A seven-lncRNA signature for predicting prognosis in breast carcinoma. Transl Cancer Res 2021;10:4033-46. [Crossref] [PubMed]
- Li B, Liu D, Xu J, et al. Overexpression of lncRNA MAPT-AS1 exacerbates cell proliferation and metastasis in breast cancer. Transl Cancer Res 2022;11:835-47. [Crossref] [PubMed]
- Tan YT, Lin JF, Li T, et al. LncRNA-mediated posttranslational modifications and reprogramming of energy metabolism in cancer. Cancer Commun (Lond) 2021;41:109-20. [Crossref] [PubMed]
- Li G, Kryczek I, Nam J, et al. LIMIT is an immunogenic lncRNA in cancer immunity and immunotherapy. Nat Cell Biol 2021;23:526-37. [Crossref] [PubMed]
- Peng WX, Koirala P, Mo YY. LncRNA-mediated regulation of cell signaling in cancer. Oncogene 2017;36:5661-7. [Crossref] [PubMed]
- Wang J, Su Z, Lu S, et al. LncRNA HOXA-AS2 and its molecular mechanisms in human cancer. Clin Chim Acta 2018;485:229-33. [Crossref] [PubMed]
- Wang Y, Lu JH, Wu QN, et al. LncRNA LINRIS stabilizes IGF2BP2 and promotes the aerobic glycolysis in colorectal cancer. Mol Cancer 2019;18:174. [Crossref] [PubMed]
- Liang Y, Song X, Li Y, et al. LncRNA BCRT1 promotes breast cancer progression by targeting miR-1303/PTBP3 axis. Mol Cancer 2020;19:85. [Crossref] [PubMed]
- Ferrè F, Colantoni A, Helmer-Citterich M. Revealing protein-lncRNA interaction. Brief Bioinform 2016;17:106-16. [Crossref] [PubMed]
- Zhu J, Fu H, Wu Y, et al. Function of lncRNAs and approaches to lncRNA-protein interactions. Sci China Life Sci 2013;56:876-85. [Crossref] [PubMed]
- Huang D, Chen J, Yang L, et al. NKILA lncRNA promotes tumor immune evasion by sensitizing T cells to activation-induced cell death. Nat Immunol 2018;19:1112-25. [Crossref] [PubMed]
- Jia H, Liang K, Liu G, et al. lncRNA DANCR Promotes Proliferation and Metastasis of Breast Cancer Cells Through Sponging miR-4319 and Upregulating VAPB. Cancer Biother Radiopharm 2022;37:650-61. [Crossref] [PubMed]
- Kim DS, Camacho CV, Setlem R, et al. Functional Characterization of lncRNA152 as an Angiogenesis-Inhibiting Tumor Suppressor in Triple-Negative Breast Cancers. Mol Cancer Res 2022;20:1623-35. [Crossref] [PubMed]
- Li ZX, Zhu QN, Zhang HB, et al. MALAT1: a potential biomarker in cancer. Cancer Manag Res 2018;10:6757-68. [Crossref] [PubMed]
- Goyal B, Yadav SRM, Awasthee N, et al. Diagnostic, prognostic, and therapeutic significance of long non-coding RNA MALAT1 in cancer. Biochim Biophys Acta Rev Cancer 2021;1875:188502. [Crossref] [PubMed]
- Yue X, Wu WY, Dong M, et al. LncRNA MALAT1 promotes breast cancer progression and doxorubicin resistance via regulating miR-570-3p. Biomed J 2021;44:S296-304. [Crossref] [PubMed]
- Shih CH, Chuang LL, Tsai MH, et al. Hypoxia-Induced MALAT1 Promotes the Proliferation and Migration of Breast Cancer Cells by Sponging MiR-3064-5p. Front Oncol 2021;11:658151. [Crossref] [PubMed]
- Huang Y, Zhou Z, Zhang J, et al. lncRNA MALAT1 participates in metformin inhibiting the proliferation of breast cancer cell. J Cell Mol Med 2021;25:7135-45. [Crossref] [PubMed]
- Meseure D, Vacher S, Lallemand F, et al. Prognostic value of a newly identified MALAT1 alternatively spliced transcript in breast cancer. Br J Cancer 2016;114:1395-404. [Crossref] [PubMed]
- Jadaliha M, Zong X, Malakar P, et al. Functional and prognostic significance of long non-coding RNA MALAT1 as a metastasis driver in ER negative lymph node negative breast cancer. Oncotarget 2016;7:40418-36. [Crossref] [PubMed]
- Ma D, Chen C, Wu J, et al. Up-regulated lncRNA AFAP1-AS1 indicates a poor prognosis and promotes carcinogenesis of breast cancer. Breast Cancer 2019;26:74-83. [Crossref] [PubMed]
- Dianatpour A, Faramarzi S, Geranpayeh L, et al. Expression analysis of AFAP1-AS1 and AFAP1 in breast cancer. Cancer Biomark 2018;22:49-54. [Crossref] [PubMed]
- Zhang X, Zhou Y, Mao F, et al. lncRNA AFAP1-AS1 promotes triple negative breast cancer cell proliferation and invasion via targeting miR-145 to regulate MTH1 expression. Sci Rep 2020;10:7662. [Crossref] [PubMed]
- Wang J, Katsaros D, Biglia N, et al. LncRNA ZNF582-AS1 Expression and Methylation in Breast Cancer and Its Biological and Clinical Implications. Cancers (Basel) 2022;14:2788. [Crossref] [PubMed]
(English Language Editor: L. Huleatt)