Integrated analysis of single-cell and bulk transcriptome identifies a signature based on NK cell marker genes to predict prognosis and therapeutic response in clear cell renal cell carcinoma
Highlight box
Key findings
• A novel signature derived from NK cell marker genes was constructed to predict ccRCC patient prognosis and guide individualized therapies.
What is known and what is new?
• As an important part of innate immune system, NK cells play an important role in anti-tumor immunity.
• Based on NK cell marker genes using scRNA-seq analysis, a signature was constructed and validated to predict prognosis and therapeutic response of ccRCC patients.
What is the implication, and what should change now?
• Our signature could guide clinical practice and individualized therapy, while further investigations are needed to validate our signature and explore the underlying mechanisms.
Introduction
Renal cell carcinoma (RCC) is the most lethal of all urinary malignant tumors that accounts for 2.2% of the total cancer incidence in 2020 worldwide, claiming 1.8% of all the deaths (1). Clear cell RCC (ccRCC) is the most common subtype of RCC and is responsible for most of the RCC-related mortality (2,3). Although early and localized tumors can be treated by surgical or ablative methods, one-third of cases will still present with or develop metastases (4). With the innovation of biological technologies, targeted and immune therapies have been widely concerned, which dramatically improved the outcomes of metastatic ccRCC patients.
ccRCC is highly immunogenic, with tumor-infiltrating immune cells and immunomodulatory molecules serving as key determinants of tumor progression, metastasis and immunotherapy efficacy (5). While research on the role of adaptive immune system has received much attention, little is known about innate immune cells in the prognosis and therapeutic efficacy of solid tumors. Natural killer (NK) cells, as a subset of lymphocytes that mainly participate in innate immunity, play a key role in anti-tumor effects (6). Other than that the anti-tumor effect of T cells depends on major histocompatibility complex (MHC), NK cells can directly recognize and kill tumor cells early in the disease, and thus regarded as the first line of defense against tumors (7). Additionally, NK cells can interact with various immunocytes associated with tumor microenvironment (TME), including macrophages, dendritic cells (DCs) and T cells, leading to the synthesis and secretion of cytokines, chemokines, and growth factors, etc. (8-10). However, current research demonstrated that tumor-infiltrating NK cells are inactive and dysfunctional, which could inhibit the immune response and block functions of killer cells, highlighting the potent role of NK cells in shaping anti-tumor immunity (10-12). In previous studies, the abundance of tumor-infiltrating NK cells has been reported to be markedly correlated with the prognosis of patients with various solid malignancies, including ccRCC (13-17). As a vital element of the anti-tumor responses in the TME, higher infiltration of NK cells indicated improved survival in ccRCC patients (18). However, few studies have focused on the molecular analysis of NK cells in ccRCC, which necessitates further investigations.
In the recent years, the development of single-cell RNA-sequencing (scRNA-seq) has provided an efficient opportunity to identify cell phenotypes in TME (19). Prior studies have used scRNA-seq profiles to construct gene signatures based on the molecular features of specific immune cells to predict the prognosis and immunotherapy response of cancer patiens (20-22). Inspired by these studies, we analyzed scRNA-seq data from ccRCC samples and identified genetic markers of tumor-infiltrated NK cells. After constructing a prognostic NK cell marker gene signature (NKMS) on The Cancer Genome Atlas (TCGA) cohort, comprehensive analysis was conducted to evaluate and validate the predictive performance of NKMS. Furthermore, the correlation between NKMS and TME, as well as therapeutic responses was investigated. We present this article in accordance with the TRIPOD reporting checklist (available at https://tcr.amegroups.com/article/view/10.21037/tcr-22-2782/rc).
Methods
Data source and acquisition
The schematic flowchart of our study is shown in Figure 1. The 10x Genomics scRNA-seq files of 9 ccRCC samples (GSE152938, n=2; GSE159115, n=7) were downloaded from the Gene Expression Omnibus (GEO) database (https://www.ncbi.nlm.nih.gov/geo/) for the identification of NK cell cluster. The bulk tumor transcriptomic and matched clinical profiles of 532 ccRCC patients and masked somatic mutation data of 358 patients in TCGA-kidney renal clear cell carcinoma (KIRC) cohort (release number: 32.0, release data: 2022-03-29) were downloaded from Genomic Data Commons Data Portal (https://portal.gdc.cancer.gov/) of TCGA for further screening of survival-related genes and signature construction. To verify the prognostic capability of the signature, we obtained expression profiles and clinical features of two independent external cohorts, E-MTAB-1980 cohort from ArrayExpress and RECA-EU from International Cancer Genome Consortium (ICGC). In addition, we acquired two therapy cohorts with transcriptomic and clinical data of ccRCC patients, PMID32472114 from online supplementary data and E-MTAB-3267 from ArrayExpress, to test the predictive ability of the signature in therapeutic response (23). All data used in our study were obtained from publicly available databases and did not require approval of the local ethics committee. Our study was conducted in accordance with the Helsinki Declaration (as revised in 2013).
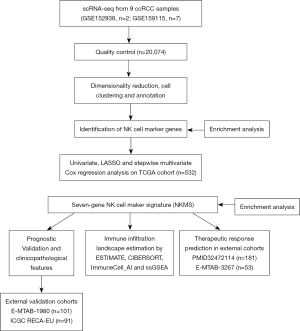
Identification of NK cell marker genes by scRNA-seq analysis
The ccRCC scRNA-seq data were analyzed with the R package “Seurat” (version 4.1.1) (24). After ScRNA-seq data of ccRCC samples merged with “merge” function, the cells were filtered with the following quality control metrics: (I) cells with more than 10% of mitochondrial counts; (II) cells with unique feature counts over 3,000 or less than 200. Then, “NormalizeData” function was utilized to normalize the data with “LogNormalize” method. The “ScaleData” function was subsequently applied to perform a linear transformation on expression values and remove the heterogeneity associated with mitochondrial contamination. Afterwards, the top 2,000 variably expressed genes were included to perform principal component analysis (PCA). Thirty PCs with P<0.05 evaluated by JackStraw analysis were selected for non-linear dimensional reduction using the algorithm of uniform manifold approximation and projection (UMAP) with “RunUMAP” function. The “FindClusters” function was utilized to cluster the cells with resolution of 0.3, and the R package “SingleR” (version 1.10.0) was applied to annotate the cell types using a reference dataset from the Human Primary Cell Atlas and typical cell markers (25,26). Then, “FindAllMarkers” function was used to identify distinct genes of each cluster with minimal percentage of cells in the cluster where the gene is detected >0.70. Genes that exhibited an absolute log2(fold change) >1 and adjusted P value <0.01 were considered as the marker genes.
Construction and validation of prognostic signature based on NK cell marker genes
The TCGA-KIRC cohort was applied as the training dataset to construct the signature. The R package “survival” (version 3.4-0) was utilized to perform the univariate Cox regression analysis to investigate the association between the expression of NK cell marker genes and the overall survival (OS) of ccRCC patients in TCGA-KIRC cohort. Marker genes with P<0.05 by univariate Cox regression were considered as candidate prognostic genes. The R package “glmnet” (version 4.1-4) was utilized to identify the prognostic hub genes from candidate genes by the least absolute shrinkage and selection operator (LASSO) Cox proportional hazards regression (27). Optimal value of tuning parameter (λ) was determined by 20-fold cross-validation with minimum criteria. “Step” function was applied to conduct the stepwise multivariate Cox regression analysis to determine the most predictive genes with P<0.1. Based on the signature built, a risk score equation was established:
β(i), the Cox regression coefficient, represented the weight of ith gene included, and Exp(i) represented the expression value of ith gene. Then, the patients in TCGA-KIRC were classified into high- or low-risk group according to the median NKMS scores. “Survminer” package (version 0.4.9) was applied to evaluate the relationship between NKMS scores and OS, progression-free survival (PFS) of ccRCC patients via Kaplan-Meier analyses, and log-rank test was utilized to estimate the significance of differences. The predictive value of this risk model was verified in two independent validation sets, which were E-MTAB-1980 with 101 ccRCC patients and RECA-EU with 91 ccRCC patients. Detailed information of TCGA-KIRC and validation sets were listed in Table 1. After normalization of mRNA expression and calculation of NKMS scores, the optimal cut-off value of NKMS scores in validating sets was determined by the “surv_cutpoint” function. The Kaplan-Meier method and log-rank test were used for survival analysis. Besides, the predictive ability of the risk model was also evaluated by the area under the curve (AUC) in time-dependent receiver operating characteristic (ROC) analysis using “pROC” R package (version 1.18.0).
Table 1
Variables | TCGA (n=532) | E-MTAB-1980 (n=101) | RECA-EU (n=91) |
---|---|---|---|
Age (years) | |||
Median | 61 | 64 | 60 |
Range | 26–90 | 35–91 | 35–83 |
Gender, n | |||
Male | 345 | 77 | 52 |
Female | 187 | 24 | 39 |
AJCC stage, n | |||
I | 266 | 66 | 54 |
II | 57 | 10 | 13 |
III | 123 | 13 | 17 |
IV | 83 | 12 | 7 |
NA | 3 | 0 | 0 |
Fuhrman grade, n | |||
1 | 14 | 13 | – |
2 | 228 | 59 | – |
3 | 206 | 22 | – |
4 | 76 | 5 | – |
NA | 8 | 2 | – |
OS status, n | |||
Alive | 357 | 78 | 61 |
Deceased | 175 | 23 | 30 |
ccRCC, clear cell renal cell carcinoma; TCGA, The Cancer Genome Atlas; AJCC, American Joint Committee on Cancer; NA, not available; OS, overall survival.
Nomogram construction
“Survival” package was utilized to perform univariate and subsequent multivariate Cox regression analyses to determine whether our signature was independent of several clinical variables. Then, a nomogram was constructed based on results of multivariate Cox regression analysis in the TCGA-KIRC cohort with “rms” (version 6.3-0) package. The total points accumulated by various covariates correspond to the predicted probability for a patient. The concordance index (C-index) was calculated to evaluate the predictive ability of the nomogram. Theoretically, a higher C-index indicates a greater level of precision in predictions. Calibration curves were used to examine the consistency between predicted and actual survival outcome. Ninety-five percent confidence interval (CI) of C-index and calibration curves were calculated by bootstrap-based 1,000 iterations resampling method.
Pathway and function enrichment analysis
“Limma” package (version 3.52.2) was implemented to screen differentially expressed genes (DEGs). False discovery rate (FDR) <0.05 and absolute value of log2(fold change) >1 was defined as the marked threshold of DEGs. Pathway and functional enrichment analysis of Kyoko Encyclopedia of Genes and Genomes (KEGG) and gene ontology (GO) were performed by Metascape, an online gene annotation and analysis resource (28).
Somatic variants analysis
“Maftools” package (version 2.12.0) was used to analyze somatic variants (29). “Oncoplot” function was applied to visualize the overall mutation status in high- and low-risk groups. “Tmb” function calculated tumor mutation burden (TMB) of two groups. “MafCompare” and “forestPlot” functions compared the differentially mutated genes between subgroups.
TME infiltration estimation
The “IOBR” R package (version 0.99.9) integrates 8 published algorithms for TME contexture estimation and was used to apply the ESTIMATE and CIBERSORT algorithms to explore the immune cells abundance between high- and low-risk groups in TCGA and E-MTAB1980 cohorts (30-32). For verification, the ImmuneCell_AI algorithm with “ImmuCellAI” package (version 0.1.0) and single sample gene set enrichment analysis (ssGSEA) with “GSVA” package (version 1.44.5) were employed for TME immune infiltration estimation (33,34). The 29 immune-related signatures for ssGSEA analysis were listed in website: https://cdn.amegroups.cn/static/public/tcr-22-2782-1.xlsx. The immune-related molecules were analyzed to understand the immune infiltration in ccRCC.
Therapeutic response prediction
To predict the response to immune checkpoint inhibitors (ICIs), we compared the number of unique T-cell receptors (TCRs richness) and the relative abundance (Shannon diversity index) of different TCRs in subgroups of TCGA-KIRC, which were obtained from the Pan-Cancer Atlas study (35). We then further explored a study (PMID32472114) which contained 181 metastatic ccRCC patients treated with nivolumab to evaluate the predictive capacity of NKMS for ICI. Additionally, we investigated whether NKMS could predict response to antiangiogenic therapy by estimating the differences in angiogenic gene expression between high- and low-risk groups in TCGA. Meanwhile, the predictive ability of NKMS was tested in E-MTAB-3267 cohort with 53 metastatic ccRCC patients treated with sunitinib.
Statistical analysis
R software (version 4.2.1) was utilized for data processing and generation of figures. Differences between variables were analyzed with Wilcoxon test and chi-square test. P<0.05 was statistically significant.
Results
scRNA-seq profiling, clustering and NK cell markers identification
After preprocessing the scRNA-seq data with the criteria mentioned, GSM4819726 and GSM4819728 were discarded due to high mitochondrial counts (Figure S1A), and 20,074 high-quality cell samples from 7 ccRCC samples were isolated for subsequent analysis. The Pearson’s correlation coefficient was 0.92 between numbers of detected genes (nFeatures) and sequencing depth (nCount) (Figure 2A). After the reduction of dimensionality by PCA (Figure 2B), UMAP algorithm was implemented on the top 30 principal components (PCs) for visualization of high dimensional scRNA-seq data. The cell samples were then divided into 25 clusters (Figure 2C), and the top 5 genes differentially expressed in each cluster were visualized in heatmap (Figure S1B). Afterwards, 8 types of cells were annotated with “SingleR” and corresponding cell markers, and the cells in cluster 6 were identified as NK cells (Figure 2D). The top 5 distinctive genes in each cluster were depicted in Figure 2E. As shown in Figure 2F, CD16 (FCGR3A), KLRD1, KLRB1, PRF1, CX3CR1, GZMB, GNLY, and SPON2 were highly expressed in the NK cell cluster, which were reported as NK and cytotoxic cell markers (36-38). The distinct gene expression profiles of cluster 6 were further analyzed. As a result, 52 genes were identified as NK cell marker genes of ccRCC (https://cdn.amegroups.cn/static/public/tcr-22-2782-2.xlsx). GO analysis showed 52 NK cell marker genes were markedly associated with cytolysis, cell activation and cell killing (Figure S2A), while KEGG analysis illustrated that NK cell marker genes participated in NK cell mediated cytotoxicity and leukocyte transendothelial migration (Figure S2B). These results confirmed the identification of the NK cell cluster.
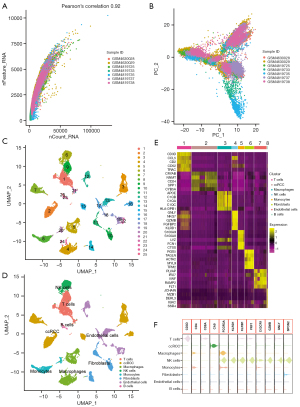
Construction of prognostic signature on NK cell marker genes
After performing a univariate Cox proportional regression analysis on the TCGA-KIRC training set, 22 out of 52 NK cell markers were exhibited significantly associated with OS (P<0.05, Figure S2C). After applying the LASSO Cox regression model on prognostic genes, 18 genes with minimal λ were screened out (Figure 3A,3B). Subsequently, a stepwise multivariate Cox regression analysis was conducted to optimize the prognostic signature to include the 7 most predictive genes (CLEC2B, PLAC8, CD7, SH3BGRL3, CALM1, KLRF1, and JAK1) (Figure 3C). The risk score was calculated as followed: NKMS score = (0.237 × CLEC2B expression) + (0.203 × PLAC8 expression) + (0.132 × CD7 expression) + (0.124 × SH3BGRL3 expression) + (−0.287 × CALM1 expression) + (−0.296 × KLRF1 expression) + (−0.308 × JAK1 expression). Among the 7 prognostic genes, 4 (CLEC2B, PLAC8, CD7, and SH3BGRL3) were regarded as risk genes [Cox hazard ratio (HR) >1], while 3 (CALM1, KLRF1, and JAK1) were protective (Cox HR <1) (Figure 3D). The risk score of each patient in TCGA-KIRC cohort was calculated (https://cdn.amegroups.cn/static/public/tcr-22-2782-3.xlsx) and the patients were sorted into high-risk (n=266) and low-risk groups (n=266) based on the median score (−0.101, Figure 3E). High-risk group comprised more deceased cases than low-risk group (Figure 3F). Kaplan-Meier analysis demonstrated that ccRCC patients in the high-risk group had significantly lower OS and PFS than low-risk patients (P<0.0001, Figure 3G,3H). The predictive accuracy of this signature was 0.724, 0.716, and 0.728 at 1, 3, and 5 years, respectively (Figure 3I).
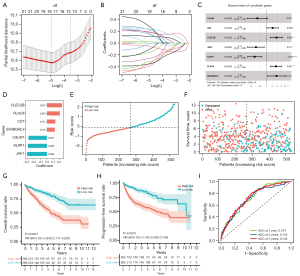
Validation of the gene signature
To examine the predictive performance of NKMS, two external cohorts (E-MTAB-1980, n=101; ICGC RECA-EU cohort, n=91) were enrolled for verification. The characteristics of TCGA-KIRC and two external cohorts are shown in Table 1. The NKMS score of each patient was calculated with the formula established after expression data normalized. The heatmaps visualized the distribution of genes in NKMS in different risk groups (Figure 4A,4B). Survival analysis depicted significantly lower OS in high-risk groups of both external cohorts (HR =5.90, P<0.001 in E-MTAB-1980, Figure 4C; HR =2.66, P=0.006 in RECA-EU, Figure 4D). The time-dependent ROC curve of two cohorts are shown in Figure 4E,4F. These findings revealed strong predictive performance of NKMS in ccRCC.
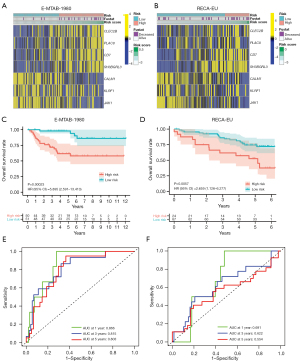
Higher NKMS score was correlated with advanced ccRCC progression
First, we evaluated the correlation between risk scores and clinical parameters in TCGA and E-MTAB-1980 cohorts which contained sufficient clinical information. In these two cohorts, higher NKMS scores were markedly enriched in groups of advanced American Joint Committee on Cancer (AJCC) stages, unfavorable survival status, high Fuhrman grade, high pT and pN stage (Figure 5A,5B). Then, survival analysis in TCGA clinical subgroups indicated that patients in high-risk group suffered shorter OS and PFS stratified by young (OS, HR =3.60, P<0.001, Figure S3A; PFS, HR =3.55, P<0.001, Figure S3B) or elder (OS, HR =2.73, P<0.001, Figure S3C; PFS, HR =2.38, P<0.001, Figure S3D), male (OS, HR =2.20, P<0.001, Figure S3E; PFS, HR =2.15, P<0.001, Figure S3F) or female (OS, HR =4.62, P<0.001, Figure S3G; PFS, HR =3.88, P<0.001, Figure S3H), low (OS, HR =1.96, P=0.033, Figure S3I; PFS, HR =3.31, P=0.002, Figure S3J) or high Fuhrman grade (OS, HR =2.23, P<0.001, Figure S3K; PFS, HR =1.87, P<0.001, Figure S3L), early (OS, HR =2.12, P=0.007, Figure S3M; PFS, HR =2.11, P=0.023, Figure S3N) or advanced AJCC stage (OS, HR =1.89, P<0.001, Figure S3O; PFS, HR =1.59, P=0.012, Figure S3P).
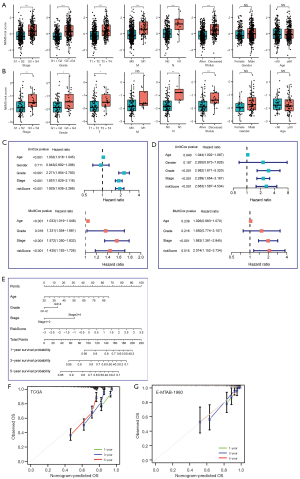
NKMS was an independent prognostic factor in ccRCC patients
To further explore the prognostic value of NKMS in ccRCC patients, univariate and multivariate Cox regression analyses were performed on clinical features and NKMS risk scores in TCGA-KIRC and E-MTAB-1980 cohorts, the results of which confirmed NKMS score was an independent prognostic factor of ccRCC (multivariate HR =1.44, P<0.001, TCGA, Figure 5C; multivariate HR =2.07, P=0.015, E-MTAB-1980, Figure 5D). Then, a nomogram was built based on the multivariate Cox regression coefficients of NKMS score and clinical parameters (age, AJCC stage, and Fuhrman grade) in TCGA cohort (Figure 5E) and was validated in E-MTAB-1980 cohort. The C-index of the nomogram was 0.757 (95% CI: 0.722–0.791) in TCGA cohort and 0.831 (95% CI: 0.761–0.904) in E-MTAB-1980. The calibration curves demonstrated favorable consistency of predictive 1-, 3-, and 5-year OS probabilities with the ideal predictions both in training and validation cohorts (Figure 5F, TCGA, the training cohort; Figure 5G, E-MTAB-1980, the validation cohort). Taken together, NKMS exhibited preferable capacity as an independent prognostic factor and the nomogram model could be a reliable tool for OS prediction of ccRCC patients.
Functional enrichment analysis of the NKMS related genes
After determining NKMS-related DEGs between high- and low-risk groups in TCGA, 181 DEGs were enriched in high-NKMS group, and 254 were identified in low-NKMS group. The DEGs were visualized by volcano plot in Figure 6A. Analyzed by Metascape, the probable GOs of DEGs in high-NKMS group were immunoglobulin complex, antigen binding, external side of plasma membrane and activation of immune response, while the KEGGs were in terms of cytokine-cytokine receptor interaction, primary immunodeficiency and complement and coagulation cascades (Figure 6B). Meanwhile, the GOs enriched in low-NKMS DEGs were mainly regarding apical part of cell, renal system process, organic hydroxy compound metabolic process and monocarboxylic acid metabolic process (Figure 6C). These findings implied the involvement of DEGs of NKMS in immune regulation and metabolic process.
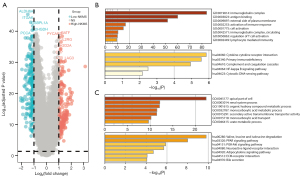
Analysis of somatic variants
The mutation landscapes of high- and low-risk groups in TCGA are visualized by waterfall plot in Figure 7A,7B. VHL, PBRM1 and TTN were most mutated genes with mutation rate >10% both in two groups. Mutation types of the most differentially mutated between two groups are illustrated in Figure 7C, including BAP1, THSD7B, SETD2, KIAA1549L, and SYNE1. Meanwhile, TMB in high-NKMS group was significantly higher than that in low-NKMS group (P<0.01, Figure 7D).
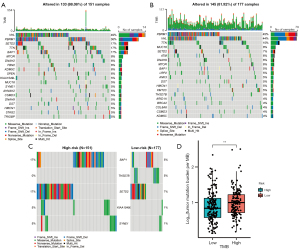
The profiles of immune cell infiltration in NKMS subgroups
To explore the underlying mechanisms between NKMS score and TME infiltration in ccRCC, four independent methods (ESTIMATE, CIBERSORT, ImmuneCell_AI, and ssGSEA) were applied to estimate the relative abundance of immune cells in high- and low-risk groups in TCGA-KIRC cohort (https://cdn.amegroups.cn/static/public/tcr-22-2782-4.xlsx, Figure 8A, Figure S4A-S4D). The immune score and differentially infiltrated immune cell types in TCGA were visualized in Figure 8B. The immune score was markedly elevated in high-risk group, which implied the presence of higher immune infiltration (Figure S4A). CIBERSORT indicated significantly higher estimates of plasma cells, CD8+ T cells, CD4+ memory T cells activated, follicular helper T (Tfh) cells, regulatory T (Treg) cells, NK cells activated and macrophages M0 infiltrated in TME of high-risk tumors. With verification of ImmuneCell_AI and ssGSEA algorithms, higher infiltration of CD8+ T cells, Treg cells and Tfh cells were observed in high-risk group (Figure 8C,8D). Of special note, higher estimates of immunosuppressive subtypes, including inducible Treg (iTreg) cells, natural Treg (nTreg) cells, helper T (Th)1, Th2, and exhausted T cells were also enriched in high-risk group, implying the exhaustion state in high-risk tumors. To prove this assumption, we compared the transcriptional expression of several inhibitory receptors and cytokines between two groups, where overexpression of PD1, CTLA4, TIGIT, LAG3, BTLA, IL-4, and IL-6 was present in high-risk group (Figure 8E). We also verified the higher estimates of Treg and Tfh cells in high-risk group with CIBERSORT in E-MTAB-1980 (Figure 8F). Survival analysis highlighted the impact of infiltrated immune cells, which showed that patients with high infiltration of Treg cells and Tfh cells suffered significantly shorter OS (Figure S4E-S4G). Taken together, evidence indicated the existence of immunosuppressed phenotype in high-NKMS ccRCC tumors, and the differences in immune cell infiltration could have a great impact on patient survival.
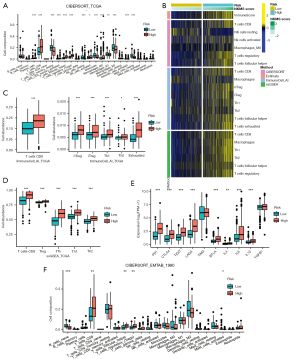
The performance of therapeutic prediction of NKMS in ccRCC
To assess the immunotherapy efficacy between low- and high-NKMS risk groups, we compared the TCR repertoire of two groups and found that both TCR diversity (Figure 9A) and richness (Figure 9B) of ccRCC patients in high-risk group were markedly higher than that in low-risk group in TCGA-KIRC. As mentioned above, several inhibitory molecules were upregulated in high-risk group (Figure 8F) and TMB in high-risk group was significantly higher than that in low-risk group (Figure 7D). Taken together, these results indicated better immunotherapy response in high-risk group. To validate our assumption, in PMID32472114 which comprised 181 metastatic ccRCC patients treated by nivolumab, favorable outcome with prolonged PFS was observed in high-risk group (Figure 9C, HR =0.68, P=0.028). Patients in high-risk group tended to receive more clinical benefits than in low-risk group (chi-square test P=0.076, Figure 9D).
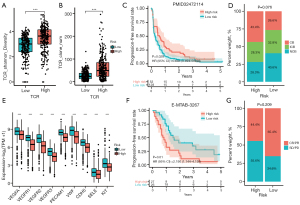
As shown in Figure 9E, we found significant higher expression of angiogenesis-related genes in low-risk group, several of which were targeted by tyrosine kinase inhibitors (TKIs), including VEGFA, VEGFR1, VEGFR2, VEGFR3, and KIT. This result implied patients in low-risk patients might be more sensitive to anti-angiogenic therapies. In E-MTAB-3267 cohort with 53 metastatic ccRCC patients treated with sunitinib, patients in low-risk group had significantly prolonged PFS (Figure 9F, HR =2.19, P=0.01), which supported our hypothesis. However, though higher proportion of patients in low-risk group responded to sunitinib treatment, no statistical significance was achieved (chi-square test P=0.209, Figure 9G). These findings demonstrated that ccRCC patients in high-risk group were more sensitive to ICIs, while patients in low-risk group were more likely to benefit from anti-angiogenic therapies.
Discussion
The advent of scRNA-seq technology has made it possible to explore heterogeneous gene expression profiles at the single-cell level. This technology provides new insights into the molecular characteristics of tumor-infiltrating immune cells in TME which impacts the tumor progression and immunotherapy efficacy (39). Recent studies have revealed the mighty capability of signatures based on NK cell marker genes to predict the prognosis and therapeutic response in lung adenocarcinoma and cutaneous melanoma (21,40). Given the complexity and heterogeneity of ccRCC TME, we were inspired to explore the tumor-infiltrating NK cells in ccRCC. In the present study, we analyzed the ccRCC scRNA-seq dataset from GEO, demonstrated the NK cell subset and identified NK cell marker genes which could not be discriminated from the bulk RNA-seq analysis. Herein, a novel prognostic signature based on NK cell marker genes (NKMS) was constructed, and the prognostic value was validated in two independent external cohorts and clinical subgroups. Higher NKMS scores were markedly correlated with advanced clinical manifestations. Univariate and multivariate Cox regression analysis proved the NKMS was an independent risk indicator for the OS of ccRCC patients. Overall, NKMS was proved to be an exceptional predictive tool for the prognosis of ccRCC patients.
In our study, the NKMS was composed of 7 NK cell marker (CLEC2B, PLAC8, CD7, SH3BGRL3, CALM1, KLRF1, and JAK1), which were all reported to be involved in the oncogenesis. CLEC2B, also known as activation-induced C-type lectin (AICL), is an identified ligand for NK-activating receptor KLRF1 (previously called NKp80) and is of importance in NK cell activation and regulation (41,42). PLAC8 is a small 12.5 kDa protein and has been reported to function in death of human lymphocytes, adipocyte differentiation and human diseases, including cancer (43). Overexpression of PLAC8 has been demonstrated to be correlated with advanced tumor progression and impaired prognosis of ccRCC (44). CD7 is a 40-kDa glycoprotein, widely expressed on cell surface of mature T cells and NK cells and involved in signal transduction and regulation of T-cell proliferation (45). SH3BGRL3 contains 93 amino acids and can inhibit tumor necrosis factor-α (TNF-α)-induced apoptosis while promoting cell survival (46). Previous studies have addressed SH3BGRL3 as a potential oncogene in the tumorigenesis of glioblastoma and urothelial carcinoma (46,47). CALM1 is a ubiquitous calcium ion (Ca2+) receptor protein and plays pivotal role in signaling pathways that modulates proliferation, migration and differentiation (48). Han et al. reported that downregulated expression of CALM1 was significantly correlated with increasing tumor size in hepatocarcinoma patients, which was consistent as a protective factor in our study (49). The presence of NK-like innate lymphoid cells with high KLRF1 expression in peripheral blood mononuclear cells (PBMCs) is reported to be associated with better PFS in large hepatocellular cohorts (50). JAK1 is involved in IL-6 class cytokine signaling and the activation of JAK1 has been reported to be related to the overexpression of immune checkpoint molecules, thus influencing the efficacy of immunotherapies (51). Chen et al. found JAK1 as a protective biomarker in breast cancer, which was consistent with our result (52). These genes identified in NKMS might be potential targets for further understanding of molecular mechanisms in ccRCC.
As the immune-related GOs enriched in high-risk group, we focused on immune cell infiltration in TME of ccRCC. Our study depicted large differences in the composition of tumor-infiltrating immune cells between NKMS subgroups. First, the immune score of the high-risk group deduced by ESTIMATE algorithm was significantly higher than that of low-risk group, indicating more complex TME in high-risk tumors. Most notably, higher infiltration of Tregs was enriched in high-risk group, which was affirmed by CIBERSORT, ImuuneCell_AI, and ssGSEA algorithms, and validated in E-MTAB-1980 cohort. Tregs are described as a subset of CD4+ T cells that exert a strong inhibitory effect on either innate or adaptive immune system (53,54), leading to dysfunctional effector T cells with reduced secretion of cytokines and increased expression of inhibitory receptors (55). We also found that higher infiltration of Tregs was markedly associated with an unfavorable prognosis of ccRCC patients, which is concurred with previous findings (56). The higher infiltration of Tregs in high-risk group might be correlated with the immunosuppressive status, which was reflected by overexpression of various inhibitory molecules, including PD1, CTLA4, TIGIT, LAG3, BTLA, IL-4, and IL-6. As a result, the suppressive TME could lead to the dysfunction of immune effective cells and impaired anti-tumor responses, like CD8+ T cells and NK cells, which has been identified in previous study (57). CD8+ T cells have been described as cytotoxic T cells and the positive prognostic value has been discovered in various malignancies (56). However, recent study has revealed the exhausted functional state of CD8+ T cells in the ccRCC TME, where high expression of LAG3 and TIM3 was observed in tumor infiltrating CD8+ T cells (58). Meanwhile, the high infiltration of exhausted CD8+ T cells was correlated with a poor prognosis (58-60). In our study, higher infiltration of CD8+ T cells was present in high-risk group without prognostic significance, which also implied the hypofunctional status of CD8+ T cells. Therefore, patients with high NKMS scores might be more sensitive to immunotherapy. Intriguingly, higher infiltration of Tfh cells was also observed and validated in high-risk group as a risk factor for ccRCC patients. Tfh cells are crucial in geminal center formation and modulation, however, the Tfh-associated tumor biology in ccRCC remains poorly understood and deserves further investigation (61).
Nowadays, the emergence of ICIs has largely improved the prognosis of advanced ccRCC patients (62,63). However, only 25–42% ccRCC patients could respond to ICI therapies, which was relatively lower compared to other solid tumors (64). As a result, it is urgent to invent a reliable biomarker which could predict ICI response. As previous studies suggested that high baseline TCR diversity was correlated with better ICI response in variety of cancer types (65), we compared richness and diversity of the TCR repertoire in TCGA and found that high-risk ccRCC patients possessed elevated richness and diversity of TCR. In addition, patients in high-risk group harbored higher TMB, which indicated better response to ICIs. As expected, significantly prolonged PFS of metastatic ccRCC patients treated with nivolumab was observed in high-risk group by investigating PMID32472114 cohort, reflecting the predictive value of NKMS in ICI efficacy. Meanwhile, low-NKMS group exhibited overexpression of various proangiogenic factors (VEGFA, VEGFR1–3, PECAM1, VWF, CDH5, SELE, and KIT), which implied that patients with low-NKMS score might respond better to anti-angiogenic agents. Sunitinib is a multi-target TKI which is one of the first-line anti-angiogenic targeted agents to inhibit vascular endothelial growth factor (VEGF)-associated signaling in metastatic ccRCC patients (64), and low-risk patients in E-MATB-3267 underwent a prolonged PFS and had higher response rate to sunitinib, which validate our assumption to some extent. Collectively, our signature could provide evidence to decide which kind of ccRCC patients may better respond to ICI or anti-angiogenesis therapy, thereby selecting the individualized treatment plan.
There are several limitations in our study. First, the expression and prognostic role of genes in NKMS at protein level needs further investigation. Second, we made the analysis with published data, and the predictive value needs to be testified in a large local cohort. Third, the number of ccRCC patients with advanced AJCC stages is relatively small. Lastly, all the mechanistic analyses in our study are descriptive and need further experimental validations.
Conclusions
Overall, with integrated analysis of scRNA and bulk RNA sequencing data, we constructed a seven-gene signature (NKMS) based on NK cell marker genes which exhibited preferable capacity to predict prognosis and therapeutic response in ccRCC patients. This signature significantly correlates with clinical features as an independent risk indicator and reveals close relationship with immunosuppression in ccRCC. Taken together, the NKMS is a promising biomarker to guide clinical practice and provide individualized therapies for ccRCC patients.
Acknowledgments
Funding: None.
Footnote
Reporting Checklist: The authors have completed the TRIPOD reporting checklist. Available at https://tcr.amegroups.com/article/view/10.21037/tcr-22-2782/rc
Peer Review File: Available at https://tcr.amegroups.com/article/view/10.21037/tcr-22-2782/prf
Conflicts of Interest: All authors have completed the ICMJE uniform disclosure form (available at https://tcr.amegroups.com/article/view/10.21037/tcr-22-2782/coif). The authors have no conflicts of interest to declare.
Ethical Statement: The authors are accountable for all aspects of the work in ensuring that questions related to the accuracy or integrity of any part of the work are appropriately investigated and resolved. The study was conducted in accordance with the Declaration of Helsinki (as revised in 2013).
Open Access Statement: This is an Open Access article distributed in accordance with the Creative Commons Attribution-NonCommercial-NoDerivs 4.0 International License (CC BY-NC-ND 4.0), which permits the non-commercial replication and distribution of the article with the strict proviso that no changes or edits are made and the original work is properly cited (including links to both the formal publication through the relevant DOI and the license). See: https://creativecommons.org/licenses/by-nc-nd/4.0/.
References
- Sung H, Ferlay J, Siegel RL, et al. Global Cancer Statistics 2020: GLOBOCAN Estimates of Incidence and Mortality Worldwide for 36 Cancers in 185 Countries. CA Cancer J Clin 2021;71:209-49. [Crossref] [PubMed]
- Hsieh JJ, Purdue MP, Signoretti S, et al. Renal cell carcinoma. Nat Rev Dis Primers 2017;3:17009. [Crossref] [PubMed]
- Moch H, Cubilla AL, Humphrey PA, et al. The 2016 WHO Classification of Tumours of the Urinary System and Male Genital Organs-Part A: Renal, Penile, and Testicular Tumours. Eur Urol 2016;70:93-105. [Crossref] [PubMed]
- Jonasch E, Walker CL, Rathmell WK. Clear cell renal cell carcinoma ontogeny and mechanisms of lethality. Nat Rev Nephrol 2021;17:245-61. [Crossref] [PubMed]
- Geissler K, Fornara P, Lautenschläger C, et al. Immune signature of tumor infiltrating immune cells in renal cancer. Oncoimmunology 2015;4:e985082. [Crossref] [PubMed]
- Hallett WH, Murphy WJ. Natural killer cells: biology and clinical use in cancer therapy. Cell Mol Immunol 2004;1:12-21. [PubMed]
- Vesely MD, Kershaw MH, Schreiber RD, et al. Natural innate and adaptive immunity to cancer. Annu Rev Immunol 2011;29:235-71. [Crossref] [PubMed]
- Schmidt L, Eskiocak B, Kohn R, et al. Enhanced adaptive immune responses in lung adenocarcinoma through natural killer cell stimulation. Proc Natl Acad Sci U S A 2019;116:17460-9. [Crossref] [PubMed]
- Gati A, Da Rocha S, Guerra N, et al. Analysis of the natural killer mediated immune response in metastatic renal cell carcinoma patients. Int J Cancer 2004;109:393-401. [Crossref] [PubMed]
- Zhou Y, Cheng L, Liu L, et al. NK cells are never alone: crosstalk and communication in tumour microenvironments. Mol Cancer 2023;22:34. [Crossref] [PubMed]
- Cantoni C, Huergo-Zapico L, Parodi M, et al. NK Cells, Tumor Cell Transition, and Tumor Progression in Solid Malignancies: New Hints for NK-Based Immunotherapy? J Immunol Res 2016;2016:4684268. [Crossref] [PubMed]
- Hasmim M, Messai Y, Ziani L, et al. Critical Role of Tumor Microenvironment in Shaping NK Cell Functions: Implication of Hypoxic Stress. Front Immunol 2015;6:482. [Crossref] [PubMed]
- Na HY, Park Y, Nam SK, et al. Prognostic significance of natural killer cell-associated markers in gastric cancer: quantitative analysis using multiplex immunohistochemistry. J Transl Med 2021;19:529. [Crossref] [PubMed]
- Wang F, Lau JKC, Yu J. The role of natural killer cell in gastrointestinal cancer: killer or helper. Oncogene 2021;40:717-30. [Crossref] [PubMed]
- Reid FSW, Egoroff N, Pockney PG, et al. A systematic scoping review on natural killer cell function in colorectal cancer. Cancer Immunol Immunother 2021;70:597-606. [Crossref] [PubMed]
- Charap AJ, Enokida T, Brody R, et al. Landscape of natural killer cell activity in head and neck squamous cell carcinoma. J Immunother Cancer 2020;8:e001523. [Crossref] [PubMed]
- Díaz-Montero CM, Rini BI, Finke JH. The immunology of renal cell carcinoma. Nat Rev Nephrol 2020;16:721-35. [Crossref] [PubMed]
- Terrén I, Orrantia A, Mikelez-Alonso I, et al. NK Cell-Based Immunotherapy in Renal Cell Carcinoma. Cancers (Basel) 2020;12:316. [Crossref] [PubMed]
- Chen H, Ye F, Guo G. Revolutionizing immunology with single-cell RNA sequencing. Cell Mol Immunol 2019;16:242-9. [Crossref] [PubMed]
- Yu M, Liu X, Xu H, et al. Comprehensive Evaluation of the m(6)A Regulator Prognostic Risk Score in the Prediction of Immunotherapy Response in Clear Cell Renal Cell Carcinoma. Front Immunol 2022;13:818120. [Crossref] [PubMed]
- Song P, Li W, Guo L, et al. Identification and Validation of a Novel Signature Based on NK Cell Marker Genes to Predict Prognosis and Immunotherapy Response in Lung Adenocarcinoma by Integrated Analysis of Single-Cell and Bulk RNA-Sequencing. Front Immunol 2022;13:850745. [Crossref] [PubMed]
- Zhang F, Yu S, Wu P, et al. Discovery and construction of prognostic model for clear cell renal cell carcinoma based on single-cell and bulk transcriptome analysis. Transl Androl Urol 2021;10:3540-54. [Crossref] [PubMed]
- Braun DA, Hou Y, Bakouny Z, et al. Interplay of somatic alterations and immune infiltration modulates response to PD-1 blockade in advanced clear cell renal cell carcinoma. Nat Med 2020;26:909-18. [Crossref] [PubMed]
- Hao Y, Hao S, Andersen-Nissen E, et al. Integrated analysis of multimodal single-cell data. Cell 2021;184:3573-87.e29. [Crossref] [PubMed]
- Aran D, Looney AP, Liu L, et al. Reference-based analysis of lung single-cell sequencing reveals a transitional profibrotic macrophage. Nat Immunol 2019;20:163-72. [Crossref] [PubMed]
- Su C, Lv Y, Lu W, et al. Single-Cell RNA Sequencing in Multiple Pathologic Types of Renal Cell Carcinoma Revealed Novel Potential Tumor-Specific Markers. Front Oncol 2021;11:719564. [Crossref] [PubMed]
- Tibshirani R. The lasso method for variable selection in the Cox model. Stat Med 1997;16:385-95. [Crossref] [PubMed]
- Zhou Y, Zhou B, Pache L, et al. Metascape provides a biologist-oriented resource for the analysis of systems-level datasets. Nat Commun 2019;10:1523. [Crossref] [PubMed]
- Mayakonda A, Lin DC, Assenov Y, et al. Maftools: efficient and comprehensive analysis of somatic variants in cancer. Genome Res 2018;28:1747-56. [Crossref] [PubMed]
- Zeng D, Ye Z, Shen R, et al. IOBR: Multi-Omics Immuno-Oncology Biological Research to Decode Tumor Microenvironment and Signatures. Front Immunol 2021;12:687975. [Crossref] [PubMed]
- Newman AM, Liu CL, Green MR, et al. Robust enumeration of cell subsets from tissue expression profiles. Nat Methods 2015;12:453-7. [Crossref] [PubMed]
- Yoshihara K, Shahmoradgoli M, Martínez E, et al. Inferring tumour purity and stromal and immune cell admixture from expression data. Nat Commun 2013;4:2612. [Crossref] [PubMed]
- Miao YR, Zhang Q, Lei Q, et al. ImmuCellAI: A Unique Method for Comprehensive T-Cell Subsets Abundance Prediction and its Application in Cancer Immunotherapy. Adv Sci (Weinh) 2020;7:1902880. [Crossref] [PubMed]
- Hänzelmann S, Castelo R, Guinney J. GSVA: gene set variation analysis for microarray and RNA-seq data. BMC Bioinformatics 2013;14:7. [Crossref] [PubMed]
- Thorsson V, Gibbs DL, Brown SD, et al. The Immune Landscape of Cancer. Immunity 2018;48:812-30.e14. [Crossref] [PubMed]
- Mathewson ND, Ashenberg O, Tirosh I, et al. Inhibitory CD161 receptor identified in glioma-infiltrating T cells by single-cell analysis. Cell 2021;184:1281-98.e26. [Crossref] [PubMed]
- Yu D, Cai W, Chen X, et al. Natural Killer Cells Disrupt Nerve Fibers by Granzyme H in Atheriosclerotic Cerebral Small Vessel Disease. J Gerontol A Biol Sci Med Sci 2023;78:414-23. [Crossref] [PubMed]
- Crinier A, Milpied P, Escalière B, et al. High-Dimensional Single-Cell Analysis Identifies Organ-Specific Signatures and Conserved NK Cell Subsets in Humans and Mice. Immunity 2018;49:971-86.e5. [Crossref] [PubMed]
- Hinshaw DC, Shevde LA. The Tumor Microenvironment Innately Modulates Cancer Progression. Cancer Res 2019;79:4557-66. [Crossref] [PubMed]
- Cursons J, Souza-Fonseca-Guimaraes F, Foroutan M, et al. A Gene Signature Predicting Natural Killer Cell Infiltration and Improved Survival in Melanoma Patients. Cancer Immunol Res 2019;7:1162-74. [Crossref] [PubMed]
- Deng G, Zheng X, Zhou J, et al. Generation and preclinical characterization of an NKp80-Fc fusion protein for redirected cytolysis of natural killer (NK) cells against leukemia. J Biol Chem 2015;290:22474-84. [Crossref] [PubMed]
- Klimosch SN, Bartel Y, Wiemann S, et al. Genetically coupled receptor-ligand pair NKp80-AICL enables autonomous control of human NK cell responses. Blood 2013;122:2380-9. [Crossref] [PubMed]
- Chen W, Wu J, Wang W, et al. PLAC8 Overexpression Promotes Lung Cancer Cell Growth via Wnt/β-Catenin Signaling. J Immunol Res 2022;2022:8854196. [Crossref] [PubMed]
- Shi L, Xiao L, Heng B, et al. Overexpression of placenta specific 8 is associated with malignant progression and poor prognosis of clear cell renal cell carcinoma. Int Urol Nephrol 2017;49:1165-76. [Crossref] [PubMed]
- Haftcheshmeh SM, Tajbakhsh A, Kazemi M, et al. The clinical importance of CD4+ CD7- in human diseases. J Cell Physiol 2019;234:1179-89. [Crossref] [PubMed]
- Chiang CY, Pan CC, Chang HY, et al. SH3BGRL3 Protein as a Potential Prognostic Biomarker for Urothelial Carcinoma: A Novel Binding Partner of Epidermal Growth Factor Receptor. Clin Cancer Res 2015;21:5601-11. [Crossref] [PubMed]
- Nie Z, Cheng D, Pan C, et al. SH3BGRL3, transcribed by STAT3, facilitates glioblastoma tumorigenesis by activating STAT3 signaling. Biochem Biophys Res Commun 2021;556:114-20. [Crossref] [PubMed]
- Liu T, Han X, Zheng S, et al. CALM1 promotes progression and dampens chemosensitivity to EGFR inhibitor in esophageal squamous cell carcinoma. Cancer Cell Int 2021;21:121. [Crossref] [PubMed]
- Han Z, Feng W, Hu R, et al. RNA-seq profiling reveals PBMC RNA as a potential biomarker for hepatocellular carcinoma. Sci Rep 2021;11:17797. [Crossref] [PubMed]
- Heinrich B, Ruf B, Subramanyam V, et al. Checkpoint Inhibitors Modulate Plasticity of Innate Lymphoid Cells in Peripheral Blood of Patients With Hepatocellular Carcinoma. Front Immunol 2022;13:849958. [Crossref] [PubMed]
- Chan LC, Li CW, Xia W, et al. IL-6/JAK1 pathway drives PD-L1 Y112 phosphorylation to promote cancer immune evasion. J Clin Invest 2019;129:3324-38. [Crossref] [PubMed]
- Chen B, Lai J, Dai D, et al. JAK1 as a prognostic marker and its correlation with immune infiltrates in breast cancer. Aging (Albany NY) 2019;11:11124-35. [Crossref] [PubMed]
- Knochelmann HM, Dwyer CJ, Bailey SR, et al. When worlds collide: Th17 and Treg cells in cancer and autoimmunity. Cell Mol Immunol 2018;15:458-69. [Crossref] [PubMed]
- Farhood B, Najafi M, Mortezaee K. CD8+ cytotoxic T lymphocytes in cancer immunotherapy: A review. J Cell Physiol 2019;234:8509-21. [Crossref] [PubMed]
- Blank CU, Haining WN, Held W, et al. Defining 'T cell exhaustion'. Nat Rev Immunol 2019;19:665-74. [Crossref] [PubMed]
- Bruni D, Angell HK, Galon J. The immune contexture and Immunoscore in cancer prognosis and therapeutic efficacy. Nat Rev Cancer 2020;20:662-80. [Crossref] [PubMed]
- Lee MH, Järvinen P, Nísen H, et al. T and NK cell abundance defines two distinct subgroups of renal cell carcinoma. Oncoimmunology 2022;11:1993042. [Crossref] [PubMed]
- Hu J, Chen Z, Bao L, et al. Single-Cell Transcriptome Analysis Reveals Intratumoral Heterogeneity in ccRCC, which Results in Different Clinical Outcomes. Mol Ther 2020;28:1658-72. [Crossref] [PubMed]
- Giraldo NA, Becht E, Pagès F, et al. Orchestration and Prognostic Significance of Immune Checkpoints in the Microenvironment of Primary and Metastatic Renal Cell Cancer. Clin Cancer Res 2015;21:3031-40. [Crossref] [PubMed]
- Drake CG, Stein MN. The Immunobiology of Kidney Cancer. J Clin Oncol 2018; Epub ahead of print. [Crossref] [PubMed]
- Crotty S. T Follicular Helper Cell Biology: A Decade of Discovery and Diseases. Immunity 2019;50:1132-48. [Crossref] [PubMed]
- Deleuze A, Saout J, Dugay F, et al. Immunotherapy in Renal Cell Carcinoma: The Future Is Now. Int J Mol Sci 2020;21:2532. [Crossref] [PubMed]
- Kase AM, George DJ, Ramalingam S. Clear Cell Renal Cell Carcinoma: From Biology to Treatment. Cancers (Basel) 2023;15:665. [Crossref] [PubMed]
- Kim MC, Jin Z, Kolb R, et al. Updates on Immunotherapy and Immune Landscape in Renal Clear Cell Carcinoma. Cancers (Basel) 2021;13:5856. [Crossref] [PubMed]
- Cardinale A, De Luca CD, Locatelli F, et al. Thymic Function and T-Cell Receptor Repertoire Diversity: Implications for Patient Response to Checkpoint Blockade Immunotherapy. Front Immunol 2021;12:752042. [Crossref] [PubMed]