Identification and validation of an epithelial-mesenchymal transition-related lncRNA pairs prognostic model for gastric cancer
Highlight box
Key findings
• This study constructed a novel model that can predict the prognosis of gastric cancer patients.
What is known and what is new?
• The known information includes the clinical information of gastric cancer patients and the expression level of lncRNA.
• To explore the relationship between the model of lncRNA pairs and the prognosis of gastric cancer patients.
What is the implication, and what should change now?
• The novel model provides a new tool for predicting the survival of patients with gastric cancer and it can be applied to clinical practice.
Introduction
Gastric cancer (GC) is an important health concern globally; it is the fifth most commonly diagnosed tumor and the fourth main reason for cancer-involved deaths, accounting for 769,000 deaths worldwide in 2020 (1). GC is a heterogeneous disease characterized by differences in epidemiology and histopathology across countries (2). In addition to the endoscopic treatment of a few very small tumors, partial or total gastrectomy with lymph node dissection is the most effective treatment for GC (3). However, because most early-stage GCs have no symptoms, patients are usually not diagnosed until the advanced stage of the disease (4). Thus, there is an urgent need to explore a novel prognostic assessment model for GC.
Long noncoding RNAs (lncRNAs) are considered to be a kind of RNA molecule with length greater than 200 nucleotides, lacking the ability to code proteins (5). Compared with protein-coding genes, lncRNAs consist of fewer exons and are in a relatively low abundance (6). LncRNAs critically function in cell differentiation, apoptosis, transcriptional regulation, and tumor microenvironment (TME) (7,8). At present, the literature has used lncRNAs to build models to predict the prognosis of GC patients (9). Besides, some studies associated with cancer has reported lncRNAs play a significant role in regulating epithelial-mesenchymal transition (EMT). For instance, lncRNA SNHG6 could regulate ZEB1 through sponging miR-101-3p to induce EMT in colorectal cancer (10). In thyroid cancer, lncRNA TUG1 can promote the formation of EMT (11). Other research reveals that ANCR modulates EMT inducer’s stability to block tumor growth and metastasis (12). Therefore, developing an EMT-related lncRNA evaluation system has an important clinical significance.
Compared with the cancer diagnostic model constructed by a single gene, the model constructed by a combination of two biomarkers has better accuracy (13). In this study, paired differentially expressed lncRNAs that were significantly related to prognosis were selected. Based on these lncRNA pairs, a prognostic model was established and its predictive ability in GC was verified. We present this article in accordance with the TRIPOD reporting checklist (available at https://tcr.amegroups.com/article/view/10.21037/tcr-22-2751/rc).
Methods
Data acquisition
The RNA-sequencing profiles together with corresponding clinical data for GC patients from The Cancer Genome Atlas (TCGA) dataset (https://portal.gdc.cancer.gov/) were obtained and used as the training set. Patients without clinical data were excluded, and 371 GC samples were defined finally for analysis. GSE62254, which contained 300 patients with GC and their clinicopathological data, was extracted from Gene Expression Omnibus (https://www.ncbi.nlm.nih.gov/geo/) as the testing set. A list of 1,184 EMT-related genes was downloaded by accessing the dbEMT2 website (http://dbemt.bioinfo-minzhao.org/download.cgi) (14). The study was conducted in accordance with the Declaration of Helsinki (as revised in 2013).
Pairing differentially expressed lncRNAs
The co-expression strategy was adopted between lncRNAs and EMT-related genes and the lncRNAs with correlation coefficient >0.4 and P value <0.001 were considered statistically significant. In order to explore differentially expressed lncRNAs, the “limma” R package was utilized and the screening criteria was set as P value <0.05 along with |log2[fold change (FC)]|>1. Then, every differentially expressed lncRNA was paired with each other and a specific score for each lncRNA pair was calculated. In the pairwise comparison, the output was 1 if the expression quantity of the first EMT-related lncRNA was greater than the following one in a specific lncRNA pair; otherwise, the output was 0. And some lncRNA pairs, in which the proportion of 0 or 1 was less than 20%, were deleted.
Construction of the lncRNA pairs model
Univariate Cox analysis was employed to ascertain the potential prognostic associated lncRNA pairs in the training set. And the “glmnet” R package was also used to conduct the least absolute shrinkage and selection operator (LASSO) regression analysis after 1,000 iterations with 10-fold cross validation. Ultimately, 20 lncRNA pairs were identified to establish the prognostic model, and each GC patient’s risk score was generated based on the following formula: risk score = (ExprlncRNApair-1 × CoeflncRNApair-1) + (ExprlncRNApair-2 × CoeflncRNApair-2) + … + (ExprlncRNApair-n × CoeflncRNApair-n). Subsequently, the model’s receiver operating characteristic (ROC) curves of the training cohort in different years were drawn, and the optimal cutoff at the five-year ROC curve was selected to separate GC patients into different risk levels.
Validation of the prognostic model
In order to validate the established model, the above formula was applied to GC patients in the testing set and assigned them into high- or low-risk subgroups by using the same cutoff point obtained from the training set. Meanwhile, Kaplan-Meier analysis was employed to assess the survival differences between patients in the two risk subgroups in both the TCGA cohort and the GSE62254 cohort. And time-dependent ROC curves of one, three, and five years for the testing cohort were used to detect the model’s predictive power. Moreover, to better clarify if the model had a good prognostic efficiency, univariate and multivariate Cox proportional-hazards analyses were used.
Correlation between the model and clinical characteristics
The relations between risk score and clinical characteristics, such as age, gender, grade, stage, T stage, N stage, M stage, were analyzed. The “survivalROC” R package was performed to confirm this model’s efficiency. The results of box plots showed the differences in risk scores among different clinical groups. The clinical factors were also stratified to calculate for a broader utility of risk score in GC patients.
Investigation of immune infiltration
A file about different immune infiltrating cells for GC samples in the TCGA database was downloaded from the TIMER2.0 website (http://timer.cistrome.org/) to study the correlation between infiltration immune cell subtypes and different risk subgroups (15). And the datasets of immune infiltration included XCELL, TIMER, QUANTISEQ, MCPCOUNTER, EPIC, CIBERSORT-ABS, and CIBERSORT. The results of spearman correlation analysis were indicated in the bubble chart.
Functional enrichment analysis
To investigate the biological mechanisms involved in this risk model, the gene set enrichment analysis was implemented by using the package “fgsea” with 10,000 permutations. The datasets related to Gene Ontology (GO) and Kyoto Encyclopedia of Genes and Genomes (KEGG) were obtained from the Molecular Signatures Database (http://www.gsea-msigdb.org/gsea/msigdb/index.jsp) (16) and they were compared in high- vs. low-risk groups. These enriched gene sets were selected with a threshold of false discovery rate (FDR)-adjusted P<0.05.
Statistical analysis
The R software (version 4.0.5) was used to complete all data analyses and the correlations of the risk score with clinical variables were investigated via a chi-square test. The survival data analyses were carried out by Kaplan-Meier survival curves with log-rank test. And all graphs were drawn using R language. P value <0.05 was defined to be a statistically significant difference.
Results
Screening of EMT-related lncRNAs in GC
A total of 371 GC patients were chosen from the TCGA cohort as the training set, and GSE62254 with 300 GC samples became the testing set. The distribution of specific clinical features in these two sets is shown in Table S1. According to the obtained genes from the dbEMT2 website, the method of co-expression analysis was utilized to identify EMT-related lncRNAs. And following the standards of |log2FC| >1 and FDR <0.05, 434 up-regulated as well as 36 down-regulated differentially expressed lncRNAs were selected. A volcano map of all lncRNAs is shown in Figure S1. Red and green dots indicate the up- and down-regulated lncRNAs in GC, and black dots indicate lncRNAs with nonsignificant differences.
Construction of a model consisting of 20 lncRNA pairs
The screened lncRNA was paired with each other and the lncRNA pairs were excluded if the score of which were 0 or 1 in less than 20% of the samples. Univariate Cox analysis was performed on these constructed lncRNA pairs, and 35 lncRNA pairs remained. Next, LASSO regression was employed to remove the overfitting in the training set (Figure 1). After 1,000 iterations, a prognostic model composed of 20 lncRNA pairs was established (Table S2). Then, the ROC curves were plotted for one, three, and five years, and the corresponding areas under the ROC curves (AUC) values were all greater than 0.7, suggesting the model had a robust performance in survival prediction. Subsequently, the maximum inflection point at the five-year ROC curve was calculated to be 0.299 and set as the optimal cutoff value for classifying all samples into either the high- or low-risk groups (Figure 2).
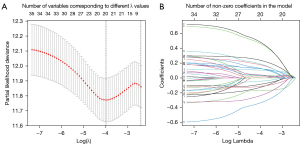
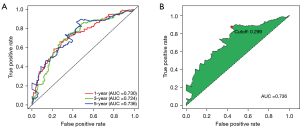
Assessment and validation of the model
All patients’ risk scores were counted, and the distribution results of low- and high-risk samples in the TCGA cohort and GSE62254 are shown in Figure 3A,3B. The scatter plots showed that the rates of death increased gradually as the improvement of the risk score in the patients with GC. Kaplan-Meier analyses were also employed and suggested that the overall survival (OS) of the group with low-risk was better than that of the group with high-risk (Figure 3C,3D). In the testing set, the AUCs at one, three, and five years were 0.642, 0.631, and 0.644, respectively, displaying the good prognostic capability of the risk model (Figure 3E). Furthermore, univariate and multivariate Cox analyses were conducted in these two sets and revealed risk score had an independent prognostic role in GC patients (Figure 4A-4D). Therefore, this model based on the 20 lncRNA pairs was closely associated with the prognosis.
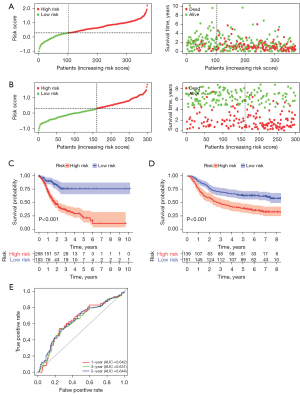
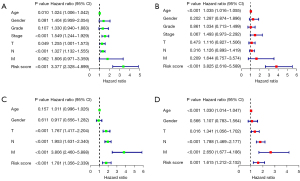
Association between clinical features and the risk score
The five-year ROC curve was compared with other traditional clinicopathological parameters (Figure 5A). The results demonstrated apparently that the predictive power of risk score was superior to the common clinical features. The relationships between the risk score and clinical characteristics were also evaluated (Figure 5B). The risk score was found to be relevant to grade, stage, and T stage, but there was no significant correlation with other clinical characteristics. Moreover, to observe whether the prognostic model was suitable for different populations, further survival analyses of stratified clinical features were performed. The survival curves showed low-risk patients presented a greater prognosis than the high-risk in all clinical features except stage I, stage II, T1, and T2 (Figure 6).
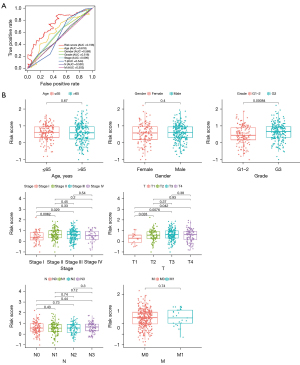
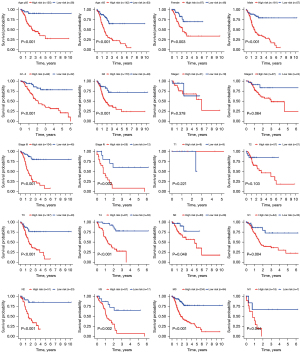
Immune cell infiltration and functional analysis
Tumor-infiltrating immune cells participate in the process of the occurrence, development, and prognosis of cancer. A file of tumor-infiltrating immune cells from the TIMER2.0 website was obtained and a bubble chart was developed after the analysis (Figure 7). If the correlation coefficient corresponding to the dot was positive, then the immune cell was positively related to the risk score of patients; otherwise, it was a negative correlation. In addition, functional enrichment analyses of GO and KEGG pathway were implemented to investigate the biological effects of the constructed lncRNA pairs model. GO analysis indicated the lncRNAs in the prognostic model were mostly correlated with digestion (Figure 8A); KEGG analysis revealed the most significant enrichment pathways involved in these lncRNAs (Figure 8B).
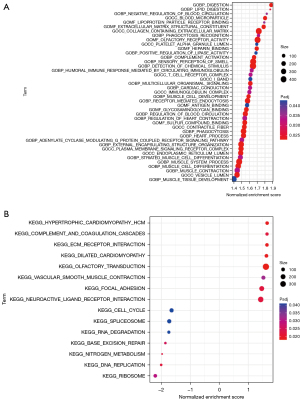
Discussion
GC is a very prevalent gastrointestinal malignant tumor, which has the features of high morbidity and mortality. Although the treatment methods of GC are developing continuously, the prognosis of advanced patients is still poor (17). With the widespread use of microarray sequencing technology, more and more biomarkers are found to be able to utilize to predict the prognosis of tumors. LncRNAs, as important regulators of multiple processes of gene expression, participate in the proliferation, invasion, and metastasis of various cancers (18,19). At present, many studies have reported the predictive model constructed by lncRNAs to identify the prognosis of cancer patients, such as bladder cancer, hepatocellular carcinoma, colorectal cancer (20-22). EMT is related to tumor progression and promotes the metastasis of cancer cells by enhancing their migration and invasion. The disorder of lncRNAs plays a crucial role in tumor metastasis, which is conducive to the occurrence of EMT and can mediate EMT-induced metastasis using multiple mechanisms. For example, lncRNAs participate in gene transcription by binding with EMT-induced transcription factors (TFs), and the interaction of lncRNAs and TFs directly regulates gene transcription by phosphorylation and ubiquitination inducing targeted protein degradation (23,24). In terms of GC, lncRNAs mediate a variety of signaling pathways, including Wnt, PI3K/AKT, Hippo, MEK/ERK and Notch1, regulating the process of EMT in GC. And lncRNAs regulate EMT-induced GC metastasis through transcription factors and sponging miRNAs (25). In addition, EMT-related lncRNAs can be used as biomarkers to judge the prognosis, treatment, and immune infiltration of tumor patients. And the dysregulation of lncRNAs expression value is closely related to the poor prognosis of GC (26). However, there are relatively few studies using lncRNA biomarkers to construct GC prognosis models.
In this research, a prognostic prediction model was constructed on the basis of the EMT-related lncRNA pairs. Different from previous prediction models, we paired the selected lncRNAs and identified the risk score in patients with GC through relative expression values of the two lncRNAs. Using this method, there was no need for batch normalization. First, we downloaded RNA-seq data of TCGA-Stomach Adenocarcinoma (TCGA-STAD) cohort, acquired EMT-related lncRNA expression profiles for GC patients, and paired differentially expressed lncRNAs. Second, adopting univariate integrated with LASSO Cox regression analyses, a risk model was established consisting of 20 survival-related lncRNA pairs. Then, each GC sample’s corresponding risk score was determined, and these samples were classified into two subgroups according to the cutoff value. Kaplan-Meier plots of OS manifested the patients with lower risk scores had a longer survival time than those with higher risk scores, and the results of ROC curves verified the excellent predictive capacity of the model. We also assessed this risk model from the perspectives of clinicopathologic features, immune cell infiltration, and biological functions. Furthermore, the analysis of GSE62254 verified the model had an excellent predictive capacity.
The role of lncRNAs in malignant tumors has become a research hotspot. Some recent researches have revealed that lncRNAs are connected with the prognosis of GC patients. Ma et al. downloaded lncRNA expression profiles in GSE62254 and constructed a six-lncRNA signature in order to evaluate GC patients’ prognosis independently (27). Chen et al. identified ten hypoxia-related lncRNAs and obtained a prognostic signature and nomogram to predict both the OS and disease-free survival in GC (28). Several lncRNAs included in our model have been identified to have an essential effect on various tumor types including GC. For example, Liu et al. reported over-expressed HOXA11-AS boosts GC cell proliferation, cell cycle progression and metastasis, proposing its exertion on oncogenic effects in GC (29). Wei et al. revealed that HOTAIR accelerates proliferation and metastasis in GC cell lines by sponging miR-1277-5p and upregulating COL5A1 (30). And HOTTIP exists in exosomes of GC patients and may be a good prognostic biomarker for GC (31). Besides, EMT, as an important biological process, is induced by the TME and is closely correlated with the malignant progression of all types of cancer (32). Consequently, the model established here has the potential to provide a novel method to predict the prognosis of GC.
TME cells are important constituents of the tumor tissue. The study has shown that there is some interaction between infiltrating TME cells and the clinical features of GC (33). Considering the importance of immune cell infiltration in GC, seven recognized reliable methods were utilized to explore the correlation between infiltrated immune cells and risk scores, including XCELL, TIMER, QUANTISEQ, MCPCOUNTER, EPIC, CIBERSORT-ABS, and CIBERSORT (34-40). Through the result reorganization and analysis, the lncRNA pairs for building the model was found to have a positive relation to the immune infiltration of macrophages, monocytes, myeloid dendritic cells, and neutrophils. These innate immune cells and adaptive immune cells were part of the TME and were associated with tumor progression (41). Given that all lncRNAs in the model were associated with EMT, the relationship between EMT and immune cells was also explored. Evidence has shown that immune cells could modulate the process of EMT by producing multiple EMT inducers and mediators (42). Tumor-associated macrophages (TAMs) derived from inflammatory monocytes are functionally effective EMT inducers and can produce a variety of growth factors and inflammatory cytokines to cause EMT of cancer cells (43). Neutrophils can also regulate EMT like TAMs. Li et al. reported that tumor-associated neutrophils promoted the migration and invasion of GC cells by inducing EMT through IL-17a (44). In addition, inflammatory cytokines generated by all immunocytes could affect EMT through indirect regulation (45). The infiltration level of specific immune cells is significantly correlated with the pathological features and clinical results of GC. Immunotherapy is an innovative treatment for GC. Cancer cells can evade immune monitoring by up-regulating programmed cell death ligand 1 (PD-L1) and other immune checkpoint proteins (46). Tumor mutational burden (TMB) is a novel biomarker for PD-L1 antibody therapy in a variety of tumors. The research has shown that OS in patients with chemotherapy-refractory GC treated with toripalimab is significantly better in the high TMB group than in the low TMB group (47). The results of gene set enrichment analysis (GSEA) revealed the model is involved in activities related to digestion. Moreover, a study has used bioinformatics and experimental methods to construct mRNA-miRNA-lncRNA networks, and explored the possible role of central genes in promoting or suppressing GC by constructing an axis, which is helpful to identify new targets for the treatment of GC (48).
This study constructed a prognostic model of GC by relative ranking and paired comparison of EMT-related lncRNAs expression values, which did not take into account of the impact of the batch effects of the different platforms. And the findings here suggested that a GC patient with a higher model risk score may have a shorter survival time and a worse prognostic outcome. However, it should be admitted that there are still some shortcomings in our research. Firstly, the variables contained in the TCGA and GSE62254 databases were not comparable, and important indicators such as the history of past illness, chemotherapy, and radiotherapy were not indicated, which may influence the treatment and prognosis of GC patients. Secondly, in vivo or in vitro experimental researches are crucial to further confirm the prognostic performance of the constructed model for GC. Finally, given the relationship between lncRNAs and the immune system, more biological experiments on immunity are worth studying. Besides, the research results of The Cancer Genome Atlas (TCGA) project proposed a molecular classification method to divide GC into four genomic subtypes, including Epstein-Barr virus (EBV)-infected tumors, microsatellite unstable tumors, genomically stable tumors, and chromosomally unstable tumors (49). This method is easier to be applied to the clinical nursing of patients with GC. Therefore, the study on the prognosis of the four molecular types of GC can serve as an in-depth direction for investigation in future research. Most studies on lncRNA are limited to bioinformatics analysis and cell experiments, and there are still many problems and challenges in the clinical application of lncRNA. For example, large-scale animal and clinical models are needed to define the regulatory role of lncRNAs. Further research is needed to determine whether lncRNAs and other RNAs may form competitive endogenous RNA networks, thus affecting the function of the body (50). In addition, there are still some difficulties in the targeted delivery and therapeutic efficacy evaluation of lncRNAs.
Conclusions
Taken together, the novel proposed model constructed by EMT-related lncRNA pairs is a promising method to predict the prognosis of GC patients, which could be beneficial for improving the survival outcomes of patients. This research may offer new insights for clinicians in clinical decision-making and individualized treatment.
Acknowledgments
We are grateful to the TCGA and GEO for providing all the data.
Funding: None.
Footnote
Reporting Checklist: The authors have completed the TRIPOD reporting checklist. Available at https://tcr.amegroups.com/article/view/10.21037/tcr-22-2751/rc
Conflicts of Interest: All authors have completed the ICMJE uniform disclosure form (available at https://tcr.amegroups.com/article/view/10.21037/tcr-22-2751/coif). The authors have no conflicts of interest to declare.
Ethical Statement: The authors are accountable for all aspects of the work in ensuring that questions related to the accuracy or integrity of any part of the work are appropriately investigated and resolved. The study was conducted in accordance with the Declaration of Helsinki (as revised in 2013).
Open Access Statement: This is an Open Access article distributed in accordance with the Creative Commons Attribution-NonCommercial-NoDerivs 4.0 International License (CC BY-NC-ND 4.0), which permits the non-commercial replication and distribution of the article with the strict proviso that no changes or edits are made and the original work is properly cited (including links to both the formal publication through the relevant DOI and the license). See: https://creativecommons.org/licenses/by-nc-nd/4.0/.
References
- Sung H, Ferlay J, Siegel RL, et al. Global Cancer Statistics 2020: GLOBOCAN Estimates of Incidence and Mortality Worldwide for 36 Cancers in 185 Countries. CA Cancer J Clin 2021;71:209-49. [Crossref] [PubMed]
- Chia NY, Tan P. Molecular classification of gastric cancer. Ann Oncol 2016;27:763-9. [Crossref] [PubMed]
- Wagner AD, Syn NL, Moehler M, et al. Chemotherapy for advanced gastric cancer. Cochrane Database Syst Rev 2017;8:CD004064. [PubMed]
- Van Cutsem E, Sagaert X, Topal B, et al. Gastric cancer. Lancet 2016;388:2654-64. [Crossref] [PubMed]
- Gutschner T, Diederichs S. The hallmarks of cancer: a long non-coding RNA point of view. RNA Biol 2012;9:703-19. [Crossref] [PubMed]
- Yan X, Hu Z, Feng Y, et al. Comprehensive Genomic Characterization of Long Non-coding RNAs across Human Cancers. Cancer Cell 2015;28:529-40. [Crossref] [PubMed]
- Jain S, Thakkar N, Chhatai J, et al. Long non-coding RNA: Functional agent for disease traits. RNA Biol 2017;14:522-35. [Crossref] [PubMed]
- Lin YH, Wu MH, Yeh CT, et al. Long Non-Coding RNAs as Mediators of Tumor Microenvironment and Liver Cancer Cell Communication. Int J Mol Sci 2018;19:3742. [Crossref] [PubMed]
- Sun J, Jiang Q, Chen H, et al. Genomic instability-associated lncRNA signature predicts prognosis and distinct immune landscape in gastric cancer. Ann Transl Med 2021;9:1326. [Crossref] [PubMed]
- Wang X, Lai Q, He J, et al. LncRNA SNHG6 promotes proliferation, invasion and migration in colorectal cancer cells by activating TGF-β/Smad signaling pathway via targeting UPF1 and inducing EMT via regulation of ZEB1. Int J Med Sci 2019;16:51-9. [Crossref] [PubMed]
- Lei H, Gao Y, Xu X. LncRNA TUG1 influences papillary thyroid cancer cell proliferation, migration and EMT formation through targeting miR-145. Acta Biochim Biophys Sin (Shanghai) 2017;49:588-97. [Crossref] [PubMed]
- Li Z, Hou P, Fan D, et al. The degradation of EZH2 mediated by lncRNA ANCR attenuated the invasion and metastasis of breast cancer. Cell Death Differ 2017;24:59-71. [Crossref] [PubMed]
- Hong W, Liang L, Gu Y, et al. Immune-Related lncRNA to Construct Novel Signature and Predict the Immune Landscape of Human Hepatocellular Carcinoma. Mol Ther Nucleic Acids 2020;22:937-47. [Crossref] [PubMed]
- Zhao M, Liu Y, Zheng C, et al. dbEMT 2.0: An updated database for epithelial-mesenchymal transition genes with experimentally verified information and precalculated regulation information for cancer metastasis. J Genet Genomics 2019;46:595-7. [Crossref] [PubMed]
- Li T, Fu J, Zeng Z, et al. TIMER2.0 for analysis of tumor-infiltrating immune cells. Nucleic Acids Res 2020;48:W509-14. [Crossref] [PubMed]
- Liberzon A, Birger C, Thorvaldsdóttir H, et al. The Molecular Signatures Database (MSigDB) hallmark gene set collection. Cell Syst 2015;1:417-25. [Crossref] [PubMed]
- Machlowska J, Baj J, Sitarz M, et al. Gastric Cancer: Epidemiology, Risk Factors, Classification, Genomic Characteristics and Treatment Strategies. Int J Mol Sci 2020;21:4012. [Crossref] [PubMed]
- Charles Richard JL, Eichhorn PJA. Platforms for Investigating LncRNA Functions. SLAS Technol 2018;23:493-506. [Crossref] [PubMed]
- Bhan A, Soleimani M, Mandal SS. Long Noncoding RNA and Cancer: A New Paradigm. Cancer Res 2017;77:3965-81. [Crossref] [PubMed]
- Tong H, Li T, Gao S, et al. An epithelial-mesenchymal transition-related long noncoding RNA signature correlates with the prognosis and progression in patients with bladder cancer. Biosci Rep 2021;41:BSR20203944. [Crossref] [PubMed]
- Li F, Bai L, Li S, et al. Construction and evaluation of a prognosis lncRNA model for hepatocellular carcinoma. J Cell Biochem 2020; Epub ahead of print. [Crossref] [PubMed]
- Liu Y, Liu B, Jin G, et al. An Integrated Three-Long Non-coding RNA Signature Predicts Prognosis in Colorectal Cancer Patients. Front Oncol 2019;9:1269. [Crossref] [PubMed]
- Long Y, Wang X, Youmans DT, et al. How do lncRNAs regulate transcription? Sci Adv 2017;3:eaao2110. [Crossref] [PubMed]
- Lamouille S, Xu J, Derynck R. Molecular mechanisms of epithelial-mesenchymal transition. Nat Rev Mol Cell Biol 2014;15:178-96. [Crossref] [PubMed]
- Feng YN, Li BY, Wang K, et al. Epithelial-mesenchymal transition-related long noncoding RNAs in gastric carcinoma. Front Mol Biosci 2022;9:977280. [Crossref] [PubMed]
- Bure IV, Nemtsova MV, Zaletaev DV. Roles of E-cadherin and Noncoding RNAs in the Epithelial-mesenchymal Transition and Progression in Gastric Cancer. Int J Mol Sci 2019;20:2870. [Crossref] [PubMed]
- Ma B, Li Y, Ren Y. Identification of a 6-lncRNA prognostic signature based on microarray re-annotation in gastric cancer. Cancer Med 2020;9:335-49. [Crossref] [PubMed]
- Chen Q, Hu L, Chen K. Construction of a Nomogram Based on a Hypoxia-Related lncRNA Signature to Improve the Prediction of Gastric Cancer Prognosis. Front Genet 2020;11:570325. [Crossref] [PubMed]
- Liu Z, Chen Z, Fan R, et al. Over-expressed long noncoding RNA HOXA11-AS promotes cell cycle progression and metastasis in gastric cancer. Mol Cancer 2017;16:82. [Crossref] [PubMed]
- Wei Z, Chen L, Meng L, et al. LncRNA HOTAIR promotes the growth and metastasis of gastric cancer by sponging miR-1277-5p and upregulating COL5A1. Gastric Cancer 2020;23:1018-32. [Crossref] [PubMed]
- Zhao R, Zhang Y, Zhang X, et al. Exosomal long noncoding RNA HOTTIP as potential novel diagnostic and prognostic biomarker test for gastric cancer. Mol Cancer 2018;17:68. [Crossref] [PubMed]
- Dongre A, Weinberg RA. New insights into the mechanisms of epithelial-mesenchymal transition and implications for cancer. Nat Rev Mol Cell Biol 2019;20:69-84. [Crossref] [PubMed]
- Zeng D, Li M, Zhou R, et al. Tumor Microenvironment Characterization in Gastric Cancer Identifies Prognostic and Immunotherapeutically Relevant Gene Signatures. Cancer Immunol Res 2019;7:737-50. [Crossref] [PubMed]
- Aran D, Hu Z, Butte AJ. xCell: digitally portraying the tissue cellular heterogeneity landscape. Genome Biol 2017;18:220. [Crossref] [PubMed]
- Li T, Fan J, Wang B, et al. TIMER: A Web Server for Comprehensive Analysis of Tumor-Infiltrating Immune Cells. Cancer Res 2017;77:e108-10. [Crossref] [PubMed]
- Plattner C, Finotello F, Rieder D. Deconvoluting tumor-infiltrating immune cells from RNA-seq data using quanTIseq. Methods Enzymol 2020;636:261-85. [Crossref] [PubMed]
- Dienstmann R, Villacampa G, Sveen A, et al. Relative contribution of clinicopathological variables, genomic markers, transcriptomic subtyping and microenvironment features for outcome prediction in stage II/III colorectal cancer. Ann Oncol 2019;30:1622-9. [Crossref] [PubMed]
- Racle J, de Jonge K, Baumgaertner P, et al. Simultaneous enumeration of cancer and immune cell types from bulk tumor gene expression data. Elife 2017;6:e26476. [Crossref] [PubMed]
- Tamminga M, Hiltermann TJN, Schuuring E, et al. Immune microenvironment composition in non-small cell lung cancer and its association with survival. Clin Transl Immunology 2020;9:e1142. [Crossref] [PubMed]
- Chen B, Khodadoust MS, Liu CL, et al. Profiling Tumor Infiltrating Immune Cells with CIBERSORT. Methods Mol Biol 2018;1711:243-59. [Crossref] [PubMed]
- Hinshaw DC, Shevde LA. The Tumor Microenvironment Innately Modulates Cancer Progression. Cancer Res 2019;79:4557-66. [Crossref] [PubMed]
- Chou MY, Yang MH. Interplay of Immunometabolism and Epithelial-Mesenchymal Transition in the Tumor Microenvironment. Int J Mol Sci 2021;22:9878. [Crossref] [PubMed]
- Suarez-Carmona M, Lesage J, Cataldo D, et al. EMT and inflammation: inseparable actors of cancer progression. Mol Oncol 2017;11:805-23. [Crossref] [PubMed]
- Li S, Cong X, Gao H, et al. Tumor-associated neutrophils induce EMT by IL-17a to promote migration and invasion in gastric cancer cells. J Exp Clin Cancer Res 2019;38:6. [Crossref] [PubMed]
- Chockley PJ, Keshamouni VG. Immunological Consequences of Epithelial-Mesenchymal Transition in Tumor Progression. J Immunol 2016;197:691-8. [Crossref] [PubMed]
- Budczies J, Allgäuer M, Litchfield K, et al. Optimizing panel-based tumor mutational burden (TMB) measurement. Ann Oncol 2019;30:1496-506. [Crossref] [PubMed]
- Kim J, Kim B, Kang SY, et al. Tumor Mutational Burden Determined by Panel Sequencing Predicts Survival After Immunotherapy in Patients With Advanced Gastric Cancer. Front Oncol 2020;10:314. [Crossref] [PubMed]
- Tang S, Liao K, Shi Y, et al. Bioinformatics analysis of potential Key lncRNA-miRNA-mRNA molecules as prognostic markers and important ceRNA axes in gastric cancer. Am J Cancer Res 2022;12:2397-418. [PubMed]
- Comprehensive molecular characterization of gastric adenocarcinoma. Nature 2014;513:202-9. [Crossref] [PubMed]
- Ren YZ, Ding SS, Jiang YP, et al. Application of exosome-derived noncoding RNAs in bone regeneration: Opportunities and challenges. World J Stem Cells 2022;14:473-89. [Crossref] [PubMed]