Identification of immune-related genes and patient selection for hepatocellular carcinoma immunotherapy
Highlight box
Key findings
• HSP90AA1, CDK4, HSPA8, HSPH1, and HSPA5 were identified as promising biomarkers for prognosis prediction and immunotherapy of HCC. Based on the coexpression module containing these 5 genes, patients with specific characteristics were selected as suitable candidates for tumor immunotherapy.
What is known, and what is new?
• Tumor immunotherapy is an emerging hotspot for the treatment of HCC; however, this approach has a few issues, including the lack of immune-related biomarkers and the difficulty in selecting a patient population suitable for immunotherapy.
• This study identified 5 immune-related biomarkers and classified patients with HCC into 3 groups, each with unique clinical, mutational, immunological, and biological process characteristics.
What is the implication, and what should change now?
• Our study provides new target genes and an appropriate patient population for HCC immunotherapy. Further experimental and clinical validations of these findings are needed.
Introduction
Hepatocellular carcinoma (HCC) is the third leading cause of cancer-related death and results in more than 500,000 deaths worldwide each year (1). Most patients with HCC are at the advanced stage of the disease when diagnosed; thus, they do not have the opportunity to undergo surgery and have a poor prognosis. The 5-year overall survival (OS) rate of HCC is only 10–18% (2). The main treatment methods for HCC include surgical resection, liver transplantation, transcatheter arterial chemoembolization, and radiofrequency ablation, which can be supplemented by systemic chemotherapy and targeted drug therapy (3-6). However, alternative treatment methods for patients with unresectable middle and advanced HCC are limited, and new treatment strategies are urgently needed (7-9).
In recent years, extensive clinical trials have been conducted to assess the potential of tumor immunotherapy (TIT), and promising preliminary results have been reported (10,11). This has led to the investigation of TIT as a treatment for advanced HCC becoming a research hotspot. The aim of TIT is to activate the immune system of an organism to kill tumor cells (12,13). Several immunotherapy strategies exist for HCC, such as immune checkpoint inhibitors (ICIs), adoptive cell therapy, tumor vaccines, and cytokine therapy, among which ICIs [anti–programmed cell death protein 1 (PD1), anti–programmed death-ligand 1 (PDL1), and anti–cytotoxic T-lymphocyte-associated (CTLA4) antibodies] have been proven to be an effective and successful TIT method (14,15). With the development of TIT, great progress has been made in the treatment and prognosis of patients with HCC (16,17). However, due to the problems of drug resistance, side effects, uncertain efficacy, and patient heterogeneity in TIT (18), the identification of novel immune-related biomarkers and the selection of a suitable patient population are urgent issues to be solved in HCC immunotherapy.
Therefore, the purpose of this study was to identify new immune-related genes for the therapy and prognosis prediction of HCC and clusters of patients with HCC at the molecular level, which will enable the selection of appropriate patients for TIT. First, we identified genes that were overexpressed in HCC tissue and confirmed the results using global high-throughput datasets that included 7,384 samples (3,941 HCC vs. 3,443 non-HCC tissues) from 84 central datasets, as well as 39 platforms. Next, genes associated with immune response pathways and expressed by a HCC subpopulation with the most differentiation potential were identified. Finally, we defined 5 genes responsible for poor prognosis and strong immune infiltration in patients with HCC as potential immune-related prognostic biomarkers. Based on the coexpression module of these 5 genes in HCC tissue samples, we defined 3 clusters of patients with HCC. Each cluster contained different clinical, mutation, immune, and biological process characteristics. Thus, our findings provide a theoretical basis for the identification of immune-related genes with strong prognostic value and the selection of appropriate patients for TIT. We present this article in accordance with the Reporting Recommendations for Tumor Marker Prognostic Studies (REMARK ) reporting checklist (available at https://tcr.amegroups.com/article/view/10.21037/tcr-22-2304/rc).
Methods
The study was conducted in accordance with the Declaration of Helsinki (as revised in 2013). The graphical abstract of our study is shown in Figure 1.
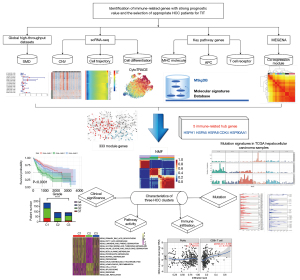
Evaluation of significantly upregulated genes in HCC tissues associated with immune response pathways among global high-throughput datasets
First, genes significantly upregulated in HCC tissues were collected via integrated analysis. The key term “hepatocellular carcinoma” was used for data retrieval in the public databases of The Cancer Genome Atlas (TCGA; https://www.cancer.gov/tcga), Gene Expression Omnibus (GEO; https://www.ncbi.nlm.nih.gov/geo/), ArrayExpress (https://www.ebi.ac.uk/arrayexpress/), Sequence Read Archive (SRA; https://trace.ncbi.nlm.nih.gov/Traces/sra/), Oncomine (https://www.oncomine.org/resource/login.html), the Cancer Cell Line Encyclopedia (CCLE; https://sites.broadinstitute.org/ccle), the International Cancer Genome Consortium (ICGC; https://dcc.icgc.org/), Genomic Expression Archive (GEA; https://www.ddbj.nig.ac.jp/gea/index-e.html), and PubMed. The inclusion criteria were as follows: messenger RNA (mRNA) expression in HCC and adjacent tissues; Homo sapiens tissue sample collected; no adjuvant treatment, such as chemotherapy or radiotherapy, for patients with HCC; and HCC parenchymal tissue but not interstitial cells or interstitial tissue. When qualified throughput data were obtained, the data were normalized with log2, and the means and standard deviations (SDs) of the genes were calculated. The standardized mean difference (SMD) of the high-throughput datasets was counted using Stata 14.0 (StataCorp). When obvious heterogeneity (I2>50%) was observed, a random effects model was selected. After exclusion of heterogeneous sources, the analysis was integrated, and Begg test was conducted to detect publication bias. The discrimination ability of genes for HCC was determined by calculating the sensitivity and specificity and using a summarized receiver operating characteristic curve (sROC). Furthermore, true positive (TP), false positive (FP), false negative (FN), and true negative (TN) were assigned to summarize the diagnostic analysis results and calculate sensitivity, specificity, negative likelihood ratio (NLR), and positive likelihood ratio (PLR). P values <0.05 were considered statistically significant.
Antigen presentation depends on the following factors: the mutant sequence can be translated into protein, the mutant protein can be processed into peptides and recognized by antigen-presenting cells (APCs), the mutant peptides have high affinity with the major histocompatibility complex (MHC) molecules of patients, and the mutant peptide–MHC complex can be identified with T-cell receptors. Therefore, the genes of the following 13 gene sets were extracted from the molecular signatures database (MSIGDB; https://www.gsea-msigdb.org/gsea/msigdb), which corresponded to the screening conditions for the candidate genes: antigen processing and presentation, T-cell receptor signaling pathway, peptide antigen assembly with MHC class II protein complex, peptide antigen assembly with MHC protein complex, positive regulation of MHC class I biosynthetic process, positive regulation of MHC class II biosynthetic process, MHC class I peptide loading complex, MHC class I protein complex, MHC class II protein complex, MHC protein complex, MHC class I receptor activity, MHC class IB receptor activity, and MHC class II receptor activity.
Use of single-cell RNA sequencing analysis to identify significant differentiation-driving genes in HCC cells
Recent studies have shown that the cell state changes during differentiation, producing cell types with stable phenotypes and immature bodies that change between phenotypes. This series of changes is mainly controlled by the gene expression in the cells (19,20). Similarly, during tumor cell development, the accumulation of early mutations leads to the production of carcinogenic metabolites, which increases intracellular genetic instability and genomic hypermethylation and eventually induces a malignant phenotype (21). Additionally, high differentiation potential is one of the characteristics of cancer stem cells (CSCs), and many studies have shown that the presence of CSCs in tumor tissues is closely related to poor prognosis (22,23). Therefore, specific differentiation-driving molecules active in the early stage of HCC cell development were explored via single-cell RNA sequencing (scRNA-seq) analysis.
The GSE112271 (24) dataset of the GEO contains 7 regions extracted from tumor tissues of 2 patients with HCC (quality control threshold: minimum cells =3, minimum features =200, percentage of mitochondrial genes <10%). The canonical correlation analysis (CCA) function in the “Seurat” package in R software (The R Foundation for Statistical Computing) was employed to correct the batch effect. Then, 2,000 genes with a large coefficient of variation between different cells were selected for follow-up analysis. The subpopulations of cells were obtained via linear dimensionality reduction principal component analysis (PCA) and t-distribution stochastic neighbor embedding (t-SNE) clustering. By taking the gene expression matrix as the input, copy number karyotype of tumors (CopyKAT) (25) was used to calculate the genomic copy number distribution of single cells through combining the Bayesian method and hierarchical clustering and to classify tumor and normal cells according to the aneuploid copy number. Monocle (26) package in R software was then applied to construct a cell development trajectory, and independent component analysis (ICA) was used to project all cells in low-dimensional space. Next, a minimum spanning tree (MST) was constructed to connect the main cell points and form a 1-dimensional ranking of all cells. This dimension, called pseudotime, was used to represent the predicted lineage trajectory of sample cells. Since the pseudotime of the cells in Monocle does not include sequencing, Cellular Trajectory Reconstruction Analysis using gene Counts and Expression (CytoTRACE) (27) package in R software was applied to determine which cells had the most differentiation potential. This provided a starting point for MST by regarding the number of genes expressed per cell as a determinant of their developmental potential.
Construction of functional gene modules of HCC based on Multiscale Embedded Gene Co-expression Network Analysis
Gene coexpression networks can be used to effectively identify functional gene modules, and Multiscale Embedded Gene Co-expression Network Analysis (MEGENA) (28) adopts the network embedding paradigm in the field of topology. MEGENA comprises the following main steps: construction of a planar filter network, multiscale cluster analysis through the compactness of the network structure, calculation of subcluster compactness, and identification of hub genes in subclusters. Notably, the hub gene is defined as the node with the highest degree in the subcluster.
Verification of candidate gene expression in HCC cells at the protein level
From the Human Protein Atlas (HPA) database (29) (https://www.proteinatlas.org/), protein expression data for HCC and non-HCC cells were obtained. The expression levels were then evaluated according to the intensity and density of immunohistochemical (IHC) staining. The intensity and density scores were multiplied to obtain an aggregate IHC staining value, and the result was placed on a protein expression scale of 0 to 9 points.
Classification of patient clusters based on the “c1_12” module genes and definition of corresponding clinical features
In recent years, nonnegative matrix factorization (NMF) has been widely used in gene expression data clustering, regulation pattern and function module recognition, and other areas (30). NMF is a valuable tool because it can recognize the local features of data to a certain extent and quantitatively describe, partially or wholly, the potential nonlinear combination relationship (31). In this study, the molecular cluster model was constructed using the NMF software package in R software (k =2–10), and the model with fine clustering stability was selected according to the clustering effect. Simultaneously, we collected the clinical data from TCGA-HCC samples, including the tumor region, TNM stage, and pathological grade. The χ2 test was conducted to evaluate the difference in clinical characteristics between the clusters. The log-rank test was then performed using OS to determine whether there were significant differences in prognosis. P values <0.05 were considered statistically significant.
As TIT research has progressed, tumor mutation burden (TMB) has emerged as a new biomarker. TMB is defined as the total number of somatic gene coding errors (i.e., base substitution, gene insertion, or gene deletion errors) detected per million bases (32). In this study, the whole-exon somatic mutation data of TCGA was in mutation annotation format (MAF). The somatic mutation data were processed using Mutect2 from GATK4 software and was selected to calculate the TMB and determine whether there were significant differences in the TMB of different clusters. The abnormal expression of immune checkpoint (ICP) molecules is a tumor immune escape mechanism, and several ICP pathways have been found to be related to tumor immune escape (33). Therefore, TMB and the expression distribution of ICP molecules in the clusters were evaluated via analysis of variance (ANOVA). The ICP molecules included tumor necrosis factor receptor superfamily member 14 (TNFRSF14), neuropilin 1 (NRP1), lymphocyte-activating 3 (LAG3), cytotoxic T-lymphocyte–associated protein 4 (CTLA4), programmed cell death 1 (PDCD1), CD276 molecule (CD276), programmed cell death 1 ligand 2 (PDCD1LG2), indoleamine 2,3-dioxygenase 1 (IDO1), V-set domain–containing T-cell activation inhibitor 1 (VTCN1), indoleamine 2,3-dioxygenase 2 (IDO2), TNF superfamily member 9 (TNFSF9), T-cell immunoreceptor with immunoglobin and ITIM domains (TIGIT), CD274 molecule (CD274), TNF receptor superfamily member 9 (TNFRSF9), and CD40 molecule (CD40).
Analysis of the mutation characteristics of the patient clusters based on mutation signature and copy number variation (CNV) level
Driver mutations are causally involved in cancer formation, which gives cancer cells a growth advantage (34). The mutation characteristics of HCC were analyzed to explore its possible etiology and mechanism. The mutation signature analysis was completed using the “MutationalPatterns” package in R (35). Bayesian NMF analysis was performed to examine the mutation categories and process in greater detail. In the TCGA-LIHC cohort, the mutations were stratified according to 96 trinucleotide backgrounds. Bayesian NMF automatically deletes irrelevant components that are not conducive to explaining the observed mutations and effectively determines the appropriate number of signatures and their sample-specific contributions.
CNV is an important source of genetic variation (36) and involves the repetition of DNA fragments greater than 1 kb. The number of fragments and type of variation (deletion, duplication, inversion, and translocation) can differ between individuals, which greatly enriches genetic diversity (37). The genomic copy information of the TCGA-LIHC samples was downloaded. The whole-genome copy information of the tumor tissue samples was analyzed using GISTIC 2.0 software on GenePattern (https://cloud.genepattern.org/gp/pages/login.jsf). The reference genome was Human_Hg38.UCSC.add_miR.160920.refgene.mat. ANOVA analysis was conducted to calculate the amplification and deletion frequency differences between the clusters.
Identification of immune infiltration and enrichment analysis of the patient clusters
Immune cells play various roles in tumorigenesis, and both the composition and characteristics of the immune cell population differ in different tumors. Therefore, in studying tumor mechanisms, quantitative examination of different types of immune cells is necessary. Tumor immune estimation resource (TIMER; https://cistrome.shinyapps.io/timer/) is an online database resource for analyzing immune cell infiltration in various cancers. The deconvolution method was used to estimate the degree of infiltration of immune cells into tumor tissues from the gene expression profiles and to analyze the correlation between different clusters and the infiltration of immune cells (B cells, CD8+ T lymphocytes, CD4+ T lymphocytes, macrophages, neutrophils, and dendritic cells). The “c2.cp.kegg.v7.5.1.symbols.gmt” gene set from the MSIGDB was downloaded as the reference gene set. Gene set variation analysis (GSVA) was performed on the clusters using the GSVA package in R software. ANOVA was conducted to evaluate the variable distribution difference between the clusters.
Statistical analysis
For data analysis and plotting, Stata 14.0, R software, and GraphPad Prism 8.0 (GraphPad Software Inc.) were used. A P value less than 0.05 was considered statistically significant.
Results
Identification of immune-related genes with strong prognostic value
Definition of 68 genes upregulated in HCC tissue that were associated with immune response
To confirm the gene expression pattern in HCC tissues, we collected high-throughput datasets based on 7,384 samples (3,941 HCC vs. 3,443 non-HCC tissues), 84 central datasets, and 39 platforms. A P value <0.05 indicated significant heterogeneity in the included datasets, and a random effects model was selected for the analysis (Figures S1-S5). A total of 8,676 genes with SMD greater than 0 were obtained. Simultaneously, we also obtained 225 genes related to 13 immune-related pathways from the MSIGDB database. According to their intersection, 68 candidate genes were then obtained.
Estimation of cell clusters as the initial step in HCC cell differentiation
After identifying the genes that were highly expressed in HCC tissues, we evaluated the expression of these genes in HCC cells with high differentiation potential. Via t-SNE, 7 integrated samples of GSE112271 were distinguished into 16 different clusters using an unsupervised clustering method (Figure 2A). In the CopyKAT prediction results, aneuploidy represents tumor cells with aneuploidy variation, and diploid represents normal cells. The identification results were mapped using t-SNE (Figure 2B). In the CNV heatmap, shown in Figure 2C, each row represents a cell, each column represents a chromosome position, and the color represents the degree of change in the copy number. To understand the differences in the degree of differentiation between malignant cells with high CNV, we constructed a pseudotime trajectory of cell differentiation based on cell regrouping (Figure 2D,2E). Afterward, genes that were differentially expressed among the malignant cells were taken as the reference gene set. Ultimately, a pseudo-temporal algorithm was employed to predict the dynamic development trajectory of malignant cells, and 4 time points of cell differentiation were defined (Figure 2F,2G). Then, the differentialGeneTest function in Monocle was used to determine the best 2 clusters based on the significant driver genes (Figure 3A). More importantly, the CytoTRACE score was calculated to predict the trajectory relationship between 15 clusters of HCC cells, and cluster 9 was estimated to have the highest differentiation potential (Figure 3B-3E). Moreover, significantly differentially expressed genes in cluster 9 were found to be enriched in pathways that included the 2019 coronavirus disease (COVID-19), ribosome, and complement and coagulation cascades (Figure 3F). Finally, 13 genes [human leukocyte antigen, class I, A (HLA-A), TAP binding protein (TAPBP), cell division cycle 42 (CDC42), heat shock protein family A (HSP70) member 1A (HSPA1A), HSP70 member 8 (HSPA8), HSP70 member 1B (HSPA1B), NFKB inhibitor epsilon (NFKBIE), HSP70 member 5 (HSPA5), HSP110 member 1 (HSPH1), HSP90 alpha family class A member 1 (HSP90AA1), Ras homolog family member A (RHOA), cyclin0dependent kinase 4 (CDK4), and HSP90A family class B member 1 (HSP90AB1)] were screened to meet the following 4 conditions: upregulated in HCC cells with high CNV accumulation, a driving factor of early differentiation of HCC cells, related to the activation of APC function, and a high affinity for T-cell receptor or MHC molecules.
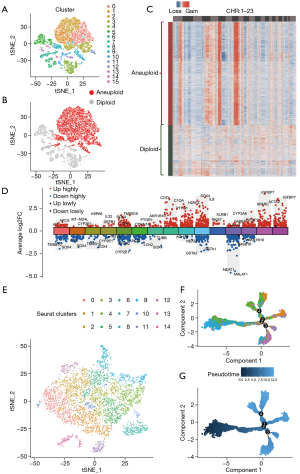
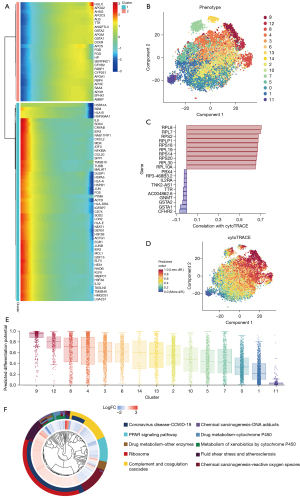
Identification of 5 genes as hub genes
The coexpression analysis was then conducted with MEGENA. Among the 13 genes, 5 (HSP90AA1, CDK4, HSPA8, HSPH1, and HSPA5) were specifically clustered in the “c1_12” module (Figure 4A), suggesting that these genes are involved in similar biological processes. Further analysis confirmed that the 5 genes were specific differentiation driving molecules that are active in the early stage of HCC (Figure 4B), and the HCC samples were clustered into 3 groups based on the expression of these genes (Figure 4C). Indexing of the specificity, sensitivity, sROC, and likelihood ratio indicated that HCC tissues could be discriminated based on these genes (Figure 5, Figures S6-S10). More importantly, the immune infiltration and survival analysis results suggested that these 5 genes were associated with the level of immune cells and the prognosis of HCC (Figure S11).
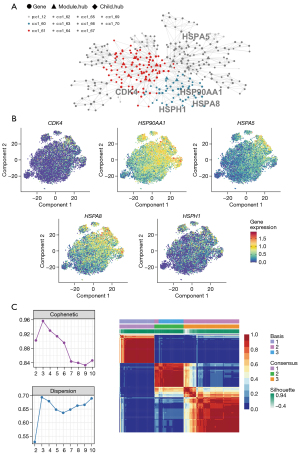
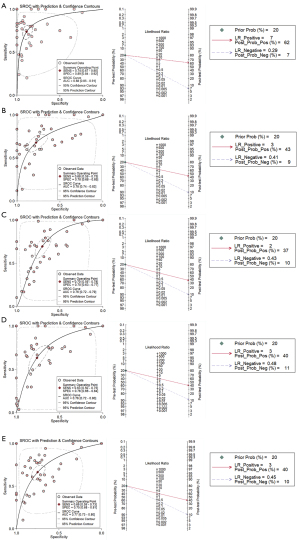
Verification of the upregulation of the proteins encoded by the 5 candidate genes in HCC
After determining the transcription levels of the candidate genes, we evaluated the associated protein levels. IHC staining was conducted to detect the protein expression associated with the 5 candidate genes in HCC tissue and hepatocyte samples. Representative IHC staining images are shown in Figure 6. The results showed that proteins encoded by these 5 genes were upregulated in HCC cells. However, due to the small sample size, the differential expression was not statistically tested and must be verified in subsequent experiments.
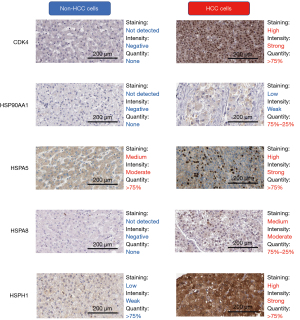
Overall, multiple levels of evidence confirmed that the genes encoding HSP90AA1, CDK4, HSPA8, HSPH1, and HSPA5 are promising biomarkers both in the prognosis prediction and immunotherapy of HCC.
Clinical features of patients classified in cluster 2
Based on the above findings, the “c1_12” module, which contains 333 genes, was regarded as a function network related to HCC immune response and prognosis. TCGA-LIHC samples were then classified according to the expression of the 333 genes, and clustering stability was comprehensively determined, with k =3 presenting the best effect (Figure 4C; Figure S12). The HCC samples were divided into 3 clusters, and χ2 testing showed that there were significant differences in the distribution of tumor region, stage, and pathological grade (Figure 7A-7D). The log-rank test showed that the prognoses associated with the 3 clusters were significantly different (P<0.0001; Figure 7E). Although no significant difference in TMB was found among the 3 clusters (Figure 7F), the abnormal upregulation of 15 ICP genes (TNFRSF14, NRP1, LAG3, CTLA4, PDCD1, CD276, PDCD1LG2, IDO1, VTCN1, IDO2, TNFSF9, TIGIT, CD274, TNFRSF9, CD40) in patients in cluster 2 (Figure 7G) suggested that immune escape was the cause of the poor prognosis for patients in cluster 2.
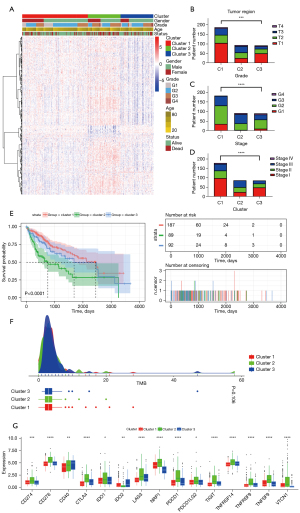
Mutation characteristics of the 3 clusters of patients with HCC
We then attempted to define the mutation differences between the 3 clusters. Bayesian NMF was used to identify the mutation characteristics of samples from 91 patients derived from TCGA-LIHC. Three mutation patterns were detected in the HCC cell genomes (Figure 8A,8B). Two of the patterns matched DNA damage patterns, namely adenine damage and guanine damage. The third mutation feature was T > C substitutions. Cluster 2 was found to be characterized by a higher proportion of adenine damage and a lower proportion of T > C substitutions (Figure 8C). In Figure 8D, the y-axis indicates 3 values: the number of mutations of each type in each specific sequence, the total number of mutations associated with the 3 mutation characteristics in each Euro-American patient with HCC, and the relative proportion of mutation types. Whole-genome CNV analysis of TCGA-LIHC tumor tissue samples was performed using GISTIC 2.0 software. The results showed that the amplification frequency in multiple regions of cluster 2 samples was the highest of all clusters, especially in regions 10p/q, 12p/q, and 17p/q (Figure 8E). However, there were no obvious differential characteristics in the focal somatic copy number alteration (SCNA) level of cluster 2 samples (Figure 8F). The mutation characteristics of the clusters suggested that different clusters of patients with HCC had different degrees of immunogenicity.
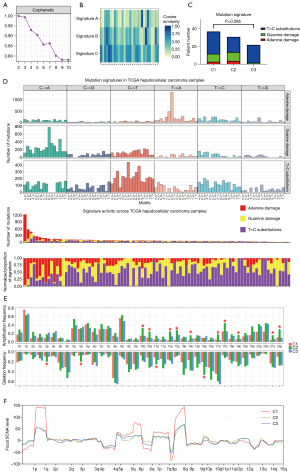
Immune infiltration and molecular mechanism characteristics of cluster 2 patients
The 3 clusters of patients were also found to have significant differences in immune infiltration and biological pathway activity. We used the TIMER algorithm to calculate the fraction of infiltrating immune cells. The results showed that cluster 2 samples had a higher expression of CD8+ T cells, CD4+ T cells, and macrophages than did samples in the other 2 clusters, and the difference was statistically significant (P<0.05; Figure 9A-9D). In addition, the pathway phenotypes of the 3 clusters were quantified based on GSVA, and it was found that the main characteristics of cluster 2 were highly active cell cycle, spliceosome, and ribosome pathways, and low primary bile acid biosynthesis (Figure 9E,9G).

Discussion
In this study, we constructed an abnormal expression map of HCC cell genes using data from 7,384 samples (3,941 HCC vs. 3,443 non-HCC tissues), 84 central datasets, and 39 platforms. By screening for both immune-related genes and those associated with high differentiation potential in HCC cell development, we identified a series of target genes, among which HSP90AA1, CDK4, HSPA8, HSPH1, and HSPA5 were promising biomarkers for HCC prognosis prediction and immunotherapy. Therefore, these genes may play a key role in the development and progression of HCC. Our research approach has the following advantages. First, our selection strategy was based on gene expression in HCC tissues and combined evidence-based concepts with scRNA-seq analysis of HCC cells, which strengthened the expression pattern specificity of the candidate genes in HCC cells. Second, through scRNA-seq cell trajectory analysis, the genes we selected were potential drivers of HCC cell differentiation and development. They were associated with malignant phenotypes identified by prognostic analysis. Finally, our strategy considered the relationships between hub genes and multiple immune response pathways, as well as their correlation with typical immune cells, suggesting that these genes may play a significant role in tumor immune escape and immunotherapy of HCC.
Although other studies associated with mining immune-related prognostic biomarkers have been published, they tend to lack precision, which impacts the amount of confidence in the techniques and results (38,39). For example, screening differentially expressed genes through a single dataset could cause result bias. In addition, in view of the complexity of the immune system, the commonly used classification methods that are based on proven immune-related genes may not be suitable. In contrast, we chose to use the coexpression network module of candidate genes as the classification feature, which fit the selection strategy for patients with HCC potentially benefiting from immunotherapy. Therefore, our data mining method, which is based on multiple levels of evidence and on the biological mechanisms of candidates, is worthy of being used for this and other related research.
Previous studies have also supported the prospect of incorporating our selected molecules into the biomarkers of TIT. Four of the candidate genes belong to the HSP family. HSP is highly expressed when cells are under stress and plays an important role in protein transport, folding, and dissociation (40). Its immunological activities include binding antigen peptides as molecular chaperones, presenting them to MHC molecules through APCs, and then inducing specific immunity (41,42). The results of recent double-blind multicenter phase II and III clinical trials of renal cell carcinoma and melanoma suggest that the HSP–antigen peptide complex is safe and effective as a TIT (43,44).
HSPH1 is a member of the HSP110 family, which has been demonstrated to be an effective immune adjuvant and has a strong chaperone function and polypeptide binding ability (45,46). HSPH1 silencing has been demonstrated to be parallel to BCL6 transcription repressor (BCL-6) and MYC proto-oncogene (c-MYC) downregulation in 4 invasive B-cell non-Hodgkin lymphoma (B-NHL) models (47). Interestingly, it was also found that HSPH1 physically interacts with c-MYC and BCL-6 in Namalwa cells and primary invasive B-NHL.
Similarly, HSPA5 and HSPA8 are members of the HSP70 family (48). HSPA5 is mainly located in the endoplasmic reticulum (49). When the endoplasmic reticulum is stressed, for example, due to a lack of glucose or interference with intercellular stored calcium ions, HSPA5 expression rises sharply, which can prevent protein misfolding, protect protein transport, and maintain cell homeostasis (50). Other studies have shown that HSPA5 can bind to caspase 7 (CASP7), prevent CASP7-induced apoptosis, and cause tumor cells to acquire drug resistance through autophagy (51,52). Many studies have shown that HSPA8 is overexpressed on tumor tissues (53-55). Sirtori et al. reported that knocking out HSPA8 resulted in the upregulation of tau, superoxide dismutase 1, and α-synuclein protein levels (56).
HSP90 is another important member of the HSP family, and it is a highly conserved chaperone protein in eukaryotes (57,58). The HSP90α protein is encoded by the HSP90AA1 gene. As a tumor promoter, HSP90 can interact with a variety of carcinogenic proteins and participate in the process of malignant tumor transformation (59). Current research on prostate cancer shows that extracellular HSP90α can be used in signal transduction to promote tumor-associated fibroblasts to produce chronic inflammation (60). This change in the extracellular environment of malignant tumors can significantly promote the progression of prostate cancer. Hence, HSP90AA1 is involved in the malignant behavior of tumor cells in both cellular stress and nonstress responses, and the properties of HSP have been used to develop anticancer vaccines that induce cytotoxic reactions against tumors. However, the biological roles that the 4 genes identified here play in HCC have not yet been confirmed, emphasizing the groundbreaking nature of our research and the necessity for subsequent functional verification.
CDK4 is a key protein in the cell cycle process, and the upregulation of CDK4 is closely related to the proliferation of HCC cells (61,62). Concurrently, the upregulation of CDK4 is a predictor for poor OS in patients with HCC (63). Importantly, Chaikovsky et al. observed that CDK4/6i enhanced tumor antigen presentation through increased type III interferon synthesis in breast cancer cell lines treated with the CDK4/6i abemaciclib (64), which indicated that CDK4/6i could be applied in patients with HCC with suppressed immune systems. In brief, we are the first team to identify these 5 genes as promising HCC biomarkers for TIT and prognosis prediction.
Since TIT may be beneficial to specific patients with cancer with specific transcriptome characteristics, we divided samples from patients with HCC into 3 clusters according to the coexpression network of the 5 candidate genes to select the appropriate population for immunotherapy. These 3 clusters showed different molecular, cellular, and clinical characteristics. Among them, the prognosis of the patients in cluster 2 was significantly lower than that of patients in the other clusters, indicating that this molecular classification could be used to predict the prognosis of patients with HCC. Simultaneously, this molecular classification could also be used to predict the treatment effect of TIT. In this study, patients in cluster 2 were found to have higher amplification frequencies, which may lead to greater reactivity to a tumor vaccine and higher immunogenicity. It is also worth noting that the tumor microenvironment may dominate the immune response to immunotherapy (65). According to the tumor microenvironment, HCC can be divided into “immune-hot” tumors and “immune-cold” tumors, with “immune-hot” tumors that show a high degree of immune infiltration being more likely to respond to ICIs (66-68). Thus, we have described the immune cell populations present in the different clusters. The cluster 2 samples were found to have significantly higher quantities of CD8+ T cells, macrophages, and CD4+ T cells than did samples from the other clusters, which suggests they are a suitable population of patients for TIT.
The current work has some limitations, the discussion of which may provide ideas for future work. First, the defined candidate biomarkers must be validated via in vitro and in vivo experiments. At the in vitro level, the malignant biological characteristics of the 5 genes in HCC cells need to be confirmed. At the in vivo level, an experimental HCC animal model should be developed to assess the tumor microenvironment status and curative effect of immunotherapy. Second, there are several types of TIT, and all these types deserve further research and analysis. Extensive analysis of tumor microenvironment and classification based on multiple factors can more comprehensively guide HCC treatment and improve the prognosis. For example, the combination of PD-1 and a tumor vaccine may help overcome the immunotolerant microenvironment prevalent in HCC and boost the immunogenicity of vaccines. Our findings show that patients with the type of HCC classified as “cluster 2” may benefit the most from such a treatment strategy. The combination of ICIs and tumor vaccines could also be investigated in an HCC animal model.
Conclusions
The genes encoding CDK4, HSP90AA1, HSPA5, HSPA8, and HSPH1 were identified as prospective biomarkers for prognosis prediction and immunotherapy of HCC. Using our molecular classification system, which is based on a function module containing 5 candidate genes, patients with the type of HCC classified as “cluster 2” might be suitable candidates for TIT. These findings provide new insights into the selection of candidate genes and patient populations for future HCC immunotherapy.
Acknowledgments
Thanks for the sharing of datasets provided by public databases and the technical support of Guangxi Key Laboratory of Medical Pathology.
Funding: This research was supported by
Footnote
Reporting Checklist: The authors have completed the REMARK reporting checklist. Available at https://tcr.amegroups.com/article/view/10.21037/tcr-22-2304/rc
Peer Review File: Available at https://tcr.amegroups.com/article/view/10.21037/tcr-22-2304/prf
Conflicts of Interest: All authors have completed the ICMJE uniform disclosure form (available at https://tcr.amegroups.com/article/view/10.21037/tcr-22-2304/coif). The authors have no conflicts of interest to declare.
Ethical Statement: The authors are accountable for all aspects of the work in ensuring that questions related to the accuracy or integrity of any part of the work are appropriately investigated and resolved. The study was conducted in accordance with the Declaration of Helsinki (as revised in 2013).
Open Access Statement: This is an Open Access article distributed in accordance with the Creative Commons Attribution-NonCommercial-NoDerivs 4.0 International License (CC BY-NC-ND 4.0), which permits the non-commercial replication and distribution of the article with the strict proviso that no changes or edits are made and the original work is properly cited (including links to both the formal publication through the relevant DOI and the license). See: https://creativecommons.org/licenses/by-nc-nd/4.0/.
References
- Ng CKY, Dazert E, Boldanova T, et al. Integrative proteogenomic characterization of hepatocellular carcinoma across etiologies and stages. Nat Commun 2022;13:2436. [Crossref] [PubMed]
- Salem R, Johnson GE, Kim E, et al. Yttrium-90 Radioembolization for the Treatment of Solitary, Unresectable HCC: The LEGACY Study. Hepatology 2021;74:2342-52. [Crossref] [PubMed]
- Anselmo A, Sensi B, Bacchiocchi G, et al. All the Routes for Laparoscopic Liver Segment VIII Resection: A Comprehensive Review of Surgical Techniques. Front Oncol 2022;12:864867. [Crossref] [PubMed]
- Zheng Z, Guan R, Jianxi W, et al. Microvascular Invasion in Hepatocellular Carcinoma: A Review of Its Definition, Clinical Significance, and Comprehensive Management. J Oncol 2022;2022:9567041. [Crossref] [PubMed]
- Huang ZH, Lu GY, Qiu LX, et al. Risk of hepatocellular carcinoma in antiviral treatment-naïve chronic hepatitis B patients treated with entecavir or tenofovir disoproxil fumarate: a network meta-analysis. BMC Cancer 2022;22:287. [Crossref] [PubMed]
- Bai XM, Cui M, Yang W, et al. The 10-year Survival Analysis of Radiofrequency Ablation for Solitary Hepatocellular Carcinoma 5 cm or Smaller: Primary versus Recurrent HCC. Radiology 2021;300:458-69. [Crossref] [PubMed]
- Wang R, Lin N, Mao B, et al. The efficacy of immune checkpoint inhibitors in advanced hepatocellular carcinoma: a meta-analysis based on 40 cohorts incorporating 3697 individuals. J Cancer Res Clin Oncol 2022;148:1195-210. [Crossref] [PubMed]
- Liang N, Yang T, Huang Q, et al. Mechanism of cancer stemness maintenance in human liver cancer. Cell Death Dis 2022;13:394. [Crossref] [PubMed]
- Jin Y, Wang W, Wang Q, et al. Alpha-1-antichymotrypsin as a novel biomarker for diagnosis, prognosis, and therapy prediction in human diseases. Cancer Cell Int 2022;22:156. [Crossref] [PubMed]
- Sivori S, Pende D, Quatrini L, et al. NK cells and ILCs in tumor immunotherapy. Mol Aspects Med 2021;80:100870. [Crossref] [PubMed]
- Xuan Y, Guan M, Zhang S. Tumor immunotherapy and multi-mode therapies mediated by medical imaging of nanoprobes. Theranostics 2021;11:7360-78. [Crossref] [PubMed]
- Ren X, Zhang L, Zhang Y, et al. Insights Gained from Single-Cell Analysis of Immune Cells in the Tumor Microenvironment. Annu Rev Immunol 2021;39:583-609. [Crossref] [PubMed]
- Sangro B, Sarobe P, Hervás-Stubbs S, et al. Advances in immunotherapy for hepatocellular carcinoma. Nat Rev Gastroenterol Hepatol 2021;18:525-43. [Crossref] [PubMed]
- Zongyi Y, Xiaowu L. Immunotherapy for hepatocellular carcinoma. Cancer Lett 2020;470:8-17. [Crossref] [PubMed]
- Lee HW, Cho KJ, Park JY. Current Status and Future Direction of Immunotherapy in Hepatocellular Carcinoma: What Do the Data Suggest? Immune Netw 2020;20:e11. [Crossref] [PubMed]
- Zhang L, Ding J, Li HY, et al. Immunotherapy for advanced hepatocellular carcinoma, where are we? Biochim Biophys Acta Rev Cancer 2020;1874:188441. [Crossref] [PubMed]
- Liu T, Tan J, Wu M, et al. High-affinity neoantigens correlate with better prognosis and trigger potent antihepatocellular carcinoma (HCC) activity by activating CD39(+)CD8(+) T cells. Gut 2021;70:1965-77. [Crossref] [PubMed]
- Liu Z, Liu X, Liang J, et al. Immunotherapy for Hepatocellular Carcinoma: Current Status and Future Prospects. Front Immunol 2021;12:765101. [Crossref] [PubMed]
- Ranzoni AM, Tangherloni A, Berest I, et al. Integrative Single-Cell RNA-Seq and ATAC-Seq Analysis of Human Developmental Hematopoiesis. Cell Stem Cell 2021;28:472-487.e7. [Crossref] [PubMed]
- Pan H, Xue C, Auerbach BJ, et al. Single-Cell Genomics Reveals a Novel Cell State During Smooth Muscle Cell Phenotypic Switching and Potential Therapeutic Targets for Atherosclerosis in Mouse and Human. Circulation 2020;142:2060-75. [Crossref] [PubMed]
- Platten M, Bunse L, Wick A, et al. A vaccine targeting mutant IDH1 in newly diagnosed glioma. Nature 2021;592:463-8. [Crossref] [PubMed]
- Duan H, Liu Y, Gao Z, et al. Recent advances in drug delivery systems for targeting cancer stem cells. Acta Pharm Sin B 2021;11:55-70. [Crossref] [PubMed]
- Wang Q, Liang N, Yang T, et al. DNMT1-mediated methylation of BEX1 regulates stemness and tumorigenicity in liver cancer. J Hepatol 2021;75:1142-53. [Crossref] [PubMed]
- Losic B, Craig AJ, Villacorta-Martin C, et al. Intratumoral heterogeneity and clonal evolution in liver cancer. Nat Commun 2020;11:291. [Crossref] [PubMed]
- Gao R, Bai S, Henderson YC, et al. Delineating copy number and clonal substructure in human tumors from single-cell transcriptomes. Nat Biotechnol 2021;39:599-608. [Crossref] [PubMed]
- Qiu X, Mao Q, Tang Y, et al. Reversed graph embedding resolves complex single-cell trajectories. Nat Methods 2017;14:979-82. [Crossref] [PubMed]
- Gulati GS, Sikandar SS, Wesche DJ, et al. Single-cell transcriptional diversity is a hallmark of developmental potential. Science 2020;367:405-11. [Crossref] [PubMed]
- Song WM, Zhang B. Multiscale Embedded Gene Co-expression Network Analysis. PLoS Comput Biol 2015;11:e1004574. [Crossref] [PubMed]
- Colwill K. Gräslund S. A roadmap to generate renewable protein binders to the human proteome. Nat Methods 2011;8:551-8. [Crossref] [PubMed]
- Pan J, Gillis N. Generalized Separable Nonnegative Matrix Factorization. IEEE Trans Pattern Anal Mach Intell 2021;43:1546-61. [Crossref] [PubMed]
- Che H, Wang J, Cichocki A. Bicriteria Sparse Nonnegative Matrix Factorization via Two-Timescale Duplex Neurodynamic Optimization. IEEE Trans Neural Netw Learn Syst 2021; Epub ahead of print. [Crossref] [PubMed]
- Chan TA, Yarchoan M, Jaffee E, et al. Development of tumor mutation burden as an immunotherapy biomarker: utility for the oncology clinic. Ann Oncol 2019;30:44-56. [Crossref] [PubMed]
- Geisler AN, Phillips GS, Barrios DM, et al. Immune checkpoint inhibitor-related dermatologic adverse events. J Am Acad Dermatol 2020;83:1255-68. [Crossref] [PubMed]
- McGrail DJ, Pilié PG, Rashid NU, et al. High tumor mutation burden fails to predict immune checkpoint blockade response across all cancer types. Ann Oncol 2021;32:661-72. [Crossref] [PubMed]
- Fantini D, Vidimar V, Yu Y, et al. MutSignatures: an R package for extraction and analysis of cancer mutational signatures. Sci Rep 2020;10:18217. [Crossref] [PubMed]
- Purwar N, Tiwari P, Mathur N, et al. Higher CNV Frequencies in Chromosome 14 of Girls With Turner Syndrome Phenotype. J Clin Endocrinol Metab 2021;106:e4935-55. [Crossref] [PubMed]
- Royer-Bertrand B, Cisarova K, Niel-Butschi F, et al. CNV Detection from Exome Sequencing Data in Routine Diagnostics of Rare Genetic Disorders: Opportunities and Limitations. Genes (Basel) 2021;12:1427. [Crossref] [PubMed]
- Dai Y, Qiang W, Lin K, et al. An immune-related gene signature for predicting survival and immunotherapy efficacy in hepatocellular carcinoma. Cancer Immunol Immunother 2021;70:967-79. [Crossref] [PubMed]
- Xia X, Tang P, Liu H, et al. Identification and Validation of an Immune-related Prognostic Signature for Hepatocellular Carcinoma. J Clin Transl Hepatol 2021;9:798-808. [Crossref] [PubMed]
- Jiang F, Chang G, Li Z, et al. The HSP/co-chaperone network in environmental cold adaptation of Chilo suppressalis. Int J Biol Macromol 2021;187:780-8. [Crossref] [PubMed]
- Ge L, Zhao N, Miao Y, et al. Inhibitory effect of edible natural compounds with di- and tri-carboxyl moiety on endogenous protease inducing disassembly and degradation of myofibrils from grass carp (Ctenopharyngodon idella). Food Res Int 2020;137:109457. [Crossref] [PubMed]
- Upadhyay A. Natural compounds in the regulation of proteostatic pathways: An invincible artillery against stress, ageing, and diseases. Acta Pharm Sin B 2021;11:2995-3014. [Crossref] [PubMed]
- Baldin AV, Zamyatnin AA Jr, Bazhin AV, et al. Advances in the Development of Anticancer HSP-based Vaccines. Curr Med Chem 2019;26:427-45. [Crossref] [PubMed]
- Dass SA, Norazmi MN, Dominguez AA, et al. Generation of a T cell receptor (TCR)-like single domain antibody (sDAb) against a Mycobacterium Tuberculosis (Mtb) heat shock protein (HSP) 16kDa antigen presented by Human Leukocyte Antigen (HLA)-A*02. Mol Immunol 2018;101:189-96. [Crossref] [PubMed]
- Zhang Y, Ren F, Ni B, et al. Tumor targeting nanoparticle E7(49-57)-HSP110-RGD elicits potent anti-tumor immune response in a CD8-dependent manner in cervical cancer-bearing mouse model. Hum Vaccin Immunother 2021;17:3529-38. [Crossref] [PubMed]
- Marcion G, Hermetet F, Neiers F, et al. Nanofitins targeting heat shock protein 110: An innovative immunotherapeutic modality in cancer. Int J Cancer 2021;148:3019-31. [Crossref] [PubMed]
- Zappasodi R, Ruggiero G, Guarnotta C, et al. HSPH1 inhibition downregulates Bcl-6 and c-Myc and hampers the growth of human aggressive B-cell non-Hodgkin lymphoma. Blood 2015;125:1768-71. [Crossref] [PubMed]
- Li J, Ge Z. High HSPA8 expression predicts adverse outcomes of acute myeloid leukemia. BMC Cancer 2021;21:475. [Crossref] [PubMed]
- Shin J, Toyoda S, Nishitani S, et al. Possible Involvement of Adipose Tissue in Patients With Older Age, Obesity, and Diabetes With SARS-CoV-2 Infection (COVID-19) via GRP78 (BIP/HSPA5): Significance of Hyperinsulinemia Management in COVID-19. Diabetes 2021;70:2745-55. [Crossref] [PubMed]
- Ullrich F. Networking counters stress. Nat Struct Mol Biol 2021;28:873. [Crossref] [PubMed]
- Cook KL, Shajahan AN, Wärri A, et al. Glucose-regulated protein 78 controls cross-talk between apoptosis and autophagy to determine antiestrogen responsiveness. Cancer Res 2012;72:3337-49. [Crossref] [PubMed]
- Reddy RK, Mao C, Baumeister P, et al. Endoplasmic reticulum chaperone protein GRP78 protects cells from apoptosis induced by topoisomerase inhibitors: role of ATP binding site in suppression of caspase-7 activation. J Biol Chem 2003;278:20915-24. [Crossref] [PubMed]
- Hoshino A, Kim HS, Bojmar L, et al. Extracellular Vesicle and Particle Biomarkers Define Multiple Human Cancers. Cell 2020;182:1044-1061.e18. [Crossref] [PubMed]
- Liu Z, Zheng W, Liu Y, et al. Targeting HSPA8 inhibits proliferation via downregulating BCR-ABL and enhances chemosensitivity in imatinib-resistant chronic myeloid leukemia cells. Exp Cell Res 2021;405:112708. [Crossref] [PubMed]
- Yang F, Xie HY, Yang LF, et al. Stabilization of MORC2 by estrogen and antiestrogens through GPER1- PRKACA-CMA pathway contributes to estrogen-induced proliferation and endocrine resistance of breast cancer cells. Autophagy 2020;16:1061-76. [Crossref] [PubMed]
- Sirtori R, Riva C, Ferrarese C, et al. HSPA8 knock-down induces the accumulation of neurodegenerative disorder-associated proteins. Neurosci Lett 2020;736:135272. [Crossref] [PubMed]
- Chakraborty A, Edkins AL. HSP90 as a regulator of extracellular matrix dynamics. Biochem Soc Trans 2021;49:2611-25. [Crossref] [PubMed]
- Calvo-Vidal MN, Zamponi N, Krumsiek J, et al. Oncogenic HSP90 Facilitates Metabolic Alterations in Aggressive B-cell Lymphomas. Cancer Res 2021;81:5202-16. [Crossref] [PubMed]
- Zeng J, He SL, Li LJ, et al. Hsp90 up-regulates PD-L1 to promote HPV-positive cervical cancer via HER2/PI3K/AKT pathway. Mol Med 2021;27:130. [Crossref] [PubMed]
- Eguchi T, Sogawa C, Ono K, et al. Cell Stress Induced Stressome Release Including Damaged Membrane Vesicles and Extracellular HSP90 by Prostate Cancer Cells. Cells 2020;9:755. [Crossref] [PubMed]
- Fassl A, Geng Y, Sicinski P. CDK4 and CDK6 kinases: From basic science to cancer therapy. Science 2022;375:eabc1495. [Crossref] [PubMed]
- Hendrychová D, Jorda R, Kryštof V. How selective are clinical CDK4/6 inhibitors? Med Res Rev 2021;41:1578-98. [Crossref] [PubMed]
- Bin X, Chen Y, Ma J, et al. circ_0001588 Induces the Malignant Progression of Hepatocellular Carcinoma by Modulating miR-874/CDK4 Signaling. J Immunol Res 2021;2021:3759879. [Crossref] [PubMed]
- Chaikovsky AC, Sage J. Beyond the Cell Cycle: Enhancing the Immune Surveillance of Tumors Via CDK4/6 Inhibition. Mol Cancer Res 2018;16:1454-7. [Crossref] [PubMed]
- Bejarano L, Jordāo MJC, Joyce JA. Therapeutic Targeting of the Tumor Microenvironment. Cancer Discov 2021;11:933-59. [Crossref] [PubMed]
- Liu Z, Liu L, Guo C, et al. Tumor suppressor gene mutations correlate with prognosis and immunotherapy benefit in hepatocellular carcinoma. Int Immunopharmacol 2021;101:108340. [Crossref] [PubMed]
- Ning YM, Lin K, Liu XP, et al. NAPSB as a predictive marker for prognosis and therapy associated with an immuno-hot tumor microenvironment in hepatocellular carcinoma. BMC Gastroenterol 2022;22:392. [Crossref] [PubMed]
- Hu Y, Sun H, Zhang H, et al. An Immunogram for an Individualized Assessment of the Antitumor Immune Response in Patients With Hepatocellular Carcinoma. Front Oncol 2020;10:1189. [Crossref] [PubMed]