Cuproptosis-related lncRNAs forecast the prognosis of acute myeloid leukemia
Highlight box
Key findings
• Cuproptosis-related lncRNAs are able to forecast the risk of AML patients.
What is known and what is new?
• Copper ions are not bystanders in the occurrence and progression of AML.
• A new CuRS system can guide prognostic stratification and precise management of AML.
What is the implication, and what should change now?
• Clinicians can develop a fresh perspective on the treatment of AML targeting cuproptosis.
Introduction
Acute myeloid leukemia (AML), the most prevalent type of leukemia in adults, is a malignant clonal disease derived from hematopoietic progenitor cells (1). The occurrence and development of AML are often accompanied by a variety of genetic abnormalities, including chromosomal abnormalities such as t [8; 21], inv [16], t [15; 17], abnormalities in genes such as FLT3, PDGFB, RUNX1, NPM1, CEBPA, ASXL1 (2). In recent years, clinicians appreciate the applications of targeted small-molecule inhibitors, such as IDH1/IDH2 inhibitors, FLT3 inhibitors, BCL2 inhibitors, and Hedgehog pathway inhibitors in addition to intensive chemotherapy (3). Emerging chimeric antigen receptor-T cell (CAR-T) therapies, antibody-based therapies, and natural killer (NK) cell therapies also deliver encouragement (1,4,5). However, off-target effects, immune escape, and drug resistance are still inevitable (6). The remission rate for high-risk refractory patients remains less than 35% (7). The prognosis of elder patients, who account for the majority of emerging cases, remains poor (8,9). One non-negligible reason is the presence of leukemic stem cells (LSCs) (10). As the origin of leukemia, LSCs are capable of self-renewal and differentiation. Most of them are in the quiescent phase and show abnormality in survival signaling pathways, which enable their escape from conventional chemotherapeutics that mainly target rapidly proliferating cells. Therefore, exploring LSC-targeting therapeutics that do not damage normal hematopoietic cells should be an effective strategy (11).
Limitations of current chemotherapy and pharmaceutical research are reflected in their narrow-mindedness of several already-known forms of cell death, such as apoptosis and ferroptosis, which hastens us to seek solutions in distinctive cell death pathways. Recently, Tsvetkov et al. demonstrated an exclusive mode of cell death, namely cuprotosis (copper-induced cell death) (12). Copper is essential for all organisms, but can be toxic when its concentration exceeds the threshold maintained by evolutionarily conserved internal homeostatic mechanisms (13). Tsvetkov et al. found that copper ions cause cell death by proteotoxic stress. Specifically, copper ions directly bind to lipoylation proteins in the tricarboxylic acid (TCA) cycle, leading to abnormal aggregation of lipoylation proteins and interference with iron-sulfur cluster proteins in the mitochondrial respiratory chain complex. In this process, genes involved in protein lipoylation such as FDX1, which are critical for mitochondrial aerobic metabolism, are key genes in promoting cuproptosis. Tsvetkov claimed that cells that rely on mitochondrial respiration were extremely more sensitive to copper ions than those that undergo glycolysis. Intriguingly, LSCs are characterized by an entire dependence on mitochondrial oxidative phosphorylation (OXPHOS) instead of glycolysis (14). Besides, Singh et al. have previously discovered the capacity of copper ions in regulating epigenetics and affecting differentiation in LSCs (15). Moreover, the treatment of LSCs with disulfiram (a copper ion carrier) in combination with copper ions renders them capable of complementing their respective drawbacks in terms of cancer-suppressing (16). A cohort study revealed a significant upregulation in the level of serum copper among leukemia patients as well (17). Nanoscale therapeutic anti-cancer agents that specifically target copper death have been demonstrated with promising outcomes in the management of bladder cancer (18). Thus, delving into the significance of cuproptosis in AML may delivering new insights into disease interpretation and blaze the trail for promising therapeutic options.
LncRNAs are transcripts of more than 200 bp in length possessing considerable portrayals on the map of oncology. Many studies confirmed lncRNA's pivotal role in cell differentiation and metabolism by forming complex secondary or tertiary structures. Published studies have endorsed the persuasiveness of lncRNAs in the prophecy of the prognosis of AML patients (19-22). For instance, deletion of lncRNA HOTTIP was found to inhibit AML cell proliferation by regulating hematopoietic chromatin and transcriptional program in an epigenetic manner (23). LncRNA HOXB-AS3 promotes the transcription of ribosomal RNA by binding to transcription factor EBP1, thus maintaining the malignant proliferation of AML cells (24). The TET2-WT1-lncRNA MEG3 signal transduction pathway has been reported as a main pathway to inhibit the progress of AML (25). The highly expressed lncRNA ANRIL and lncRNA HOTAIRM1 affect the proliferation of AML cells and the sensitivity to chemotherapy drugs by participating in metabolic pathways (26,27). Albeit extant reports on cuproptosis-associated lncRNAs in AML and other malignancies (28-30), their profiles in drug resistance and specific roles in AML remain poorly documented. To compensate for the above deficiencies, guide the imminent renewal of AML treatment and the precise stratification of AML sufferers, we made an earnest endeavor to draw up a list of practical prognostic factors intertwined with cuproptosis. We present the this in accordance with the TRIPOD reporting checklist (available at https://tcr.amegroups.com/article/view/10.21037/tcr-22-2526/rc).
Methods
Acquisition of lncRNAs related to cuproptosis
RNA-sequencing material of 151 AML patients (TCGA-LAML.htseq_fpkm, version 07-19-2019) and their matching clinical characteristics (TCGA-LAML.survival, version 07-19-2019 and TCGA-LAML.GDC_phenotype, version 08-07-2019) were retrieved in the University of California Santa Cruz (UCSC) database (31). The dataset used to validate the relationship between FAM30A and the prognosis of AML was obtained from Gene Expression Omnibus (GEO) cohort GSE12417-GPL96 and GSE114868. All patients enrolled were initially diagnosed by bone marrow biopsy. Expression volumes were processed by log2(x+1) and samples lacking survival time and survival status data were removed. An expression-survival data matrix consisting of 140 AML cases was operated. Genes relevant to cuproptosis were picked up in the study of Tsvetkov et al. (Table S1). Pearson correlation analysis was conducted on the expression data of lncRNAs and cuproptosis-related genes using the limma R package (threshold P value <0.001, correlation coefficient >0.4) to screen out cuproptosis-related lncRNAs.
Constructing a cuproptosis-related risk score (CuRS) system
The prognosis of patients was assessed by the length of overall survival (OS) incorporated in the file TCGA-LAML.survival, version 07-19-2019. The TCGA-LAML cohort was classified into a training set and a testing set through a thousand times of the caret R package random cycling. Between-set discrepancies were investigated by the chi-square test. Univariate Cox analysis was applied to select specific lncRNAs associated with OS (P<0.05) with the survival R package. The least absolute shrinkage and selection operator (LASSO) regression analysis was for the prevention of overfitting by the glnmet R package (32). After the final multivariate Cox analysis, our signature named CuRS was fabricated and the formula is:
By arranging the midpoint of the CuRS in the training set as borderline, each patient was endowed with a CuRS property and was assigned to the corresponding group of high- or low-risk.
Verifying the prognostic signature CuRS
Principal components analysis (PCA) plots and risk survival curves were worked out by the ggplot and pheatmap R packages, respectively. Kaplan-Meier curve analysis for revealing variation in OS was executed by the survminer R package. The survivalROC R package supported the conduction of the time-dependent receiver operating characteristic (ROC) curves and the calculation of the area under the ROC curve (AUC).
Nomogram and clinical correlation heatmap
A nomogram based on the CuRS signature was created for individualized prognosis prophecy by the usage of the rms R package. The corresponding calibration curves were designed for accuracy estimation. The heatmap of clinical correlation was performed by the limma R package and pheatmap R package.
Gene Set Enrichment Analysis (GSEA) and analysis in sensitivity of chemotherapeutics
Between-group divergence in biological pathways was investigated by GSEA software (version 4.2.1). Significant thresholds were considered as P<0.05, |normalized enrichment score (NES)| >1.5 and false discovery rate (FDR) <0.20. The CIBERSORT algorithm was used for the differential analysis of immune cells and immune-related processes. With the pRRophetic R package, individual sensitivity to chemotherapeutics in Genomics of Drug Sensitivity in Cancer (GDSC) was determined by their half-maximal inhibitory concentration (IC50).
Agents and antibodies
HRP Goat Anti-Rabbit IgG (H+L) (AS014), AUF1 Rabbit pAb (A15679), GAPDH Rabbit mAb (A19056), and LCK Rabbit pAb (A2177) were bought from Abclonal. Primary antibodies and secondary antibodies were diluted in QuickBlock™ Primary Antibody Dilution Buffer for Western Blot (Beyotime) and QuickBlock™ Secondary Antibody Dilution Buffer for Western Blot respectively (Beyotime).
Cell culture and RNAi
Leukemia cell lines including KG1a, K562, THP-1, and HL-60 stored at the Department of Hematology of Wuhan Union Hospital were selected for the subsequent experiments. KG1a cells were cultured in Roswell Park Memorial Institute (RPMI) 1640 (Gibco), 1% penicillin-streptomycin (Procell), and 20% fetal bovine serum (FBS) (Gibco) while K562 and THP-1 were in 10% FBS. HL-60 cells were fostered in Iscove’s Modified Dulbecco Medium (IMDM) (Gibco) with 1% penicillin-streptomycin and 10% FBS. All cell lines were offered an atmosphere of 37 ℃, 5% CO2.
Lentiviruses with short hairpin RNA (shRNA) sequences for FAM30A and empty vector lentiviruses expressing green fluorescent protein (GFP) were designed and synthesized by Genomeditech. KG1a cells were cultured until the logarithmic growth period and infected with lentivirus with polybrene at 5 µg/mL and multiplicity of infection (MOI) at 100. Cells with a stably knocked-down expression of FAM30A were used for subsequent experiments.
RNA extraction and quantification
This study was conducted in accordance with the Declaration of Helsinki (as revised in 2013). It was approved by the ethics committee of Wuhan Union Hospital [No. (2020) IEC-J (Jeny320)]. Written consent was obtained from patients for acquisition of all clinical samples. Clinical peripheral blood samples were obtained from three healthy controllers and unmedicated patients who presented to Wuhan Union Medical College Hospital in August 2022 with a primary diagnosis of AML. Fresh blood samples were equiproportionally diluted with phosphate buffered saline (PBS) (Gibco) and then added on top of an equal amount of Ficoll paque (cytiva). After centrifuging at 300×g for 20 minutes with no break, the cloudy layer between the Ficoll layer and the plasma layer, namely peripheral blood mononuclear cells (PBMCs), was aspirated using a pipette.
Cells were fully lysed by 1 mL of TRIzol (Takara) and shaken thoroughly after adding 200 µL of chloroform. The mixtures were centrifuged at 12,000×g for 20 min at 4 °C. The supernatant was extracted and an equal volume of isopropanol was added for the subsequent mixing. After centrifuging at 12,000×g for 15 min at 4 °C, the supernatant was discarded and the precipitate was washed twice with pre-cooled 75% ethanol and then dried at room temperature. Twenty µL of diethyl-pyrocarbonate-treated (DEPC) water (Beyotime) was added to dissolve the precipitate and 1 µL end product was used in the Nanodrop system to detect the purity and concentration of RNA. Real-time quantitative polymerase chain reaction (RT-qPCR) were carried out with the instructions of HiScript® III RT SuperMix for qPCR (+ gDNA wiper) (Vazyme) with Veriti 96 well Thermal Cycler (Applied Biosystems) and ChamQ Universal SYBR qPCR Master Mix (Vazyme) with 7500 Fast Real-Time PCR System (Applied Biosystems). Primers used: human β-ACTIN: F: AGCGAGCATCCCCCAAAGTT, R: GGGCACGAAGGCTCATCATT; human FAM30A: F: TGGCAAAGGCAAGTGAC, R: GGCAGAAGGATGAACCC.
Cell migration assays
Two hundred µL of serum-free cell suspension was added to the upper chamber of Transwell and 600 µL of complete medium was added to the lower chamber. After 36 h of incubation, the cells in the lower chambers were added with 60 µL CCK-8 solution and incubated at 37 ℃ for 60 min, 200 µL of which was transferred to a 96-well plate and the absorbance value at 450nm was read in the MicroplateReader.
Cell proliferation assays
The cells were calculated with a Bio-Rad cell counting plate and fabricated into cell suspensions (2×104/mL) with concentrations of Daunorubicin (DNR) at 8, 6, 4, 2, 0.8, 0.4, 0 µM, then inoculated into 96-well plates at 200 µL per well with 4 replicate wells. CCK-8 solution (20 µL/well) was added and incubated at 37 ℃ for 60 min. Subsequently, the absorbance value at 450 nm was measured with a MicroplateReader, and the growth curves were plotted based on the recorded values.
RNA fluorescence in situ hybridization (FISH)
RNA FISH was conducted with the FISH kit from GenePharma. Cy3-labeled FISH probes for FAM30A, 18S, and U6 were ordered from RiboBio. The cell suspension was dropped evenly onto a polylysine-treated slide (Boster Bio) and baked to dry on the flame of an alcohol lamp. Cells on the slides were permeabilized with buffer A, and the probes were diluted by buffer E and denatured at 73 ℃. Slides are spiked with a denatured probe mixture, protected from light, and placed in a 37 ℃ incubator overnight. After washing the slides with buffer F and buffer C, DAPI working solution was added dropwise to stain for 20 minutes. Slides were washed with PBS, then observed under a fluorescent microscope (OLYMPUS), and photographed.
RNA pull-down (RPD)
Briefly, cell lysates were incubated with streptavidin magnetic beads (MedChemExpress) and biotinylated probes for FAM30A or scramble probes (RiboBio). Part of the beads were incubated in the SDS-PAGE Sample Loading Buffer (2×, Beyotime) at 95 °C for 10 min for SDS PAGE silver stain with Fast Silver Stain Kit (Beyotime) and Western blot. The remaining magnetic beads were subjected to mass spectrometry detection.
Western blot
After quantification with Bicinchoninic Acid Protein Assay Kit (Pierce) and high-temperature denaturation, protein samples with the same concentration were added to the wells of FuturePAGE 4–20% performed gel (12 Wells, ACE) and electrophoresis was performed following the manufacturer’s instructions. A gel, a methanol-soaked PVDF membrane (Millipore), and filter papers were placed on the membrane transfer device in a reasonable order for transfer at a constant current in the transfer buffer (Sevicebio). After transfer, membrane blocking with QuickBlock™ Blocking Buffer for Western Blot (Beyotime) and incubation of primary and secondary antibodies were performed. Finally, the bands were observed by the Molecular Imager ChemiDoc XRS+ (BIORAD) after the addition of NcmECL Ultra Reagent A/B (NCM). ImageJ software favors the quantitative analysis of bands.
Mass spectrometry (MS) detection
The MS detection was performed by Novogene. In brief, protein denaturation, reduction, and alkylation were conducted by the incubation of magnetic beads with a reaction solution. An equal volume of water, and trypsin at a mass ratio of 1:50 enzyme to protein were added at 37 ℃ for overnight shaking for digestion. To terminate the digestion, trifluoroacetic acid (TFA) was added the next day. The supernatant was desalted by centrifugation at 16000 g then dried and stored at −20 ℃. Mass spectrometry data were gathered by the Q Exactive HF-X mass spectrometer in tandem with an EASY-nLC 1200 liquid phase LC system (Thermo Scientific).
RNA immunoprecipitation (RIP)
The RIP assay was conducted with the guidance of the RIP kit (BerSinBio). In brief, the RIP lysing buffer participated in the lysing of 2×107 cells. Lysates were incubated with 5µg primary antibody of AUF1 or Rabbit control IgG, together with protein A/G magnetic beads. The immunoprecipitated RNA is reverse transcribed and fluorescently quantified by the above-mentioned steps.
Transcriptomic analysis
Cell line samples from the knock-down and control groups were centrifuged and lysed in TRIZOL and delivered to the Beijing Genomics Institute for RNA-Seq (Quantification). SOAPnuke (v1.5.2) assisted in the filtering of the sequencing data. The subsequent analyses were performed on Dr. Tom Multi-omics Data mining system (https://biosys.bgi.com). The significance threshold of the differential genes was set to |log2 fold change (FC)| ≥0 and q<0.05. The outcome of Kyoto Encyclopedia of Genes and Genomes (KEGG) and Gene Ontology (GO) enrichment analysis was screened with q<0.05 and an enrichment number greater than 1. Protein-protein interaction networks (PPI) results were obtained by scoring the transcript mapping relationships using the STRING11 database and the National Center for Biotechnology Information (NCBI) Reference on a scale of 0 to 1000. The higher the score, the more accurate the PPI relationships are likely to be and the fewer the associated mRNAs.
Statistical analyses
R software (version 4.1.1) was applied in all bioinformatic statistical analyses with P<0.05 as acceptable unless otherwise stated. Data normalization to the fold change over the median of the control was carried out in the quantitative analysis of immunoblotting and mRNA expression to decrease benchmark discrepancy between separate experiments. Comparisons between the two groups were checked by a 2-tailed Student’s t-test. Statistical analyses of experimental data were conducted by Graphpad Prism 8.0.
Results
Construction of a prognostic signature related to cuproptosis
Figure 1A displays the flowchart of the bioinformatics research. A total of 140 AML cases with pathological diagnosis as AML from 2001 to 2010 were enrolled and their clinical characteristics are presented in Table 1. Two hundred and forty-four lncRNAs relevant to cuproptosis were initially identified. Patients’ survival time and survival status data with their lncRNA expression level information were combined into a matrix. One thousand times of caret package randomly assigned patients in the matrix to a training set with 72 cases and a testing set with 68 cases. Patients’ clinical characteristics in the two sets were matched and are shown in Table 2. Subsequently, 9 prognosis-associated lncRNAs (TRAF3IP2-AS1, MAN1B1-DT, EP300-AS1, PSMD6-AS2, NBR2, NADK2-AS1, TP53TG1, FAM30A, and PSMA3-AS1) were sorted by univariate Cox analysis. LASSO regression was utilized for the prevention of overfitting (Figure 1B,1C). Eventually, by multivariate Cox analysis, we developed a prognostic signature containing 4 lncRNAs named CuRS (Table 3). The formula is: CuRS = (−1.106242875) × expression level of TRAF3IP2-AS1 + (−0.214435146) × expression level of NBR2 + 0.414597295 × expression level of TP53TG1 + 0.035357611 × expression level of FAM30A. LncRNAs with a coefficient greater than zero (TP53TG1 and FAM30A) were risk-increasing factors and those less than zero (TRAF3IP2-AS1 and NBR2) were protective factors. The regulatory patterns of the CuRS lncRNAs with the cuproptosis-linked genes are exhibited in Table 4. Patients with CuRS greater than the median value of the training set were bestowed a high-risk property, and those otherwise were considered low-risk.
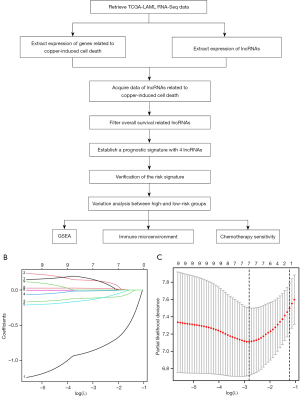
Table 1
Characteristics | N=140, n (%) |
---|---|
Set | |
Testing | 68 (48.6) |
Training | 72 (51.4) |
Cytogenetics risk category | |
Unknow | 2 (1.43) |
Favorable | 31 (22.1) |
Intermediate/normal | 76 (54.3) |
Poor | 31 (22.1) |
Age (years old) | |
<65 | 97 (69.3) |
≥65 | 43 (30.7) |
History of neoadjuvant treatment | |
No | 107 (76.4) |
Yes | 33 (23.6) |
FAB type | |
M0 undifferentiated | 14 (10.0) |
M1 | 30 (21.4) |
M2 | 34 (24.3) |
M3 | 15 (10.7) |
M4 | 28 (20.0) |
M5 | 15 (10.7) |
M6 | 2 (1.43) |
M7 | 1 (0.71) |
Not classified | 1 (0.71) |
Ethnicity | |
Hispanic or latino | 1 (0.71) |
Not hispanic or latino | 136 (97.1) |
Not reported | 3 (2.14) |
Gender | |
Female | 63 (45.0) |
Male | 77 (55.0) |
Survival state | |
Alive | 53 (37.9) |
Dead | 87 (62.1) |
TCGA-LAML, The Cancer Genome Atlas-Acute Myeloid Leukemia.
Table 2
Characteristics | Testing (N=68), n (%) | Training (N=72), n (%) | P value |
---|---|---|---|
Cytogenetics risk category | 0.780 | ||
Unknow | 1 (1.47) | 1 (1.39) | |
Favorable | 14 (20.6) | 17 (23.6) | |
Intermediate/normal | 40 (58.8) | 36 (50.0) | |
Poor | 13 (19.1) | 18 (25.0) | |
Age (years) | 0.091 | ||
<65 | 42 (61.8) | 55 (76.4) | |
≥65 | 26 (38.2) | 17 (23.6) | |
FAB type | 0.778 | ||
M0 undifferentiated | 6 (8.82) | 8 (11.1) | |
M1 | 16 (23.5) | 14 (19.4) | |
M2 | 15 (22.1) | 19 (26.4) | |
M3 | 9 (13.2) | 6 (8.33) | |
M4 | 11 (16.2) | 17 (23.6) | |
M5 | 9 (13.2) | 6 (8.33) | |
M6 | 1 (1.47) | 1 (1.39) | |
M7 | 0 (0.00) | 1 (1.39) | |
Not classified | 1 (1.47) | 0 (0.00) | |
Ethnicity | 0.802 | ||
Hispanic or latino | 0 (0.00) | 1 (1.39) | |
Not hispanic or latino | 66 (97.1) | 70 (97.2) | |
Not reported | 2 (2.94) | 1 (1.39) | |
Gender | 0.185 | ||
Female | 35 (51.5) | 28 (38.9) | |
Male | 33 (48.5) | 44 (61.1) | |
Survival state | 0.258 | ||
Alive | 22 (32.4) | 31 (43.1) | |
Dead | 46 (67.6) | 41 (56.9) |
Table 3
LncRNA | Coefficient | HR | 95% CI | P value |
---|---|---|---|---|
TRAF3IP2-AS1 | −1.10624 | 0.330799 | 0.145099–0.754164 | 0.008513 |
NBR2 | −0.21444 | 0.806997 | 0.612119–1.063917 | 0.128358 |
TP53TG1 | 0.414597 | 1.513761 | 1.039186–2.205065 | 0.030754 |
FAM30A | 0.035358 | 1.03599 | 1.017854–1.05445 | 8.72E-05 |
HR value greater than one indicates that the lncRNA is a risk-increasing factor. lncRNA, long non-coding RNA; HR, hazard ratio; CI, confidence interval.
Table 4
Cuproptosis gene | LncRNA | Correlation coefficient | P value | Regulation |
---|---|---|---|---|
SLC31A1 | TRAF3IP2-AS1 | −0.52124 | 6.81E-12 | Negative |
SLC31A1 | NBR2 | −0.42555 | 5.12E-08 | Negative |
GCSH | TP53TG1 | 0.409028 | 1.84E-07 | Positive |
PDHB | FAM30A | −0.40661 | 2.21E-07 | Negative |
lncRNAs, long non-coding RNAs.
Verification of the accuracy and independence of the prognostic signature
PCA plots approved the above division of high- and low-risk sets (Figure 2A,2B). The risk diagrams presented that the CuRS and the survival time, the number of survivors were inversely related (Figure 2C,2D). Kaplan-Meier analyses confirmed the high-risk population with significantly poorer prognosis manifested by shorter OS time (Figure 2E,2F). In the training set, the AUC of the 1-year, 3-year, and 5-year predicted OS was 0.833, 0.833, 0.943, while in the testing set, was correspondingly 0.756, 0.733, 0.779. AUC values greater than 0.7 rendered the CuRS signature plausible (Figure 2G,2H). The autonomy of age, cytogenetic risk, and CuRS in prognostic provision were affirmed through univariate and multivariate Cox analysis (Figure S1). As the factor with the largest AUC in the combined ROC curve, the persuasive power of our CuRS signature was certified once again (Figure 2I).
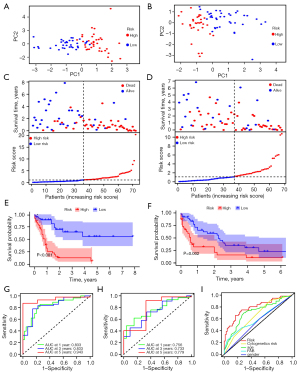
Nomogram and clinical correlation
A nomogram was fabricated to render the CuRS signature credible in personalized prognostication (C-index =0.7203595) (Figure 3A). The association between higher expression of TRAF3IP2-AS1 and NBR2 and the better prognosis was confirmed again, while the opposite effect was present in TP53TG1 and FAM30A. The calibration curve plots approved the accountability of the CuRS signature for 1-, 3-, and 5-year forecasting of chances of survival (Figure 3B-3D). By ranking each individual in the cohort by risk score, we plotted a heatmap of the association between CuRS lncRNA expression and each clinical trait (Figure S2). It can be seen that as the CuRS increases, the expression of TP53TG1 and FAM30A was heightened, while the opposite is true for TRAF3IP2-AS1 and NBR2. We also found significant distinctions in CALGB cytogenetics risk, age and FAB type of patients between different risk groups.
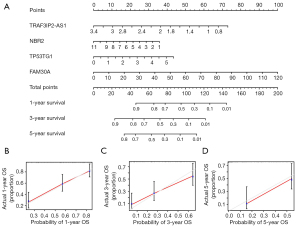
Biological significance research
We performed a GSEA analysis to explore the biological significance of this signature and noticed that the natural killer (NK) cell-mediated cytotoxicity pathway and ATP-binding cassette (ABC) transporters pathway were enriched in the high-risk group (Table S2). Variations in NK cell toxicity prompted us to conduct studies on the association of this model with the immune environment. Through the CIBERSORT algorithm, we found that mast cells resting showed a relatively higher content in low-risk patients (Figure 4A,4B). Analysis of immune function also validated the discrepancy related to the immune environment (Figure 4C,4D). We also examined the between-group divergence in immune checkpoint genes and found relatively intense expression of CTLA4, CD276, TNFSF15, PDCD1, TNFRSF8, and TNFRSF9 in the high-risk group (Figure 4E,4F), which reflects that patients in different risk groups may react differently to immune checkpoint inhibitor therapy. ABC transporter proteins have been adopted as multidrug resistance inhibition targets in oncology clinical treatment because of their capacity to excrete tumor therapeutic drugs from tumor cells (33). Therefore, we speculated on the existence of between-group disparity in the sensitivity of chemotherapeutic drugs and conducted a drug sensitivity assessment. The results indicated higher application values of 12 kinds of drugs including BMS.536924, Bortezomib, CGP.60474, CGP.082996, JW.7.52.1, KIN001.135, MG.132, NVP.TAE684, Paclitaxel, Rapamycin, Roscovitine, WZ.1.84 in high-risk groups, and 18 kinds of drugs with higher sensitivity in the low-risk group (Table 5).
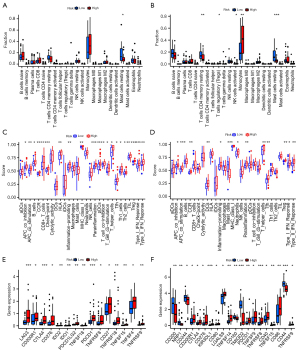
Table 5
Chemotherapy with higher sensitivity | P value |
---|---|
High-risk group | |
BMS.536924 | 4.30E-06 |
Bortezomib | 4.60E-06 |
CGP.60474 | 3.40E-04 |
CGP.082996 | 4.40E-06 |
JW.7.52.1 | 2.30E-05 |
KIN001.135 | 1.00E-04 |
MG.132 | 2.10E-05 |
NVP.TAE684 | 4.60E-04 |
Paclitaxel | 2.50E-04 |
Rapamycin | 3.60E-06 |
Roscovitine | 7.80E-08 |
WZ.1.84 | 7.20E-06 |
Low-risk group | |
ABT.263 | 1.90E-12 |
AKT.inhibitor.VIII | 2.40E-05 |
AP.24534 | 6.00E-06 |
AZD.2281 | 8.50E-04 |
AZD7762 | 2.30E-05 |
BIBW2992 | 9.50E-07 |
BX.795 | 1.80E-08 |
CCT007093 | 1.80E-09 |
CCT018159 | 9.50E-07 |
GDC0941 | 8.90E-10 |
Gefitinib | 1.40E-04 |
JNJ.26854165 | 2.10E-04 |
Midostaurin | 4.80E-04 |
SB.216763 | 1.10E-05 |
TW.37 | 1.50E-05 |
Vorinostat | 2.00E-05 |
VX.702 | 1.40E-07 |
ZM.447439 | 7.70E-05 |
Exploration of the biological function of FAM30A
Survival analysis by the Gene Expression Profiling Interactive Analysis (GEPIA) website and the GEO cohort GSE12417-GPL96 revealed the significance of FAM30A in prognostic forecasting (Figure 5A-5C). A comparison of bone marrow mononuclear cells of AML patients and disease-free controls in the GSE114868 population detected remarkable variation in FAM30A expression (Figure 5D). The RT-qPCR analysis of peripheral blood samples from our collection of three primary cases of AML and two healthy individuals demonstrated that AML patients presented with significantly higher expression levels of FAM30A (Figure 5E). KG1a cell line, well known as LSCs, were found to express the highest level of FAM30A among the cell lines we selected (Figure 5F). Therefore, the knockdown of FAM30A and the subsequent in vitro and in vivo experiments were performed on KG1a cells. Through RNA FISH, we found that FAM30A was mainly expressed in the cytoplasm (Figure S3). Given the property that lncRNAs often function in combination with proteins, we entered the full-length sequence of FAM30A in the catRAPID database for prediction and obtained a bunch of results. Thus, we performed RPD experiments and examined the beads by mass spectrometry. Several proteins that diverged most referring to the value of |log2FC| between the probe group and the NC group were selected for validation. Western blot results suggested the combination of AUF1 and FAM30A, which was subsequently proved by RIP experiments (Figure S4, Figure 5G-5I). No changes in RNA expression of AUF1 were found by RT-qPCR performed on the knock-down and the natural control groups prompting a physical combination. However, the knock-down group was characterized by poorer migration ability, slower proliferation rate and higher sensitivity to DNR (Figure 5J-5L).
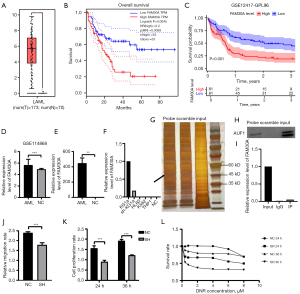
Furthermore, we carried out a transcriptomic analysis of empty vector-infected and shRNA-sequence-containing lentivirus-infected KG1a cells and identified 54 differential genes among the two groups, including 23 upregulated genes and 31 downregulated genes in the knock-down group (Figure 6A). Consistent with the outcome of KEGG enrichment analysis (namely the enrichment of differential genes in Th1 cell, Th2 cell, Th17 cell differentiation, and T cell receptor signaling), GO enrichment analysis illustrated their engagement in the T cell receptor complex, and its binding, differentiation, and signaling (Figure 6B-6E). The involvement of differential genes in PD-L1 expression and PD-1 checkpoint pathway demonstrated the possibility of its participation in cancer immune therapy (34). PPI network interaction analysis identified a group of highly correlated genes, of which LCK featured the maximum number of connections (Figure 6F). The validation of the differential expression of LCK (Lymphocyte Cell-Specific Protein-Tyrosine Kinase) was performed through a Western blot (Figure S5).
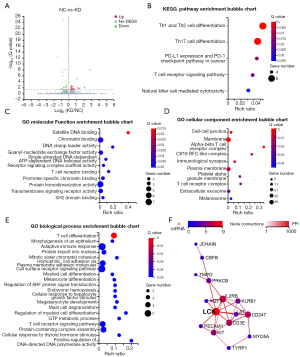
Discussion
Great progress has been achieved in the treatment of AML in recent years. For AML patients able to accept intensive treatments, cytarabine for seven days and anthracyclines for three days (the “7+3” regimen) in combination with sequential allogeneic hematopoietic stem cell transplantation is still the primary choice. Small molecule targeted drugs, antibody-based drugs, CAR-T therapy, or NK cell therapy in combination with traditional cytotoxic regimens generate more opportunities for transplantation for all AML sufferers, especially those who cannot endure high-dose chemotherapy. However, drug resistance and relapse always bother clinical disposition, mostly due to the presence of CD34+CD38−CD123+ LSCs that cannot be cleared by chemotherapy (35). This phenomenon can attribute to their mostly being in the quiescence of the cell cycle, enhanced DNA repair and scavenging of reactive oxygen species (ROS), and the prioritized expression of multiple drug-resistant proteins, such as P-glycoprotein and BCL2 (36). Phenotypes of LSCs distinct from hematopoietic stem cells (HSCs) and bulk cancer cells render the exploitation of LSCs-targeted treatments feasible. As is known to all, the linkage of the TCA cycle and OXPHOS is indispensable for the production of ATPs in cells. The purpose of the ‘reverse Warburg effect’ enabled us to be aware of the more intense reliance of LSCs on mitochondrial respiration instead of glycolysis compared to mature cancer cells (14,37,38). By depletion of glucose, Jones et al. found out that LSCs from de novo AML patients survive on amino acid-promoted OXPHOS (39). Multiple works have demonstrated the practicability of attacking the mitochondrial OXPHOS pathway to restrain LSCs as well (40,41). Tsvetkov et al. also emphasized the indispensable contribution of mitochondrial respiration in cuproptosis by increased lipoylated TCA enzymes in TCA-cycle rushing cells (12). The integration of copper ions and lipoylated components in the TCA cycle results in the aggregation of the lipoylated protein, missing Fe-S cluster-containing proteins, intense proteotoxic stress, and eventually cell death. Coadministration of copper ions and their vehicles has demonstrated a favorable cancer suppressive effect in in vivo and in vitro trials (16). Thus, it is reasonable to inquire into the relationship between cuproptosis and AML.
In our study, we initially broke the TCGA-LAML cohort into a training set and a testing set randomly with no significant difference in clinical tendencies. A risk-scoring signature CuRS containing four cuproptosis-related lncRNAs (TRAF3IP2-AS1, NBR2, TP53TG1, and FAM30A) was created. The effectiveness of the signature in prognostication was demonstrated by PCA analysis, Kaplan-Meier survival analysis, and ROC curves. Its prognostic autonomy was authenticated by univariate and multivariate Cox analysis and combined ROC curves. To further investigate the biological connotation of CuRS, we executed a GSEA analysis on KEGG pathways. The NK cell-mediated cytotoxicity pathway was enriched in the high-risk group, implying the relevance of the CuRS signature and the immune environment of AML. The CIBERSORT is an analytical algorithm that assesses the relative plenty of each cell type, by which we found that resting mast cells showed a relatively elevated abundance in the high-risk group. Almost universally enhanced immune-related processes in the high-risk populations were detected as well. The specific mechanism of cuproptosis in the AML immune environment requires further exploration. Since the ABC transporter pathway enriched in the high-risk group was highly corroborated with the chemoresistance of cancer cells, we undertook a variational analysis of chemotherapy sensitivity in populations with diverse risk properties and obtained promising results, which serves as a basis for the subsequent application of this signature in directing individualized regimens. Compared to other prognostic signatures, our CuRS system delivers a refreshing approach for risk profiling and demonstrates sound predictive efficacy.
With the continuous research on lncRNAs, more and more lncRNAs have been discovered in hematological cancers, and their mechanisms of action are gradually being explored. LncRNAs play important roles as signaling molecules, decoy molecules, guidance molecules, or scaffolding molecules in the occurrence, development, and prognosis of AML, and also offer fresh ideas for clinical diagnosis and treatment (42). TRAF3IP2-AS1 was previously screened as a protective lncRNA associated with N6-methyladenosine in AML and a related pair with SRSF10 that has been regarded as an appealing target of anti-cancer therapeutic, such as hepatocellular carcinoma, rectal cancer, head and neck cancer (43-45). NBR2 is also a regulator in multiple cancers and has been suggested to be involved in cancer cell sensitivity to some therapeutics such as biguanides (which has been documented to exhibit amelioration of chemo-resistance in AML) (46-49). In our study, TRAF3IP2-AS1 and NBR2 were regarded to be risk-decreasing and negatively related to SLC31A1 (also known as copper importer CTR1). TP53TG1, namely tumor protein 53 target gene 1, is a risk-increasing factor and has been proven to be correlated with the etiology of various cancers (50-52). Albeit the role of p53 as a therapeutic target, functions of TP53TG1 in AML have not been researched yet (53). FAM30A, also named lncRNA KIAA0125, has been selected as a risk-raising element in AML and was assumed to be negatively related to PDHB according to our research (54). A relatively higher expression level of FAM30A in B cells is positively correlated with after-vaccination antibody levels, recommending that FAM30A is engaged in human immune-associated events (55). We carried out separate survival analyses of four lncRNAs in the GEPIA database and the results implied that FAM30A seems to be capable of impacting prognosis autonomously. Wang et al. have portrayed the distinctive properties of AML sufferers with higher expression of lncRNA FAM30A (56). By mediation analysis, Hornung et al. announced FAM30A associated with t [8; 21] and RUNX1 mutation (57). FAM30A was also utilized as a risk contributor in a 17-gene stemness scoring signature for ascertaining the risk of AML patients (58). In our research, elevated expression of FAM30A was found in the KG1a cell line, which has been generally applied as LSCs in previous research. Further, we identified the combination of FAM30A and AUF1. AUF1, namely heterogeneous nuclear ribonucleoprotein D (hnRNP D), is the first purified and cloned AU-rich element-binding protein (ARE-BP) with four types of isoforms (p45, p42, p40, and p37). Researchers have found that ARE-like sequences are present in as many as 5% to 8% of genes (59). ARE-BPs such as AUF1 recruit and incorporate AREs at the 3'-untranslated regions of mRNAs, thereby positively or negatively modulating their degradation or translation (60,61). The versatile characteristics of AUF1 have been documented in various cancers (62-65). The mRNAs affected by AUF1 include regulators in the cell cycle, apoptosis, metastasis, inflammation, DNA repair and replication (66-74). AUF1 was documented to be involved in the translational regulation of the MYC mRNA in two leukemia cell lines (K562 and THP1) (66) and in connection with the stability of BCL2 mRNA in MV-4-11 cells (75). The biological significance of its binding to FAM30A in KG1a cells still requires more experimental explorations.
Through the knockdown of FAM30A, we found alterations in proliferation, migration, and drug resistance. Subsequent transcriptomic studies revealed remarkable enrichment of differential genes in T cell differentiation and signaling. LCK, an Src family tyrosine kinase, was screened as a focal point (76,77). Mutations and overexpression of LCK were previously observed to be capable of driving the proliferation of an AML cell line (78). In a high-risk AML type, i.e., patients with coexistence of NUP98-NSD1 and FLT3-ITD, a relatively higher expression of LCK was found (79). Its relationship with CEBPA has been demonstrated as well (80). Notably, one of its transcripts showed a markable increase in the immature AML type (M0 and M1) (81). We hypothesize that the high presence of LSCs may result in a relative increase in FAM30A expression, which in turn elevates LCK expression and ultimately increases the malignancy of leukemia. In the GO enrichment analysis of cellular components, we discovered significantly varied genes in the cell-cell junction. Thus, we propose that the upregulated expression of FAM30A in LSCs enhances its crosstalk with the bone marrow microenvironment by increasing the expression of intercellular molecules, which may subsequently lead to the occurrence of immune escape (82,83).
However, there are remaining imperfections in our study. We only trained and validated the CuRS signature in the TCGA-LAML cohort. The consequential effects of the conjugation of FAM30A with AUF1 have not been well investigated. Our hypothesis based on transcriptomic evidence still demands further in vivo and in vitro explorations and validation.
Conclusions
Above all, our CuRS signature related to cuproptosis and the immune environment sheds light on risk stratification for AML. The research of between-group divergences in chemotherapeutic drug sensitivity puts forward innovative personalized treatment ideas. In particular, we confirmed that the expression of FAM30A solely impacts AML prognosis. The binding of FAM30A to AUF1 was discovered and illustrated for the first time.
Acknowledgments
Funding: This research was funded by
Footnote
Reporting Checklist: The authors have completed the TRIPOD reporting checklist. Available at https://tcr.amegroups.com/article/view/10.21037/tcr-22-2526/rc
Data Sharing Statement: Available at https://tcr.amegroups.com/article/view/10.21037/tcr-22-2526/dss
Conflicts of Interest: All authors have completed the ICMJE uniform disclosure form (available at https://tcr.amegroups.com/article/view/10.21037/tcr-22-2526/coif). The authors report that this research was funded by the National Natural Science Foundation of China (No. 82000141 to DL and No. 31620103909 to YH). The authors have no other conflicts of interest to declare.
Ethical Statement: The authors are accountable for all aspects of the work in ensuring that questions related to the accuracy or integrity of any part of the work are appropriately investigated and resolved. This study was conducted in accordance with the Declaration of Helsinki (as revised in 2013). It was approved by the ethics committee of Wuhan Union Hospital [No. (2020) IEC-J (320)]. Written consent was obtained from patients for acquisition of all clinical samples.
Open Access Statement: This is an Open Access article distributed in accordance with the Creative Commons Attribution-NonCommercial-NoDerivs 4.0 International License (CC BY-NC-ND 4.0), which permits the non-commercial replication and distribution of the article with the strict proviso that no changes or edits are made and the original work is properly cited (including links to both the formal publication through the relevant DOI and the license). See: https://creativecommons.org/licenses/by-nc-nd/4.0/.
References
- Newell LF, Cook RJ. Advances in acute myeloid leukemia. BMJ 2021;375: [PubMed]
- Deschler B, Lübbert M. Acute myeloid leukemia: epidemiology and etiology. Cancer 2006;107:2099-107. [Crossref] [PubMed]
- Liu H. Emerging agents and regimens for AML. J Hematol Oncol 2021;14:49. [Crossref] [PubMed]
- Marofi F, Rahman HS, Al-Obaidi ZMJ, et al. Novel CAR T therapy is a ray of hope in the treatment of seriously ill AML patients. Stem Cell Res Ther 2021;12:465. [Crossref] [PubMed]
- Gurney M, O'Dwyer M. Realizing Innate Potential: CAR-NK Cell Therapies for Acute Myeloid Leukemia. Cancers (Basel) 2021;13:1568. [Crossref] [PubMed]
- Estey EH. Acute myeloid leukemia: 2021 update on risk-stratification and management. Am J Hematol 2020;95:1368-98. [Crossref] [PubMed]
- Breems DA, Van Putten WL, Huijgens PC, et al. Prognostic index for adult patients with acute myeloid leukemia in first relapse. J Clin Oncol 2005;23:1969-78. [Crossref] [PubMed]
- Lancet JE. Is the overall survival for older adults with AML finally improving? Best Pract Res Clin Haematol 2018;31:387-90. [Crossref] [PubMed]
- National Cancer Institute Surveillance E, and End Results Program. Cancer Stat Facts: Leukemia — Acute Myeloid Leukemia (AML). Available online: https://seer.cancer.gov/statfacts/html/amyl.html
- Vetrie D, Helgason GV, Copland M. The leukaemia stem cell: similarities, differences and clinical prospects in CML and AML. Nat Rev Cancer 2020;20:158-73. [Crossref] [PubMed]
- Mitchell K, Steidl U. Targeting Immunophenotypic Markers on Leukemic Stem Cells: How Lessons from Current Approaches and Advances in the Leukemia Stem Cell (LSC) Model Can Inform Better Strategies for Treating Acute Myeloid Leukemia (AML). Cold Spring Harb Perspect Med 2020;10:a036251. [Crossref] [PubMed]
- Tsvetkov P, Coy S, Petrova B, et al. Copper induces cell death by targeting lipoylated TCA cycle proteins. Science 2022;375:1254-61. [Crossref] [PubMed]
- Ge EJ, Bush AI, Casini A, et al. Connecting copper and cancer: from transition metal signalling to metalloplasia. Nat Rev Cancer 2022;22:102-13. [Crossref] [PubMed]
- Lagadinou ED, Sach A, Callahan K, et al. BCL-2 inhibition targets oxidative phosphorylation and selectively eradicates quiescent human leukemia stem cells. Cell Stem Cell 2013;12:329-41. [Crossref] [PubMed]
- Singh RP, Jeyaraju DV, Voisin V, et al. Disrupting Mitochondrial Copper Distribution Inhibits Leukemic Stem Cell Self-Renewal. Cell Stem Cell 2020;26:926-937.e10. [Crossref] [PubMed]
- Xu B, Wang S, Li R, et al. Disulfiram/copper selectively eradicates AML leukemia stem cells in vitro and in vivo by simultaneous induction of ROS-JNK and inhibition of NF-κB and Nrf2. Cell Death Dis 2017;8:e2797. [Crossref] [PubMed]
- Valadbeigi S, Javadian S, Ebrahimi-Rad M, et al. Assessment of trace elements in serum of acute lymphoblastic and myeloid leukemia patients. Exp Oncol 2019;41:69-71. [PubMed]
- Xu Y, Liu SY, Zeng L, et al. An Enzyme-Engineered Nonporous Copper(I) Coordination Polymer Nanoplatform for Cuproptosis-Based Synergistic Cancer Therapy. Adv Mater 2022;34:e2204733. [Crossref] [PubMed]
- Liu Y, Cheng Z, Pang Y, et al. Role of microRNAs, circRNAs and long noncoding RNAs in acute myeloid leukemia. J Hematol Oncol 2019;12:51. [Crossref] [PubMed]
- Kirtonia A, Ashrafizadeh M, Zarrabi A, et al. Long noncoding RNAs: A novel insight in the leukemogenesis and drug resistance in acute myeloid leukemia. J Cell Physiol 2022;237:450-65. [Crossref] [PubMed]
- Gasic V, Karan-Djurasevic T, Pavlovic D, et al. Diagnostic and Therapeutic Implications of Long Non-Coding RNAs in Leukemia. Life (Basel) 2022;12:1770. [Crossref] [PubMed]
- Wurm AA, Pina C. Long Non-coding RNAs as Functional and Structural Chromatin Modulators in Acute Myeloid Leukemia. Front Oncol 2019;9:899. [Crossref] [PubMed]
- Luo H, Zhu G, Xu J, et al. HOTTIP lncRNA Promotes Hematopoietic Stem Cell Self-Renewal Leading to AML-like Disease in Mice. Cancer Cell 2019;36:645-659.e8. [Crossref] [PubMed]
- Papaioannou D, Petri A, Dovey OM, et al. The long non-coding RNA HOXB-AS3 regulates ribosomal RNA transcription in NPM1-mutated acute myeloid leukemia. Nat Commun 2019;10:5351. [Crossref] [PubMed]
- Lyu Y, Lou J, Yang Y, et al. Dysfunction of the WT1-MEG3 signaling promotes AML leukemogenesis via p53-dependent and -independent pathways. Leukemia 2017;31:2543-51. [Crossref] [PubMed]
- Sun LY, Li XJ, Sun YM, et al. LncRNA ANRIL regulates AML development through modulating the glucose metabolism pathway of AdipoR1/AMPK/SIRT1. Mol Cancer 2018;17:127. [Crossref] [PubMed]
- Chen L, Hu N, Wang C, et al. HOTAIRM1 knockdown enhances cytarabine-induced cytotoxicity by suppression of glycolysis through the Wnt/β-catenin/PFKP pathway in acute myeloid leukemia cells. Arch Biochem Biophys 2020;680:108244. [Crossref] [PubMed]
- Li D, Wu X, Fan X, et al. Comprehensive analysis of cuproptosis-related lncRNAs in the prognosis and therapy response of patients with bladder cancer. Ann Transl Med 2022;10:1232. [Crossref] [PubMed]
- Li P, Li J, Wen F, et al. A novel cuproptosis-related LncRNA signature: Prognostic and therapeutic value for acute myeloid leukemia. Front Oncol 2022;12:966920. [Crossref] [PubMed]
- Sha S, Si L, Wu X, et al. Prognostic analysis of cuproptosis-related gene in triple-negative breast cancer. Front Immunol 2022;13:922780. [Crossref] [PubMed]
- Navarro Gonzalez J, Zweig AS, Speir ML, et al. The UCSC Genome Browser database: 2021 update. Nucleic Acids Res 2021;49:D1046-57. [Crossref] [PubMed]
- Duan J, Soussen C, Brie D, et al. Generalized LASSO with under-determined regularization matrices. Signal Processing 2016;127:239-46. [Crossref] [PubMed]
- Schinkel AH, Jonker JW. Mammalian drug efflux transporters of the ATP binding cassette (ABC) family: an overview. Adv Drug Deliv Rev 2003;55:3-29. [Crossref] [PubMed]
- Dermani FK, Samadi P, Rahmani G, et al. PD-1/PD-L1 immune checkpoint: Potential target for cancer therapy. J Cell Physiol 2019;234:1313-25. [Crossref] [PubMed]
- Bencomo-Alvarez AE, Rubio AJ, Gonzalez MA, et al. Energy metabolism and drug response in myeloid leukaemic stem cells. Br J Haematol 2019;186:524-37. [Crossref] [PubMed]
- Lytle NK, Barber AG, Reya T. Stem cell fate in cancer growth, progression and therapy resistance. Nat Rev Cancer 2018;18:669-80. [Crossref] [PubMed]
- Kuntz EM, Baquero P, Michie AM, et al. Targeting mitochondrial oxidative phosphorylation eradicates therapy-resistant chronic myeloid leukemia stem cells. Nat Med 2017;23:1234-40. [Crossref] [PubMed]
- Fu Y, Liu S, Yin S, et al. The reverse Warburg effect is likely to be an Achilles' heel of cancer that can be exploited for cancer therapy. Oncotarget 2017;8:57813-25. [Crossref] [PubMed]
- Jones CL, Stevens BM, D'Alessandro A, et al. Inhibition of Amino Acid Metabolism Selectively Targets Human Leukemia Stem Cells. Cancer Cell 2018;34:724-740.e4. [Crossref] [PubMed]
- Skrtić M, Sriskanthadevan S, Jhas B, et al. Inhibition of mitochondrial translation as a therapeutic strategy for human acute myeloid leukemia. Cancer Cell 2011;20:674-88. [Crossref] [PubMed]
- Molina JR, Sun Y, Protopopova M, et al. An inhibitor of oxidative phosphorylation exploits cancer vulnerability. Nat Med 2018;24:1036-46. [Crossref] [PubMed]
- Bhat AA, Younes SN, Raza SS, et al. Role of non-coding RNA networks in leukemia progression, metastasis and drug resistance. Mol Cancer 2020;19:57. [Crossref] [PubMed]
- Zhong F, Yao F, Cheng Y, et al. m6A-related lncRNAs predict prognosis and indicate immune microenvironment in acute myeloid leukemia. Sci Rep 2022;12:1759. [Crossref] [PubMed]
- Yang L, Chen Y, Liu N, et al. Low expression of TRAF3IP2-AS1 promotes progression of NONO-TFE3 translocation renal cell carcinoma by stimulating N(6)-methyladenosine of PARP1 mRNA and downregulating PTEN. J Hematol Oncol 2021;14:46. [Crossref] [PubMed]
- He R, Wu S, Gao R, et al. Identification of a Long Noncoding RNA TRAF3IP2-AS1 as Key Regulator of IL-17 Signaling through the SRSF10-IRF1-Act1 Axis in Autoimmune Diseases. J Immunol 2021;206:2353-65. [Crossref] [PubMed]
- Liu X, Gan B. lncRNA NBR2 modulates cancer cell sensitivity to phenformin through GLUT1. Cell Cycle 2016;15:3471-81. [Crossref] [PubMed]
- Yu H, Xie Y, Zhou Z, et al. Curcumin Regulates the Progression of Colorectal Cancer via LncRNA NBR2/AMPK Pathway. Technol Cancer Res Treat 2019;18:1533033819870781. [Crossref] [PubMed]
- Yuan F, Cheng C, Xiao F, et al. Inhibition of mTORC1/P70S6K pathway by Metformin synergistically sensitizes Acute Myeloid Leukemia to Ara-C. Life Sci 2020;243:117276. [Crossref] [PubMed]
- You R, Wang B, Chen P, et al. Metformin sensitizes AML cells to chemotherapy through blocking mitochondrial transfer from stromal cells to AML cells. Cancer Lett 2022;532:215582. [Crossref] [PubMed]
- Chen B, Lan J, Xiao Y, et al. Long noncoding RNA TP53TG1 suppresses the growth and metastasis of hepatocellular carcinoma by regulating the PRDX4/β-catenin pathway. Cancer Lett 2021;513:75-89. [Crossref] [PubMed]
- Gao W, Qiao M, Luo K. Long Noncoding RNA TP53TG1 Contributes to Radioresistance of Glioma Cells Via miR-524-5p/RAB5A Axis. Cancer Biother Radiopharm 2021;36:600-12. [Crossref] [PubMed]
- Xiao H, Liu Y, Liang P, et al. TP53TG1 enhances cisplatin sensitivity of non-small cell lung cancer cells through regulating miR-18a/PTEN axis. Cell Biosci 2018;8:23. [Crossref] [PubMed]
- Prokocimer M, Molchadsky A, Rotter V. Dysfunctional diversity of p53 proteins in adult acute myeloid leukemia: projections on diagnostic workup and therapy. Blood 2017;130:699-712. [Crossref] [PubMed]
- Guo C, Gao YY, Ju QQ, et al. The landscape of gene co-expression modules correlating with prognostic genetic abnormalities in AML. J Transl Med 2021;19:228. [Crossref] [PubMed]
- de Lima DS, Cardozo LE, Maracaja-Coutinho V, et al. Long noncoding RNAs are involved in multiple immunological pathways in response to vaccination. Proc Natl Acad Sci U S A 2019;116:17121-6. [Crossref] [PubMed]
- Wang YH, Lin CC, Hsu CL, et al. Distinct clinical and biological characteristics of acute myeloid leukemia with higher expression of long noncoding RNA KIAA0125. Ann Hematol 2021;100:487-98. [Crossref] [PubMed]
- Hornung R, Jurinovic V, Batcha AMN, et al. Mediation analysis reveals common mechanisms of RUNX1 point mutations and RUNX1/RUNX1T1 fusions influencing survival of patients with acute myeloid leukemia. Sci Rep 2018;8:11293. [Crossref] [PubMed]
- Ng SW, Mitchell A, Kennedy JA, et al. A 17-gene stemness score for rapid determination of risk in acute leukaemia. Nature 2016;540:433-7. [Crossref] [PubMed]
- Bakheet T, Williams BR, Khabar KS. ARED 3.0: the large and diverse AU-rich transcriptome. Nucleic Acids Res 2006;34:D111-4. [Crossref] [PubMed]
- White EJ, Matsangos AE, Wilson GM. AUF1 regulation of coding and noncoding RNA. Wiley Interdiscip Rev RNA 2017; [Crossref] [PubMed]
- Barreau C, Paillard L, Osborne HB. AU-rich elements and associated factors: are there unifying principles? Nucleic Acids Res 2006;33:7138-50. [Crossref] [PubMed]
- Tsitsipatis D, Grammatikakis I, Driscoll RK, et al. AUF1 ligand circPCNX reduces cell proliferation by competing with p21 mRNA to increase p21 production. Nucleic Acids Res 2021;49:1631-46. [Crossref] [PubMed]
- Al-Tweigeri T, AlRaouji NN, Tulbah A, et al. High AUF1 level in stromal fibroblasts promotes carcinogenesis and chemoresistance and predicts unfavorable prognosis among locally advanced breast cancer patients. Breast Cancer Res 2022;24:46. [Crossref] [PubMed]
- AlAhmari MM, Al-Khalaf HH, Al-Mohanna FH, et al. AUF1 promotes stemness in human mammary epithelial cells through stabilization of the EMT transcription factors TWIST1 and SNAIL1. Oncogenesis 2020;9:70. [Crossref] [PubMed]
- Zhang Z, Fan W, Gao Q, et al. Hsa_Circ_0000826 inhibits the proliferation of colorectal cancer by targeting AUF1. J Genet Genomics 2023;50:192-203. [Crossref] [PubMed]
- Liao B, Hu Y, Brewer G. Competitive binding of AUF1 and TIAR to MYC mRNA controls its translation. Nat Struct Mol Biol 2007;14:511-8. [Crossref] [PubMed]
- Wang W, Martindale JL, Yang X, et al. Increased stability of the p16 mRNA with replicative senescence. EMBO Rep 2005;6:158-64. [Crossref] [PubMed]
- Lal A, Mazan-Mamczarz K, Kawai T, et al. Concurrent versus individual binding of HuR and AUF1 to common labile target mRNAs. EMBO J 2004;23:3092-102. [Crossref] [PubMed]
- Raineri I, Wegmueller D, Gross B, et al. Roles of AUF1 isoforms, HuR and BRF1 in ARE-dependent mRNA turnover studied by RNA interference. Nucleic Acids Res 2004;32:1279-88. [Crossref] [PubMed]
- Lapucci A, Donnini M, Papucci L, et al. AUF1 Is a bcl-2 A + U-rich element-binding protein involved in bcl-2 mRNA destabilization during apoptosis. J Biol Chem 2002;277:16139-46. [Crossref] [PubMed]
- Wilson GM, Lu J, Sutphen K, et al. Phosphorylation of p40AUF1 regulates binding to A + U-rich mRNA-destabilizing elements and protein-induced changes in ribonucleoprotein structure. J Biol Chem 2003;278:33039-48. [Crossref] [PubMed]
- Palanisamy V, Park NJ, Wang J, et al. AUF1 and HuR proteins stabilize interleukin-8 mRNA in human saliva. J Dent Res 2008;87:772-6. [Crossref] [PubMed]
- Gouble A, Grazide S, Meggetto F, et al. A new player in oncogenesis: AUF1/hnRNPD overexpression leads to tumorigenesis in transgenic mice. Cancer Res 2002;62:1489-95. [PubMed]
- Zucconi BE, Wilson GM. Modulation of neoplastic gene regulatory pathways by the RNA-binding factor AUF1. Front Biosci (Landmark Ed) 2011;16:2307-25. [Crossref] [PubMed]
- Ishimaru D, Zuraw L, Ramalingam S, et al. Mechanism of regulation of bcl-2 mRNA by nucleolin and A+U-rich element-binding factor 1 (AUF1). J Biol Chem 2010;285:27182-91. [Crossref] [PubMed]
- Marth JD, Peet R, Krebs EG, et al. A lymphocyte-specific protein-tyrosine kinase gene is rearranged and overexpressed in the murine T cell lymphoma LSTRA. Cell 1985;43:393-404. [Crossref] [PubMed]
- Voronova AF, Sefton BM. Expression of a new tyrosine protein kinase is stimulated by retrovirus promoter insertion. Nature 1986;319:682-5. [Crossref] [PubMed]
- Li L, Cui Y, Shen J, et al. Evidence for activated Lck protein tyrosine kinase as the driver of proliferation in acute myeloid leukemia cell, CTV-1. Leuk Res 2019;78:12-20. [Crossref] [PubMed]
- Kivioja JL, Thanasopoulou A, Kumar A, et al. Dasatinib and navitoclax act synergistically to target NUP98-NSD1(+)/FLT3-ITD(+) acute myeloid leukemia. Leukemia 2019;33:1360-72. [Crossref] [PubMed]
- Wouters BJ, Jordà MA, Keeshan K, et al. Distinct gene expression profiles of acute myeloid/T-lymphoid leukemia with silenced CEBPA and mutations in NOTCH1. Blood 2007;110:3706-14. [Crossref] [PubMed]
- Rouer E, Dreyfus F, Melle J, et al. Pattern of expression of five alternative transcripts of the lck gene in different hematopoietic malignancies: correlation of the level of lck messenger RNA I B with the immature phenotype of the malignancy. Cell Growth Differ 1994;5:659-66. [PubMed]
- Ghobrial IM, Detappe A, Anderson KC, et al. The bone-marrow niche in MDS and MGUS: implications for AML and MM. Nat Rev Clin Oncol 2018;15:219-33. [Crossref] [PubMed]
- Kumar B, Garcia M, Weng L, et al. Acute myeloid leukemia transforms the bone marrow niche into a leukemia-permissive microenvironment through exosome secretion. Leukemia 2018;32:575-87. [Crossref] [PubMed]