A novel prognostic biomarker: GINS3 is correlated with methylation and immune escape in liver hepatocellular carcinoma
Highlight box
Key findings
• This study’s comprehensive analyses supported GINS3 as a novel targeted biomarker in liver hepatocellular carcinoma (LIHC).
What is known and what is new?
• Liver cancer has become the most frequent fatal malignancy. Despite the booming development in medical technology, there is still a startling lack of treatments for liver cancer. And several researchers have certified GINS3 as a reliable biomarker for diagnosis and prognosis in many cancers;
• GINS3 was significantly upregulated and correlated with poor prognosis methylation, and immune escape in LIHC.
What is the implication, and what should change now?
• GINS3 could be a promising targeted biomarker for more advanced and effective treatments in LIHC.
Introduction
Nowadays, liver cancer, the sixth most common cancer, has become the most frequent fatal malignancy (1,2). Liver cancer usually develops with the risk factor of hepatitis virus, alcohol, obesity, smoking, and diabetes (2,3). In addition, the patient’s odds are dismayingly low unless liver cancer is diagnosed early (4). Despite the booming development in medical technology, there is still a startling lack of treatments for liver cancer (4,5). Therefore, researchers have devoted themselves to investigating the potential biomarkers for further effective treatments (6,7). To be perspective, combing immune checkpoint inhibitors and gene-targeted drugs has recently been unveiled as an auspicious cancer treatment (8). Hence, searching for underlying targeted genes and understanding the molecular mechanisms are urgently needed in liver hepatocellular carcinoma (LIHC).
The GINS tetrameric complex integrates with MCM and CDC45 turning into the productive CDC45-MCM-GINS (CMG) helicases required for genome duplication (9). GINS complex subunit 3 (GINS3) (Psf3), part of the GINS tetrameric complex, is also required for helicase activity which is involved in regulating DNA replication (10-13). In the meantime, variants of GINS3 display impaired growth, S phase progression defects, and decreased Psf3 protein stability (10). Moreover, dysregulation of GINS3 expression has been reported in correlation with poor prognosis as a biomarker in many cancers (14,15). However, the exact role of GINS3 in LIHC is still obscure.
In human cancer, tumor-associated immune cells can be divided into two types: tumor-antagonizing [effector T cells, natural killer (NK) cells dendritic cells (DCs), M1-polarized macrophages and N1-polarized neutrophils] and tumor-promoting immune cells [regulatory T cells (Tregs) and myeloid-derived suppressor cells (MDSCs)] (16). Moreover, the tumor microenvironment (TME) has already been substantiated to interact with cancer cells and then plays a determinative role in tumor survival and progression (17,18). Furthermore, the TME of liver cancer can affect tumor progression, invasion, metastasis, and recurrence (19). It is well known that immune escape is a key cause of tumor progression, and liver fibrosis has been proven crucial in promoting tumor immune escape (20,21). Meanwhile, changes in DNA methylation status were common in tumors. DNA hypermethylation might affect the DNA structure and then repress gene transcription which would silence the related gene (22). Strikingly, the m6A methylation might play dual biological functions in human cancer (23) and regulate TME by affecting immune-inflamed, immune-excluded, and immune-desert respectively (24). Consequently, studying the internal function of LIHC TME and methylation would contribute to a better comprehension of the molecular mechanism and the development of immunotherapy.
Hence, considering the regulatory roles of GINS3 in DNA replication, in this study, multiple online public databases were utilized to evaluate the expression and protein levels of GINS3 in LIHC. Further, the diagnostic and predictive roles of GINS3 were also analyzed by those resources. Eventually, based on our findings, GINS3 was concluded to be an auspicious prognostic biomarker and is associated with methylation and immune escape in LIHC. With the findings of this study, hopefully, advanced immunotherapies and targeted therapies in LIHC will be developed in the near future. We present this article in accordance with the TRIPOD reporting checklist (available at https://tcr.amegroups.com/article/view/10.21037/tcr-22-2565/rc).
Methods
The Cancer Genome Atlas (TCGA) datasets
The data on GINS3 mRNA expression were obtained from TCGA datasets (25) (http://cancergenome.nih.gov/), which were further used to analyze the differential expression of GINS3 in 33 types of human cancer, in 50 LIHC tissues with their paired adjacent normal liver tissues, and in 374 LIHC tissues with 50 normal liver tissues. Clinical characteristics of LIHC patients and associated genes data were also downloaded. Moreover, the correlation between the expression level of GINS3 and the expression of m6A-related genes in LIHC samples and the differences in expression in m6A-related genes between the high and low GINS3 expression groups were also analyzed. M6A-related genes include METTL3, YTHDC1, YTHDC2, METTL14, RBM15, RBM15B, IGF2BP1, IGF2BP2, IGF2BP3, VIRMA, WTAP, YTHDF1, YTHDF2, YTHDF3, ZC3H13, HNRNPA2B1, HNRNPC, RBMX, FTO and ALKBH5 (26). In addition, the expression correlation of GINS3 with immune checkpoints including PD-1, PD-L1, PD-L2, LAG3, CTLA4, and TIM3 in LIHC was also evaluated by data from TCGA. P value <0.05 was considered statistically significant.
Protein expression analysis
The Clinical Proteomic Tumor Analysis Consortium (CPTAC) database (https://proteomics.cancer.gov/programs/cptac) is a central repository for the public dissemination of proteomic sequence datasets which can comprehensively characterize cancer types and support clinically-relevant research projects that elucidate biological mechanisms of response in clinical trials (27). UALCAN (http://ualcan.path.uab.edu/) is a user-friendly and interactive web resource for analyzing cancer data, which can provide protein expression analysis options using data from the CPTAC dataset. In this study, protein expression of GINS3 in LIHC was analyzed by UALCAN using CPTAC datasets.
The Human Protein Atlas (HPA) database (https://www.proteinatlas.org) is an open-access resource with the aim to map all the human proteins in cells, tissues, and organs. Here, the immunohistochemical (IHC) staining results of GINS3 in normal liver tissue and LIHC tissue were collected from HPA.
Survival and prognostic analysis
Kaplan-Meier plotter (KM-plotter) (http://kmplot.com), a potent public online database, is capable to assess the effect of all gene expressions (mRNA, miRNA, protein) on survival in over 30,000 samples from 21 cancer types, including liver cancer (n=364). Here, the overall survival (OS), progression-free survival (PFS), disease-specific survival (DSS), and relapse-free survival (RFS) of GINS3 in liver cancer were analyzed with associated patient samples separated into two groups by median expression. The hazard ratio (HR) with a 95% confidence interval (CI) and log-rank P value were also contained.
Gene-gene interaction and protein-protein interaction (PPI) networks
GeneMANIA (http://www.genemania.org) is a flexible user-friendly website for generating hypotheses about gene function, analyzing gene lists, and prioritizing genes for functional assays (28). STRING (https://string-preview.org/) is an online database for PPI network functional enrichment analysis. In this study, gene-gene and PPI networks of GINS3 were conducted by GeneMANIA and STRING respectively.
Gene Ontology (GO) term and Kyoto Encyclopedia of Genes and Genomes (KEGG) pathway enrichment analysis
The top 300 genes most positively associated with GINS3 from the TCGA database were implemented in functional enrichment, containing biological processes (BPs), cellular components (CCs), molecular functions (MFs), and the KEGG pathway. Functional enrichment analyses of co-expression genes were performed by the EnrichGO and EnrichKEGG function in the R package “ClusterProfiler” and visualized by the package “ggplot2”, with the enrichment value set to P<0.05.
cBioPortal database analysis
The cBioPortal database for Cancer Genomics (https://www.cbioportal.org/) provides an open-access web resource for the interactive exploration of multidimensional cancer genomics data sets. Here the cBioPortal was employed to identify different types of GINS3 genetic alterations in different cancers and then focused on the alteration in LIHC. KM plots for survival outcomes including OS, DSS, PFS, and RFS of GINS3 alterations were contained and the log-rank test was performed.
Methylation analysis
MethSurv (https://biit.cs.ut.ee/methsurv/), a web portal providing univariable and multivariable survival analysis based on DNA methylation biomarkers using TCGA data, was implemented to investigate the DNA methylation sites of GINS3 and evaluate the prognostic values of corresponding cytosine-guanine (CpG) methylation. The HR with 95% CI of the OS was computed and P<0.05 was considered statically significant.
Tumor Immune Estimation Resource (TIMER) analysis
TIMER (http://timer.cistrome.org/) is a comprehensive online resource for the systematical analysis of immune infiltrates across diverse cancer types by inputting the gene expression profile data of tumor samples. In this study, TIMER was utilized to elucidate the correlation between GINS3 expression in LIHC and six tumor-infiltrating immune cells (B cells, CD4+ T cells, CD8+ T cells, neutrophils, macrophages, and DCs). Moreover, we assessed how GINS3 expression correlated with the expression of particular immune checkpoint genes including PDCD1 (PD-1), CD274 (PD-L1), PDCD1LG2 (PD-L2), LAG3, CTLA4, and HAVCR2 (TIM3).
Tumor-Immune System Interaction Database (TISIDB) analysis
TISIDB (http://cis.hku.hk/TISIDB/) is an online web for tumor and immune system interaction, which integrates multiple heterogeneous data types (29). Here, TISIDB analysis was conducted to identify the expression of GINS3 and 28 types of tumor-infiltrating lymphocytes (TILs) across human cancers. The study was performed in accordance with the Declaration of Helsinki (as revised in 2013).
Statistical analysis
The statistical analysis calculated by the online database in this study was mentioned above. ROC curve was performed to identify the cutoff value of GINS3 using the R software package “pROC”. Univariate and multivariate logistic regression analyses were employed to the correlation between GINS3 expression and clinical characteristics. The heat maps of the correlations between GINS3 and the top 50 positively or negatively associated genes were generated by the R software package “pheatmap” with Spearman’s correlation. P value <0.05 or log-rank P value <0.05 was considered statistically significant.
Results
Analysis of upregulated GINS3 mRNA and protein expression in LIHC
In this study, the TCGA database was utilized for the first time to evaluate the mRNA expression pattern of GINS3 across 33 different cancer types relative to normal tissues. As shown in Figure 1A, compared with normal tissues, GINS3 was significantly upregulated in 26 cancer types including LIHC. This indicated that the mRNA expression of GINS3 was aberrant across different cancer types.
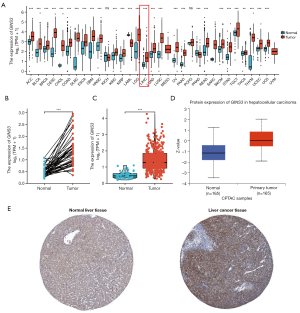
Next, further analyses of the GINS3 expression data from the TCGA and HPA database were conducted to evaluate the mRNA and protein expression of GINS3 in LIHC. As shown in Figure 1B,1C, paired data analysis revealed that the mRNA expression of GINS3 was significantly higher than that in the adjacent normal tissues (n=50) (1.380±0.642 vs. 0.501±0.218, P<0.001), which was also confirmed in unpaired data analysis in 374 LIHC tissues compared with 50 adjacent normal tissues [1.270 (0.928–1.851) vs. 0.455 (0.349–0.601), Mann-Whitney U test, P<0.001]. GINS3 was also assessed at a protein level through the National Cancer Institute’s CPTAC dataset. The result showed that the total protein expression of GINS3 was significantly higher in LIHC tumor tissues compared to normal tissues (Figure 1D, P<0.001).
Furthermore, immunohistochemical staining by the HPA database demonstrated that normal liver tissues had negative or medium GINS3 IHC staining, while LIHC tumor tissues had medium or strong staining (Figure 1E).
Relationship between GINS3 expression and clinical features of LIHC patients
To evaluate the relation between the mRNA expression of GINS3 and clinical features of LIHC samples, Chi-square test, Fisher test and Mann-Whitney U-test were conducted. The statistics showed that the expression level of GINS3 was significantly upregulated in patients with higher T classification (P=0.003) (Table 1). However, no statistically significant association was found between the expression levels of GINS3 and other clinical features including N classification and M classification (Table 1). The above results were consistent with the actual clinical practice that liver cancer was usually found at an advanced stage due to multiple factors.
Table 1
Characteristic | Total (n=374) | Low expression of GINS3 (n=187) | High expression of GINS3 (n=187) | P |
---|---|---|---|---|
T stage, n (%) | 0.003 | |||
T1 | 183 | 107 (28.8) | 76 (20.5) | |
T2 | 95 | 43 (11.6) | 52 (14.0) | |
T3 | 80 | 30 (8.1) | 50 (13.5) | |
T4 | 13 | 3 (0.8) | 10 (2.7) | |
N stage, n (%) | 0.623 | |||
N0 | 254 | 124 (48.1) | 130 (50.4) | |
N1 | 4 | 1 (0.4) | 3 (1.2) | |
M stage, n (%) | 1.000 | |||
M0 | 268 | 129 (47.7) | 139 (51.1) | |
M1 | 4 | 2 (0.7) | 2 (0.7) | |
Age (years), median (IQR) | 373 | 62.0 (52.0, 69.5) | 60.5 (51.0, 68.0) | 0.133 |
TCGA, The Cancer Genome Atlas; IQR, interquartile range.
Diagnostic and prognostic value of GINS3 in LIHC
To estimate the value of GINS3 in distinguishing LIHC samples from normal samples, ROC and Kaplan-Meier (KM) curve analyses were performed. Results showed GINS3 had an area under the curve (AUC) value of 0.939 (95% CI: 0.909–0.969) (Figure 2A). Those results suggested GINS3 as a potential biomarker for distinguishing LIHC tissue from normal tissue.
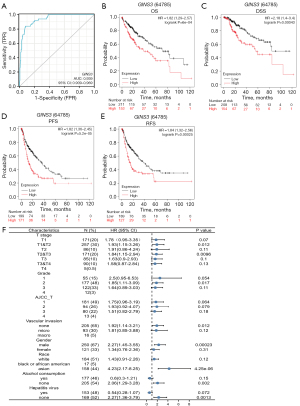
Next, in order to clarify how GINS3 expression related to prognosis in LIHC, cancer cases were divided into high and low expression groups based on the expression level of GINS3, and then those data were analyzed by the KM-plotter. As shown in Figure 2B-2E, compared to low expression of GINS3, higher expression was significantly connected to OS, DSS, PFS, and RFS (all P<0.001).
To better evaluate the prognostic value of GINS3 expression, further exploration was performed to unveil the correlation between the mRNA expression of GINS3 and prognosis. Results (Figure 2F) demonstrated that higher expression of GINS3 was a hazard factor to all T stages, cancer grades, American Joint Committee on Cancer (AJCC) stage, and no microvascular invasion in LIHC. Interestingly, higher expression of GINS3 was obviously a risk factor for people regardless of gender and race. However, GINS3 was a protective factor for LIHC patients with alcohol consumption and hepatitis virus.
Moreover, to further estimate the prognostic potential of GINS3 in LIHC, univariate and multivariate cox regression analyses were performed (Table 2). In the univariate cox regression analysis, T3 & T4, M1, stage III & IV, and high GINS3 expression were all independent risk factors for OS (all P<0.1); in the multivariate cox regression analysis, only high GINS3 expression was the independent risk factor for OS (P=0.052). In conclusion, those results suggested that high GINS3 expression was associated with poor clinical prognosis.
Table 2
Characteristics | Total (n=373) | Univariate analysis | Multivariate analysis | |||
---|---|---|---|---|---|---|
Hazard ratio (95% CI) | P value | Hazard ratio (95% CI) | P value | |||
T stage | 370 | |||||
T1 | 183 | Reference | – | Reference | – | |
T2 | 94 | 1.428 (0.901–2.264) | 0.129 | 1.517 (0.841–2.736) | 0.166 | |
T3 & T4 | 93 | 2.949 (1.982–4.386) | <0.001 | 2.242 (0.296–16.996) | 0.435 | |
N stage | 258 | |||||
N0 | 254 | Reference | – | – | – | |
N1 | 4 | 2.029 (0.497–8.281) | 0.324 | – | – | |
M stage | 272 | |||||
M0 | 268 | Reference | – | Reference | – | |
M1 | 4 | 4.077 (1.281–12.973) | 0.017 | 1.882 (0.575–6.158) | 0.296 | |
Pathologic stage | 349 | |||||
I & II | 259 | Reference | – | Reference | – | |
III & IV | 90 | 2.504 (1.727–3.631) | <0.001 | 1.317 (0.178–9.733) | 0.787 | |
Gender | 373 | |||||
Female | 121 | Reference | – | – | – | |
Male | 252 | 0.793 (0.557–1.130) | 0.200 | – | – | |
Age, years | 373 | |||||
≤60 | 177 | Reference | – | – | – | |
>60 | 196 | 1.205 (0.850–1.708) | 0.295 | – | – | |
Residual tumor | 344 | |||||
R0 | 326 | Reference | – | – | – | |
R1 & R2 | 18 | 1.604 (0.812–3.169) | 0.174 | – | – | |
GINS3 | 373 | |||||
Low | 187 | Reference | – | Reference | – | |
High | 186 | 1.633 (1.153–2.313) | 0.006 | 1.569 (0.996–2.471) | 0.052 |
OS, overall survival; LIHC, liver hepatocellular carcinoma; CI, confidence interval.
Enrichment analysis of GINS3-interacting genes and proteins in LIHC
To find out the essential biological pathways and molecular mechanisms through the BP, enrichment analyses were conducted by GeneMANIA and STRING databases. As shown in Figure 3A, the 20 most frequently altered genes which closely related to GINS3 include GINS2, GINS4, GINS1, and MCM2. Meanwhile, the PPI network of GINS3 through STRING analysis showed 11 nodes including GINS4, CDC45, CDT1, and ORC3 (Figure 3B).
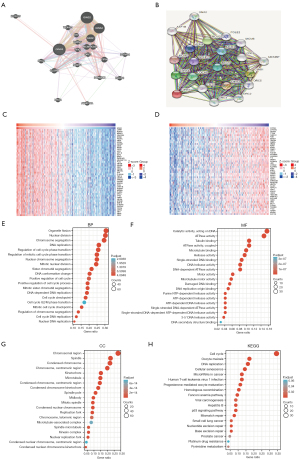
Next, the co-expression genes of GINS3 were investigated to learn about the sequence of gene interaction by utilizing the TCGA database. Results found the top 50 genes that were positively and negatively correlated with GINS3 in LIHC (Figure 3C,3D). Meanwhile, to investigate GINS3-related pathways and biological functions, the KEGG and GO enrichment analyses were performed by using the top 300 genes that are positively related to GINS3. The top 20 significant terms of BP, MF, and CC enrichment analyses are demonstrated in Figure 3E-3G. In terms of BP, GINS3 was enriched in organelle fission, nuclear division, chromosome segregation, and DNA replication. In terms of CC, GINS3 was enriched in the chromosomal region, spindle, and condensed chromosome. In terms of MF, GINS3 was enriched in catalytic activity, acting on DNA. Moreover, the top 19 KEGG pathways for GINS3 and its-correlated genes are presented in Figure 3H. Results showed that GINS3 was closely connected with many DNA replications and cell cycle-related pathways, including cell cycle, oocyte meiosis, DNA replication, and cellular senescence.
Genetic alteration of GINS3 in LIHC
In general, human cancers develop due to the intrinsic factor—the accumulation of genetic alterations. Accordingly, the GINS3 genetic alterations were investigated in human cancer samples and then focused on LIHC through the cBioPortal database. The analysis showed that different types of GINS3 genetic alteration existed in different cancers at different frequencies (Figure 4A). Besides, the main GINS3 genetic alteration in LIHC was the deep deletion and the percentage was 0.2% (Figure 4A,4B). Meanwhile, for clarity, two pie charts of the mutation types are shown in Figure 4C. Missense substitutions occurred in approximately 38.29% of the samples, synonymous substitution occurred in approximately 7.43% of the samples, frameshift insertion occurred in approximately 1.14% of the samples, and other types occurred in approximately 13.71% of the samples (Figure 4C). The substitution mutations mainly occurred at C>T (25.64%) and G>A (24.36%). Moreover, a systematic study was performed to ascertain the correlation between GINS3 genetic alterations and the clinical survival prognosis of LIHC patients. Patients with genetic alteration of GINS3 in LIHC showed a better prognosis in DSS (P=0.0706) and OS (P=0.0400), but not DFS (P=0.828) and PFS (P=0.364), compared to patients without GINS3 genetic alterations (Figure 4D).
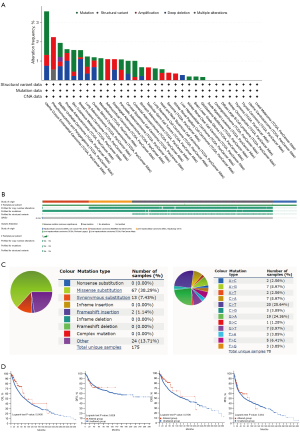
Methylation of GINS3 and its correlation with m6A methylation regulators in LIHC
To investigate the methylation of GINS3 in LIHC, the DNA methylation sites of GINS3 were analyzed and the prognostic values of corresponding cytosine-guanine (CpG) methylation was evaluated through the MethSurv. Totally, 12 methylation CpG sites of GINS3 were observed and cg01783195 had the highest DNA methylation (Figure 5A). And 6 methylation CpG sites were related to the prognosis of LIHC patients. Hypermethylation of GINS3 at cg03919836 and cg05732130 sites was related to a worse OS in LIHC, while hypermethylation of GINS3 at cg02655227, cg05425326, cg09431182, and cg26635363 sites was related to a better OS (Table 3).
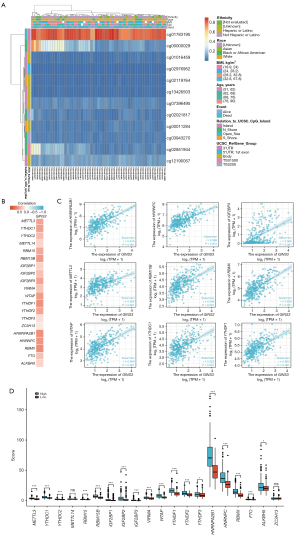
Table 3
Name | HR (95% CI) | P value |
---|---|---|
5'UTR;1stExon-Island-cg00885732 | 0.734 (0.478, 1.128) | 0.16 |
TSS200-Island-cg02655227* | 0.645 (0.44, 0.947) | 0.025 |
5'UTR;1stExon-Island-cg03919836* | 1.607 (1.031, 2.504) | 0.036 |
TSS200-Island-cg04246829 | 0.823 (0.562, 1.204) | 0.32 |
TSS1500-N_Shore-cg04840669 | 1.369 (0.879, 2.131) | 0.16 |
3'UTR-Open_Sea-cg05425326* | 0.672 (0.46, 0.981) | 0.039 |
TSS200-Island-cg05732130* | 1.948 (1.38, 2.749) | 0.0001 |
TSS200-Island-cg09431182* | 0.573 (0.396, 0.829) | 0.0031 |
Body-Island-cg15518345 | 1.067 (0.758, 1.503) | 0.71 |
Body-Island-cg26635363* | 0.424 (0.282, 0.637) | <0.01 |
TSS200-Island-cg26851141 | 1.340 (0.947, 1.895) | 0.099 |
*, methylation CpG sites related to prognosis (P<0.05). LIHC, liver hepatocellular carcinoma; HR, hazard ratio; CI, confidence interval; UTR, untranslated region; CpG, cytosine-guanine.
Furthermore, the TCGA-LIHC data was analyzed to determine the correlation between GINS3 expression and 20 m6A-related genes in LIHC, which were demonstrated as a heat map and scatter plots (Figure 5B,5C). Results showed that GINS3 expression was positively correlated with 12 m6A-related genes in LIHC, including METTL3 (r=0.506, P<0.001), YTHDC1 (r=0.522, P<0.001), RBM15 (r=0.478, P<0.001), RBM15B (r=0.548, P<0.001), IGF2BP2 (r=0.341, P<0.001), IGF2BP3 (r=0.506, P<0.001), WTAP (r=0.545, P<0.001), YTHDF1 (r=0.600, P<0.001), YTHDF2 (r=0.496, P<0.001), HNRNPA2B1 (r=0.616, P<0.001), HNRNPC (r=0.586, P<0.001), and RBMX (r=0.628, P<0.001). Moreover, 424 LIHC patient samples were divided into two groups by median expression of GINS3 to explore the relationship of 20 m6A-related genes with GINS1 expression. Higher expression of METTL3, YTHDC1, RBM15, RBM15B, IGF2BP1, IGF2BP3, VIRMA, WTAP, YTHDF1, YTHDF2, YTHDF3, HNRNPA2B1, HNRNPC, RBMX, and ALKBH5 were observed in the high GINS3 expression group compared with the low GINS3 expression group (Figure 5D). Those results indicated that GINS3 was closely correlated with m6A modification which was involved in the tumor progression including LIHC.
Assessment of the correlation between GINS3 expression and immune cell infiltration in LIHC
To access the level of immune cell infiltration in the LIHC microenvironment, the correlation between GINS3 expression and six types of tumor-infiltrating immune cells was analyzed by the TIMER database. Results demonstrated that GINS3 expression was related to tumor purity (cor =0.1, P=6.43e−02), B cell (cor =0.399, P=1.31e−14), CD8+ T cell (cor =0.388, P=1.06e−18), CD4+ T cell (cor =0.342, P=6.88e−11), macrophage (cor =0.494, P=2.37e−22), neutrophil (cor =0.435, P=2.41e−17), DC (cor =0.467, P=7.61e−20) (Figure 6A). Moreover, further study of the correlation between GINS3 expression and 28 types of TILs across human cancers was conducted in the TISIDB database (Figure 6B). The above results suggested that GINS3 somehow could affect the immune infiltration and then make a progress in LIHC progression.
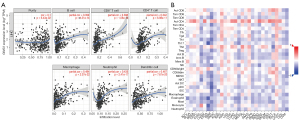
Relationship between GINS3 expression and immune checkpoints in LIHC
To further evaluate the correlation between GINS3 expression and immune escape, the investigation was performed for the immunotherapy checkpoint genes including PDCD1 (PD-1), CD274 (PD-L1), PDCD1LG2 (PD-L2), LAG3, CTLA4, and HAVCR2 (TIM3). The heatmap suggested that the above immune checkpoint genes were positively correlated with GINS3 expression (Figure 7A). In more detail, scatter plots showed the close association of individual immune checkpoint genes with GINS3 expression (Figure 7B). The correlation indicated the important effect of immune escape in GINS3-mediated carcinogenesis of LIHC and then hopefully advanced immunotherapy could be employed in LIHC treatment.
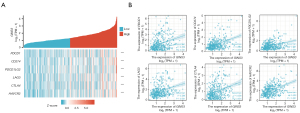
Discussion
For developing the potential therapeutic strategies, it is pivotal to have a comprehensive study of the molecular mechanisms in LIHC. Therefore, in this study, great efforts were made to elucidate that GINS3 was correlated with prognosis, methylation, and immune escape in LIHC.
As a part of the GINS subcomplex, GINS3 is required for helicase activity and is, therefore, involved in DNA replication (9). Also, research has proved that DNA replication drives tumor development and is considered a hallmark of cancer (30,31). Therefore, recently, researchers have focused on the potential correlation between the GINS subunits and cancer (32-34). However, the expression and role of GINS3 in LIHC have not been clarified yet. Here, elucidation of the internal connection between GINS3 and LIHC was made by an integrated analysis of public datasets. Results demonstrated that GINS3 was significantly upregulated in many cancers based on pan-cancer analysis and then further analyses proved that GINS3 is conspicuously upregulated in LIHC. Additionally, it was exhibited that the expression level of GINS3 was significantly upregulated in patients with higher T classification. Those results suggested GINS3 could be a sensitive index for LIHC diagnosis.
Several researchers have certified GINS3 as a reliable biomarker for diagnosis and prognosis in many cancers. In malignant colon cancer, a study showed that overexpression of GINS3 is closely connected with higher-grade tumors and might be a potential biomarker (35). Besides, it has been proved that high GINS3 expression was a poor prognostic marker for pulmonary adenocarcinoma (36). Meanwhile, GINS3 also acted as a factor significantly affecting the prognosis of colorectal cancer patients (37). On the other hand, it was hypothesized that survival time might be prolonged by suppressing the expression of GINS3. Therefore, great efforts were made to investigate the diagnostic and prognostic value of GINS3 in LIHC for more treatment possibilities. The ROC curve analysis proved GINS3 had a good diagnostic value in LIHC. Meanwhile, the KM analyses showed that high GINS3 expression was significantly correlated with poor OS, PFS, DSS, and RFS. Also, the forest plot and univariate and multivariate cox regression analyses both indicated high GINS3 expression as a risk factor in LIHC. According to strong evidence, GINS3 was confirmed to be a promising biomarker in the diagnosis and prognosis of LIHC.
Previous study has proved that the GINS gene is essential for the viability of human cells (38). GINS1, another component of the GINS complex, is an essential component of the RAS/RAF/MAPK signaling pathway which is related to various cancers (39,40). In melanoma, the RAS/RAF/MAPK signaling pathway, regulated by microRNA-340, could suppress the tumorigenic phenotype (41). However, especially in LIHC, the essential biological pathways and the underlying mechanism of GINS3 have not been clarified yet. In this study, co-expression analyses were conducted and found that GINS3 was significantly correlated with GINS4, GINS1, GINS2, and MCM2. Meanwhile, the KEGG and GO enrichment analyses indicated that GINS3 was enriched in the chromosomal region and highly involved in cell cycle progression by affecting organelle fission, DNA replication, catalytic activity, and ATPase activity. The above results suggested that GINS3 might be an essential prerequisite for the progression of LIHC and therefore, could be a promising therapeutic target of LIHC.
Genetic alteration is known to be associated with DNA replication, DNA damage repair, cancer, and senescence. And the accumulation of genetic alterations has been perceived as a driving factor in cancer progression (42). Based on this, the exact pattern of GINS3 genetic alteration was probed into. Analyses showed that GINS3 genetic alteration which existed in multiple cancers was only 0.2% in LIHC. The alteration was mainly deep deletion and missense substitutions that occurred at C>T and G>A. Moreover, the results also showed a better prognosis in LIHC patients with genetic alteration of GINS3. In conclusion, genetic alteration of GINS3 played a certain role in LIHC progression.
Changes in DNA methylation status were common in all forms of cancer. Generally, DNA hypermethylation might affect the DNA structure and then repress gene transcription which would silence the related gene (22). However, the CpG island was the methylated site to regulate the expression of downstream genes (22). Therefore, the analyses were conducted to learn that there were 12 methylation CpG sites of GINS3 and some were associated with prognosis in LIHC. Meanwhile, m6A methylation, the most important and abundant form of internal modifications in eukaryotic cells, has been proven to be pivotal in tumor proliferation, migration, and invasion (26,43). By regulating oncogenes or suppressor genes, the m6A methylation could interfere with the immune infiltration phenotype and the progression of cancer (23,44). Moreover, the m6A methylation also could regulate the expression level of m6A and the activity of m6A methyltransferases, therefore affecting the role of m6A in cancer (45,46). The m6A methylation had been certified targeting the IL-7/STAT5/SOCS pathways to control T cell homeostasis which regulates TME in turn (47). Interestingly, dysregulated m6A methylation was proved to associate with hepatocellular (48,49). A paper from Qi et al. demonstrated a close association between the high expression of m6A-related genes and poor OS in LIHC (50). Also, the key m6A-related genes METTL3 and METTL14 were reported to be active components of the m6A methyltransferase complex and correlated with tumor proliferation, differentiation, tumorigenesis, invasion, and metastasis (51,52). METLL3 had been proven to possess oncogenic functions in liver cancer, therefore promoting the growth of liver cancer (48). Research revealed that, in LIHC, upregulation of METTL14 by lipopolysaccharide (LPS) promotes the m6A methylation of MIR155HG, which modulates the expression of programmed death-ligand 1 (PD-L1) by miR-223/STAT1 axis (53). Consequently, further analyses were performed to unveil the exact relation between GINS3 expression and m6A modification in LIHC. A series of m6A-related genes were positively correlated with GINS3 expression, including METTL3, YTHDC1, RBM15, RBM15B, IGF2BP2, IGF2BP3, WTAP, YTHDF1, YTHDF2, HNRNPA2B1, HNRNPC, and RBMX. Based on those results, it was speculated that GINS3 was closely associated with m6A modification and therefore affected the proliferation, migration, and metastasis of LIHC.
TME comprising innate and adaptive immune cells was proved to play a determinative role in tumor survival and progression (17,18). And it is well known that immune escape is a key cause of tumor progression. Therefore, in liver cancer, lots of research had been conducted and found that β-Catenin activation and PD-L1 promoted immune escape (54,55). Furthermore, the activation of STAT3 and NF-κB signaling pathways can induce PD-L1 expression directly and indirectly (55). Meanwhile, liver fibrosis is highly promoted by activated hepatic stellate cells (HSCs) which have immunomodulatory activity by expressing proteins such as PD-L1 and stimulate the expansion of immunosuppressive cells such as Tregs and MDSC (20,21,56). Since the liver is the most frequent organ affected by metastasis after lymph nodes, multiple efforts have been made to unveil that tumor immunity is a fundamental part of metastasis in LIHC (57-60). In addition, the liver itself has an immunosuppressive polarization, however, the metastatic microenvironment underwent remarkable spatial reprogramming of immunosuppressive cells such as MRC1+CCL18+ M2-like macrophages (57,58). Strikingly, liver metastasis can alter the immune microenvironment of the liver by inducing systemic loss of antigen-specific T cells and recruiting and polarizing monocyte-derived macrophages (59,60). Thus, we further assessed the association between GINS3 expression and tumor immune microenvironment in LIHC. By the TIMER database, we found that GINS3 expression was related to tumor purity, CD8+ T cell, CD4+ T cell, macrophage, neutrophil, and DC. Combing the above results and GINS3 expression in 28 types of TILs, it was assumed that GINS3 could somehow alter the LIHC immune infiltration and then affect LIHC progression.
Recently, more and more studies have extended the scope of immunotherapy by unveiling immune escape as a novel target for cancer treatment (61,62). As for the treatment of liver cancer patients, nonspecific immune stimulation, adaptive cell transfer, immune-checkpoint inhibitors, and DC-based vaccination have been developed as promising immunotherapy (16). Most recently, in LIHC, the combination of atezolizumab (immune-checkpoint inhibitor) and bevacizumab (anti-angiogenic agent) is the first treatment regimen that has been shown to improve OS relative to sorafenib (63). As immune checkpoints are indispensable in immune escape, the correlation between GINS3 expression and immune checkpoints was investigated in this study. The heatmap and scatter plots showed a strong connection to the immune checkpoints, containing PDCD1, CD274, PDCD1LG2, LAG3, CTLA4, and HAVCR. In conclusion, findings from the mechanism-based hypothesis shed light on GINS3 as a promising immunotherapeutic target.
Conclusions
In this study, the comprehensive analyses could prove that GINS3 was significantly upregulated and correlated with poor prognosis methylation, and immune escape in LIHC. Based on the findings of the study, higher GINS3 expression could be a novel targeted biomarker for more advanced and effective treatments in LIHC.
Acknowledgments
Funding: This study was supported by grants from
Footnote
Reporting Checklist: The authors have completed the TRIPOD reporting checklist. Available at https://tcr.amegroups.com/article/view/10.21037/tcr-22-2565/rc
Conflicts of Interest: All authors have completed the ICMJE uniform disclosure form (available at https://tcr.amegroups.com/article/view/10.21037/tcr-22-2565/coif) and report that this study was supported by grants from the Medical Scientific Research Foundation of Guangdong Province (No. A202109), the Fundamental Research Funds for the Central Universities (No. 21622312), the Basic and Applied Basic Research Project of Guangzhou Basic Research Program (No. 2023A04J01917), the Flagship Specialty Construction Project-General Surgery of the First Affiliated Hospital of the Jinan University (No. 711003), and the Special Foundation for Scientific Research Development of the Affiliated Shunde Hospital of Jinan University (No. 202101004). The authors have no other conflicts of interest to declare.
Ethical Statement: The authors are accountable for all aspects of the work in ensuring that questions related to the accuracy or integrity of any part of the work are appropriately investigated and resolved. The study was performed in accordance with the Declaration of Helsinki (as revised in 2013).
Open Access Statement: This is an Open Access article distributed in accordance with the Creative Commons Attribution-NonCommercial-NoDerivs 4.0 International License (CC BY-NC-ND 4.0), which permits the non-commercial replication and distribution of the article with the strict proviso that no changes or edits are made and the original work is properly cited (including links to both the formal publication through the relevant DOI and the license). See: https://creativecommons.org/licenses/by-nc-nd/4.0/.
References
- Sung H, Ferlay J, Siegel RL, et al. Global Cancer Statistics 2020: GLOBOCAN Estimates of Incidence and Mortality Worldwide for 36 Cancers in 185 Countries. CA Cancer J Clin 2021;71:209-49. [Crossref] [PubMed]
- Villanueva A. Hepatocellular Carcinoma. N Engl J Med 2019;380:1450-62. [Crossref] [PubMed]
- Marengo A, Rosso C, Bugianesi E. Liver Cancer: Connections with Obesity, Fatty Liver, and Cirrhosis. Annu Rev Med 2016;67:103-17. [Crossref] [PubMed]
- Gravitz L. Liver cancer. Nature 2014;516:S1. [Crossref] [PubMed]
- Anwanwan D, Singh SK, Singh S, et al. Challenges in liver cancer and possible treatment approaches. Biochim Biophys Acta Rev Cancer 2020;1873:188314. [Crossref] [PubMed]
- Luan M, Si H. Novel hypoxia features with appealing implications in discriminating the prognosis, immune escape and drug responses of 947 hepatocellular carcinoma patients. Transl Cancer Res 2022;11:2097-121. [Crossref] [PubMed]
- Bai E, Dong M, Lin X, et al. Expressional and functional characteristics of checkpoint kinase 1 as a prognostic biomarker in hepatocellular carcinoma. Transl Cancer Res 2022;11:4272-88. [Crossref] [PubMed]
- Colli LM, Machiela MJ, Zhang H, et al. Landscape of Combination Immunotherapy and Targeted Therapy to Improve Cancer Management. Cancer Res 2017;77:3666-71. [Crossref] [PubMed]
- Sedlackova H, Rask MB, Gupta R, et al. Equilibrium between nascent and parental MCM proteins protects replicating genomes. Nature 2020;587:297-302. [Crossref] [PubMed]
- McQuaid ME, Ahmed K, Tran S, et al. Hypomorphic GINS3 variants alter DNA replication and cause Meier-Gorlin syndrome. JCI Insight 2022;7:e155648. [Crossref] [PubMed]
- Wu L, Liu Y, Kong D. Mechanism of chromosomal DNA replication initiation and replication fork stabilization in eukaryotes. Sci China Life Sci 2014;57:482-7. [Crossref] [PubMed]
- Jenkyn-Bedford M, Jones ML, Baris Y, et al. A conserved mechanism for regulating replisome disassembly in eukaryotes. Nature 2021;600:743-7. [Crossref] [PubMed]
- Labib K, Gambus A. A key role for the GINS complex at DNA replication forks. Trends Cell Biol 2007;17:271-8. [Crossref] [PubMed]
- Tauchi S, Sakai Y, Fujimoto S, et al. Psf3 is a prognostic biomarker in lung adenocarcinoma: a larger trial using tissue microarrays of 864 consecutive resections. Eur J Cardiothorac Surg 2016;50:758-64. [Crossref] [PubMed]
- Tane S, Sakai Y, Hokka D, et al. Significant role of Psf3 expression in non-small-cell lung cancer. Cancer Sci 2015;106:1625-34. [Crossref] [PubMed]
- Lei X, Lei Y, Li JK, et al. Immune cells within the tumor microenvironment: Biological functions and roles in cancer immunotherapy. Cancer Lett 2020;470:126-33. [Crossref] [PubMed]
- Hinshaw DC, Shevde LA. The Tumor Microenvironment Innately Modulates Cancer Progression. Cancer Res 2019;79:4557-66. [Crossref] [PubMed]
- Xia L, Oyang L, Lin J, et al. The cancer metabolic reprogramming and immune response. Mol Cancer 2021;20:28. [Crossref] [PubMed]
- Fu Y, Liu S, Zeng S, et al. From bench to bed: the tumor immune microenvironment and current immunotherapeutic strategies for hepatocellular carcinoma. J Exp Clin Cancer Res 2019;38:396. [Crossref] [PubMed]
- Vinay DS, Ryan EP, Pawelec G, et al. Immune evasion in cancer: Mechanistic basis and therapeutic strategies. Semin Cancer Biol 2015;35:S185-98. [Crossref] [PubMed]
- Ke MY, Xu T, Fang Y, et al. Liver fibrosis promotes immune escape in hepatocellular carcinoma via GOLM1-mediated PD-L1 upregulation. Cancer Lett 2021;513:14-25. [Crossref] [PubMed]
- Klutstein M, Nejman D, Greenfield R, et al. DNA Methylation in Cancer and Aging. Cancer Res 2016;76:3446-50. [Crossref] [PubMed]
- Chen XY, Zhang J, Zhu JS. The role of m(6)A RNA methylation in human cancer. Mol Cancer 2019;18:103. [Crossref] [PubMed]
- Chong W, Shang L, Liu J, et al. m(6)A regulator-based methylation modification patterns characterized by distinct tumor microenvironment immune profiles in colon cancer. Theranostics 2021;11:2201-17. [Crossref] [PubMed]
- Tomczak K, Czerwińska P, Wiznerowicz M. The Cancer Genome Atlas (TCGA): an immeasurable source of knowledge. Contemp Oncol (Pozn) 2015;19:A68-77. [Crossref] [PubMed]
- Li Y, Xiao J, Bai J, et al. Molecular characterization and clinical relevance of m(6)A regulators across 33 cancer types. Mol Cancer 2019;18:137. [Crossref] [PubMed]
- Edwards NJ, Oberti M, Thangudu RR, et al. The CPTAC Data Portal: A Resource for Cancer Proteomics Research. J Proteome Res 2015;14:2707-13. [Crossref] [PubMed]
- Franz M, Rodriguez H, Lopes C, et al. GeneMANIA update 2018. Nucleic Acids Res 2018;46:W60-4. [Crossref] [PubMed]
- Ru B, Wong CN, Tong Y, et al. TISIDB: an integrated repository portal for tumor-immune system interactions. Bioinformatics 2019;35:4200-2. [Crossref] [PubMed]
- Macheret M, Halazonetis TD. DNA replication stress as a hallmark of cancer. Annu Rev Pathol 2015;10:425-48. [Crossref] [PubMed]
- Gaillard H, García-Muse T, Aguilera A. Replication stress and cancer. Nat Rev Cancer 2015;15:276-89. [Crossref] [PubMed]
- Zhu Z, Yu Z, Rong Z, et al. The novel GINS4 axis promotes gastric cancer growth and progression by activating Rac1 and CDC42. Theranostics 2019;9:8294-311. [Crossref] [PubMed]
- Rong Z, Luo Z, Zhang J, et al. GINS complex subunit 4, a prognostic biomarker and reversely mediated by Krüppel-like factor 4, promotes the growth of colorectal cancer. Cancer Sci 2020;111:1203-17. [Crossref] [PubMed]
- Zhan L, Yang J, Liu Y, et al. MicroRNA miR-502-5p inhibits ovarian cancer genesis by downregulation of GINS complex subunit 2. Bioengineered 2021;12:3336-47. [Crossref] [PubMed]
- Nagahama Y, Ueno M, Haraguchi N, et al. PSF3 marks malignant colon cancer and has a role in cancer cell proliferation. Biochem Biophys Res Commun 2010;392:150-4. [Crossref] [PubMed]
- Hokka D, Maniwa Y, Tane S, et al. Psf3 is a prognostic biomarker in lung adenocarcinoma. Lung Cancer 2013;79:77-82. [Crossref] [PubMed]
- Sun X, Sui W, Huang M, et al. Partner of Sld five 3: a potential prognostic biomarker for colorectal cancer. Diagn Pathol 2014;9:217. [Crossref] [PubMed]
- Luo H, Lin Y, Liu T, et al. DEG 15, an update of the Database of Essential Genes that includes built-in analysis tools. Nucleic Acids Res 2021;49:D677-86. [Crossref] [PubMed]
- Li S, Wu L, Zhang H, et al. GINS1 Induced Sorafenib Resistance by Promoting Cancer Stem Properties in Human Hepatocellular Cancer Cells. Front Cell Dev Biol 2021;9:711894. [Crossref] [PubMed]
- Dimri M, Satyanarayana A. Molecular Signaling Pathways and Therapeutic Targets in Hepatocellular Carcinoma. Cancers (Basel) 2020;12:491. [Crossref] [PubMed]
- Poenitzsch Strong AM, Setaluri V, Spiegelman VS. MicroRNA-340 as a modulator of RAS-RAF-MAPK signaling in melanoma. Arch Biochem Biophys 2014;563:118-24. [Crossref] [PubMed]
- Garnis C, Buys TP, Lam WL. Genetic alteration and gene expression modulation during cancer progression. Mol Cancer 2004;3:9. [Crossref] [PubMed]
- Zhang B, Wu Q, Li B, et al. m6A regulator-mediated methylation modification patterns and tumor microenvironment infiltration characterization in gastric cancer. Mol Cancer 2020;19:53. [Crossref] [PubMed]
- Du J, Ji H, Ma S, et al. m6A regulator-mediated methylation modification patterns and characteristics of immunity and stemness in low-grade glioma. Brief Bioinform 2021;22:bbab013. [Crossref] [PubMed]
- Jiang X, Liu B, Nie Z, et al. The role of m6A modification in the biological functions and diseases. Signal Transduct Target Ther 2021;6:74. [Crossref] [PubMed]
- Liu J, Harada BT, He C. Regulation of Gene Expression by N(6)-methyladenosine in Cancer. Trends Cell Biol 2019;29:487-99. [Crossref] [PubMed]
- Li HB, Tong J, Zhu S, et al. m(6)A mRNA methylation controls T cell homeostasis by targeting the IL-7/STAT5/SOCS pathways. Nature 2017;548:338-42. [Crossref] [PubMed]
- Chen M, Wong CM. The emerging roles of N6-methyladenosine (m6A) deregulation in liver carcinogenesis. Mol Cancer 2020;19:44. [Crossref] [PubMed]
- Yang Y, Cai J, Yang X, et al. Dysregulated m6A modification promotes lipogenesis and development of non-alcoholic fatty liver disease and hepatocellular carcinoma. Mol Ther 2022;30:2342-53. [Crossref] [PubMed]
- Qi LW, Jia JH, Jiang CH, et al. Contributions and Prognostic Values of N6-Methyladenosine RNA Methylation Regulators in Hepatocellular Carcinoma. Front Genet 2021;11:614566. [Crossref] [PubMed]
- Lin S, Choe J, Du P, et al. The m(6)A Methyltransferase METTL3 Promotes Translation in Human Cancer Cells. Mol Cell 2016;62:335-45. [Crossref] [PubMed]
- Fu Y, Dominissini D, Rechavi G, et al. Gene expression regulation mediated through reversible m6A RNA methylation. Nat Rev Genet 2014;15:293-306. [Crossref] [PubMed]
- Peng L, Pan B, Zhang X, et al. Lipopolysaccharide facilitates immune escape of hepatocellular carcinoma cells via m6A modification of lncRNA MIR155HG to upregulate PD-L1 expression. Cell Biol Toxicol 2022;38:1159-73. [Crossref] [PubMed]
- Ruiz de Galarreta M, Bresnahan E, Molina-Sánchez P, et al. β-Catenin Activation Promotes Immune Escape and Resistance to Anti-PD-1 Therapy in Hepatocellular Carcinoma. Cancer Discov 2019;9:1124-41. [Crossref] [PubMed]
- Wen Q, Han T, Wang Z, et al. Role and mechanism of programmed death-ligand 1 in hypoxia-induced liver cancer immune escape. Oncol Lett 2020;19:2595-601. [Crossref] [PubMed]
- Li T, Liu J, Wang Y, et al. Liver fibrosis promotes immunity escape but limits the size of liver tumor in a rat orthotopic transplantation model. Sci Rep 2021;11:22846. [Crossref] [PubMed]
- Wu Y, Yang S, Ma J, et al. Spatiotemporal Immune Landscape of Colorectal Cancer Liver Metastasis at Single-Cell Level. Cancer Discov 2022;12:134-53. [Crossref] [PubMed]
- Li X, Ramadori P, Pfister D, et al. The immunological and metabolic landscape in primary and metastatic liver cancer. Nat Rev Cancer 2021;21:541-57. [Crossref] [PubMed]
- Yu J, Green MD, Li S, et al. Liver metastasis restrains immunotherapy efficacy via macrophage-mediated T cell elimination. Nat Med 2021;27:152-64. [Crossref] [PubMed]
- Brodt P. Role of the Microenvironment in Liver Metastasis: From Pre- to Prometastatic Niches. Clin Cancer Res 2016;22:5971-82. [Crossref] [PubMed]
- Reisländer T, Groelly FJ, Tarsounas M. DNA Damage and Cancer Immunotherapy: A STING in the Tale. Mol Cell 2020;80:21-8. [Crossref] [PubMed]
- Topalian SL, Taube JM, Anders RA, et al. Mechanism-driven biomarkers to guide immune checkpoint blockade in cancer therapy. Nat Rev Cancer 2016;16:275-87. [Crossref] [PubMed]
- Llovet JM, Castet F, Heikenwalder M, et al. Immunotherapies for hepatocellular carcinoma. Nat Rev Clin Oncol 2022;19:151-72. [Crossref] [PubMed]