Use of machine learning with two-dimensional synthetic mammography for axillary lymph node metastasis prediction in breast cancer: a preliminary study
Highlight box
Key Findings
• This study aimed to investigate the possibility of predicting axillary lymph node (ALN) metastasis using radiomic analysis of synthetic mammography (SM) images. The ALN prediction model using radiomic features extracted from SM images demonstrated the possibility of enhancing the accuracy of diagnostic imaging when utilised together with traditional imaging techniques.
What is known and what is new?
• The use of mammographic radiomic features to predict ALN metastases has been investigated in several studies.
• This study assessed the possibility of predicting ALN metastasis using radiomic analysis of SM images generated from digital breast tomosynthesis (DBT).
What is the implication, and what should change now?
• The result of the current study implies the claim that the radiomic features extracted from SM images might be as informative as those from full-field digital mammography (FFDM).
• Thus, our findings suggest that it may be possible to perform DBT without the need for FFDM.
Introduction
Breast cancer is the most prevalent type of cancer and the fifth most common cause of cancer-related deaths worldwide (1). Axillary lymph node (ALN) metastasis in patients with breast cancer is a critical factor in pathological staging, treatment strategy, and prognostic evaluation (2). Initially, the primary breast cancer drains into the sentinel lymph nodes (SLNs); thus, SLN dissection (SLND) or biopsy is performed to assess ALN metastasis. SLND poses certain complications, such as infection, seromas, lymphedema, and sensory loss (3-5). Additionally, SLND requires practical experience and nuclear medicine facilities, making it a challenging task to perform it in primary hospitals. Therefore, a non-invasive ALN metastasis prediction method would be valuable in reducing the risk of complications in primary hospitals.
Mammography is the standard modality for breast cancer screening and diagnosis; however, full-field digital mammography (FFDM) is less effective in patients with dense breasts or small tumours (<1 mm) (6,7). To combat this problem, digital breast tomosynthesis (DBT) has been developed to improve lesion conspicuity, especially in dense breasts, by unravelling the overlapping breast tissues through projections from different angles. The combination of FFDM and DBT improves cancer detection rates and decreases the recall rate (6,8,9). However, the radiation dose increases with the use of both FFDM and DBT (10). The European Commission Initiative on Breast Cancer (ECIBC)’s Guidelines Development Group (11) has recommended that both FFDM and DBT are not to be used. To overcome this problem, two-dimensional synthetic mammography (SM) was emerged and approved for clinical use by the US Food and Drug Administration (FDA) in 2013. SM images are created by summing and filtering the stack of reconstructed DBT slices and circumventing the need for additional radiation doses. SM showed a higher cancer detection rate and lower recall rate in combination with DBT compared with FFDM alone (8,12,13). SM could be an alternative methodology for FFDM without additional radiation exposure.
Radiomics is an emerging quantitative technique for obtaining and analysing large quantities of data from medical images (14,15). Radiomic features include tumour size, shape, location, intensity (histogram-based), and texture analysis, reflecting tumour heterogeneity and offering information regarding tumour genes and molecules. Radiomics analyses using mammography have been reported to predict breast cancer characteristics (16) such as ALN status (17-19). The ALN predictive model using radiomic features is of great significance in a non-invasive method. As the role of DBT increases, the significance of SM also increases; however, to the best of our knowledge, no study has investigated whether the ALN predictive model using radiomic features extracted from SM images could be developed. The aim of this study was to investigate whether radiomic features extracted from SM images and reconstructed from DBT could predict ALN status. We present this article in accordance with the TRIPOD reporting checklist (available at https://tcr.amegroups.com/article/view/10.21037/tcr-22-2668/rc).
Methods
Patients
The study was conducted in accordance with the Declaration of Helsinki (as revised in 2013). This study was approved by Institutional Review Board of St. Marianna University (No. 5362). As our study was retrospective in nature with no personally identifiable information, the need for informed consent was waived. We surveyed the pathology reports of patients diagnosed with breast cancer at our institute between January 2019 and January 2021. The inclusion criteria for the study were as follows: (I) breast surgery was performed without neoadjuvant chemotherapy, (II) pathologically confirmed breast cancer, (III) no previous history of breast cancer, and (IV) both FFDM and DBT were performed. Thus, 103 patients were enrolled. The exclusion criteria were as follows: (I) SLND or ALN dissection was not performed (n=3) and (II) mass lesion was not detected (n=23). A flowchart of this process is shown in Figure 1. In total, 77 patients were selected to participate in this study.
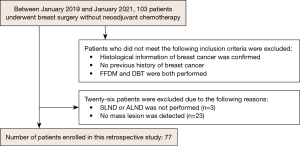
The clinical and pathological characteristics including patient age at surgery, histological grade of the tumour, oestrogen receptor (ER), progesterone receptor (PR), human epidermal growth factor receptor 2 (HER2), lymphatic invasion, Ki-67 levels, nuclear grade, and ALN metastasis were obtained. The tumours were categorised as invasive or non-invasive carcinomas based on the histological grade. The ER and PR statuses were determined using immunostaining and were defined as positive at 1% or higher in the immunostained cells. The HER2-positive status was determined as an immunohistochemistry staining score of 3. Fluorescence in situ hybridisation (FISH) was performed to determine the HER2 status when the HER2 score was 2. The Ki-67 positive status was defined as 14% or higher for immunostained cells. Lymphatic invasion was classified as ly1 and ly0. The histological grades were categorised as grades 1, 2, or 3. ALN metastasis was characterised by the presence of at least one metastasis.
Mammography technique
All patients underwent DBT and FFDM using the Selenia Dimensions (Hologic, USA). DBT and FFDM images of each breast were sequentially acquired during a single breast compression per view. The radiation dose associated with DBT acquisition was set to be approximately the same as that of FFDM. DBT and FFDM images with bilateral craniocaudal (CC) and mediolateral oblique (MLO) views were obtained. DBT images were acquired with the X-ray tube rotated by a 15°. The image processing software used in this study was “Intelligent2D” (Hologic), which reconstructed 15 projection images to obtain SM images for each view.
Segmentation and radiomic feature extraction
The radiomics analysis was performed as shown in Figure 2.
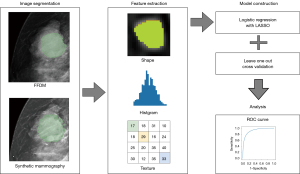
All mammography images were imported to an open-source software platform for medical imaging (3D Slicer 5.0.3; www.slicer.org), supported by the National Institutes of Health and a worldwide developer community (20). The regions of interest (ROI) of mass lesions were manually delineated by two breast radiological technologists using a thresholding method and further confirmed by a radiologist with more than 10 years of experience. Subsequently, radiomic features were extracted using PyRadiomics extension in 3D slicer (PyRadiomics 3.0.1; pyradiomics.readthedocs.io). There were no missing values.
Model development and evaluation of predictive model
The FFDM and SM radiomic features were used to construct logistic regression models (Python Version 3.7, Python Software Foundation, USA). Their performance was evaluated based on the area under the curve (AUC) value, sensitivity, specificity, positive predictive value (PPV), and negative predictive value (NPV) which was calculated using the optimal threshold according to the maximum Youden index. These two models were evaluated using the leave-one-out cross-validation (LOOCV) with the least absolute shrinkage and selection operator (LASSO) regularisation. The alpha parameter for LASSO regularisation was optimised to 0.1 and 0.03 for the FFDM and SM models, respectively.
Statistical analysis
Statistical analysis for clinical characteristics of the ALN-positive and ALN-negative groups was performed using the R software (version 4.2.1; http://www.r-project.org). With age as a continuous variable, we calculated the median and interquartile range and assessed the differences between the two groups using the Mann–Whitney U test. For categorical variables except for age, Pearson’s chi-squared test was performed to assess the differences between the two groups. The AUC values were compared using Delong’s test. Statistical significance was set at P<0.05.
Results
Clinical characteristics
This study included 77 patients, 23 with and 54 without ALN metastasis. The clinical characteristics of the ALN-positive and ALN-negative groups are presented in Table 1. Some of the clinical characteristics have missing values. Only lymph invasion and nuclear grades showed significant differences between the two groups.
Table 1
Characteristics | ALN-positive group (n=23) | ALN-negative group (n=54) | P value |
---|---|---|---|
Age (years), median [IQR] | 64 [56, 73.5] | 57.5 [52.0, 68.75] | 0.134 |
Histology, n (%) | 0.180 | ||
Invasive carcinoma | 23 (100.0) | 50 (92.6) | |
Non-invasive carcinoma | 0 (0.0) | 4 (7.4) | |
Estrogen receptor status, n (%) | 0.809 | ||
Positive | 20 (87.0) | 48 (88.9) | |
Negative | 3 (13.0) | 6 (11.1) | |
Progesterone receptor status, n (%) | 0.801 | ||
Positive | 19 (82.6) | 48 (88.9) | |
Negative | 4 (17.4) | 3 (5.6) | |
HER2 status, n (%) | 0.65 | ||
Positive | 2 (8.7) | 3 (5.6) | |
Negative | 21 (91.3) | 48 (88.9) | |
Ki-67 status, n (%) | 0.390 | ||
Positive | 11 (47.8) | 31 (57.4) | |
Negative | 12 (52.2) | 22 (40.7) | |
Lymphatic invasion, n (%) | <0.0001 | ||
1 | 15 (65.2) | 50 (92.6) | |
0 | 8 (34.8) | 4 (7.4) | |
Nuclear grade, n (%) | 0.029 | ||
2 or 3 | 14 (60.9) | 18 (33.3) | |
1 | 9 (39.1) | 35 (64.8) |
ALN, axillary lymph node; HER2, human epidermal growth factor receptor 2; IQR, interquartile range.
Radiomic features
The radiomic features that were selected frequently in the FFDM and SM model are shown in Table 2. The common feature between both was the maximum diameter feature of the histogram.
Table 2
Category | Subcategory | Radiomic feature |
---|---|---|
FFDM | ||
Morphological | – | Maximum diameter, sphericity |
Histogram | – | Interquartile range |
Texture | GLCM | Maximum probability, joint entropy |
SM model | ||
Morphological | – | Elongation, maximum diameter |
Histogram | – | Maximum |
Texture | GLCM | Small dependence high grey level emphasis |
Texture | GLSZM | Large area high grey level emphasis |
FFDM, full-field digital mammography; SM, synthetic mammography; GLCM, gray level co-occurrence matrix; GLSZM, gray level size zone matrix, AUC, area under the curve.
Evaluation of predictive model
The receiver operating characteristic (ROC) curve is shown in Figure 3. As shown in Table 3, the FFDM model yielded an AUC value of 0.738 (95% CI: 0.608–0.867), sensitivity of 0.826, specificity of 0.630, PPV of 0.488, and NPV of 0.894. The SM model yielded an AUC value of 0.742 (95% CI: 0.613–0.871), sensitivity of 0.783, specificity of 0.630, PPV of 0.474, and NPV of 0.871. No significant differences were observed between the two models.
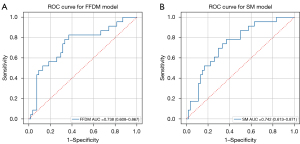
Table 3
Modality | AUC value (95% CI) | Sensitivity | Specificity | PPV | NPV |
---|---|---|---|---|---|
FFDM | 0.738 (0.608–0.867) | 0.826 | 0.630 | 0.488 | 0.894 |
SM | 0.742 (0.613–0.871) | 0.783 | 0.630 | 0.474 | 0.871 |
ALN, axillary lymph node; AUC, the area under curve; PPV, positive predictive value; NPV, negative predictive value; FFDM, full-field digital mammography; SM, synthetic mammography.
Discussion
In this preliminary study, we evaluated the performance of ALN predictive models using FFDM and SM radiomic features. The FFDM model yielded an AUC value of 0.738, and the SM model yielded an AUC value of 0.742. This finding indicates that SM could be an alternative to FFDM in the prediction of ALN status.
An accurate diagnosis of ALN metastasis in patients with breast cancer is vital for the assessment of disease progression and effective treatment strategies (21) and could help avoid unnecessary SLND. Although ALN status is evaluated according to the traditional imaging characteristics in practical settings, current evaluation methods are based on the subjectivity of the radiologist (22-25). Radiomics is emerging as an objective and quantitative methodology that is capable of extracting high-dimensional features from medical images. This method provides information about tumour size, shape, greyscale patterns, and the connection between the intensity values at the voxel level. To date, radiomics analysis to predict ALN status using mammography has been reported (17-19,26).
The SM images generated from DBT is considered to be an alternative imaging for FFDM. However, SM plays a significant role in improving diagnostic accuracy in combination with FFDM with a challenging issue of increased radiation exposure. To date, few radiomics analyses using DBT or SM images were reported. Tagliafico et al. reported two radiomics analyses using DBT features. One is an exploratory radiomics analysis examining whether DBT could distinguish between cancer tissues with negative mammograms and normal breast tissues in women with dense breasts (27). This analysis indicated that some radiomic features could differentiate between cancers and normal breast tissue. Another report investigated the association between the radiomic features extracted from DBT and Ki-67 expression in breast cancer (28). This report showed that 34 radiomic features were significantly correlated with Ki-67 expression, and five radiomic features had a correlation coefficient >0.5. Few studies have been reported on radiomics analysis using SM features. Son et al. showed that assessing the radiomic features obtained from SM images could help detect triple-negative breast cancers using this technique and can be used to detect different subtypes (29).
The current study showed that the FFDM and SM models yielded equivalent AUC values of approximately 0.74. This result may not exactly have been satisfactory. Previous reports using FFDM images showed that a model using radiomic features extracted from FFDM exhibited an AUC value of 0.875 (17), and 0.862 (18) in the validation set. One of the reasons for not reaching sufficient results is the small data set which can lead to underfitting, where the model is not able to accurately capture the patterns and relationships in the data. With regard to SM, prediction for ALN was a difficult task. The image quality of SM to delineate morphological features is degraded through synthetic process. The development of a model to predict ALN with modified mammography is a difficult challenge. A previous study reported that a radiomics nomogram of contrast-enhanced spectral mammography yielded an AUC value of 0.68 in the validation set (19). The SM model achieved performance in predicting ALN metastasis comparable with that of the FFDM model. This result might support the claim that the radiomic features extracted from SM images are as informative as those from FFDM. The advantage of using DBT combined with SM images, which does not lead to additional radiation exposure by omitting FFDM examination, requires further validation.
In the current study, the sensitivity, specificity, PPV, and NPV of SM model was 0.783, 0.630, 0.474, and 0.871, respectively. A recent report described the diagnostic performance of traditional imaging method such as ultrasound, magnetic resonance imaging (MRI), and positron emission tomography (PET)/computed tomography (CT) with 18F-fluorodeoxyglucose (FDG) in detecting the ALN metastasis (30). The ultrasound results showed a sensitivity of 0.83, specificity of 0.62, PPV of 0.592, and NPV of 0.548. Meanwhile, the MRI results showed a sensitivity of 0.861, specificity of 0.75, PPV of 0.685, and NPV of 0.516. Lastly, the 18F-FDG-PET/CT results showed a sensitivity of 0.78, specificity of 0.53, PPV of 0.562, and NPV of 0.514. Compared to traditional imaging methods, the SM model had lower sensitivity, similar specificity, lower PPV, and higher NPV. The SM model can be combined with traditional imaging methods to enhance the overall accuracy of diagnoses.
This study has certain limitations. First, it is a preliminary study with a small dataset. A small dataset leads to under-training and inadequate accuracy in machine learning. Therefore, we used the LOOCV method as opposed to separating the dataset into training and validation datasets. Second, although frequent radiomic features were presented, the model explainability could not be reported because of the LOOCV method. Third, this was a single-centre study. Assessment of the generalisation performance using images from other institutions would be ideal. Fourth, the patients were not stratified based on risk. Hence, we were unable to specifically assess the ability to predict ALN metastasis in low-risk patients.
Conclusions
In conclusion, the ALN prediction model using radiomic features extracted from SM images demonstrated the possibility of enhancing the accuracy of diagnostic imaging when utilised together with traditional imaging techniques. To confirm the practicality of this method, further studies with a larger number of cases should be carried out.
Acknowledgments
We acknowledge the contribution of all members of our laboratory.
Funding: None.
Footnote
Reporting Checklist: The authors have completed the TRIPOD reporting checklist. Available at https://tcr.amegroups.com/article/view/10.21037/tcr-22-2668/rc
Data Sharing Statement: Available at https://tcr.amegroups.com/article/view/10.21037/tcr-22-2668/dss
Peer Review File: Available at https://tcr.amegroups.com/article/view/10.21037/tcr-22-2668/prf
Conflicts of Interest: All authors have completed the ICMJE uniform disclosure form (available at https://tcr.amegroups.com/article/view/10.21037/tcr-22-2668/coif). TH belongs to the endowed chair of Canon Medical Systems. KT obtained lecture fees from Pfizer Japan Inc. YK acquired research funding through Canon Medical Systems. The other authors have no conflicts of interest to declare.
Ethical Statement: The authors are accountable for all aspects of the work in ensuring that questions related to the accuracy or integrity of any part of the work are appropriately investigated and resolved. The study was conducted in accordance with the Declaration of Helsinki (as revised in 2013). This study was approved by Institutional Review Board of St. Marianna University (No. 5362). As our study was retrospective in nature with no personally identifiable information, the need for informed consent was waived.
Open Access Statement: This is an Open Access article distributed in accordance with the Creative Commons Attribution-NonCommercial-NoDerivs 4.0 International License (CC BY-NC-ND 4.0), which permits the non-commercial replication and distribution of the article with the strict proviso that no changes or edits are made and the original work is properly cited (including links to both the formal publication through the relevant DOI and the license). See: https://creativecommons.org/licenses/by-nc-nd/4.0/.
References
- Sung H, Ferlay J, Siegel RL, et al. Global Cancer Statistics 2020: GLOBOCAN Estimates of Incidence and Mortality Worldwide for 36 Cancers in 185 Countries. CA Cancer J Clin 2021;71:209-49. [Crossref] [PubMed]
- Cianfrocca M, Goldstein LJ. Prognostic and predictive factors in early-stage breast cancer. Oncologist 2004;9:606-16. [Crossref] [PubMed]
- Langer I, Guller U, Berclaz G, et al. Morbidity of sentinel lymph node biopsy (SLN) alone versus SLN and completion axillary lymph node dissection after breast cancer surgery: a prospective Swiss multicenter study on 659 patients. Ann Surg 2007;245:452-61. [Crossref] [PubMed]
- Lucci A, McCall LM, Beitsch PD, et al. Surgical complications associated with sentinel lymph node dissection (SLND) plus axillary lymph node dissection compared with SLND alone in the American College of Surgeons Oncology Group Trial Z0011. J Clin Oncol 2007;25:3657-63. [Crossref] [PubMed]
- Mansel RE, Fallowfield L, Kissin M, et al. Randomized multicenter trial of sentinel node biopsy versus standard axillary treatment in operable breast cancer: the ALMANAC Trial. J Natl Cancer Inst 2006;98:599-609. [Crossref] [PubMed]
- Kerlikowske K, Zhu W, Tosteson AN, et al. Identifying women with dense breasts at high risk for interval cancer: a cohort study. Ann Intern Med 2015;162:673-81. [Crossref] [PubMed]
- Wang L. Early Diagnosis of Breast Cancer. Sensors (Basel) 2017;17:1572. [Crossref] [PubMed]
- Heindel W, Weigel S, Gerß J, et al. Digital breast tomosynthesis plus synthesised mammography versus digital screening mammography for the detection of invasive breast cancer (TOSYMA): a multicentre, open-label, randomised, controlled, superiority trial. Lancet Oncol 2022;23:601-11. [Crossref] [PubMed]
- Friedewald SM, Rafferty EA, Rose SL, et al. Breast cancer screening using tomosynthesis in combination with digital mammography. JAMA 2014;311:2499-507. [Crossref] [PubMed]
- Gennaro G, Bernardi D, Houssami N. Radiation dose with digital breast tomosynthesis compared to digital mammography: per-view analysis. Eur Radiol 2018;28:573-81. [Crossref] [PubMed]
- European guidelines on breast cancer screening and diagnosis. Accessed 23 9 2022. Available online: https://healthcare-quality.jrc.ec.europa.eu/ecibc/european-breast-cancer-guidelines
- Alabousi M, Wadera A, Kashif Al-Ghita M, et al. Performance of Digital Breast Tomosynthesis, Synthetic Mammography, and Digital Mammography in Breast Cancer Screening: A Systematic Review and Meta-Analysis. J Natl Cancer Inst 2021;113:680-90. [Crossref] [PubMed]
- Heywang-Köbrunner SH, Jänsch A, Hacker A, et al. Digital breast tomosynthesis (DBT) plus synthesised two-dimensional mammography (s2D) in breast cancer screening is associated with higher cancer detection and lower recalls compared to digital mammography (DM) alone: results of a systematic review and meta-analysis. Eur Radiol 2022;32:2301-12. [Crossref] [PubMed]
- Lambin P, Rios-Velazquez E, Leijenaar R, et al. Radiomics: extracting more information from medical images using advanced feature analysis. Eur J Cancer 2012;48:441-6. [Crossref] [PubMed]
- Lambin P, Leijenaar RTH, Deist TM, et al. Radiomics: the bridge between medical imaging and personalized medicine. Nat Rev Clin Oncol 2017;14:749-62. [Crossref] [PubMed]
- Siviengphanom S, Gandomkar Z, Lewis SJ, et al. Mammography-based Radiomics in Breast Cancer: A Scoping Review of Current Knowledge and Future Needs. Acad Radiol 2022;29:1228-47. [Crossref] [PubMed]
- Yang J, Wang T, Yang L, et al. Preoperative Prediction of Axillary Lymph Node Metastasis in Breast Cancer Using Mammography-Based Radiomics Method. Sci Rep 2019;9:4429. [Crossref] [PubMed]
- Tan H, Wu Y, Bao F, et al. Mammography-based radiomics nomogram: a potential biomarker to predict axillary lymph node metastasis in breast cancer. Br J Radiol 2020;93:20191019. [Crossref] [PubMed]
- Mao N, Yin P, Li Q, et al. Radiomics nomogram of contrast-enhanced spectral mammography for prediction of axillary lymph node metastasis in breast cancer: a multicenter study. Eur Radiol 2020;30:6732-9. [Crossref] [PubMed]
- Fedorov A, Beichel R, Kalpathy-Cramer J, et al. 3D Slicer as an image computing platform for the Quantitative Imaging Network. Magn Reson Imaging 2012;30:1323-41. [Crossref] [PubMed]
- Ahmed M, Purushotham AD, Douek M. Novel techniques for sentinel lymph node biopsy in breast cancer: a systematic review. Lancet Oncol 2014;15:e351-62. [Crossref] [PubMed]
- Liu Q, Xing P, Dong H, et al. Preoperative assessment of axillary lymph node status in breast cancer patients by ultrasonography combined with mammography: A STROBE compliant article. Medicine (Baltimore) 2018;97:e11441. [Crossref] [PubMed]
- Hu Z, Cheng X, Li J, et al. Preliminary study of real-time three-dimensional contrast-enhanced ultrasound of sentinel lymph nodes in breast cancer. Eur Radiol 2020;30:1426-35. [Crossref] [PubMed]
- Chen CF, Zhang YL, Cai ZL, et al. Predictive Value of Preoperative Multidetector-Row Computed Tomography for Axillary Lymph Nodes Metastasis in Patients With Breast Cancer. Front Oncol 2019;8:666. [Crossref] [PubMed]
- Chinnappan S, Chandra P, Kumar S, et al. Prediction of Sentinel Lymph Node Biopsy Status in Breast Cancers with PET/CT Negative Axilla. World J Nucl Med 2022;21:120-6. [Crossref] [PubMed]
- Tan H, Gan F, Wu Y, et al. Preoperative Prediction of Axillary Lymph Node Metastasis in Breast Carcinoma Using Radiomics Features Based on the Fat-Suppressed T2 Sequence. Acad Radiol 2020;27:1217-25. [Crossref] [PubMed]
- Tagliafico AS, Valdora F, Mariscotti G, et al. An exploratory radiomics analysis on digital breast tomosynthesis in women with mammographically negative dense breasts. Breast 2018;40:92-6. [Crossref] [PubMed]
- Tagliafico AS, Bignotti B, Rossi F, et al. Breast cancer Ki-67 expression prediction by digital breast tomosynthesis radiomics features. Eur Radiol Exp 2019;3:36. [Crossref] [PubMed]
- Son J, Lee SE, Kim EK, et al. Prediction of breast cancer molecular subtypes using radiomics signatures of synthetic mammography from digital breast tomosynthesis. Sci Rep 2020;10:21566. [Crossref] [PubMed]
- Aktaş A, Gürleyik MG, Aydın Aksu S, et al. Diagnostic Value of Axillary Ultrasound, MRI, and (18)F-FDG-PET/ CT in Determining Axillary Lymph Node Status in Breast Cancer Patients. Eur J Breast Health 2021;18:37-47. [Crossref] [PubMed]